
Econometrics2011
.pdf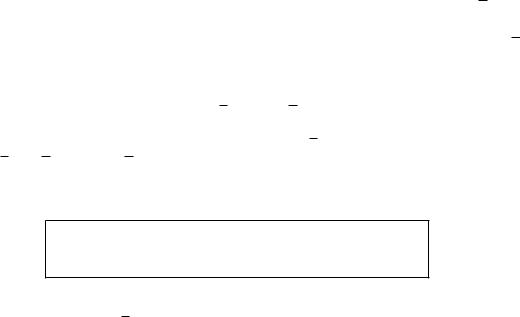
CHAPTER 11. GENERALIZED METHOD OF MOMENTS |
194 |
11.2GMM Estimator
De…ne the sample analog of (11.2)
1 |
n |
1 |
|
n |
|
|
1 |
|
|
|
||||
|
|
|
X |
|
|
|
Xi |
|
|
|
|
|
|
(11.3) |
|
|
|
|
|
|
|
|
|||||||
gn( ) = n |
i=1 |
gi( ) = n |
=1 |
zi yi xi0 |
|
= n |
|
Z0y Z0X : |
||||||
|
|
|
|
|
|
|
|
|
|
|
|
|
The method of moments estimator for is de…ned as the parameter value which sets gn( ) = 0. This is generally not possible when ` > k; as there are more equations than free parameters. The idea of the generalized method of moments (GMM) is to de…ne an estimator which sets gn( ) “close”to zero.
For some ` ` weight matrix W n > 0; let
Jn( ) = n gn( )0W n gn( ):
This is a non-negative measure of the “length”of the vector gn( ): For example, if W n = I; then, Jn( ) = n gn( )0gn( ) = n kgn( )k2 ; the square of the Euclidean length. The GMM estimator minimizes Jn ( ).
b
De…nition 11.2.1 GMM = argmin Jn ( ) :
b
Note that if k = `; then gn( ) = 0; and the GMM estimator is the method of moments estimator: The …rst order conditions for the GMM estimator are
|
|
0 |
= |
@ |
|
|
|
|
|
b |
|
|
|
|
|
|
|
|
|
|
|
|||
|
|
|
|
|
|
|
|
|
|
|
|
|
|
|
|
|
|
|
|
|
||||
|
|
@ @ |
|
|
|
|
|
|
|
|
|
|
|
|||||||||||
|
|
|
|
Jn( ) |
|
|
|
|
|
|
|
|
|
|
|
|||||||||
|
|
|
= 2 |
|
|
|
|
|
|
|
( )0W |
|
|
|
( ) |
|
|
|
|
|||||
|
|
|
|
|
|
g |
|
g |
|
|
|
|
||||||||||||
|
|
|
|
|
|
|
|
|
|
|||||||||||||||
|
|
|
|
|
@ |
|
|
1n |
b |
|
n |
|
n |
b1 |
|
b |
|
|
||||||
so |
|
|
|
|
|
|
|
|
|
|
|
|
|
|
|
|
|
|
|
|
||||
|
|
|
= 2 nX0Z W n nZ0 |
y X |
|
|
||||||||||||||||||
|
|
2 X0Z W n Z0X = 2 X0Z W n Z0y |
||||||||||||||||||||||
which establishes the |
following. |
|
|
|
|
|
|
b |
|
|
|
|
|
|
|
|||||||||
|
|
|
|
|
|
|
|
|
||||||||||||||||
|
Proposition 11.2.1 |
|
|
|
|
|
|
|
|
|
|
|
|
|
|
|
|
|
|
|
|
|
||
|
|
GMM = X0Z W n Z0X 1 X0Z W n Z0y : |
||||||||||||||||||||||
|
|
b |
|
|
|
|
|
|
|
|
|
|
|
|
|
|
|
|
|
|
|
|
|
|
While the estimator depends on W n; the dependence is only up to scale, for if W n is replaced
b
by cW n for some c > 0; GMM does not change.
