
Econometrics2011
.pdf

CHAPTER 17. PANEL DATA |
244 |
Another derivation of the estimator is to take the equation |
|
yit = x0it + ui + eit;
and then take individual-speci…c means by taking the average for the i0th individual:
|
|
|
|
|
|
|
|
|
|
|
|
|
|
|
|
1 ti |
yit = |
1 |
|
ti |
x0 + ui + |
1 |
ti |
eit |
|||||||
|
tXi |
|
|
Xi |
|
Xi |
|||||||||
Ti =t |
|
Ti t=t |
it |
Ti t=t |
|
||||||||||
|
|
|
or
yi = x0i + ui + ei:
Subtracting, we …nd
yit = x0it + eit;
which is free of the individual-e¤ect ui:
17.3Dynamic Panel Regression
A dynamic panel regression has a lagged dependent variable
yit = yit 1 + xit0 + ui + eit: |
(17.3) |
This is a model suitable for studying dynamic behavior of individual agents.
Unfortunately, the …xed e¤ects estimator is inconsistent, at least if T is held …nite as n ! 1: This is because the sample mean of yit 1 is correlated with that of eit:
The standard approach to estimate a dynamic panel is to combine …rst-di¤erencing with IV or GMM. Taking …rst-di¤erences of (17.3) eliminates the individual-speci…c e¤ect:
yit = yit 1 + xit0 + eit: |
(17.4) |
However, if eit is iid, then it will be correlated with yit 1 :
E( yit 1 eit) = E((yit 1 yit 2) (eit eit 1)) = E(yit 1eit 1) = 2e:
So OLS on (17.4) will be inconsistent.
But if there are valid instruments, then IV or GMM can be used to estimate the equation. Typically, we use lags of the dependent variable, two periods back, as yt 2 is uncorrelated witheit: Thus values of yit k; k 2, are valid instruments.
Hence a valid estimator of and is to estimate (17.4) by IV using yt 2 as an instrument foryt 1 (which is just identi…ed). Alternatively, GMM using yt 2 and yt 3 as instruments (which is overidenti…ed, but loses a time-series observation).
A more sophisticated GMM estimator recognizes that for time-periods later in the sample, there are more instruments available, so the instrument list should be di¤erent for each equation. This is conveniently organized by the GMM principle, as this enables the moments from the di¤erent timeperiods to be stacked together to create a list of all the moment conditions. A simple application of GMM yields the parameter estimates and standard errors.


CHAPTER 18. NONPARAMETRICS |
246 |
This estimator is the average of a set of weights. If a large number of the observations Xi are near x; then the weights are relatively large and f^(x) is larger. Conversely, if only a few Xi are near x; then the weights are small and f^(x) is small. The bandwidth h controls the meaning of “near”.
Interestingly, f^(x) is a valid density. That is, f^(x) 0 for all x; and |
|
|
||||||||||||||
Z 1 f^(x)dx = Z |
1 |
n |
|
1 n |
Z 1 Kh (Xi x) dx = |
1 n |
Z |
1 K (u) du = 1 |
||||||||
1 |
|
i=1 Kh (Xi x) dx = |
|
i=1 |
|
i=1 |
||||||||||
n |
n |
n |
||||||||||||||
1 |
1 |
X |
|
|
X |
1 |
|
|
X |
|
1 |
|||||
where the second-to-last equality makes the change-of-variables u = (Xi x)=h: |
|
|||||||||||||||
We can also calculate the moments of the density f^(x): The mean is |
|
|
||||||||||||||
Z |
1 xf^(x)dx = |
1 n |
Z 1 xKh (Xi x) dx |
|
|
|
|
|
||||||||
|
i=1 |
|
|
|
|
|
||||||||||
n |
|
|
|
|
|
|||||||||||
1 |
|
|
X |
1 |
|
|
|
|
|
|
|
|
||||
|
|
|
|
1 n |
|
|
|
|
|
|
|
|
|
|
|
|
|
|
|
|
|
X |
1 |
|
|
|
|
|
|
|
|
||
|
|
|
|
|
|
|
|
|
|
|
|
|
||||
|
|
