
Encyclopedia of Sociology Vol
.5.pdf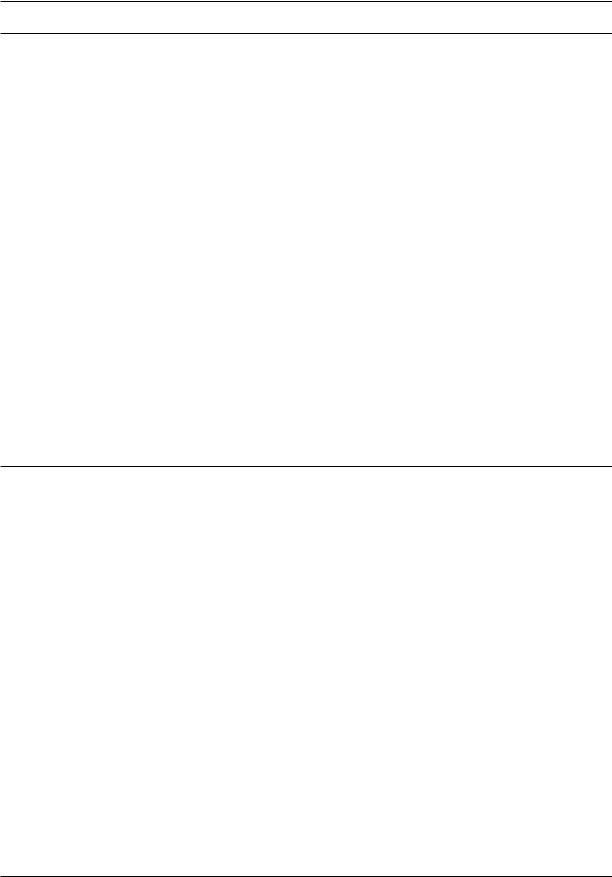
TABULAR ANALYSIS
Design Matrices for Row-Column Association Models for 3 × 3 Table
T |
|
A |
|
B |
C |
D |
E |
|
F |
|
G |
|
H |
|
I |
|
|
|
J |
|
|
|
|
|
|
|
|
C*D |
A*~D |
B*~D |
|
F+G |
|
(F~G)† |
|
|
A*~B |
|
|||
1 |
1 |
0 |
1 |
0 |
1 |
1 |
1 |
1 |
0 |
1 |
0 |
2 |
0 |
1 |
0 |
0 |
1 |
0 |
0 |
0 |
1 |
1 |
0 |
0 |
1 |
1 |
0 |
0 |
0 |
0 |
0 |
1 |
0 |
1 |
0 |
0 |
1 |
0 |
0 |
1 |
0 |
1 |
1 |
0 |
–1 |
–1 |
1 |
–1 |
–1 |
–1 |
0 |
–1 |
–1 |
–2 |
–1 |
–1 |
0 |
–1 |
–1 |
0 |
–1 |
0 |
1 |
0 |
1 |
1 |
0 |
0 |
1 |
0 |
0 |
1 |
0 |
0 |
0 |
1 |
0 |
1 |
0 |
0 |
1 |
0 |
0 |
1 |
0 |
1 |
0 |
1 |
0 |
0 |
0 |
0 |
0 |
0 |
0 |
0 |
0 |
0 |
0 |
0 |
0 |
0 |
0 |
1 |
1 |
0 |
1 |
–1 |
–1 |
0 |
–1 |
0 |
0 |
–1 |
0 |
0 |
0 |
–1 |
0 |
–1 |
0 |
0 |
–1 |
0 |
–1 |
1 |
–1 |
–1 |
1 |
0 |
–1 |
1 |
–1 |
–1 |
–1 |
–1 |
0 |
–2 |
–1 |
–1 |
–1 |
0 |
–1 |
–1 |
0 |
0 |
1 |
–1 |
–1 |
0 |
1 |
–1 |
0 |
0 |
0 |
0 |
0 |
–1 |
0 |
–1 |
0 |
0 |
–1 |
0 |
0 |
–1 |
–1 |
1 |
–1 |
–1 |
–1 |
–1 |
–1 |
–1 |
1 |
1 |
1 |
1 |
1 |
2 |
2 |
1 |
1 |
1 |
1 |
1 |
1 |
1 |
T: design vector for the constant term.
A:effect coding for row variable.
B:effect coding for column variable.
C:linear contrasts for row variable—(R + 1)/2 – i; any consecutive numbering will do; for variables with three categories, this is the same as the first code for the row variable.
D:linear contrasts for column variable—(C + 1)/2 –j.
E:design for the linear-by-linear interaction or uniform association, obtained by multiplying the linear contrast vector for the row and for the column.
F:design vectors for the row effects model, obtained by multiplying the design vectors for the row categories and the linear contrast vector for the column.
G:design vectors for column effects model, obtained by multiplying the design vectors for the column variable and the linear contrast for the row variable.
H:homogeneous row-column effects model, obtained by adding each vector in the matrix for the row and the corresponding vector in the matrix for the column.
I:row and column effects model—concatenation of F and G minus the redundant linear-by-linear interaction vector.
J: interaction vectors for saturated model, obtained by multiplying each vector in A with each vector in B.
Design matrix for each type of model is obtained by concatenating relevant vectors from above, and the degrees of freedom by number of cells in the table minus the number of columns in the design matrix.
|
Vectors |
df |
Independence model |
T~A~B |
4 |
Uniform association model |
T~A~B~E |
3 |
Row-effects model |
T~A~B~F |
2 |
Column-effects model |
T~A~B~G |
2 |
Homogeneous row–column effects model |
T~A~B~H |
2 |
Row and column effects model |
T~A~B~I |
1 |
Saturated model |
T~A~B~J |
0 |
Note: ~ (Horizontal concatenation); * (Multiplication); *~ (Horizontal direct product); † (Excluding redundant vector).
Table 6
obvious. Those parameters pertaining to each cell, denoted by tij, are equivalent to the product of the relevant part of the design matrix and the corresponding coefficients.
