
guide2008_en
.pdf
Table 4.66 Economic analysis (thousands of Euros)
|
CF |
1 |
2 |
3 |
4 |
5 |
6 |
7 |
8 |
9 |
10 |
|
|||||||||||
Product A |
1.000 |
0 |
1,200 |
1,800 |
3,060 |
4,766 |
4,934 |
5,108 |
5,287 |
5,473 |
5,665 |
Product B |
1.000 |
0 |
750 |
1,050 |
1,680 |
2,206 |
2,272 |
2,341 |
2,412 |
2,485 |
2,534 |
Product C |
1.000 |
0 |
2,400 |
3,840 |
6,912 |
20,798 |
27,119 |
27,744 |
28,384 |
29,038 |
29,708 |
SALES |
|
0 |
4,350 |
6,690 |
11,652 |
27,770 |
34,325 |
35,193 |
36,083 |
36,996 |
37,907 |
Raw materials |
0.950 |
0 |
2,108 |
3,241 |
5,646 |
13,455 |
16,631 |
17,051 |
17,482 |
17,925 |
18,366 |
Labour |
0.600 |
0 |
177 |
492 |
851 |
861 |
871 |
881 |
892 |
902 |
913 |
Electricity |
0.970 |
0 |
173 |
273 |
486 |
1,185 |
1,499 |
1,570 |
1,645 |
1,723 |
1,801 |
Fuel |
0.970 |
0 |
224 |
364 |
666 |
1,670 |
2,164 |
2,321 |
2,485 |
2,656 |
2,831 |
Maintenance |
1.000 |
0 |
131 |
201 |
350 |
833 |
1,030 |
1,056 |
1,082 |
1,110 |
1,137 |
General industrial costs |
1.000 |
0 |
124 |
181 |
297 |
666 |
772 |
739 |
704 |
666 |
625 |
Administrative costs |
1.000 |
0 |
126 |
187 |
315 |
722 |
858 |
845 |
830 |
814 |
796 |
Sales expenditure |
1.000 |
0 |
114 |
173 |
297 |
647 |
781 |
802 |
823 |
844 |
865 |
TOTAL OPERATING COSTS |
|
0 |
3,177 |
5,112 |
8,908 |
20,040 |
24,606 |
25,266 |
25,943 |
26,640 |
27,335 |
RETIREMENT BONUS |
1,000 |
0 |
0 |
0 |
0 |
0 |
0 |
0 |
0 |
0 |
0 |
Land |
1,235 |
3,705 |
0 |
0 |
0 |
0 |
0 |
0 |
0 |
0 |
0 |
Buildings |
0.715 |
4,290 |
4,290 |
3,575 |
0 |
0 |
0 |
0 |
0 |
0 |
0 |
New Equipment |
0.990 |
9,900 |
13,860 |
17,820 |
0 |
0 |
0 |
0 |
0 |
0 |
0 |
Used Equipment |
0,990 |
0 |
0 |
0 |
0 |
0 |
0 |
0 |
0 |
0 |
0 |
Extraordinary Maintenance |
0,756 |
0 |
0 |
0 |
0 |
0 |
0 |
0 |
0 |
0 |
0 |
Fixed Assets |
|
17,895 |
18,150 |
21,395 |
0 |
0 |
0 |
0 |
0 |
0 |
0 |
Licenses |
1.000 |
0 |
0 |
500 |
0 |
0 |
0 |
0 |
0 |
0 |
0 |
Patents |
1.000 |
0 |
0 |
500 |
0 |
0 |
0 |
0 |
0 |
0 |
0 |
Other pre-prod. expenses |
1.000 |
0 |
0 |
0 |
0 |
0 |
0 |
0 |
0 |
0 |
0 |
Pre-production expenditure |
|
0 |
0 |
1,000 |
0 |
0 |
0 |
0 |
0 |
0 |
0 |
Investments costs |
|
17,895 |
18,150 |
22,395 |
0 |
0 |
0 |
0 |
0 |
0 |
0 |
Cash |
1.000 |
50 |
125 |
90 |
90 |
90 |
90 |
90 |
90 |
90 |
90 |
Client |
1.000 |
110 |
460 |
600 |
600 |
600 |
600 |
600 |
600 |
600 |
600 |
Stock |
1.000 |
1,400 |
2,000 |
2,000 |
2,000 |
2,000 |
2,000 |
2,000 |
2,000 |
2,000 |
2,000 |
Current Liabilities |
1.000 |
1,060 |
1,185 |
1,190 |
1,190 |
1,190 |
1,190 |
1,190 |
1,190 |
1,190 |
1,190 |
NET WORKING CAPITAL |
|
500 |
1,400 |
1,500 |
1,500 |
1,500 |
1,500 |
1,500 |
1,500 |
1,500 |
1,500 |
Variations in working capital |
|
500 |
900 |
100 |
0 |
0 |
0 |
0 |
0 |
0 |
0 |
Repl. of short-life equipment |
0.756 |
0 |
0 |
0 |
0 |
0 |
181 |
318 |
408 |
224 |
392 |
Residual value |
0.928 |
0 |
0 |
0 |
0 |
0 |
0 |
0 |
0 |
0 |
-25,984 |
Other investment items |
|
0 |
0 |
0 |
0 |
0 |
181 |
318 |
408 |
224 |
-25,984 |
TOTAL INVESTMENT COSTS |
|
18,395 |
19,050 |
22,495 |
0 |
0 |
181 |
318 |
408 |
224 |
-25,424 |
TOTAL EXPENDITURE |