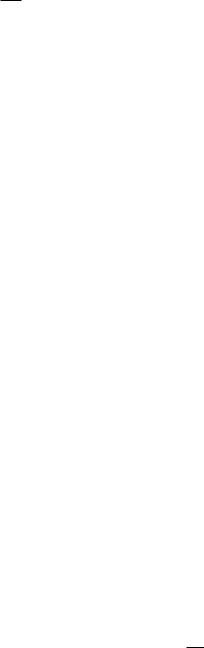
CHAPTER 11. GENERALIZED METHOD OF MOMENTS |
195 |
11.3Distribution of GMM Estimator
|
|
p |
|
|
|
|
|
|
|
|
|
|
|
|
||
Assume that W n ! W > 0: Let |
Q = E zixi0 |
|||||||||||||||
and |
|
|
|
|
|
|
|
|||||||||
where gi = ziei: Then |
|
|
|
|
= E zizi0ei2 = E gigi0 ; |
|||||||||||
|
|
|
|
|
1 |
|
|
|
|
|
1 |
|
p |
|||
|
|
|
|
|
|
X0Z W n |
|
Z0X ! Q0W Q |
||||||||
|
|
n |
n |
|||||||||||||
and |
1 |
|
|
|
|
|
|
|
1 |
|
|
|
|
|||
|
|
|
|
|
|
|
|
|
|
|
|
d |
||||
|
|
|
|
X0Z W n |
p |
|
Z0e |
! Q0W N (0; ) : |
||||||||
|
|
n |
||||||||||||||
|
|
n |
||||||||||||||
We conclude: |
|
|
|
|
|
|
|
|
|
|
|
|
|
|
|
|
|
|
|
|
|||||||||||||
|
Theorem 11.3.1 Asymptotic Distribution of GMM Estimator |
|||||||||||||||
|
|
|
|
|
|
|
|
|
|
|
|
|
|
d |
|
|
|
|
|
|
|
|
pn |
|
|
|
|
|
|||||
|
|
|
|
|
|
! N (0; V ) ; |
||||||||||
|
where |
|
|
|
|
|
|
|
b |
|
|
|
|
|
|
|
|
|
V = |
Q0W Q 1 Q0W W Q Q0W Q 1 : |
In general, GMM estimators are asymptotically normal with “sandwich form”asymptotic variances.
The optimal weight matrix W 0 is one which minimizes V : This turns out to be W 0 = 1: The proof is left as an exercise. This yields the e¢ cient GMM estimator:
|
|
= X0Z 1Z0X 1 |
X0Z 1Z0y: |
|
||||||
Thus we have |
b |
|
|
|
|
|
|
|||
|
Theorem 11.3.2 Asymptotic Distribution of E¢ cient GMM Es- |
|
|
|||||||
|
timator |
|
|
b |
d |
|
|
1 |
|
|
|
|
|
|
|
|
|||||
|
|
pn |
0; |
Q0 1Q |
|
|
||||
|
|
! N |
: |
|
|
|||||
|
|
|
|
|
|
|
|
|
p |
; |
W 0 = 1 is not known in practice, but it can be estimated consistently. For any W n ! W 0 |
b
we still call the e¢ cient GMM estimator, as it has the same asymptotic distribution.
By “e¢ cient”, we mean that this estimator has the smallest asymptotic variance in the class of GMM estimators with this set of moment conditions. This is a weak concept of optimality, as we are only considering alternative weight matrices W n: However, it turns out that the GMM estimator is semiparametrically e¢ cient, as shown by Gary Chamberlain (1987).
If it is known that E(gi( )) = 0; and this is all that is known, this is a semi-parametric problem, as the distribution of the data is unknown. Chamberlain showed that in this context, no semiparametric estimator (one which is consistent globally for the class of models considered) can have a smaller asymptotic variance than G0 1G 1 where G = E@@ 0 gi( ): Since the GMM estimator has this asymptotic variance, it is semiparametrically e¢ cient.
