
Econometrics2011
.pdf
APPENDIX B. PROBABILITY |
|
|
|
|
|
|
|
|
|
|
|
|
|
|
|
273 |
function |
|
|
|
|
|
|
|
|
|
|
|
|
|
|
|
|
F (x) = Pr |
|
Z |
|
x! |
|
|||||||||||
p |
|
|
|
|
|
|
||||||||||
Q=r |
|
|
||||||||||||||
|
( |
|
|
|
|
|
|
) |
|
|
|
|||||
|
|
|
|
|
r |
|
|
|
|
|
|
|||||
= |
E |
Z x |
|
|
Q |
|
|
|
||||||||
|
|
|
|
|
|
|
|
|||||||||
|
|
r |
|
|
|
|||||||||||
|
" |
|
|
|
|
|
|
|
|
r |
|
|
|
!# |
||
= |
E Pr Z x |
Q |
j Q |
|||||||||||||
|
|
|||||||||||||||
r |
||||||||||||||||
|
|
|
|
|
|
|
|
|
|
|
|
|
|
|
|
|
= |
E |
|
xr |
Q |
! |
|
|
|
|
|
|
|||||
|
|
|
|
|
|
|
|
|
||||||||
|
r |
|
|
|
|
|
|
Thus its density is
r!
f (x) = E |
d |
|
x |
Q |
|
r |
|||
dx |
|
r!r !
= |
E |
|
x |
|
|
Q |
|
|
Q |
|
|
|
|
|
|
|
|
|
|
||||||
|
|
r |
|
|
|
r |
|
|
r |
|
|
|
|
2r=2 qr=2 1 exp ( q=2)!dq |
|||||||||||
|
Z |
|
|
|
|
|
|
|
|
|
|
|
|
|
|
||||||||||
= |
1 |
p2 exp |
|
|
2r |
|
2 |
|
|||||||||||||||||
0 |
|
|
|
|
|
r |
r |
|
|||||||||||||||||
|
|
|
|
|
|
1 |
|
|
|
|
|
|
|
qx2 |
|
q |
|
|
|
1 |
|
|
|||
|
|
|
r |
|
|
|
|
|
|
|
|
|
|
|
|
|
|
|
|
|
|
|
|||
|
|
|
|
|
2 |
|
|
|
|
|
|
(r+12 ) |
|
|
|
|
|||||||||
|
|
|
r+1 |
|
|
|
|
|
x2 |
|
|
|
|
|
|
||||||||||
|
|
|
|
2 |
|
|
|
|
|
|
|
|
|
|
|
|
|
|
|
|
|
|
|
|
|
= |
|
p |
|
|
|
r |
|
|
1 + r |
|
|
|
|
|
|
|
|
|
|
|
|||||
|
|
|
|
|
|
|
|
|
|
|
|
|
|
|
|
|
|||||||||
which is that of the student t with r degrees of freedom. |
|
|
|
B.10 |
Inequalities |
|
|
|
|
Jensen’ |
Inequality (…nite form). If g( |
) : |
R ! R |
is convex, then for any non-negative weights |
|
m |
|
|
|
||
aj such that Pj=1 aj = 1; and any real numbers xj |
|
01
|
|
|
m |
|
|
A |
|
|
m |
|
|
|
|
|
||
|
|
@Xj |
|
|
|
X |
|
|
|
|
(B.12) |
|||||
g |
|
|
ajxj |
|
|
|
|
ajg (xj) : |
||||||||
|
|
|
=1 |
|
|
|
|
j=1 |
|
|
|
|
|
|||
In particular, setting aj = 1=m; then |
|
|
|
|
|
|
|
|
|
|
|
|
|
|
||
|
0 |
1 |
m |
|
|
1 |
|
1 |
|
m |
|
|
(B.13) |
|||
g |
|
=1 xj |
|
j=1 g (xj) : |
||||||||||||
m |
m |
|||||||||||||||
|
@ |
|
Xj |
|
A |
|
|
|
X |
|
|
|
||||
Loève’s cr Inequality. For r > 0; |
|
X |
|
|
|
|
|
X |
|
|
|
|
||||
|
|
|
|
|
|
|
|
|
|
|
||||||
|
|
|
|
m |
aj |
|
|
|
cr |
m |
|
aj |
|
r |
(B.14) |
|
|
|
|
|
r |
|
|
j |
|||||||||
|
|
|
j=1 |
|
|
|
|
j=1 j |
|
|
|
|||||
|
|
|
|
|
|
|
|
|
|
|
|
|
|
|
|
|
|
|
|
|
|
|
|
|
|
|
|
|
|
|
|
|
|
