
- •2. General Information, Conversion Tables, and Mathematics
- •Table 2.6 Abbreviations and Standard Letter Symbols
- •Table 2.7 Conversion Factors
- •2.1.1 Conversion of Thermometer Scales
- •2.1.3 Barometry and Barometric Corrections
- •Table 2.13 Viscosity Conversion Table
- •Table 2.15 Hydrometer Conversion Table
- •Table 2.16 Pressure Conversion Chart
- •Table 2.17 Corrections to Be Added to Molar Values to Convert to Molal
- •Table 2.21 Transmittance-Absorbance Conversion Table
- •2.2 Mathematical Tables
- •2.2.1 Logarithms
- •2.3 Statistics in Chemical Analysis
- •2.3.1 Introduction
- •2.3.2 Errors in Quantitative Analysis
- •2.3.3 Representation of Sets of Data
- •2.3.4 The Normal Distribution of Measurements
- •2.3.5 Standard Deviation as a Measure of Dispersion
- •2.3.7 Hypotheses About Means
- •2.3.10 Curve Fitting
- •2.3.11 Control Charts
- •Bibliography
GENERAL INFORMATION, CONVERSION TABLES, AND MATHEMATICS |
2.133 |
2.3.10Curve Fitting
Very often in practice a relationship is found (or known) to exist between two or more variables. It is frequently desirable to express this relationship in mathematical form by determining an equation
connecting the variables. |
|
|
|
||||
|
The first step is the collection of data showing corresponding values of the variables under |
||||||
consideration. From a scatter diagram, a plot of |
Y (ordinate) versus |
X (abscissa), it is often possible |
|||||
to visualize a smooth curve approximating the data. For purposes of reference, several types of |
|||||||
approximating curves and their equations are listed. All letters other than |
X and Y represent constants. |
||||||
1. |
Y |
a 0 |
a 1X |
|
|
Straight line |
|
2. |
Y |
a 0 |
a 1X |
a 2X 2 |
|
Parabola or quadratic curve |
|
3. |
Y |
a 0 |
a 1X |
a 2X 2 a 3 X |
3 |
Cubic curve |
|
4. |
Y |
a 0 |
a 1X |
a 2 · · |
· a n X n |
n th degree curve |
|
As other possible equations (among many) used in practice, these may be mentioned:
5. |
Y |
(a 0 |
a 1X ) 1 |
|
or 1/Y |
a 0 a 1X |
Hyperbola |
||||
6. |
Y |
ab |
X |
|
or |
log |
Y |
log |
a |
(log b )X |
Exponential curve |
7. |
Y |
aX |
b |
or |
log |
Y |
log |
a |
b log X |
Geometric curve |
|
8. |
Y |
ab |
X |
|
g |
|
|
|
|
|
Modified exponential curve |
9. |
Y |
aX |
n |
g |
|
|
|
|
|
Modified geometric curve |
When we draw a scatter plot of all |
X |
versus |
Y data, we see |
that some sort of shape can be |
||||||||
described by the data points. From the scatter plot we can take a basic guess as to which type of |
|
|
||||||||||
curve will best describe the |
X |
9Y |
relationship. To aid in the decision process, it is helpful to obtain |
|||||||||
scatter plots of transformed variables. For example, if a scatter plot of log |
|
|
Y versus |
X |
shows a linear |
|||||||
relationship, the equation has the form of number 6 above, while if log |
|
Y |
versus log |
X |
shows a linear |
|||||||
relationship, the equation has the form of number 7. To facilitate this we frequently employ special |
|
|||||||||||
graph paper for which one or both scales are calibrated logarithmically. These are referred to as |
|
|
||||||||||
semilog |
or |
log-log graph paper |
, respectively. |
|
|
|
|
|
|
|||
2.3.10.1 |
The |
Least Squares or |
Best-fit Line. |
|
|
The simplest type of approximating curve is a |
||||||
straight line, the equation of which can be written as in form number 1 above. It is customary to |
|
|
||||||||||
employ the above definition when |
|
X is the independent variable and |
Y |
is the dependent variable. |
||||||||
To avoid individual judgment in constructing any approximating curve to fit sets of data, it is |
||||||||||||
necessary to agree on a definition of a |
best-fit line |
. One could construct what would be considered |
||||||||||
the best-fit line through the plotted pairs of data points. For a given |
value |
of |
X |
1, |
there will be a |
|||||||
difference |
D 1 between |
the value |
|
Y 1 and the constituent value |
Yˆ as determined by the calibration |
|||||||
model. Since we are assuming that all the |
errors are |
in |
Y , |
we are seeking the best-fit line that |
||||||||
minimizes the deviations in the |
Y |
direction between the experimental points and the calculated line. |
||||||||||
This condition will be met when the sum of squares for the differences, called residuals (or the sum |
|
|
||||||||||
of squares due to error), |
|
|
|
|
|
|
|
|
|
|
N (Y i Yˆi )2 (D 21 D 22 · · · D 2N )
i 1
is the least possible value when compared to all other possible lines fitted to that data. If the sum
of squares for residuals is equal to zero, the calibration line is a perfect fit to the data. With a

2.134 SECTION 2
mathematical treatment known as linear regression, one can find the “best” straight line through these real world points by minimizing the residuals.