This result shows that in the linear model, no estimator has greater asymptotic e¢ ciency than the e¢ cient linear GMM estimator. No estimator can do better (in this …rst-order asymptotic sense), without imposing additional assumptions.

CHAPTER 11. GENERALIZED METHOD OF MOMENTS |
196 |
11.4Estimation of the E¢ cient Weight Matrix
Given any weight matrix W n > 0; the GMM estimator is consistent yet ine¢ cient. For |
|||||||||||||||||||||||||||
example, we can set W n |
= I`: In the linear model, a betterbchoice is W n |
= (Z0Z) 1 : Given |
|||||||||||||||||||||||||
any such …rst-step estimator, we can de…ne the residuals e^i = yi |
|
|
x0 |
and moment equations |
|||||||||||||||||||||||
g^i = zie^i = g(yi; xi; zi; ): Construct |
|
|
|
|
|
|
|
|
|
|
|
|
|
|
|
|
|
|
|
i b |
|
||||||
b |
|
|
|
|
= |
|
( ) = |
1 |
|
n |
g^ ; |
|
|
|
|
|
|
||||||||||
|
g |
g |
Xi |
|
|
|
|
|
|
||||||||||||||||||
|
|
|
|
|
|
|
|
|
|
|
|||||||||||||||||
|
|
|
|
|
n |
|
|
n |
b |
|
|
n |
|
i |
|
|
|
|
|
|
|||||||
|
|
|
|
|
|
|
|
|
|
|
|
|
|
|
|
|
|
|
|||||||||
|
|
|
|
|
|
|
|
|
|
|
|
|
|
=1 |
|
|
|
|
|
|
|
|
|
|
|||
|
|
|
|
|
|
g^i = g^i |
|
n; |
|
|
|
|
|
|
|
|
|
|
|||||||||
|
|
|
|
|
|
g |
|
|
|
|
|
|
|
|
|
|
|||||||||||
and de…ne |
|
1 n |
|
|
|
! |
1 |
|
1 |
|
n |
|
|
|
|
|
|
! |
1 |
|
|||||||
|
|
|
|
|
|
|
|
|
|
|
|
|
|
||||||||||||||
|
|
|
X |
|
|
|
|
|
|
|
|
|
|
|
Xi |
|
|
|
|
|
|
|
|
|
|||
W n = |
n |
i=1 g^i g^i 0 |
|
= |
|
n |
=1 g^ig^i0 |
g |
n |
g |
n0 |
|
: |
(11.4) |
|||||||||||||
p |
; and GMM using W n as the weight matrix is asymptotically e¢ cient. |
||||||||||||||||||||||||||
Then W n ! 1 = W 0 |
|||||||||||||||||||||||||||
A common alternative choice is to set |
|
|
|
|
|
|
|
|
|
|
|
|
|
|
|
|
|
|
|
|
|
||||||
|
|
|
|
|
|
|
|
|
|
1 |
|
n |
|
|
1 |
|
|
|
|
|
|
||||||
|
|
|
|
|
|
|
|
|
|
|
|
Xi |
|
|
|
|
|
|
|
|
|
|
|
||||
|
|
|
W n = |
|
|
n |
=1 g^ig^i0! |
|
|
|
|
|
|
|
|
|
which uses the uncentered moment conditions. Since Egi = 0; these two estimators are asymptotically equivalent under the hypothesis of correct speci…cation. However, Alastair Hall (2000) has shown that the uncentered estimator is a poor choice. When constructing hypothesis tests, under the alternative hypothesis the moment conditions are violated, i.e. Egi 6= 0; so the uncentered estimator will contain an undesirable bias term and the power of the test will be adversely a¤ected. A simple solution is to use the centered moment conditions to construct the weight matrix, as in (11.4) above.