where cr = 1 when r 1 and cr = mr 1 when r 1:
Jensen’ Inequality (probabilistic form). If g( ) : Rm ! R is convex, then for any random
APPENDIX B. PROBABILITY |
274 |
vector x for which Ekxk < 1 and Ejg (x)j < 1; |
|
g(E(x)) E(g (x)) : |
(B.15) |
Conditional Jensen’ Inequality. If g( ) : Rm ! R is convex, then for any random vectors (y; x) for which Ekyk < 1 and Ekg (y)k < 1;
g(E(y j x)) E(g (y) j x) : |
(B.16) |
Conditional Expectation Inequality. For any r such that Ejyjr < 1; then |
|
EjE(y j x)jr Ejyjr < 1: |
(B.17) |
Expectation Inequality. For any random matrix Y for which EkY k < 1; |
|
kE(Y )k EkY k : |
(B.18) |
Hölder’s Inequality. If p > 1 and q > 1 and p1 + 1q = 1; then for any random m n matrices X and Y;
|
|
|
|
|
(B.19) |
E X0Y (EkXkp)1=p (EkY kq)1=q : |
|
||||
Cauchy-Schwarz Inequality. For any random m n matrices X and Y; |
|
||||
E X0Y |
EkXk2 |
1=2 EkY k2 |
1=2 |
: |
(B.20) |
|
|
|
|
|
|
Matrix Cauchy-Schwarz Inequality. Tripathi (1999). For any random x 2 Rm and y 2 R`,
Eyx0 Exx0 Exy0 Eyy0 |
(B.21) |
Minkowski’ Inequality. For any random m n matrices X and Y; |
|
(EkX + Y kp)1=p (EkXkp)1=p + (EkY kp)1=p |
(B.22) |
Liapunov’s Inequality. For any random m n matrix X and 1 r p; |
|
(EkXkr)1=r (EkXkp)1=p |
(B.23) |
Markov’s Inequality (standard form). For any random vector x and non-negative function
g(x) 0; |
|
Pr(g(x) > ) 1Eg(x): |
(B.24) |
Markov’s Inequality (strong form). For any random vector x and non-negative function
g(x) 0; |
|
|
|
|
Pr(g(x) > ) 1E(g (x) |
1 (g(x) > )) : |
(B.25) |
||
Chebyshev’ Inequality. For any random variable x; |
|
|
|
|
Pr(jx Exj > ) |
var (x) |
: |
(B.26) |
|
|
|
|||
|
2 |

APPENDIX B. PROBABILITY |
277 |
Proof of Minkowski’ Inequality. Note that by rewriting, using the triangle inequality (A.9), and then Hölder’s Inequality to the two expectations
EkX + Y kp = E kX + Y k kX + Y kp 1 |
|
|
|
|
|
||||||
|
E |
X |
X + Y |
|
p 1 |
+ E |
Y |
kX + Y kp 1 |
|
|
|
|
k |
|
k k |
k |
|
k |
|
k1=q |
|
E kX + Y kq(p 1) |
1=q |
(EkXkp)1=p E kX + Y kq(p 1) |
+ (EkY kp)1=p |
|
|||||||||
= |
(EkXkp)1=p + (EkY kp)1=p E(kX + Y kp)(p 1)=p |
|
where the second equality picks q to satisfy 1=p+1=q = 1; and the …nal equality uses this fact to make the substitution q = p=(p 1) and then collects terms. Dividing both sides by E(kX + Y kp)(p 1)=p ; we obtain (B.22).