SOME EXTENSIONS
There are several ways in which one can extend the basic features of the log-linear models examined so far. Among these, the following three seem important: (1) utilizing the ANOVA-like structure
3121
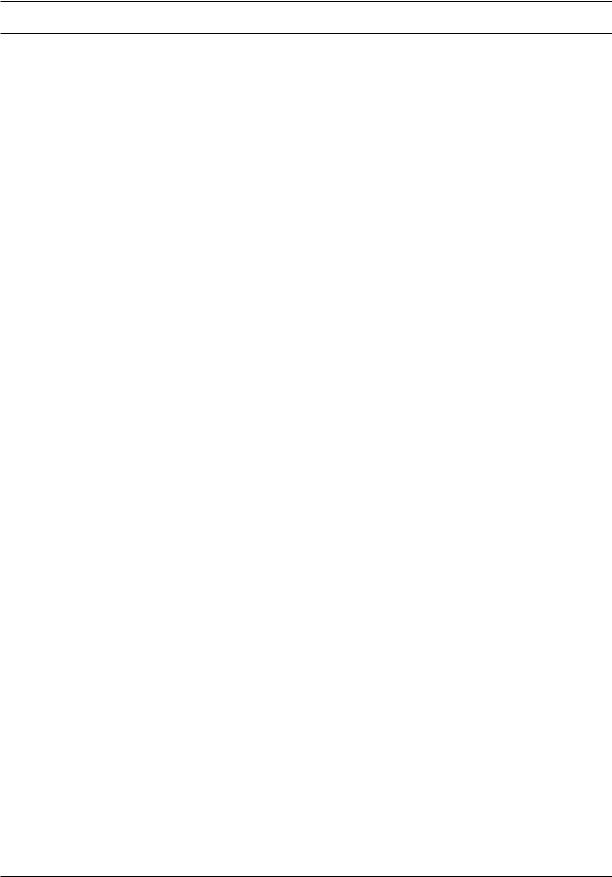
TABULAR ANALYSIS
Hypothetical Tables Illustrating Some Association Models
a) Row-Effects Association Model |
|
|
|
|
||
|
|
Frequency |
|
Odds Ratio |
|
|
|
|
X |
|
|
|
|
|
400 |
400 |
50 |
4 |
4 |
|
Y |
200 |
800 |
400 |
2 |
2 |
|
|
100 |
800 |
800 |
|
|
|
|
|
Log Parameters |
|
|
Multiplicative Parameter |
|
|
1.155 |
0 |
–1.555 |
3.175 |
1 |
.315 |
|
–.231 |
0 |
.231 |
.794 |
1 |
1.260 |
|
–.924 |
0 |
.924 |
.397 |
1 |
2.520 |
|
|
|
|
|
||
b) Homogeneous Row–Column Effects Model |
|
|
|
|
||
|
|
Frequency |
|
Odds Ratio |
|
|
|
|
X |
|
|
|
|
|
400 |
100 |
100 |
4 |
2 |
|
Y |
100 |
100 |
200 |
2 |
1 |
|
|
100 |
200 |
400 |
|
|
|
|
|
Log Parameters |
|
|
Multiplicative Parameters |
|
|
.924 |
–.231 |
–.693 |
2.520 |
.794 |
.500 |
|
–.231 |
0 |
.231 |
.794 |
1 |
1.260 |
|
–.693 |
.231 |
.462 |
.500 |
1.260 |
1.587 |
Table 7
of the log-linear model and the well-developed sampling theory to explore interaction patterns of multivariate categorical data, (2) manipulating the design matrices to examine more specific hypotheses and models, and (3) combining the strategic features of subgroup analysis and the flexibility and power of the log-linear models to produce more readily accessible analysis results. These three extensions are discussed below.
General Extension of Log-Linear Models. The most straightforward and widely used application of the log-linear model is to explore the interaction pattern of multivariate data by exploiting the ANOVA-like structure of the model. Given several variables to examine, especially when each variable contains more than two categories, it is almost impossible to examine the data structure in detail.
The ANOVA-like structure allows one to develop a convenient strategy to explore the existence of multiway relationships among the variables.
This strategy requires that one start with a design matrix for each variable (containing k-1 vectors, where k is the number of categories in the variable). It does not matter whether one uses dummy coding or effect coding. To examine all the possible interrelationships in the data, one needs design matrices corresponding to each twoway interaction to m-way interaction where m is the number of variables. To construct a design matrix for a two-way interaction between variable A and variable B, simply cross-multiply the design vectors for A with those for B. (This method is illustrated in Table 6.) This general approach to design matrices is extended to m-way. For example, a three-way
3122
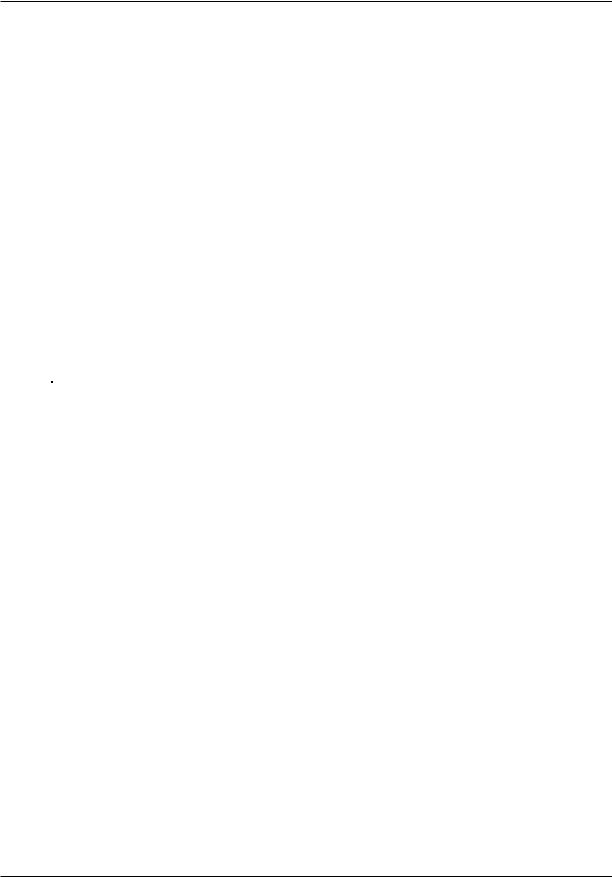
TABULAR ANALYSIS
interaction is handled by cross-multiplying each two-way vector with the basic design vectors for a third variable, and so on.