|
= n i=1 |
Z 1 |
(Xi + uh) K (u) du |
|
|
|
|
|
||||||
|
|
|
|
|
n |
Xi Z |
|
|
|
|
n |
|
|
|
|
|
|
|
|
|
|
X |
1 |
|
|
X |
1 |
|
|
||||
|
|
|
|
|
|
|
|
|||||||||
|
|
|
= n i=1 |
1 K (u) du + n i=1 h Z |
1 uK (u) du |
|||||||||||
|
|
|
1 |
|
|
|
|
1 |
|
|
|
|
|
|
1Xn
=n i=1 Xi
the sample mean of the Xi; where the second-to-last equality used the change-of-variables u = (Xi x)=h which has Jacobian h:
The second moment of the estimated density is |
|
|
|
|
|
|
|
|
|
|||||||||||
Z |
|
1 |
n |
|
Z |
|
|
|
|
|
|
|
|
|
|
|
|
|
|
|
1 x2f^(x)dx = |
|
i=1 |
1 x2Kh (Xi x) dx |
|
|
|
|
|
||||||||||||
n |
|
|
|
|
|
|||||||||||||||
|
1 |
|
|
X |
Z |
1 |
|
|
|
|
|
|
|
|
|
|
|
|||
|
|
1 |
n |
|
|
|
|
|
|
|
|
|
|
|
|
|
|
|
||
|
|
|
|
X |
1 |
|
|
|
|
|
|
|
|
|
|
|
||||
|
|
|
|
|
|
|
|
|
|
|
|
|
|
|
||||||
|
|
= n i=1 |
1 (Xi + uh)2 K (u) du |
|
|
|
|
|
||||||||||||
|
|
1 |
n |
|
|
|
2 n |
|
|
1 |
n |
|
|
|
||||||
|
|
|
|
X |
|
|
|
X |
|
|
|
|
|
X |
|
|
|
|||
|
|
|
|
|
|
|
1 |
1 |
|
|||||||||||
|
|
= n i=1 Xi2 + n i=1 Xih Z |
1 K(u)du + n i=1 h2 Z |
|
1 u2K (u) du |
|||||||||||||||
|
|
= |
1 |
n |
|
Xi2 + h2 K2 |
|
|
|
|
|
|
|
|
|
|
|
|||
|
|
|
Xi |
|
|
|
|
|
|
|
|
|
|
|
||||||
|
|
|
|
|
|
|
|
|
|
|
|
|
|
|
|
|
|
|
||
|
|
n =1 |
|
|
|
|
|
|
|
|
|
|
|
|
|
|
|
|||
where |
|
|
|
|
|
|
|
|
|
|
1 |
|
|
|
|
|
|
|
|
|
|
|
|
|
|
|
|
|
|
|
|
|
|
|
|
|
|
|
|
|
|
|
|
|
|
|
|
|
K2 = Z 1 u2K (u) du |
|
|
|
|
|
||||||||
is the variance of the kernel. It follows that the variance of the density f^(x) is |
|
|||||||||||||||||||
|
|
1 |
|
|
1 |
|
|
|
2 |
|
|
1 n |
|
1 n |
2 |
|||||
|
Z |
|
|
xf^(x)dx |
|
|
|
! |
||||||||||||
|
1 |
1 |
|
|
|
|
X |
|
|
|
X |
|||||||||
|
|
|
|
|
|
|
|
|||||||||||||
|
x2f^(x)dx Z |
|
|
|
= n i=1 Xi2 + h2 K2 n i=1 Xi |
|||||||||||||||
|
|
|
|
|
|
|
|
|
|
|
= |
^2 + h2 K2 |
|
|
|
|
|
|||
Thus the variance of the estimated density is in‡ated by the factor h2 2 |
relative to the sample |
|||||||||||||||||||
|
|
|
|
|
|
|
|
|
|
|
|
|
|
|
|
K |
|
|
|
moment.
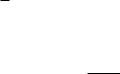

CHAPTER 18. NONPARAMETRICS |
248 |
A global measure of precision is the asymptotic mean integrated squared error (AMISE)
AMISEh = Z |
|
h4 |
4 R(f |
) |
|
(K) |
|
|
|
AMSEh(x)dx = |
|
K |
00 |
|
+ |
R |
: |
(18.1) |
|
|
4 |
|
|
nh |
where R(f00) = R (f00(x))2 dx is the roughness of f00: Notice that the …rst term (the squared bias) is increasing in h and the second term (the variance) is decreasing in nh: Thus for the AMISE to decline with n; we need h ! 0 but nh ! 1: That is, h must tend to zero, but at a slower rate than n 1:
Equation (18.1) is an asymptotic approximation to the MSE. We de…ne the asymptotically optimal bandwidth h0 as the value which minimizes this approximate MSE. That is,
h0 = argmin AMISEh
h
It can be found by solving the …rst order condition
|
d |
|
AMISE |
|
= h3 4 |
R(f00) |
|
R(K) |
= 0 |
||||
|
|
|
h |
|
|
||||||||
|
dh |
nh2 |
|||||||||||
|
|
|
K |
|
|
|
|
||||||
yielding |
h0 = |
|
|
|
|
|
|
|
|
|
|||
|
|
|
|
R(K) |
|
1=5 |
|
|
|
|
|||
|
|
|
|
|
|
n 1=2: |
(18.2) |
||||||
|
|
|
|
|
|
||||||||
|
|
|
|
K4 R(f00) |
|
This solution takes the form h0 = cn 1=5 where c is a function of K and f; but not of n: We thus say that the optimal bandwidth is of order O(n 1=5): Note that this h declines to zero, but at a very slow rate.