Here is a simple way to compute the e¢ cient GMM estimator for the linear model. First, set W n = (Z0Z) 1, estimate using this weight matrix, and construct the residual e^i = yi x0i :
|
g^ be the associated n |
|
` matrix: Then the e¢ cient GMM estimator is |
||||||||
Then set g^i = zie^i; and let b |
|
|
|
|
b |
||||||
|
= X0Z g^0g^ n |
|
n |
|
n0 1 Z0X 1 X0Z g^0g^ n |
|
n |
|
n0 1 Z0y: |
||
|
g |
g |
g |
g |
|||||||
In most cases, |
when we say “GMM”, we actually mean “e¢ cient GMM”. There is little point in |
||||||||||
b |
|
|
|
|
|
|
using an ine¢ cient GMM estimator when the e¢ cient estimator is easy to compute.
|
^ |
|
|
|
|
An estimator of the asymptotic variance of can be seen from the above formula. Set |
|||||
|
V = n X0Z g^0g^ n |
|
n |
|
n0 1 Z0X 1 : |
|
g |
g |
|||
Asymptotic standard errors |
are given by the square roots of the diagonal elements of V : |
||||
b |
There is an important alternative to the two-step GMM estimator just described. Instead, we |
|||||||
can let the weight matrix be considered as a function of : The criterion function is thenb |
|||||||
|
|
|
1 n |
1 |
|||
|
|
|
|
Xi |
|
|
|
J( ) = n |
g |
n( )0 |
n |
=1 gi ( )gi ( )0! |
|
g |
n( ): |
where
gi ( ) = gi( ) gn( )
b
The which minimizes this function is called the continuously-updated GMM estimator, and was introduced by L. Hansen, Heaton and Yaron (1996).
The estimator appears to have some better properties than traditional GMM, but can be numerically tricky to obtain in some cases. This is a current area of research in econometrics.
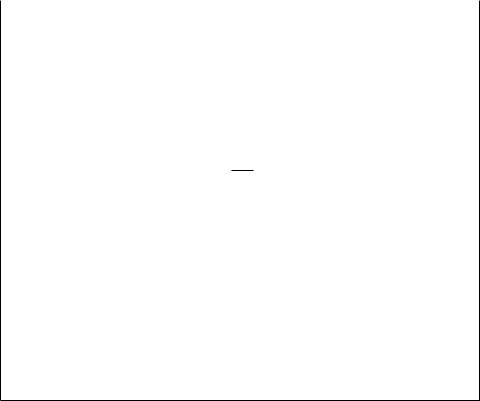
CHAPTER 11. GENERALIZED METHOD OF MOMENTS |
197 |
11.5GMM: The General Case
In its most general form, GMM applies whenever an economic or statistical model implies the ` 1 moment condition
E(gi( )) = 0:
Often, this is all that is known. Identi…cation requires l k = dim( ): The GMM estimator minimizes
J( ) = n |
|
n( )0W n |
|
|
n( ) |
|
|||||||||||
g |
g |
|
|||||||||||||||
where |
|
|
|
|
|
|
n |
|
|
|
|||||||
|
|
1 |
|
|
|
||||||||||||
|
|
|
|
|
|
|
|
Xi |
|
|
|
||||||
|
|
|
|
|
|
|
|
|
|
|
|||||||
gn( ) = |
gi( ) |
|
|
|
|||||||||||||
|
|
|
|
|
|
|
n |
=1 |
|
|
|
|
|
|
|
|
|
and |
|
1 n |
|
|
|
|
|
|
|
! |
1 |
|
|||||
|
|
|
|
|
|
|
|
|
|
|
|||||||
|
|
|
|
Xi |
|
|
|
|
|
|
|
|
|
||||
W n = |
|
n |
=1 g^ig^i0 |
g |
n |
g |
n0 |
|
; |
with g^i = gi( ) constructed using a preliminary consistent estimator , perhaps obtained by …rst
setting W n =eI: Since the GMM |
estimator depends upon the …rst-stage estimator, often the weight |
||||||||
recomputed. This estimator can bee iterated if needed. |
|||||||||
matrix W n is updated, and then b |
|
|
|
|
|
|
|||
|
Theorem 11.5.1 Distribution of Nonlinear GMM Estimator |
|
|||||||
|
Under general regularity conditions, |
|
|
|
|
|
|||
|
where |
|
b |
d |
|
1 |
|
||
|
pn |
0; G0 1G |
|
|
|
||||
|
! N |
|
; |
||||||
|
|
|
= E gigi0 1 |
|
|
|
|
and
@
G = E@ 0 gi( ):
b
The variance of may be estimated by
|
V |
= G^0 ^ 1G^ 1 |
|
where |
b |
|
|
|
^ = n 1 Xi |
g^i g^i0 |
and |
@ |
|
|
|
|
||
G^ |
= n 1 Xi |
|
gi(^): |
@ 0 |
The general theory of GMM estimation and testing was exposited by L. Hansen (1982).