This calibration model for the best-fit fit line requires that the line pass through the “centroid”
of the points (X |
, Y ).It can be shown that: |
|
i |
|
|
|
|
|
|
|
|
|
|
|
|
|
|
|
|
|
|
|
|
|
|
|
|
||||||||||
|
|
|
|
|
|
|
|
|
(X i X |
|
) (Y i Y |
) |
|
|
|
|
(2.17) |
||||||||||||||||||||
|
|
|
|
|
|
|
|
b |
i |
|
|
|
|
|
|
|
|
|
|
|
|
|
|
|
|||||||||||||
|
|
|
|
|
|
|
|
(X i X |
)2 |
|
|
|
|
|
|
|
|
|
|
|
|
|
|||||||||||||||
|
|
|
|
|
|
|
|
|
|
|
a |
|
|
|
|
bX |
|
|
|
|
|
|
|
|
|
|
|
(2.18) |
|||||||||
|
|
|
|
|
|
|
|
|
|
|
Y |
|
|
|
|
|
|
|
|
|
|
|
|
|
|||||||||||||
The line thus calculated is known as the line of regression of |
|
|
|
|
|
|
|
|
Y on X , that is, the line indicating how |
||||||||||||||||||||||||||||
Y varies when |
X is set to chosen values. |
|
|
|
|
|
|
|
|
|
|
|
|
|
|
|
|
|
|
|
|
|
|
|
|
|
|
|
|
|
|
||||||
If X |
is the dependent variable, the definition is modified by considering horizontal instead of |
||||||||||||||||||||||||||||||||||||
vertical deviations. In general these two definitions lead to different least square curves. |
|
|
|
|
|
|
|||||||||||||||||||||||||||||||
Example |
13 |
The following data were recorded for the potential |
|
|
E |
|
of an |
electrode, measured |
|||||||||||||||||||||||||||||
against the saturated calomel electrode, as a function of concentration |
|
|
C |
|
(moles liter 1). |
||||||||||||||||||||||||||||||||
|
|
|
|
|
|
|
|
|
|
|
|
|
|
|
|
|
|
|
|
|
|
|
|
|
|
|
|
|
|
|
|
|
|
|
|||
|
|
|
|
|
|
|
log C |
E |
, mV |
|
|
|
|
|
|
|
log |
C |
|
E , |
mV |
|
|
|
|
|
|
||||||||||
|
|
|
|
|
|
|
|
|
|
|
|
|
|
|
|
|
|
|
|
|
|
|
|
|
|
|
|
|
|
|
|||||||
|
|
|
|
1.00 |
|
106 |
|
|
|
|
|
|
2.10 |
174 |
|
|
|
|
|
|
|
||||||||||||||||
|
|
|
|
1.10 |
|
115 |
|
|
|
|
|
|
2.20 |
182 |
|
|
|
|
|
|
|
||||||||||||||||
|
|
|
|
1.20 |
|
121 |
|
|
|
|
|
|
2.40 |
187 |
|
|
|
|
|
|
|
||||||||||||||||
|
|
|
|
1.50 |
|
139 |
|
|
|
|
|
|
2.70 |
|
211 |
|
|
|
|
|
|
|
|||||||||||||||
|
|
|
|
1.70 |
|
153 |
|
|
|
|
|
|
2.90 |
|
220 |
|
|
|
|
|
|
|
|||||||||||||||
|
|
|
|
1.90 |
|
158 |
|
|
|
|
|
|
3.00 |
|
226 |
|
|
|
|
|
|
|
|||||||||||||||
|
|
|
|
|
|
|
|
|
|
|
|
|
|
|
|
|
|
|
|
|
|
|
|
|
|
||||||||||||
Fit the best straight line to these data; |
X |
i represents |
|
log |
C |
, and Y i represents |
E . We will perform |
||||||||||||||||||||||||||||||
the calculation manually, using the following tabular lay-out. |
|
|
|
|
|
|
|
|
|
|
|
|
|
|
|
|
|
|
|
|
|
|
|
|
|||||||||||||
|
|
|
|
|
|
|
|
|
|
|
|
|
|
|
|
|
|
|
|
|
|
|
|
|
|
|
|
||||||||||
|
|
|
X i |
|
|
Y i |
|
|
|
|
|
|
|
|
|
|
|
|
|
|
|
|
|
|
|
|
|||||||||||
|
|
|
(X i X |
) |
|
|
|
|
(X i X )2 |
|
|
(Y i Y ) |
(X i X )(Y i Y ) |