If one includes in the model all the vectors covering up to m-way interactions, the resulting model is saturated, and each frequency in the multiway table is completely described. In general, one wants to explore and, if possible, find a parsimonious way to describe the data structure. One general strategy, perhaps overused, is to examine systematically the hierarchical pattern inherent in the design constraints and serially examine a nested set of models. To illustrate, consider that there are three variables and that the basic design vectors for each variable are represented by A, B, and C, respectively. Let T stand for the constant vector. Then an example of a nested set of models is illustrated below. The commas indicate concatenation, and two or more letters together indicate cross-multiplication of the basic design vectors for each variable.
H1 : T
H2 : T , A, B,C
H3a : T , A, B,C , AB
H3b : T , A, B,C , AB, AC
H3c : T , A, B,C , AB, AC , BC
H4 : T , A, B,C , AB, AC , BC , ABC
(H 1) Equiprobability
(H 2) Total independence
(H 3a) One twoway interaction (H 3b) Two two - way interactions (H3c) No threeway interaction (H 4) Saturated model
Each hypothesis is tested, using the appropriate degrees of freedom, which is given by the number of the cells in the frequency table minus the number of vectors contained in the design matrix and the χ2 or L2 statistics associated with each model. The sequence from the hypotheses in set (3) is arbitrary; one may choose any nested set or directly examine 3c. One usually would accept the simplest hypothesis that is compatible with the data.
If variables contain many categories, even the simplest two-way interactions will use up many
degrees of freedom. This type of generic testing does not incorporate into the design matrix any special relationships that may exist between variables. Models of this type are routinely available in standard computer packages and therefore are quite accessible. For that reason, they are overused. Moreover, the sequential nature of the testing violates some of the assumptions of classical hypothesis testing. Nevertheless, in the hands of an experienced researcher, they become a flexible tool for exploring the multivariate data structure.
The Uses of Constrained Models. The flexibility and power of log-linear models are fully realized only when one incorporates a specific hypothesis about the data into the design matrices. There are virtually endless varieties one can consider. Some of the simple but strategic models of association were introduced in the preceding section.
Incorporating such models into a multivariate analysis is not difficult if one views the task in the context of design matrices. For instance, suppose one suspects that a certain pattern of relationship exists between X and Y (for instance, the social class of origin and destination in intergenerational mobility). Furthermore, one may have an additional hypothesis that these relationships vary systematically across different political systems (or across societies with different levels of economic development). If one can translate these ideas into appropriate design matrices, using such a model will provide a much more powerful test than the generic statistical models described in the previous section can provide. Many social mobility studies incorporate such design matrices as a way of incorporating a special pattern of social mobility in the overall design (for some examples, see Duncan 1979; Hout 1984; Yamaguchi 1987 and for new developments, see DiPrete 1990; Stier and Grusky 1990; Wong 1990, 1992, 1995; Xie 1992).
In general, there are two problems in using such design matrices. The first, which depends in part on the researcher’s creative ability, is the problem of translating theoretically relevant models into appropriate design matrices. The second is finding a way to obtain a good statistical solution for the model, but this is no longer much of a problem because of the wide availability of computer programs that allow the incorporation of design matrices (see Breen 1984 for a discussion of
3123
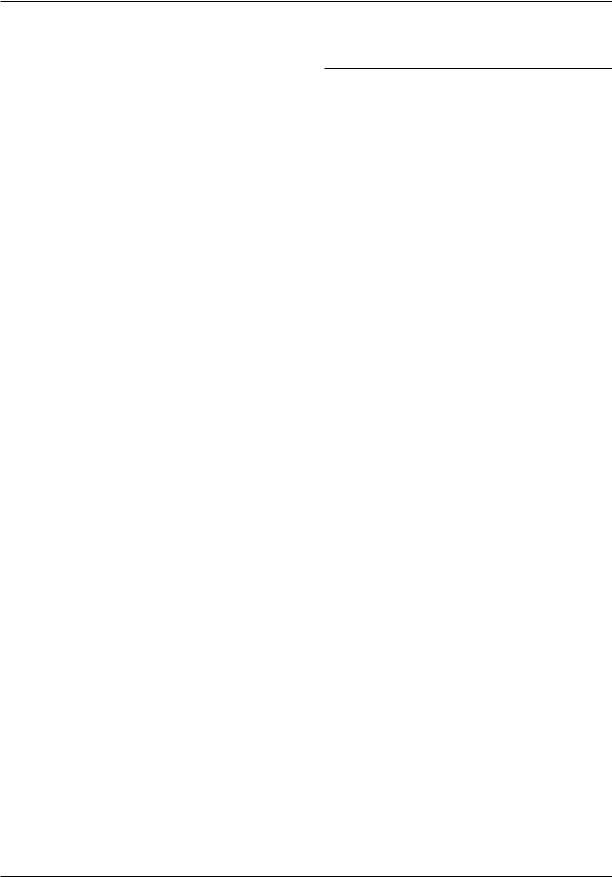
TABULAR ANALYSIS
preparing design matrices for a computer program that handles generalized linear systems).
One of the general problems has been that researchers often do not make the underlying design matrices explicit and as a result sometimes misinterpret the results. A solution for this problem is to think explicitly in terms of the design matrices, not in analogy to a generic (presumed) ANOVA model.
Use of Percentage Tables in Log-Linear Modeling. Multivariate analysis is in general complex. Categorical analysis is especially so, because one conceptual variable has to be treated as if it were (k- 1) variables, with k being the number of categories in the variable. Therefore, even with a limited number of variables, if each variable contains more than two categories, examining the multivariate pattern becomes extremely difficult. Therefore, the tendency is to rely on the general hypothesis testing discussed earlier.
It is useful to borrow two of the strategies of subgroup analysis: focusing on a bivariate relationship and using percentage distributions. After an acceptable log-linear model is identified, one therefore may display the relationship between two key variables while the effects of other variables are controlled or purged (Clogg 1978; Clogg and Eliason 1988a; Clogg et al. 1990; Kaufman and Schervish 1986). Furthermore, a percentage distribution for the bivariate distribution may be compared with the corresponding percentage distributions when different sets of variables are controlled in this manner. Fortunately, the log-linear modeling can provide a very attractive way in which the confounding effects of many variables can be purged from the relationship that is under special scrutiny (Clogg 1978; Kaufman and Schervish 1986; Clogg and Eliason 1988a; Clogg et al. 1990). Clogg et al. (1990) show a general framework under which almost all the known variations in adjustments can be considered a special case. Furthermore, they also describe statistical testing procedures for variety of statistics associated with such adjustments.