|
18,395 |
22,227 |
27,607 |
8,908 |
20,040 |
24,787 |
25,584 |
26,351 |
26,864 |
1,911 |
|
|
|
|
|
|
|
|
|
|
|
|
NEGATIVE EXTERNALITIES |
|
0 |
18 |
27 |
47 |
102 |
124 |
127 |
129 |
132 |
135 |
|
|
|
|
|
|
|
|
|
|
|
|
TOTAL ECONOMIC |
|
18,395 |
22,245 |
27,634 |
8,955 |
20,142 |
24,911 |
25,710 |
26,480 |
26,996 |
1,878 |
EXPENDITURES |
|
||||||||||
|
|
|
|
|
|
|
|
|
|
|
|
|
|
|
|
|
|
|
|
|
|
|
|
NET ECONOMIC FLOW |
|
-18,395 |
-17,895 |
-20,944 |
2,697 |
7,629 |
9,414 |
9,483 |
9,603 |
10,000 |
36,029 |
|
|
|
|
|
|
|
|
|
|
|
|
Discount Rate |
|
5.5% |
|
|
|
|
|
|
|
|
|
ENPV |
|
3,537.5 |
|
|
|
|
|
|
|
|
|
ERR |
|
6.7% |
|
|
|
|
|
|
|
|
|
B/C |
|
1.02 |
|
|
|
|
|
|
|
|
|
200



The method applied for the forecasting must be clearly explained and details on how the forecasts were prepared may help in understanding the consistency and realism of forecasts.
Interviewing experts
Whenever, for budget or time reasons, a quantitative methodology for demand forecasting cannot be applied, interviewing experts can provide independent external estimations of the expected impact of a project. The advantages of this approach are low cost and speed. Of course, this kind of estimation can be only qualitative or, if quantitative, very approximate. Indeed, this approach can be recommended only for a very preliminary stage of the forecasting procedure.
Trend extrapolation
Extrapolation of past trends involves fitting a trend to data points from the past, usually with regression analysis. Various mathematical relationships are available that link time to the variable being forecasted (e.g. expected demand). The simplest assumption is a linear relationship, i.e.:
Y= a + bT
where Y is the variable being forecasted and T is time. Another common model assumes constant growth rate, i.e.:
Y= a(1+g)t
where Y is the variable being forecasted, a is a constant, g is the growth rate and t is time.
The choice of the best model depends mainly on data. Whenever data is available for different times (e.g. years) statistical techniques can be used to find the best fitted model. When data is available only twice any model can be fitted in principle (i.e. for each functional form parameters will always exist such as the two points lie on the curve). In such cases, additional information (e.g. trends observed in other contexts, different countries, etc.) should be used. Often, the Occam’s razor principle is applied: the simplest form is assumed unless specific information suggests a different choice. Therefore, a linear trend or a constant growth rate is applied in most cases.
Extending an observed past trend is a commonly used approach, although one should be aware of its limitations. First, trend extrapolation does not explain demand, it just assumes that an observed past behaviour will continue in the future. This may be quite a naïve assumption however. This is particularly true when new big projects are under study; significant changes on the supply side can give rise to a break in past trends. Induced transport demand is a common example.