||||||||||||||||||||||||
|
|
|
|
|
|
|
|
|
|
|
|
|
|
|
|
|
|
|
|
|
|
||||||||||||||||
1.00 |
106 |
0.975 |
|
|
0.951 |
|
|
|
|
60 |
|
|
|
58.5 |
|
|
|
||||||||||||||||||||
1.10 |
115 |
0.875 |
|
|
|
|
|
|
0.766 |
|
|
|
51 |
|
|
|
44.6 |
|
|
|
|||||||||||||||||
1.20 |
121 |
0.775 |
|
|
|
|
|
|
0.600 |
|
|
|
45 |
|
|
|
34.9 |
|
|
|
|||||||||||||||||
1.50 |
139 |
0.475 |
|
|
|
|
|
|
0.226 |
|
|
|
27 |
|
|
|
12.8 |
|
|
|
|||||||||||||||||
1.70 |
153 |
0.275 |
|
|
|
|
|
|
0.076 |
|
|
|
13 |
|
|
|
3.6 |
|
|
|
|||||||||||||||||
1.90 |
158 |
0.075 |
|
|
|
|
|
|
0.006 |
|
|
|
8 |
|
|
|
0.6 |
|
|
|
|||||||||||||||||
2.10 |
174 |
0.125 |
|
|
|
|
|
|
0.016 |
|
|
8 |
|
|
|
1.0 |
|
|
|
||||||||||||||||||
2.20 |
182 |
0.225 |
|
|
|
|
|
|
0.051 |
|
16 |
|
|
|
3.6 |
|
|
|
|||||||||||||||||||
2.40 |
187 |
0.425 |
|
|
|
|
|
|
0.181 |
|
21 |
|
|
|
8.9 |
|
|
|
|||||||||||||||||||
2.70 |
211 |
0.725 |
|
|
|
|
|
|
0.526 |
|
45 |
|
|
|
32.6 |
|
|
|
|||||||||||||||||||
2.90 |
220 |
0.925 |
|
|
|
0.856 |
|
|
54 |
|
|
|
|
50.0 |
|
|
|
||||||||||||||||||||
3.00 |
226 |
1.025 |
|
|
1.051 |
|
|
60 |
|
|
|
61.5 |
|
|
|
||||||||||||||||||||||
|
|
|
|
|
|
|
|
|
|
|
|
|
|
|
|
|
|
|
|
|
|||||||||||||||||
|
X |
i 23.7 |
Y i 1992 |
0 |
|
|
|
|
5.306 |
|
|
0 |
|
|
|
312.6 |
|||||||||||||||||||||
|
|
1.975 |
|
|
|
|
|
|
|
|
|
|
|
|
|
|
|
|
|
|
|
|
|
|
|
|
|
|
|
|
|
|
|
|
|
||
|
X |
Y 166 |
|
|
|
|
|
|
|
|
|
|
|
|
|
|
|
|
|
|
|
|
|
|
|
|
|
|
|
|
|
|
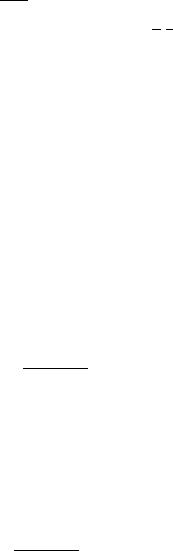
GENERAL INFORMATION, CONVERSION TABLES, AND MATHEMATICS |
2.135 |
Now substituting the proper terms into Equation 17, the slope is:
b312.6 58.91 5.306
and from Equation 18, and substituting the “centroid” values of the points |
, the intercept(X , Y ) |
is: |
||||||||||||||||||
|
|
a |
166 58.91(1.975) |
49.64 |
|
|
||||||||||||||
The best-fit equation is therefore: |
|
|
|
|
|
|
|
|
|
|
|
|
|
|
|
|
|
|
||
|
|
|
E 49.64 58.91 log |
|
C |
|
|
|
|
|
||||||||||
2.3.10.2 Errors |
in the Slope and |
Intercept of the |
Best-fit |
Line. |
|
|
|
|
|
|
|
|
|
|
Upon examination of the plot |
|||||
of pairs of data points, the calibration line, it will be obvious that the precision involved in analyzing |
||||||||||||||||||||
an unknown sample will be considerably poorer than that indicated by replicate error alone. The |
|
|||||||||||||||||||
scatter of these original points about the calibration line is a good measure of the error to be expected |
|
|||||||||||||||||||
in analyzing an unknown sample. And this same error is considerably larger than the replication |
||||||||||||||||||||
error because it will include other sources of variability due to a variety of causes. One possible |