Proof of Markov’s Inequality. Let F denote the distribution function of x: Then
Z
Pr (g(x) ) = |
dF (u) |
|||
|
fg(u) g |
|
||
|
Zfg(u) g |
u) |
|
|
|
g( |
|
dF (u) |
|
|
= |
1 Z 1 (g(u) > ) g(u)dF (u) |
= |
1E(g (x) 1 (g(x) > )) |
the inequality using the region of integration fg(u) > g: This establishes the strong form (B.25). Since 1 (g(x) > ) 1; the …nal expression is less than 1E(g(x)) ; establishing the standard form (B.24).
Proof of Chebyshev’ Inequality. De…ne y = (x Ex)2 and note that Ey = var (x) : The events fjx Exj > g and y > 2 are equal, so by an application Markov’s inequality we …nd
Pr(jx Exj > ) = Pr(y > 2) 2E(y) = 2 var (x)
as stated.
B.11 Maximum Likelihood
In this section we provide a brief review of the asymptotic theory of maximum likelihood estimation.
When the density of yi is f(y j ) where F is a known distribution function and 2 is an unknown m 1 vector, we say that the distribution is parametric and that is the parameter of the distribution F: The space is the set of permissible value for : In this setting the method of maximum likelihood is an appropriate technique for estimation and inference on : We let denote a generic value of the parameter and let 0 denote its true value.
The joint density of a random sample (y1; :::; yn) is
Yn
fn (y1; :::; yn j ) = f (yi j ) :
i=1
The likelihood of the sample is this joint density evaluated at the observed sample values, viewed as a function of . The log-likelihood function is its natural logarithm
Xn
log L( ) = log f (yi j ) :
i=1

APPENDIX B. PROBABILITY |
278 |
The likelihood score is the derivative of the log-likelihood, evaluated at the true parameter value.
@ |
log f (yi j 0) : |
|
|
|||||||
|
|
Si = |
|
|
|
|
||||
@ |
|
|
||||||||
We also de…ne the Hessian |
|
|
|
|||||||
@2 |
|
|
|
|
||||||
|
H = E |
|
log f (yi j 0) |
|
(B.28) |
|||||
@ @ 0 |
||||||||||
and the outer product matrix |
|
|
|
|||||||
|
|
|
= E SiSi0 : |
|
|
(B.29) |
||||
We now present three important features of the likelihood. |
|
|
||||||||
|
|
|
|
|
|
|
||||
|
Theorem B.11.1 |
|
|
|
||||||
|
|
@@ Elog f (y j ) = 0 |
= 0 |
(B.30) |
|
|||||
|
|
|
|
|
|
|
|
|
|
|
|
|
|
|
|
|
|
|
|
|
|
|
|
|
|
|
ESi = 0 |
|
(B.31) |
|
||
|
and |
|
|
|
||||||
|
|
|
H = I |
|
(B.32) |
|
||||
|
|
|
|
|
|
|
|
|
|
|
The matrix I is called the information, and the equality (B.32) is called the information matrix equality.
^
The maximum likelihood estimator (MLE) is the parameter value which maximizes the likelihood (equivalently, which maximizes the log-likelihood). We can write this as
^ |
= argmax log L( ): |
(B.33) |
|
2
^
In some simple cases, we can …nd an explicit expression for as a function of the data, but these
^
cases are rare. More typically, the MLE must be found by numerical methods.