Tables 8 and 9 contain examples of traditional subgroup analysis, log-linear analysis, and the uses of standardization or purging methods. The upper panel of Table 8 contains a bivariate table showing
(1) the race of the defendant (X) and (2) the
Death Penalty Verdict (Y) by Defendant’s Race (X) and Victim’s Race (Z)
Death Penalty
Victim’s |
Defendant’s |
Yes |
No |
Percentage |
Race |
Race |
|
|
Yes |
|
|
|
|
|
Total |
White |
53 |
430 |
11.0 |
|
Black |
15 |
176 |
7.9 |
White |
White |
53 |
414 |
11.3 |
|
Black |
11 |
37 |
22.9 |
Black |
White |
0† |
16 |
0.0 |
|
Black |
4 |
139 |
2.8 |
Table 8
SOURCE: Radelet and Pierce (1991), p. 25, and Agresti (1996), p. 54.
NOTE: (1) For log-linear analysis, 0.5 is added to zero cell. (2) In the original data, there are two cases that involve both white and black victims. (3) The data do not consistently identify Spanish ancestry. Most defendants and victims with Spanish ancestry are coded as white. For detailed information, see Radelet and Pierce (1991).
verdict—death penalty versus other penalties (Y), while the lower panel contains the result of traditional three-variables subgroup analysis, in which the original relationship between X and Y is reanalyzed within the categories of the third variable, the race of the victims (Z). (These data are based on individuals who were convicted of multiple homicides in Florida. See Radelet and Peierce 1991; Agresti 1996.)
The original bivariate relationship seems to indicate that whites are more likely to receive the death penalty than are blacks. However, when the race of the victims is controlled, the partial relationship between X and Y is reversed: Within each category of victim, blacks are more likely to receive the death penalty than are whites. The underlying reasons for this reversal are two related facts: (1) There is a strong association between the race of the defendant (X) and the race of the victims (Z): white defendants are more likely to kill whites than blacks, while black defendants are more likely to kill blacks than whites, and (2) there is a strong relationship between the race of the victims and the death penalty: those who killed white victims are more likely to receive the death penalty than are those who killed blacks. Once these relation-
3124

TABULAR ANALYSIS
Design Matrix, Expected Frequencies, and Standardized Percentages under the Model without Three-Way Interaction
a) Design Matrix and Coefficients for the Model without Three-Way Interaction |
|
|
|
|||||||
T |
Z |
X |
Y |
XZ |
YZ |
XY |
Parameter |
Coefficient |
z-value |
|
1 |
1 |
1 |
1 |
1 |
1 |
1 |
Constant (T) |
2.959 |
21.0 |
|
1 |
1 |
1 |
–1 |
1 |
–1 |
–1 |
Z |
1.039 |
6.9 |
|
1 |
1 |
–1 |
1 |
–1 |
1 |
–1 |
X |
–0.135 |
–1.3 |
|
1 |
1 |
–1 |
–1 |
–1 |
–1 |
1 |
Y |
–1.382 |
–11.1 |
|
1 |
–1 |
1 |
1 |
–1 |
–1 |
1 |
XZ |
1.137 |
14.7 |
|
1 |
–1 |
1 |
–1 |
–1 |
1 |
–1 |
YZ |
0.558 |
3.9 |
|
1 |
–1 |
–1 |
1 |
1 |
–1 |
–1 |
XY |
–0.201 |
–2.2 |
|
1 |
–1 |
–1 |
–1 |
1 |
1 |
1 |
L2 = 0.284 |
df =1 |
Prob. = 0.594 |
|
b) Expected Frequencies under the Model without Three-Way Interaction |
|
|
|
|||||||
|
|
|
|
|
|
|
Death Penalty |
|
|
|
|
Victim’s Race |
Defendant’s Race |
|
Yes |
No |
Percentage Yes |
||||
|
Total |
|
|
White |
|
|
53.5 |
430.0 |
11.1 |
|
|
|
|
|
Black |
|
|
15.0 |
176.0 |
7.9 |
|
|
|
|
|
|
|
|
|
|
|
|
|
White |
|
|
White |
|
|
53.3 |
413.7 |
11.4 |
|
|
|
|
|
Black |
|
|
10.7 |
37.3 |
22.3 |
|
|
Black |
|
|
White |
|
|
0.2 |
16.3 |
1.2 |
|
|
|
|
|
Black |
|
|
4.3 |
138.7 |
3.0 |
|
c) Direct Standardization |
|
|
|
|
|
|
|
|||
|
Total |
|
|
White |
|
|
35.87 |
447.62 |
7.4 |
|
|
|
|
|
Black |
|
|
28.16 |
162.84 |
14.7 |
|
|
|
|
|
|
|
|
|
|
|
|
|
White |
|
|
White |
|
|
33.58 |
260.67 |
11.4 |
|
|
|
|
|
Black |
|
|
25.91 |
90.33 |
22.3 |
|
|
Black |
|
|
White |
|
|
2.29 |
186.95 |
1.2 |
|
|
|
|
|
Black |
|
|
2.25 |
72.51 |
3.0 |
|
d) Purging XZ (= purging XZ and XYZ)
Total |
White |
17.7 |
183.2 |
8.8 |
|
Black |
34.9 |
160.9 |
17.8 |
White |
White |
17.0 |
132.3 |
11.4 |
|
Black |
33.5 |
116.6 |
22.3 |
Black |
White |
0.7 |
50.9 |
1.4 |
|
Black |
1.4 |
44.4 |
3.1 |
e) Purging XZ and YZ (= purging XZ, YZ, and XYZ)
Total |
White |
11.0 |
260.9 |
4.0 |
|
Black |
21.5 |
228.7 |
8.6 |
|
|
|
|
|
White |
White |
9.8 |
231.9 |
4.0 |
|
Black |
19.1 |
203.2 |
8.6 |
Black |
White |
1.2 |
29.0 |
4.0 |
|
Black |
2.4 |
25.4 |
8.6 |
Table 9
3125

TABULAR ANALYSIS
ships are taken into consideration, blacks receive a higher rate of the death penalty than do whites.