11.6Over-Identi…cation Test
Overidenti…ed models (` > k) are special in the sense that there may not be a parameter valuesuch that the moment condition
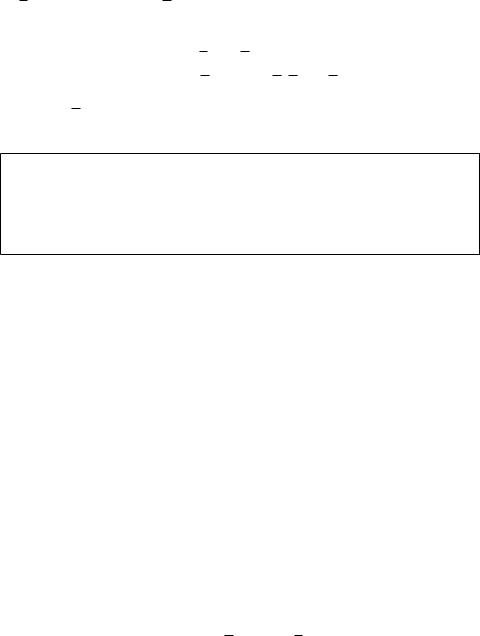
CHAPTER 11. GENERALIZED METHOD OF MOMENTS |
|
|
|
|
|
|
198 |
||||||||
Eg(yi; xi; zi; ) = 0 |
|
|
|
|
|
|
|
|
|
||||||
holds. Thus the model –the overidentifying restrictions –are testable. |
|
|
|
|
|
||||||||||
For example, take the linear model y |
i |
= 0 x |
1i |
+ 0 x |
2i |
+e |
i |
with |
E |
(x |
e ) = 0 and |
E |
(x |
e |
) = 0: |
|
1 |
2 |
|
|
|
1i i |
|
2i i |
|
It is possible that 2 = 0; so that the linear equation may be written as yi = 01x1i + ei: However, it is possible that 2 =6 0; and in this case it would be impossible to …nd a value of 1 so that both E(x1i (yi x01i 1)) = 0 and E(x2i (yi x01i 1)) = 0 hold simultaneously. In this sense an exclusion restriction can be seen as an overidentifying restriction.
p
Note that gn ! Egi; and thus gn can be used to assess whether or not the hypothesis that Egi = 0 is true or not. The criterion function at the parameter estimates is
Jn = n g0nW ngn
= n2g0n g^0g^ ngng0n 1 gn:
is a quadratic form in gn; and is thus a natural test statistic for H0 : Egi = 0:
Theorem 11.6.1 (Sargan-Hansen). Under the hypothesis of correct spec- i…cation, and if the weight matrix is asymptotically e¢ cient,
) |
d |
2 |
: |
Jn = Jn(b |
! |
` k |
|
The proof of the theorem is left as an exercise. This result was established by Sargan (1958) for a specialized case, and by L. Hansen (1982) for the general case.