^
To understand why the MLE is a natural estimator for the parameter observe that the standardized log-likelihood is a sample average and an estimator of Elog f (yi j ) :
1 |
1 |
|
n |
p |
|
|
|
|
|
Xi |
|
n log L( ) = n |
|
||||
=1 log f (yi j ) ! Elog f (yi j ) : |
^
As the MLE maximizes the left-hand-side, we can see that it is an estimator of the maximizer of the right-hand-side. The …rst-order condition for the latter problem is
@
0 = @ Elog f (yi j )
^
which holds at = 0 by (B.30). This suggests that is an estimator of 0: In. fact, under
^ |
^ |
p |
as n ! 1: Furthermore, we can derive |
||||||
conventional regularity conditions, is consistent, |
! 0 |
||||||||
its asymptotic distribution. |
|
|
|
|
|
|
|
|
|
|
|
|
|
|
|
|
|
|
|
|
|
|
|
|
|
|
d |
|
|
|
Theorem B.11.2 Under regularity conditions, pn ^ 0 |
|
0; I 1 . |
|
|||||
|
! N |
|
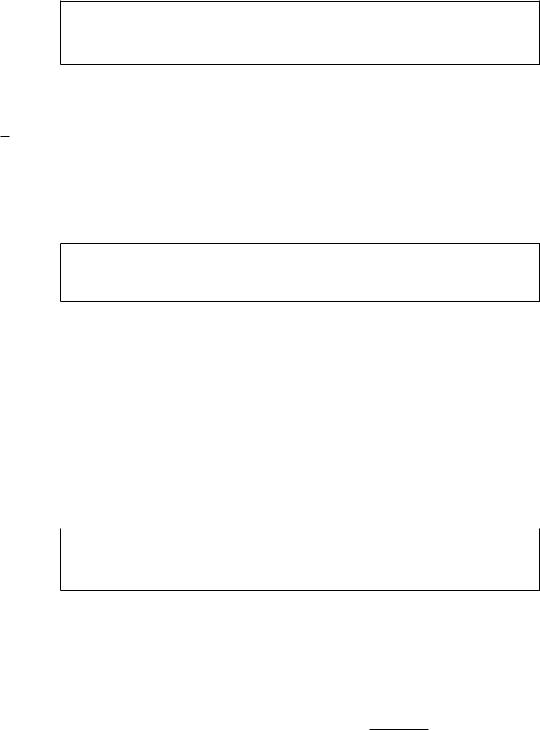
APPENDIX B. PROBABILITY |
279 |
We omit the regularity conditions for Theorem B.11.2, but the result holds quite broadly for models which are smooth functions of the parameters. Theorem B.11.2 gives the general form for the asymptotic distribution of the MLE. A famous result shows that the asymptotic variance is the smallest possible.
Theorem B.11.3 Cramer-Rao Lower Bound. If is an unbiased reg-
e |
e |
ular estimator of ; then var( ) (nI) : |
The Cramer-Rao Theorem shows that the …nite sample variance of an unbiased estimator is
bounded below by (nI) 1 : This means that the asymptotic variance of the standardized estimator p e 1
n 0 is bounded below by I : In other words, the best possible asymptotic variance among
all (regular) estimators is I 1: An estimator is called asymptotically e¢ cient if its asymptotic variance equals this lower bound. Theorem B.11.2 shows that the MLE has this asymptotic variance, and is thus asymptotically e¢ cient.
Theorem B.11.4 The MLE is asymptotically e¢ cient in the sense that its asymptotic variance equals the Cramer-Rao Lower Bound.
Theorem B.11.4 gives a strong endorsement for the MLE in parametric models.
b b
Finally, consider functions of parameters. If = g( ) then the MLE of is = g( ): This is because maximization (e.g. (B.33)) is una¤ected by parameterization and transformation. Applying the Delta Method to Theorem B.11.2 we conclude that
@ |
|
|
|
b |
|
|
|
b |
d |
|
|
|
|||
|
|
|
pn |
|
|
' G0pn |
|
|
|
(B.34) |
|||||
|
|
|
|
! N 0; G0I 1G |
|||||||||||
where G = |
|
g( 0): By Theorem B.11.4, |
|
is an asymptotically e¢ cient estimator for |
. The |
||||||||||
@ |
|
||||||||||||||
asymptotic variance G0I 1G is the Cramerb-Rao lower bound for estimation of . |
|
||||||||||||||
|
Theorem B.11.5 The Cramer-Rao lower bound for |
= g( ) is G0I 1G |
|
|
bb
,and the MLE = g( ) is asymptotically e¢ cient.