The log-linear analysis can supplement such a traditional subgroup analysis in several convenient ways. Panel (a) of Table 9 shows design matrix, coefficients, and standardized values under the model without three-way interaction. These statistics show several things that are not obvious in the conventional subgroup analysis: (1) The three-way interaction is not statistically significant, (2) all three bivariate relationships are statistically significant, and (3) in some sense, the association between X and Z is the strongest and that between X and Y is the weakest among the three bivariate relationships.
Panel (b) shows expected frequencies and relevant percentages under the model (where the three-way interaction is assumed to be zero). The pattern revealed in each subtable is very similar to that under the traditional subgroup analysis shown in Table 8. (This is as it should be, given no threeway interaction effect.) Within each category of victim’s race, black defendants are more likely to receive the death penalty than are white defendants. The standardization or purging, then, allows one to summarize this underlying relationship between X and Y under the hypothetical condition that the effect of the third variable is controlled or purged. There are many different ways of controlling the effects of the third variable:
(1) direct standardization, panel (c), (2) when the effects of XZ relationship are purged (in addition to the purging of the three-way interaction) panel (d), (3) when, in addition to the previous purging, the effects of the YZ relationship are also purged, panel (e). Although the percentage differences seem to vary, the underlying log-linear effect remains constant: Blacks are twice more likely to receive the death penalty than are whites when both kill a victim of the same race (see Clogg 1978; Clogg and Eliason 1988b; Clogg et al. 1990).
(SEE ALSO: Analysis of Variance and Covariance; Causal Inference Models; Measures of Association; Nonparametric Statistics; Statistical Methods)
REFERENCES
Agresti, Alan 1983 ‘‘A Survey of Strategies for Modeling Cross-Classifications Having Ordinal Variables.’’ Journal of the American Statistical Association 78:184–198.
———1984 Analysis of Ordinal Categorical Data. New York: Wiley.
———1990 Categorical Data Analysis. New York: Wiley.
———1996 An Introduction to Categorical Data Analysis. New York: Wiley.
Alba, Richard D. 1988 ‘‘Interpreting the Parameters of Log-Linear Models.’’ In J. Scott Long, ed., Common Problems/Proper Solutions. Beverly Hills, Calif.: Sage.
Alwin, Dwane F., and Robert M. Hauser 1975 ‘‘The Decomposition of Effects in Path Analysis.’’ American Sociological Review 40:37–47.
Anderson, J. A. 1984 ‘‘Regression and Ordered Categorical Variables.’’ Journal of the Royal Statistical Society B46:1–30.
Arminger, G., and G. W. Bohrnstedt 1987 ‘‘Making It Count Even More: A Review and Critique of Stanley Lieberson’s Making It Count: The Improvement of Social Theory and Research.’’ Sociological Methodology
17:347–362.
Berk, R. A. 1983 ‘‘An Introduction to Sample Selection Bias in Sociological Data.’’ American Sociological Review 48:386–398.
——— 1986 ‘‘Review of Making It Count: The Improvement of Social Research and Theory.’’ American Journal of Sociology 92:462–465.
Bishop, Yvonne M. M., Stephen E. Fienberg, and Paul W. Holland 1975 Discrete Multivariate Analysis: Theory and Practice. Cambridge, Mass.: MIT Press.
Blalock, Hubert M., Jr. 1964 Causal Inferences in Nonexperimental Research. Chapel Hill: University of North Carolina Press.
———, ed. 1985a Causal Models in the Social Sciences, 2nd ed. New York: Aldine.
———, ed. 1985b Causal Models in Panel and Experimental Designs. New York: Aldine.
Bollen, K. A. 1989 Structural Equations with Latent Variables. New York: Wiley.
Breen, Richard 1984 ‘‘Fitting Non-Hierarchical and Association Models Using GLIM.’’ Sociological Methods and Research 13:77–107.
Bunge, Mario 1979 Causality and Modem Science, 3rd rev. ed. New York: Dover.
Campbell, D. T., and J. C. Stanley 1966 Experimental and Quasi-Experimental Designs for Research. Boston: Houghton Mifflin.
Clogg, Clifford C. 1978 ‘‘Adjustment of Rates Using Multiplicative Models.’’ Demography 15:523–539.
——— 1982a ‘‘Using Association Models in Sociological Research: Some Examples.’’ American Journal of Sociology 88:114–134.
3126
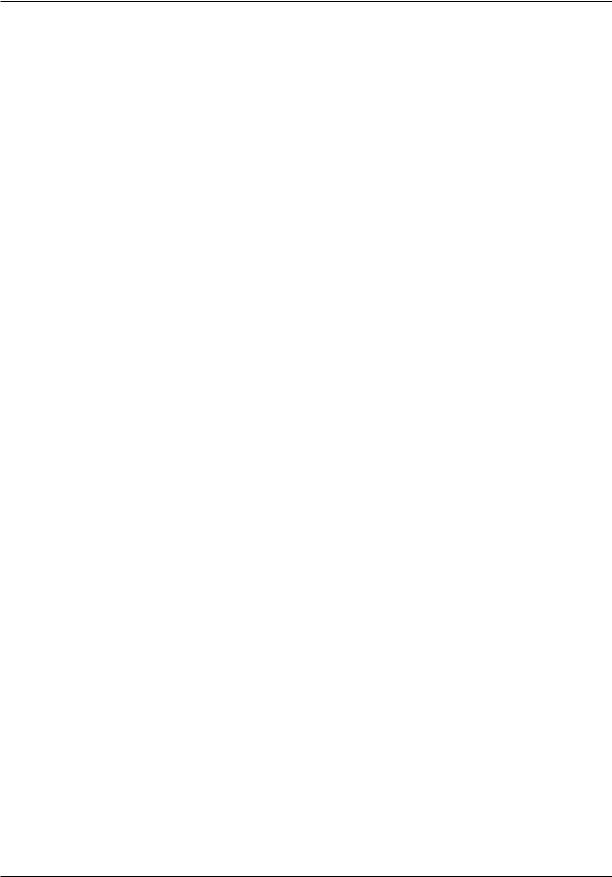
TABULAR ANALYSIS
——— 1982b ‘‘Some Models for the Analysis of Association in Multiway Cross-Classifications Having Ordered Categories.’’ Journal of the American Statistical Association 77:803–815.