The degrees of freedom of the asymptotic distribution are the number of overidentifying restrictions. If the statistic J exceeds the chi-square critical value, we can reject the model. Based on this information alone, it is unclear what is wrong, but it is typically cause for concern. The GMM overidenti…cation test is a very useful by-product of the GMM methodology, and it is advisable to report the statistic J whenever GMM is the estimation method.
When over-identi…ed models are estimated by GMM, it is customary to report the J statistic as a general test of model adequacy.
11.7Hypothesis Testing: The Distance Statistic
We described before how to construct estimates of the asymptotic covariance matrix of the GMM estimates. These may be used to construct Wald tests of statistical hypotheses.
If the hypothesis is non-linear, a better approach is to directly use the GMM criterion function. This is sometimes called the GMM Distance statistic, and sometimes called a LR-like statistic (the LR is for likelihood-ratio). The idea was …rst put forward by Newey and West (1987).
For a given weight matrix W n; the GMM criterion function is
Jn( ) = n gn( )0W n gn( )
For h : Rk ! Rr; the hypothesis is
H0 : h( ) = 0:
The estimates under H1 are
b
= argmin Jn( )
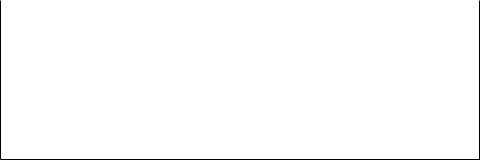
CHAPTER 11. GENERALIZED METHOD OF MOMENTS |
199 |
|
and those under H0 are |
|
|
= argmin J( ): |
|
|
e |
h( )=0 |
|
The two minimizing criterion functions are J ( ) and Jn( ): The GMM distance statistic is the |
||||||
di¤erence |
n b) |
|
J |
|
( ):e |
|
|
Dn = Jn(e |
|
n |
b |
|
|
|
Proposition 11.7.1 If the same weight matrix W n is used for both null |
|||||
|
and alternative, |
|
|
|
|
|
1. D 0
d2
2.D ! r
3.If h is linear in ; then D equals the Wald statistic.
If h is non-linear, the Wald statistic can work quite poorly. In contrast, current evidence suggests that the Dn statistic appears to have quite good sampling properties, and is the preferred test statistic.
Newey and West (1987) suggested to use the same weight matrix W n for both null and alternative, as this ensures that Dn 0. This reasoning is not compelling, however, and some current research suggests that this restriction is not necessary for good performance of the test.
This test shares the useful feature of LR tests in that it is a natural by-product of the computation of alternative models.
11.8Conditional Moment Restrictions
In many contexts, the model implies more than an unconditional moment restriction of the form Egi( ) = 0: It implies a conditional moment restriction of the form
E(ei( ) j zi) = 0
where ei( ) is some s 1 function of the observation and the parameters. In many cases, s = 1. It turns out that this conditional moment restriction is much more powerful, and restrictive,
than the unconditional moment restriction discussed above.
Our linear model yi = x0i + ei with instruments zi falls into this class under the stronger assumption E(ei j zi) = 0: Then ei( ) = yi x0i :
It is also helpful to realize that conventional regression models also fall into this class, except that in this case xi = zi: For example, in linear regression, ei( ) = yi x0i , while in a nonlinear regression model ei( ) = yi g(xi; ): In a joint model of the conditional mean and variance
ei ( ; ) = |
8 |
|
|
yi xi0 |
: |
||
|
: |
(yi |
xi0 )2 |
|
f (xi)0 |
|
|
|
< |
|
|
|
Here s = 2:
Given a conditional moment restriction, an unconditional moment restriction can always be constructed. That is for any ` 1 function (xi; ) ; we can set gi( ) = (xi; ) ei( ) which satis…es Egi( ) = 0 and hence de…nes a GMM estimator. The obvious problem is that the class of functions is in…nite. Which should be selected?