|
|||||||||||||||||||
source of variability might be the presence of different amounts of an extraneous material in the |
|
|||||||||||||||||||
various samples used to establish the calibration curve. While this variability causes scatter about |
|
|||||||||||||||||||
the calibration curve, it will not be reflected in the replication error of any one sample if the sample |
||||||||||||||||||||
is homogeneous. |
|
|
|
|
|
|
|
|
|
|
|
|
|
|
|
|
|
|
|
|
The scatter of the points around the calibration line or random errors are of importance since the |
|
|||||||||||||||||||
best-fit line will be used to estimate the concentration of test samples by interpolation. The method |
|
|||||||||||||||||||
used to calculate the random errors in the values for the slope and intercept is now considered. We |
|
|||||||||||||||||||
must first calculate the standard deviation |
|
s Y/X , which is given by: |
|
|
||||||||||||||||
|
|
|
|
|
|
|
i |
(Y i Yˆ)2 |
|
|
|
|
|
|||||||
|
|
|
s Y/X |
q |
|
N |
2 |
|
|
|
|
(2.19) |
||||||||
Equation 19 utilizes the |
Y-residuals |
, Y i |
Yˆ, where |
|
Yˆi are the points on the calculated best-fit line |
|||||||||||||||
or the fitted |
Y i values. The appropriate number of degrees of freedom is |
|
|
|
|
|
|
N 2; the minus 2 arises |
||||||||||||
from the fact that linear calibration lines are derived from both a slope and an intercept which leads |
|
|||||||||||||||||||
to a loss of two degrees of freedom. |
|
|
|
|
|
|
|
|
|
|
|
|
|
|
|
|
|
|
||
Now we can calculate the standard deviations for the slope and |
the |
intercept. These are |
|
|||||||||||||||||
given by: |
|
|
|
|
|
|
|
|
|
|
|
|
|
|
|
|
|
|
|
|
|
|
|
s b |
|
|
|
|
s Y/X |
|
|
|
|
|
|
|
|
|
(2.20) |
||
|
|
|
q |
i |
|
|
|
|
|
|
|
|
||||||||
|
|
|
|
(X i X |
)2 |
|
|
|
|
|
|
|||||||||
|
|
|
|
|
|
|
|
|
|
|
|
|
|
|
|
|
|
|||
|
|
|
s a sY/X q |
|
|
i |
X i2 |
|
|
|
|
(2.21) |
||||||||
|
|
|
N |
|
i |
(X i |
|
|
|
)2 |
|
|
||||||||
|
|
|
|
X |
|
|
|
2.136 |
|
|
|
|
|
|
|
SECTION |
|
2 |
|
|
|
|
|
|
|
|
|
||||
The confidence limits for the slope are given by |
|
|
|
|
|
b tb , where the |
t-value is taken at the desired |
|
|||||||||||||||
confidence level and ( |
N 2) degrees of freedom. Similarly, the confidence limits for the intercept |
|
|||||||||||||||||||||
are given by |
a |
ts a |
. The closeness of |
|
xˆ to |
x i is answered in terms of a confidence interval for |
x 0 |
||||||||||||||||
that extends from an upper confidence (UCL) to a lower confidence (LCL) level. Let us choose 95% |
|
||||||||||||||||||||||
for the confidence interval. Then, remembering that this is a two-tailed test (UCL and LCL), we |
|
||||||||||||||||||||||