In practice, how should the bandwidth be selected? This is a di¢ cult problem, and there is a large and continuing literature on the subject. The asymptotically optimal choice given in (18.2) depends on R(K); 2K; and R(f00): The …rst two are determined by the kernel function. Their values for the three functions introduced in the previous section are given here.
K |
K2 = |
1 u2K (u) du R(K) = |
11 K (u)2 du |
|||
Gaussian |
|
R 11 |
1/R(2p |
|
) |
|
|
|
|||||
Epanechnikov |
|
1=5 |
|
1=5 |
|
|
Biweight |
|
1=7 |
|
5=7 |
|
|
An obvious di¢ culty is that R(f00) is unknown. A classic simple solution proposed by Silverman (1986)has come to be known as the reference bandwidth or Silverman’s Rule-of-Thumb. It uses formula (18.2) but replaces R(f00) with ^ 5R( 00); where is the N(0; 1) distribution and ^2 is an estimate of 2 = var(X): This choice for h gives an optimal rule when f(x) is normal, and gives a nearly optimal rule when f(x) is close to normal. The downside is that if the density is very far from normal, the rule-of-thumb h can be quite ine¢ cient. We can calculate that R( 00) = 3= (8p ) : Together with the above table, we …nd the reference rules for the three kernel functions introduced earlier.
Gaussian Kernel: hrule = 1:06^n 1=5 Epanechnikov Kernel: hrule = 2:34^n 1=5 Biweight (Quartic) Kernel: hrule = 2:78^n 1=5
Unless you delve more deeply into kernel estimation methods the rule-of-thumb bandwidth is a good practical bandwidth choice, perhaps adjusted by visual inspection of the resulting estimate f^(x): There are other approaches, but implementation can be delicate. I now discuss some of these choices. The plug-in approach is to estimate R(f00) in a …rst step, and then plug this estimate into the formula (18.2). This is more treacherous than may …rst appear, as the optimal h for estimation of the roughness R(f00) is quite di¤erent than the optimal h for estimation of f(x): However, there
CHAPTER 18. NONPARAMETRICS |
249 |
are modern versions of this estimator work well, in particular the iterative method of Sheather and Jones (1991). Another popular choice for selection of h is cross-validation. This works by constructing an estimate of the MISE using leave-one-out estimators. There are some desirable properties of cross-validation bandwidths, but they are also known to converge very slowly to the optimal values. They are also quite ill-behaved when the data has some discretization (as is common in economics), in which case the cross-validation rule can sometimes select very small bandwidths leading to dramatically undersmoothed estimates. Fortunately there are remedies, which are known as smoothed cross-validation which is a close cousin of the bootstrap.
APPENDIX A. MATRIX ALGEBRA |
251 |
are row vectors.
The transpose of a matrix, denoted A0; is obtained by ‡ipping the matrix on its diagonal.
Thus |
2 a12 |
a22 |
|
ak2 |
3 |
|||
|
||||||||
|
|
a11 |
a21 |
|
ak1 |
7 |
||
A0 = |
6 ... |
... |
|
a |
... |
|||
|
6 a |
|
a |
|
|
7 |
||
|
4 |
|
1r |
2r |
|
kr |
5 |
|
|
6 |
|
|
|
7 |
|||
Alternatively, letting B = A0; then bij |
= aji. |
Note that if A is k r, then A0 is r k: If a is a |
k 1 vector, then a0 is a 1 k row vector. An alternative notation for the transpose of A is A>: A matrix is square if k = r: A square matrix is symmetric if A = A0; which requires aij = aji:
A square matrix is diagonal if the o¤-diagonal elements are all zero, so that aij = 0 if i =6 j: A square matrix is upper (lower) diagonal if all elements below (above) the diagonal equal zero.