CHAPTER 11. GENERALIZED METHOD OF MOMENTS |
200 |
This is equivalent to the problem of selection of the best instruments. If xi 2 R is a valid instrument satisfying E(ei j xi) = 0; then xi; x2i ; x3i ; :::; etc., are all valid instruments. Which should be used?
One solution is to construct an in…nite list of potent instruments, and then use the …rst k instruments. How is k to be determined? This is an area of theory still under development. A recent study of this problem is Donald and Newey (2001).
Another approach is to construct the optimal instrument. The form was uncovered by Chamberlain (1987). Take the case s = 1: Let
@
Ri = E @ ei( ) j zi
and
2i = E ei( )2 j zi :
Then the “optimal instrument”is
Ai = i 2Ri
so the optimal moment is
gi( ) = Aiei( ):
Setting gi ( ) to be this choice (which is k 1; so is just-identi…ed) yields the best GMM estimator possible.
In practice, Ai is unknown, but its form does help us think about construction of optimal instruments.
In the linear model ei( ) = yi x0i ; note that
Ri = E(xi j zi)
and |
ei2 j zi ; |
i2 = E |
so
Ai = i 2E(xi j zi) :
In the case of linear regression, xi = zi; so Ai = i 2zi: Hence e¢ cient GMM is GLS, as we discussed earlier in the course.
In the case of endogenous variables, note that the e¢ cient instrument Ai involves the estimation of the conditional mean of xi given zi: In other words, to get the best instrument for xi; we need the best conditional mean model for xi given zi, not just an arbitrary linear projection. The e¢ cient instrument is also inversely proportional to the conditional variance of ei: This is the same as the GLS estimator; namely that improved e¢ ciency can be obtained if the observations are weighted inversely to the conditional variance of the errors.
11.9Bootstrap GMM Inference
b
Let be the 2SLS or GMM estimator of . Using the EDF of (yi; zi; xi), we can apply the
b bootstrap methods discussed in Chapter 10 to compute estimates of the bias and variance of ;
and construct con…dence intervals for ; identically as in the regression model. However, caution should be applied when interpreting such results.
A straightforward application of the nonparametric bootstrap works in the sense of consistently achieving the …rst-order asymptotic distribution. This has been shown by Hahn (1996). However, it fails to achieve an asymptotic re…nement when the model is over-identi…ed, jeopardizing the theoretical justi…cation for percentile-t methods. Furthermore, the bootstrap applied J test will yield the wrong answer.

CHAPTER 11. GENERALIZED METHOD OF MOMENTS |
201 |
||||
|
|
|
|
|
^ |
|
|
|
|
|
|
The problem is that in the sample, is the “true”value and yet gn( ) 6= 0: Thus according to |
|||||
random variables (yi ; zi ; xi ) drawn |
from the EDF F |
; |
|
|
|
b |
n |
|
|
|
E gi |
b |
|
|
^ |
|
||||
= gn( ) 6= 0: |
||||
|
|
|
|
|
This means that (yi ; zi ; xi ) do not satisfy the same moment conditions as the population distribution.
A correction suggested by Hall and Horowitz (1996) can solve the problem. Given the bootstrap sample (y ; Z ; X ); de…ne the bootstrap GMM criterion
^ 0 ^
Jn( ) = n gn( ) gn( ) W n gn( ) gn( )
where |
g |
n( ) is from the in-sample data, not from the bootstrap data. |
|
|
||
Let bminimize Jn( ); and de…ne all statistics and tests accordingly. In the linear model, this |
||||||
|
that the bootstrap estimator is |
|
|
|||
implies b |
|
n = X0Z W nZ0X 1 X0Z W n Z0y Z0e^ : |
|
|
||
where e^ = y |
|
b |
|
|
||
|
X are the in-sample residuals. The bootstrap J statistic is J |
( ): |
||||
|
|
|
|
n |
|
|
Brown and |
Newey (2002) have an alternative solution. They note that we can sample from |
|||||
b |
b |
|
the observations with the empirical likelihood probabilities p^i described in Chapter 12. Since |
||
P |
n |
b |
|
i=1 p^igi |
= 0; this sampling scheme preserves the moment conditions of the model, so no |
recentering or adjustments is needed. Brown and Newey argue that this bootstrap procedure will be more e¢ cient than the Hall-Horowitz GMM bootstrap.