Multiple regression models
In the regression technique, forecasts are made on the basis of a linear relationship estimated between the forecast (or dependent) variable and the explanatory (or independent) variables. Different combinations of independent variables can be tested with data, until an accurate forecasting equation is derived. The nature of the independent variables depends on the specific variable to be forecasted.
Some specific models have been developed to correlate demand to some relevant variables. For instance, the consumption-level method considers the level of consumption, using standards and defined coefficients, and can be usefully adopted for consumer products. A major determinant of consumption level is consumer income, influencing, inter alia, the household budget allocations that consumers are willing to make for a given product. With few exceptions, product consumption levels demonstrate a high degree of positive correlation with the income levels of consumers.
Regression models are widely used and can have a strong forecasting power. The main drawbacks of this technique are the need for a large amount of data (as one should explore the role of several independent variables and, for each one, a large set of values is required, across time or space) and the need for projections for the independent variables, which may be difficult. For instance, once we assume that consumption is income-dependent, the issue is then to forecast future income levels.
A generalisation of the regression models is the econometric analysis where more sophisticated mathematical forms are used in which the variable being forecasted is determined by explanatory variables such as population, income, GDP, etc. As in the regression models, the coefficients are obtained from a statistical analysis and the forecasts depend on projections of the explanatory variables.
204


Furthermore, travel demand depends on the locations of activities and families, and therefore trends in the distribution of economic activities by sector and population should also be considered. Location patterns affect not only the distance travelled, but also the frequency of trips and thus the total demand. Accessibility is one factor affecting the choices of firms and families about where to locate, and as a consequence of these choices the ‘with project’ and ‘without project’ demand may not be the same.
The price of the service provided is not the only determinant of travel demand. The choice of how much travel to consume or how far to ship a good depends on the travel cost and the time spent in travelling. Elasticity to travel time is a further determinant to be introduced in travel demand predictions. As for price elasticity, also in the case of travel time, direct and cross-elasticity are relevant. Demand for a specific mode of transport can be influenced by an increase in the speed of that mode, but also by an increase/decrease in the speed of the competing mode(s).
Demand characteristics, price, income and cross elasticity, value of time, value attributable to comfort for passengers and damage for freight will vary with the different segments of the market, as will the transport costs, type of service demanded etc. It is therefore extremely useful to disaggregate travel demand into homogeneous segments. The characteristics of the different type of commodities, the income group to which the individuals belong as well as the purpose of the trip are important determinants in predicting travel demand107.
107 Despite the considerable experience and the wide range of techniques available, forecasting transport demand remains a challenging task. Recent studies (Flyvberg et al., 2006) found considerable deviations between forecast and actual traffic volumes in more than 200 large-scale transport projects. Forecast inaccuracy is often higher in rail than in road projects. This is not to say that road forecasts are always accurate; in fact, the rate of inaccuracy in road projects is significant, but it is more balanced between overand underestimation. For rail travel the inaccuracies are systematically higher and overestimates are the rule. Many factors contribute to making rail travel forecasts less accurate than road travel forecasts: railway projects are, in general, bigger in size (but a study on aviation showed no correlation between size and demand forecast inaccuracy), and have a longer implementation phase. However, overestimation of rail traffic seems to be linked to an overoptimistic expectation of modal shift.