———, and Scott R. Eliason 1988a ‘‘A Flexible Procedure for Adjusting Rates and Proportions, Including Statistical Methods for Group Comparisons.’’ American Sociological Review 53:267–283.
——— 1988b ‘‘Some Common Problems in Log-Linear Analysis.’’ In J. Scott Long, ed., Common Problems/ Proper Solutions. Beverly Hills, Calif.: Sage.
———, and Edward S. Shihadeh 1994 Statistical Models for Ordinal Variables. Thousand Oaks, Calif.: Sage.
———, James W. Shockey, and Scott R. Eliason 1990 ‘‘A General Statistical Framework for Adjustment of Rates.’’ Sociological Methods and Research 19:156–195.
Cook, Thomas D., and Donald T. Campbell 1979 QuasiExperimentation: Design and Analysis Issues for Field Settings. Chicago: Rand McNally.
Davis, James A. 1984 ‘‘Extending Rosenberg’s Technique for Standardizing Percentage Tables.’’ Social Forces 62:679–708.
———, and Ann M. Jacobs 1968 ‘‘Tabular Presentations.’’ In David L. Sills, ed., The International Encyclopedia of the Social Sciences, vol.15. New York: Macmillan and Free Press.
Duncan, Otis Dudley 1966 ‘‘Path Analysis: Sociological Examples.’’ American Journal of Sociology 72:1–16.
———1975 Introduction to Structural Equation Models. New York: Academic Press.
———1979 ‘‘How Destination Depends on Origin in the Occupational Mobility Table.’’ American Journal of Sociology 84:793–803.
Fienberg, Stephen E. 1980 The Analysis of Cross-Classified Data, 2nd ed. Cambridge, Mass.: MIT Press.
Fisher, F. M. 1966 The Identification Problem in Econometrics. New York: McGraw-Hill.
Fleiss, J. L. 1981 Statistical Methods for Rates and Proportions,2nd ed. New York: Wiley Interscience.
Goldberger, Arthur S., and Otis Dudley Duncan (eds.) 1973 Structural Equation Models in the Social Sciences. New York and London: Seminar Press.
Goodman, Leo A. 1978 Analyzing Qualitative/Categorical Data: Log-Linear Analysis and Latent Structure Analysis. Cambridge, Mass.: Abt.
——— 1981 ‘‘Three Elementary Views of Loglinear Models for the Analysis of Cross-Classifications Having Ordered Categories.’’ In Karl F. Schuessler, ed., Sociological Methodology. San Francisco: Jossey-Bass.
———1984 The Analysis of Cross-Classified Categorical Data Having Ordered Categories. Cambridge, Mass.: Harvard University Press.
———1985 ‘‘The Analysis of Cross-Classified Data Having Ordered and/or Unordered Categories: Association Models, Correlation Models, and Asymmetry Models for Contingency Tables with or without Missing Entries.’’ Annals of Statistics 13:10–69.
———1987 ‘‘The Analysis of a Set of Multidimensional Contingency Tables Using Log-Linear Models, Latent Class Models, and Correlation Models: The Solomon Data Revisited.’’ In A. E. Gelfand, ed.,
Contributions to the Theory and Applications of Statistics: A Volume in Honor of Herbert Solomon. New York: Academic Press.
———1990 ‘‘Total-Score Models and Rasch-Type Models for the Analysis of a Multidimensional Contingency Table, or a Set of Multidimensional Contingency Tables, with Specified and/or Unspecified Order for Response Categories.’’ In Karl F. Schuessler, ed., Sociological Methodology. San Francisco: Jossey-Bass.
Haberman, Shelby J. 1978 Analysis of Qualitative Data, vol. 1: Introductory Topics. New York: Academic Press.
——— 1979 Analysis of Qualitative Data, vol. 2: New Developments.New York: Academic Press.
Hausman, J. A. 1978 ‘‘Specification Tests in Econometrics.’’ Econometrica 46:1251–1272.
Heckman, J. J. 1979 ‘‘Sample Selection Bias as a Specification Error.’’ Econometrica 47 153–161.
———, and R. Robb 1986 ‘‘Alternative Methods for Solving the Problem of Selection Bias in Evaluating the Impact of Treatments on Outcomes.’’ In H. Wainer, ed., Drawing Inferences from Self-Selected Samples.New York: Springer-Verlag.
Heise, David R. 1975 Causal Analysis. New York: Wiley.
Hout, Michael 1984 ‘‘Status, Autonomy, Training in Occupational Mobility.’’ American Journal of Sociology
89:1379–1409.
Hyman, Herbert 1955 Survey Design and Analysis: Principles, Cases and Procedures. Glencoe, Ill.: Free Press.
Kaufman, Robert L., and Paul G. Schervish 1986 ‘‘Using Adjusted Crosstabulations to Interpret Log-Linear Relationships.’’ American Sociological Review 51:717–733.
Kendall, Patricia L., and Paul Lazarsfeld 1950 ‘‘Problems of Survey Analysis.’’ In Robert K. Merton and Paul F. Lazarsfeld, eds., Continuities in Social Research: Studies in the Scope and Method of the American Soldier. Glencoe, Ill.: Free Press.
Kish, Leslie 1959 ‘‘Some Statistical Problems in Research Design.’’ American Sociological Review 24:328–338.
3127

TERRITORIAL BELONGING
Lazarsfeld, Paul F. 1955 ‘‘Interpretation of Statistical Relations as a Research Operation.’’ In Paul F. Lazarsfeld and Morris Rosenberg, eds., The Language of Social Research. Glencoe, Ill.: Free Press.
———, Ann K. Pasanella, and Morris Rosenberg, eds. 1972 Continuities in the Language of Social Research. New York: Free Press.
Leamer, E. E. 1978 Specification Searches: Ad Hoc Inference with Nonexperimental Data. New York: Wiley Interscience.
Lieberson, Stanley 1985 Making It Count: The Improvement of Social Research and Theory. Berkeley and Los Angeles: University of California Press.