CHAPTER 11. GENERALIZED METHOD OF MOMENTS |
202 |
Exercises
Exercise 11.1 Take the model
yi = x0i + ei
E(xiei) = 0
e2i = z0i + i
E(zi i) = 0:
^
Find the method of moments estimators ; ^ for ( ; ) :
Exercise 11.2 Take the single equation |
|
|
|
|
|
|
|
|
|
|
|
|
|
|
||||||||
|
|
|
|
|
|
|
|
|
|
y |
= |
X + e |
|
|
|
|
|
|
|
|||
|
E ei |
j zi |
|
|
|
E(e j Z) |
= |
0 |
|
|
|
|
|
|
|
|
|
|||||
then |
: Show that if ^ |
is |
d |
|
|
|
|
|
1 |
|
|
n |
0 |
Z) 1 ; |
||||||||
Assume |
|
2 |
= 2 |
|
|
estimated by GMM with weight matrix W |
|
|
= (Z |
|||||||||||||
|
|
|
|
|
|
|
b |
|
|
|
0; 2 |
|
|
|
|
|
|
|
|
|
|
|
|
|
|
|
|
|
|
|
|
|
|
|
|
|
|
|
|
||||||
|
|
|
|
pn ! N |
|
|
Q0M 1Q |
|
|
|
|
|
|
|||||||||
where Q = |
E |
(zix0) and M = |
E |
(ziz0) : |
|
|
|
|
|
|
|
|
|
|
|
|
|
|
|
|||
|
|
i |
|
i |
|
|
|
|
|
|
|
|
|
|
|
|
|
|
|
|||
Exercise 11.3 Take the model yi = x0 |
+ ei |
with |
E |
(ziei) = 0: Let e^i = yi |
|
x0 |
where is |
|||||||||||||||
|
|
|
|
|
|
|
|
i |
|
|
|
|
|
|
|
i |
|
|
|
consistent for (e.g. a GMM estimator with arbitrary weight matrix). De…ne the estimate of the |
|||||
optimal GMM weight matrix |
1 n |
zizi0e^i2! |
b |
b |
|
W n = |
1 |
|
|||
|
|
: |
|
||
n |
|
||||
|
=1 |
|
|
|
|
|
|
Xi |
|
|
|
p |
zizi0ei2 : |
Show that W n ! 1 where = E |
Exercise 11.4 In the linear model estimated by GMM with general weight matrix W ; the asymp-
^
totic variance of GMM is
V= Q0W Q 1 Q0W W Q Q0W Q 1
(a)Let V 0 be this matrix when W = 1: Show that V 0 = Q0 1Q 1 :
(b)We want to show that for any W ; V V 0 is positive semi-de…nite (for then V 0 is the smaller possible covariance matrix and W = 1 is the e¢ cient weight matrix). To do this, start by …nding matrices A and B such that V = A0 A and V 0 = B0 B:
(c)Show that B0 A = B0 B and therefore that B0 (A B) = 0:
(d) Use the expressions V = A0 A; A = B + (A |
|
B) ; and B0 |
(A |
|
B) = 0 to show that |
|
V V 0: |
|
|
|
|
||
|
|
|
|
|
|
|
Exercise 11.5 The equation of interest is |
|
|
|
|
|
|
yi |
= g(xi; ) + ei |
|
|
|
||
E(ziei) |
= 0: |
|
|
|
|
|
The observed data is (yi; zi; xi). zi is ` 1 and is k 1; ` k: Show how to construct an e¢ cient GMM estimator for .