206
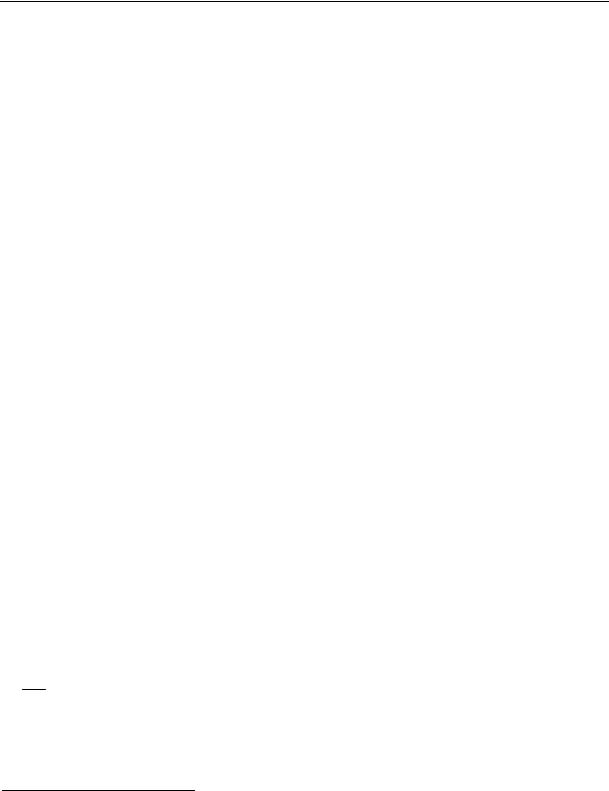

This Guide supports a unique reference FDR value based on the assumption that the funds are drawn from the EU median taxpayer. This means that even if the project is regionor beneficiary-specific, the relevant opportunity cost of capital should be based on a European portfolio. Moreover, the integration of financial markets should lead to a unique value as long as convergence of both inflation and interest rates across EU countries is expected in the longterm. This may not, however, be true of IPA countries and, under specific circumstances, of some EU Member States.
It should be noted that as long as the FDR is taken as a real discount rate, the analysis should be carried out at constant prices. If current prices are used throughout the financial analysis, a nominal discount rate (which includes inflation) must be employed.
The social discount rate
The discount rate in the economic analysis of investment projects - the social discount rate (SDR) – should reflect the social view on how future benefits and costs are to be valued against present ones. It may differ from the financial rate of return because of market failures in financial markets.
The main theoretical approaches are the following:
-a traditional view proposes that marginal public investment should have the same return as the private one, as public projects can displace private projects;
-another approach is to derive the social discount rate from the predicted long-term growth in the economy, as further explained below in the social time preference approach;
-a third, more recent approach, and one that is especially relevant in the appraisal of very long-term projects, is based on the application of variable rates over time. This approach involves decreasing marginal discount rates over time and is designed to give more weight to project impacts on future generations. These decreasing rates help mitigate the so-called ‘exponential effect’ from the structure of discount factors, which almost cancels more distant economic flows when discounted in a standard way.
In practice a shortcut solution is to consider a standard cut-off benchmark rate. The aim here is to set a required rate of return that broadly reflects the social planner’s objectives.
Still, consensus is growing around the social time preference rate (STPR) approach. This approach is based on the long term rate of growth in the economy and considers the preference for benefits over time, taking into account the expectation of increased income, or consumption, or public expenditure. An approximate and generally used formula for estimating the social discount rate from the growth rate can be expressed as follows:
r = eg + p
where r is the real social discount rate of public funds expressed in an appropriate currency (e.g. Euro); g is the growth rate of public expenditure; e is the elasticity of marginal social welfare with respect to public expenditure, and p is a rate of pure time preference.
On the basis of social time preference, France set a 4% real discount rate in 2005 (formerly fixed at 8%); in 2004 Germany reduced its social discount rate from 4% to 3%. The HM Treasury Green Book of 2003 was actually the precursor of these reductions: the real discount rate in the UK was reduced from 6% to 3.5%109.
The EC, DG Regio, has suggested a 5.5% SDR for the Cohesion countries and 3.5% for the others (EC Working Document 4)110. Every Member State should assess its country-specific social discount rate. In any case, there may be good arguments in favour of using these two benchmark values for broad macro-areas in terms of their potential for economic growth (see below).
For our practical purposes, it may be useful to reinterpret the STRP formula in terms of consumption. Let us suppose g is the growth rate of consumption, e is the elasticity of marginal utility with respect to consumption, and p is the inter-temporal preference rate.
109The application of declining discount rates, and the associated hyperbolic path for the present value weights or discount factors attached to future benefits and costs, merits a fuller consideration, especially as some of the projects considered in the Guide have investment horizons exceeding 50 years. The HM Treasury Green Book (2003) includes a schedule of declining long-term discount rates for very long-term projects based on a starting STPR of 3.5% (the standard discount rate for normal long-term projects with investment horizons of up to 30 years). The Green Book also includes a table showing the marginal discount factors up to 500 years ahead. The Stern Review (2006) on Climate Change uses a 0.1% per year, and discusses declining social discount rates.
110See also Florio (2006) for a non-technical discussion
208