Long, J. Scott 1984 ‘‘Estimable Functions in Loglinear Models.’’ Sociological Methods and Research 12:399–432.
———, ed. 1988 Common Problems/Proper Solutions: Avoiding Error in Quantitative Research. Beverly Hills, Calif.: Sage.
Mare, Robert D., and Christopher Winship 1988 ‘‘Endogenous Switching Regression Models for the Causes and Effects of Discrete Variables.’’ In J. Scott Long, ed., Common Problems/Proper Solutions. Beverly Hills, Calif: Sage.
McCullagh, P. 1978 ‘‘A Class of Parametric Models for the Analysis of Square Contingency Tables with Ordered Categories.’’ Biometrika 65:413–418.
———, and J. Nelder 1983 Generalized Linear Models. London: Chapman and Hall.
Mosteller, F. 1968 ‘‘Association and Estimation in Contingency Tables.’’ Journal of the American Statistical Association 63:1–28.
———, and John W. Tukey 1977 Data Analysis and Regression. Reading, Mass.: Addison-Wesley.
Nelder, J. A., and R. W. M. Wedderburn 1972 ‘‘Generalized Linear Models.’’ Journal of the Royal Statistical Society A135:370–384.
Plackett, R. L. 1974 The Analysis of Categorical Data. London: Griffin.
Press, S. L., and S. Wilson 1978 ‘‘Choosing between Logistic Regression and Discriminant Analysis.’’ Journal of the American Statistical Association 73:699–705.
Radelet, Michael I., and Glenn L. Pierce 1991 ‘‘Choosing Those Who Will Die: Race and the Death Penalty in Florida.’’ Florida Law Review 43:1–34.
Rosenberg, Morris 1968 The Logic of Survey Analysis. New York: Basic Books.
Rubin, D. B. 1977 ‘‘Assignment to Treatment Group on the Basis of a Covatiance.’’ Journal of Educational Statistics 2:1–26.
Simon, Herbert A. 1954 ‘‘Spurious Correlation: A Causal Interpretation.’’ Journal of the American Statistical As- sociation49:467–479.
——— 1979 ‘‘The Meaning of Causal Ordering.’’ In Robert K. Merton, James S. Coleman, and Peter H. Rossi, eds., Qualitative and Quantitative Social Research: Papers in Honor of Paul F. Lazarsfeld. New York: Free Press.
Singer, Burton, and Margaret Mooney Marini 1987 ‘‘Advancing Social Research: An Essay Based on Stanley Lieberson’s Making It Count.’’ In Clifford C. Clogg, ed., Sociological Methodology. Washington, D.C.: American Sociological Association.
Thiel, Henri 1971 Principles of Econometrics New York: Wiley.
Williams, D. A. 1976 ‘‘Improved Likelihood Ratio Tests for Complete Contingency Tables.’’ Biometrika 63:33–37.
Xie, Yu 1989 ‘‘An Alternative Purging Method: Controlling the Composition-Dependent Interaction in an Analysis of Rates.’’ Demography 26:711–716.
Yamaguchi, Kazuo 1987 ‘‘Models for Comparing Mobility Tables: Toward Parsimony and Substance.’’ American Sociological Review 52:482–494.
Zeisel, Hans 1985 Say It with Figures, 6th ed. New York: Harper & Row.
JAE-ON KIM
MYOUNG-JIN LEE
TECHNOLOGICAL RISKS AND SOCIETY
See Society and Technological Risks.
TERRITORIAL BELONGING
DEFINITION
Belonging is defined as the state of being part of something. Territorial belonging implies being part of a territory. The definition of a territory, although it is conditioned by the morphology of space, is essentially a social operation that is connected with the factors that induce the perception of boundaries. These are complex factors that researchers in the ‘‘psychology of form’’ (Gestaltpsychologie) have attempted to specify (Reusch 1956, pp. 340–361). Campbell has identified seven
3128

TERRITORIAL BELONGING
of these factors. Those analytically most relevant to social systems are similarity and shared destiny or ‘‘common fate’’ (Campbell 1958), to which the ecological, economic, and sociological traditions (Hawley 1950, p. 258) add interdependence, which is related to Campbell’s (1958) notion of internal diffusion.
Territorial belonging is therefore a form of social belonging (for a detailed treatment, see Pollini 1987) that is displayed by a spatially defined collectivity. Spatial definition more or less precisely and more or less sharply delimits (where the concept of a boundary refers to a line or zone) a territory to which a name is given. Belonging to a spatially defined collectivity thus may be related to the name given to a territory, so that it becomes simply territorial without ceasing to be social as well. To emphasize its twofold nature, it also may be called ‘‘socioterritorial belonging’’ (Pollini 1992, pp. 55–58).
THE MULTIPLICITY OF TERRITORIAL
BELONGINGS
Like any form of social belonging, territorial belonging may relate to objective or subjective elements and may be defined by the self or by others (Merton 1963). Like social belonging, it may be largely exclusive or admit to multiplicity and may be ascribed or acquired (Simmel 1908).
Workers in human ecology, human geography, sociology, and land economics have long attempted to provide a definition of the most suitable territorial units for social purposes. They have oscillated between emphasizing the principle of similarity (the morphology of the territory, the physical and cultural features of the individuals who inhabit it, the predominant type of economic activity, etc.) and emphasizing the principle of interdependence (on the basis of gravitational flows for work and services, areas of relatively intense exchange, etc.) (see Galtung 1968). The implementation of the political function of governing human communities, moreover, has led to the fixing of territorial boundaries that express (and produce) a common fate (Hawley 1950, p. 258).
Reciprocal relations are among the main criteria used to define territorial units (similarity, interdependence, and common fate, taking the proximity of elements for granted). As studies of ‘‘nation building’’ have shown (see Deutsch [1953] 1966), similarity tends to create relations of interdependence and interdependence generates perceptions of similarity (Simmel 1890, p. 40; Shils 1975, p. 17); in the same manner, a common fate induces perceptions of similarity and interdependence and similarity and interdependence heighten the perception of a common fate.
The most enduring and significant spatial units are those with multi-confirmed boundaries (Campbell 1958), that is, those for which the criteria of similarity, functional interdependence, and common fate are congruent. The European nationstates of the nineteenth and twentieth centuries exemplify the successful achievement of this congruence (Eisenstadt 1973, pp. 231–235).