Proof of Theorem B.11.1. To see (B.30); |
|
|
|
|
|
|
|
|
|
|
|||
|
@ |
|
|
|
@ |
|
|
|
|
|
|
|
|
|
@ |
Elog f (y j ) = 0 |
= |
|
@ |
Z |
log f (y j ) f (y j 0) dy = 0 |
||||||
|
|
|
|
|
|
|
|
|
|
j |
0) |
|
|
|
|
|
|
|
@ |
|
|
f (y |
|
|
|
||
|
|
|
= |
@ |
|
f (y ) |
|
j |
|
dy |
|
||
|
|
|
|
|
|
||||||||
|
|
|
|
@ |
|
j |
f (y ) |
|
|
||||
|
|
|
|
Z |
|
|
= 0 |
||||||
|
|
|
|
@ |
|
|
|
|
|
|
|
|
|
|
|
|
|
|
|
|
|
|
|
|
|||
|
|
|
= |
|
@ |
Z |
f (y j ) dy = 0 |
|
|||||
|
|
|
|
|
|
|
|
|
|
|
|
|
|
|
|
|
|
|
|
|
|
|
|
|
|
|
|
|
|
|
= |
@ |
1 = 0 |
= 0: |
|
|
|
|
|||
|
|
|
|
|
|
|
|
|
|
|
|
|
|

APPENDIX B. PROBABILITY |
|
|
|
281 |
||||
Together, |
|
|
|
|
||||
|
|
d |
0; H 1 H 1 = N 0; I 1 ; |
|
||||
|
|
|
||||||
pn ^ 0 ! H 1N (0; ) = N |
|
|||||||
the …nal equality using Theorem B.11.1 . |
|
|
|
|
||||
Proof of Theorem B.11.3. Let Y = (y1; :::; yn) be the sample, and set |
|
|||||||
|
|
|
|
|
|
n |
|
|
@ |
|
Xi |
|
|||||
|
|
S = |
|
log fn (Y ; 0) = |
|
Si |
|
|
|
|
@ |
|
|
||||
|
|
|
|
|
|
=1 |
|
|
which by Theorem (B.11.1) has mean zero and variance n |
|
: Write the estimator = (Y ) as a |
||||||
|
|
e |
|
I |
|
e e |
||
function of the data. Since is unbiased for any ; |
|
|
Z
ee
= E = (Y ) f (Y ; ) dY :
Di¤erentiating with respect to and evaluating at 0 yields |
|
|
e |
|
e |
|
|||||||||||||||||||
|
|
e |
@ |
|
|
e |
|
|
@ |
|
|
|
|
|
|
|
|
|
|||||||
I = Z (Y ) |
@ 0 |
f (Y ; ) dY |
= Z |
(Y ) |
|
@ 0 |
log f (Y |
; ) f (Y ; 0) dY |
= E |
S0 |
= E |
0 |
S0 |
||||||||||||
the …nal equality since E(S) = 0 |
|
|
|
|
|
|
|
|
|
|
e |
|
S0 = I; and var (S) = E(SS0) = |
||||||||||||
|
I |
|
|
|
|
|
|
|
|
|
|
|
|
|
|
|
|||||||||
n |
|
;By the matrix Cauchy-Schwarz inequality (B.21), |
E |
0 |
|||||||||||||||||||||
|
|
|
|
e |
|
E |
e |
|
|
|
e |
|
|
0 |
|
|
|
|
0 |
|
|
|
|||
|
|
|
|
var |
= |
|
|
0 |
0 |
|
|
e |
|
|
|
|
|
|
|||||||
|
|
|
|
|
|
e |
|
|
|
|
|
|
|
|
|
|
0 |
|
|
|
|||||
|
|
|
|
|
E |
|
0 S0 E SS0 |
|
E S |
|
|
|
|
||||||||||||
|
|
|
|
|
= |
SS |
0 |
|
|
|
|
|
|
|
|
|
|
|
|
|
|
||||
|
|
|
|
|
= (En |
) |
|
|
|
|
|
|
|
|
|
|
|
|
|
|
|
||||
|
|
|
|
|
|
I |
|
|
|
|
|
|
|
|
|
|
|
|
|
|
|
|
|
|
|
as stated. |
|
|
|
|
|
|
|
|
|
|
|
|
|
|
|
|
|
|
|
|
|
|