An important diagonal matrix is the identity matrix, which has ones on the diagonal. The k k identity matrix is denoted as
23
|
6 |
1 |
0 |
|
0 |
7 |
|
Ik = |
0... |
1... |
|
0... |
: |
||
|
6 |
0 |
0 |
|
1 |
7 |
|
|
6 |
|
|
|
|
7 |
|
|
4 |
|
|
|
|
5 |
|
A partitioned matrix takes the form
A = |
|
A11 |
A12 |
|
A1r |
7 |
|||
6 ... ... |
... |
||||||||
|
2 |
A21 |
A22 |
|
A2r |
3 |
|||
|
6 |
A |
|
A |
|
|
A |
|
7 |
|
4 |
|
k1 |
|
k2 |
|
|
kr |
5 |
|
6 |
|
|
|
|
7 |
where the Aij denote matrices, vectors and/or scalars.
A.2 Matrix Addition
If the matrices A = (aij) and B = (bij) are of the same order, we de…ne the sum
A + B = (aij + bij) :
Matrix addition follows the communtative and associative laws:
A + B = |
B + A |
A + (B + C) = |
(A + B) + C: |
A.3 Matrix Multiplication
If A is k r and c is real, we de…ne their product as
Ac = cA = (aijc) :
If a and b are both k 1; then their inner product is
Xk
a0b = a1b1 + a2b2 + + akbk = ajbj:
j=1
Note that a0b = b0a: We say that two vectors a and b are orthogonal if a0b = 0:
APPENDIX A. MATRIX ALGEBRA |
252 |
If A is k r and B is r s; so that the number of columns of A equals the number of rows of B; we say that A and B are conformable. In this event the matrix product AB is de…ned. Writing A as a set of row vectors and B as a set of column vectors (each of length r); then the
matrix product is de…ned as |
2 a20 |
3 |
|
|
|
|
|
|
|
||
|
|
|
|
|
|
|
|
||||
|
a10 |
|
|
|
|
|
|
|
|
|
|
AB = |
6 a |
k0 |
7 |
|
b2 |
|
bs |
|
|
||
6 |
7 |
|
|
|
|||||||
6 ... |
7 b1 |
|
|
|
|||||||
|
4 |
|
5 |
|
|
|
|
|
3 |
|
|
|
a10 b1 |
a10 b2 |
|
a10 bs |
|
||||||
|
2 a20 b1 |
a20 b2 |
a20 bs |
|
|||||||
= |
6 ... |
|
... |
|
|
... |
|
7 |
: |
||
|
4 |
k0 |
1 |
k0 |
2 |
|
k0 |
|
s |
5 |
|
|
6 |
|
b |
7 |
|
||||||
|
6 a |
|
b |
a b |
|
|
a |
|
7 |
|
Matrix multiplication is not communicative: in general AB 6= BA: However, it is associative and distributive:
A (BC) = (AB) C
A (B + C) = AB + AC
An alternative way to write the matrix product is to use matrix partitions. For example,
|
|
A11 |
A12 |
B11 |
B12 |
|
|
|
|
|
AB = |
A21 |
A22 |
B21 |
B22 |
|
|
|
|
||
= |
|
A11B11 + A12B21 |
A11B12 + A12B22 |
: |
||||||
A21B11 + A22B21 |
A21B12 + A22B22 |
|||||||||
As another example, |
|
|
|
|
|
2 |
|
|
3 |
|
|
|
|
|
|
|
B2 |
|
|||
|
|
|
|
|
|
|
B1 |
|
|
|
AB = |
A1 A2 |
Ar |
6 |
B |
r |
7 |
|
|||
6 |
|
7 |
|
|||||||
6 ... |
|
7 |
|
|||||||
|
|
|
|
|
|
4 |
|
|
5 |
|
=A1B1 + A2B2 + + ArBr
Xr
=AjBj
j=1
An important property of the identity matrix is that if A is k r; then AIr = A and IkA = A: The k r matrix A, r k, is called orthogonal if A0A = Ir:
A.4 Trace
The trace of a k k square matrix A is the sum of its diagonal elements
Xk
tr (A) = aii:
i=1
Some straightforward properties for square matrices A and B and real c are
tr (cA) = c tr (A)
tr A0 |
= tr (A) |
|
+ B) = |
tr (A) + tr (B) |
|
tr (A |
|
|
tr (Ik) |
= |
k: |