obtain from a table of Student’s |
|
t |
distribution the critical value of |
tc (t0.975 ) and the appropriate |
|||||||||||||||||||
number of degrees of freedom. |
|
|
|
|
|
|
|
|
|
|
|
|
|
|
|
|
|
|
|
|
|
||
Example 14 |
|
For the best-fit line found in Example 13, express the result in terms of confidence |
|
||||||||||||||||||||
intervals for the slope and intercept. We will choose 95% for the confidence interval. |
|
||||||||||||||||||||||
The standard |
deviation |
s Y/X is given |
by Equation 19, |
but first a supplementary table must be |
|
||||||||||||||||||
constructed for the |
Y |
residuals and other data which will be needed in subsequent equations. |
|
||||||||||||||||||||
|
|
|
|
|
|
|
|
|
|
|
|
|
|
|
|
|
|
|
|
||||
|
|
|
|
Yˆ |
|
(Y i Yˆ) |
|
|
|
(Y i Yˆ)2 |
X i2 |
|
|
||||||||||
|
|
|
108.6 |
|
|
2.55 |
|
|
|
|
|
|
|
|
6.50 |
1.00 |
|
||||||
|
|
|
114.4 |
|
0.56 |
|
|
|
|
|
|
|
|
|
0.31 |
1.21 |
|
||||||
|
|
|
120.3 |
|
0.67 |
|
|
|
|
|
|
|
|
|
0.45 |
1.44 |
|
||||||
|
|
|
138.0 |
|
1.00 |
|
|
|
|
|
|
|
|
|
1.00 |
2.25 |
|
||||||
|
|
|
149.8 |
|
3.21 |
|
|
|
|
|
|
|
|
10.32 |
2.89 |
|
|
||||||
|
|
|
161.6 |
|
|
3.57 |
|
|
|
|
|
|
|
|
12.94 |
3.61 |
|
|
|||||
|
|
|
173.4 |
|
0.65 |
|
|
|
|
|
|
|
|
|
0.42 |
4.41 |
|
||||||
|
|
|
179.2 |
|
2.76 |
|
|
|
|
|
|
|
|
|
7.61 |
4.84 |
|
||||||
|
|
|
191.0 |
|
|
4.02 |
|
|
|
|
|
|
|
|
16.16 |
5.76 |
|
||||||
|
|
|
208.7 |
|
2.30 |
|
|
|
|
|
|
|
|
|
5.30 |
7.29 |
|
|
|||||
|
|
|
220.5 |
|
|
0.48 |
|
|
|
|
|
|
|
|
0.23 |
8.41 |
|
||||||
|
|
|
226.4 |
|
|
0.40 |
|
|
|
|
|
|
|
|
0.16 |
9.00 |
|
||||||
|
|
|
|
|
|
|
|
|
|
|
|
|
|
|
|
|
|
|
|
|
|
||
|
|
|
|
|
|
|
61.20 |
|
|
|
|
52.11 |
|
|
|
||||||||
Now substitute the appropriate values into Equation 19 where there are 12 |
|
2 10 degrees of |
|
||||||||||||||||||||
freedom: |
|
|
|
|
|
|
|
|
|
|
|
|
|
|
|
|
|
|
|
|
|
|
|
|
|
|
|
|
|
|
|
|
|
|
|
|
|
|
|
|
|
|
|
|
|
|
|
|
|
|
|
|
|
|
s X/Y |
q |
61.20 |
|
2.47 |
|
|
|
|||||||||
|
|
|
|
|
|
|
|
10 |
|
|
|
|
|||||||||||
We can now calculate |
|
s b |
and |
s a |
from Equations 20 and 21, respectively: |
|
|||||||||||||||||
|
|
|
|
|
|
|
s b |
s Y/X |
|
1.07 |
|
|
|
||||||||||
|
|
|
|
|
|
|
|
|
|
|
|
|
|
|
|
||||||||
|
|
|
|
|
|
|
|
|
p5.31 |
|
|
|
|
|
|
|
|||||||
and |
|
|
|
|
|
|
|
|
|
|
|
|
|
|
|
|
|
|
|
|
|
|
|
|
|
|
|
|
|
|
|
|
|
|
|
|
|
|
|
|
|
|
|
|
|||
|
|
|
|
|
|
s a |
2.47 q |
|
|
52.11 |
|
2.23 |
|
|
|
||||||||
|
|
|
|
|
|
12(5.306) |
|
|
|
||||||||||||||
Now, using a two-tailed value for Student’s |
|
|
t: |
|
|
|
|
|
|
|
|
|
|
|
|||||||||
|
|
|
|
b |
ts b |
58.91 2.23(1.07) |
58.91 2.39 |
|
|||||||||||||||
|
|
|
|
a |
ts a |
49.64 2.23(2.23) |
49.64 4.97 |
|