In traditional nomadic and agricultural societies, similarity, interdependence, and common fate are properties that relate substantially to a single socioterritorial unit that includes everyday life almost in its entirety, except when extraordinary occasions (great feasts and celebrations, great markets, wars, etc.) demonstrate the importance of a broader socioterritorial unit that is ethnic and/or tribal in nature or sometimes is of state dimension (kingdoms and empires, churches and great religious organizations). This is the social order that is called the ‘‘segmentary society of mechanical solidarity’’ by Durkheim (1893), Gemeinschaft by Toennies (1887), and the ‘‘independent community’’ by Hawley (1950, pp. 223 ff) and is exemplified by numerous contemporary societies (Dyson Hudson 1966).
As a significant division of territorial labor develops—induced by the reduced spatial friction brought about by advances in transport and communications that allow more frequent exchanges over longer distances (the ‘‘mobiletic revolution’’ of Russet 1967) and by technical progress, which requires the greater accumulation of capital and is not uniformly distributed across the territory (industrial revolution)—the areas of interdependence
3129
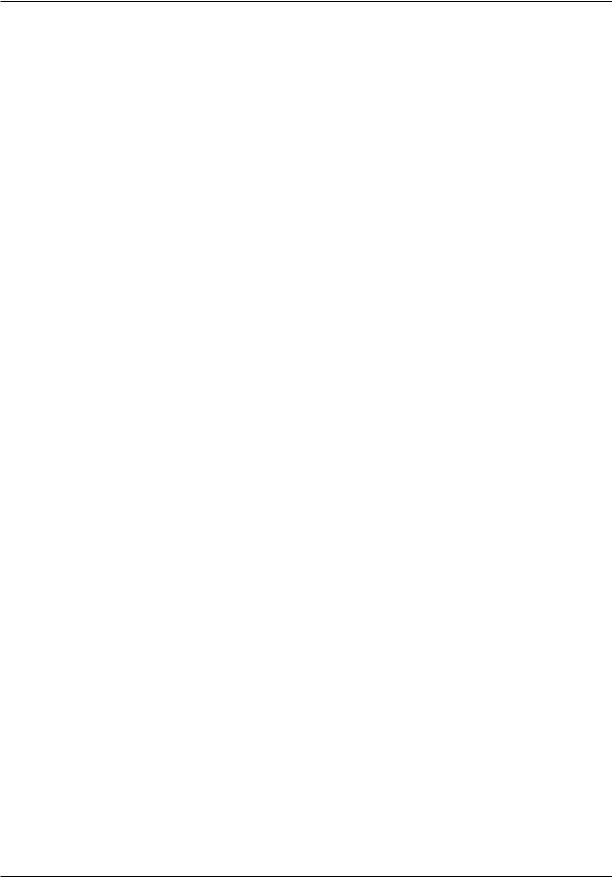
TERRITORIAL BELONGING
expand and are structured into several levels (Hawley 1950, pp. 236–257). This has evident effects on areas of common fate. Increased interdependence facilitates temporary or permanent movements across the territory and thus alters similarities and differences as well as the criteria for their definition and perception (Sola Pool 1965).
In short, mainly as a result of these phenomena, a socioterritorial structure grows more complex. Important socioterritorial units proliferate, intersect, and are organized into larger (Parsons 1961, pp. 123 ff) and relatively fluid systems, while the congruence among the three main principals of sociospatial structuring diminishes. These changes have been interpreted as the decay of Gemeinschaft (Toennies 1887) and of the territorial state (Herz 1957), as stages in an ongoing evolution into cosmopolitanism, and as the onset of a single overarching socioterritorial unit: the world in its entirety. Parsons (1951) introduced in his theoretical pattern of variables for the definition of roles the dichotomy between particularism and universalism. Although of general analytic significance, this dichotomy also has been used to characterize the process of modernization (Parsons 1971), which, with reference to territorial belonging, includes the localism–cosmopolitanism dichotomy. The term ‘‘globalization,’’ which now has general currency although it dates from the early 1970s (Kaufman 1974), has been employed more recently to define this trend.
The increased complexity of socioterritorial belonging is obviously correlated to the complexity of the social structuring of the territory. All individuals are involved in relational networks that may be micro-local (habitation), local, regional, national, continental, and global, and they shift easily and rapidly from one level to another by virtue of the ease of communications and transportation or simply pass subjectively from one role to another (Webber 1963, 1964).
Owing to the ease of communications and transport, every individual may encounter and assimilate elements of other cultures. Cultural diversity has dwindled before the advance of modern culture as it is interpreted by Western society.
Intellectual elements (‘‘scientific’’ criteria for the reliability of knowledge) and most emotional (aspirations and values) and evaluative ones (ethics and hierarchies of values) are widely shared by humankind (Inglehart 1997). Consequently, similarities and differences are difficult to define in territorial terms, and when they are thus definable, they are increasingly so only as symbols (languages, flags, cultural artifacts, physical resemblances, etc.), since knowledge-evoking and evaluative elements have been reduced to being options that are private, individual, and socially irrelevant (ethical and gnoseological relativism, individualism) (Thomas and Znaniecki 1918–1920; Halman, et al. 1987).
The state itself, which is called on to define the conditions of the collective control of the collective destiny, although it is still characterized by distinct territorial boundaries, is undergoing profound change as a result of the erosion of its sovereignty and power by the rise of supranational political organizations (e.g., the United Nations) and, in centralized states, infrastate political organizations (Galtung 1967), as well as by the growth of multinational economic enterprises and other noneconomic associations over which it can exert little or no control.
Consequently, if the continuing determination of the territorial boundaries of political and/or administrative units (Herz 1968) allows belongings to be related to them, those belongings grow increasingly less socially significant in regards to not only interdependencies and similarities but also common destiny. This occurs because social relevance is divided among several units organized into a system of relationships that need not be hierarchical and may indeed compete with each other.
TERRITORIAL BELONGING SUBJECTIVELY DEFINED BY REFERENCE TO THE SELF (THE SENTIMENT OF TERRITORIAL BELONGING)
The localism–cosmopolitanism of territorial belonging: A single-or multidimensional concept?
The growing complexity of territorial belonging,
3130