
Bradley, Manna. The Calculus of Computation, Springer, 2007
.pdf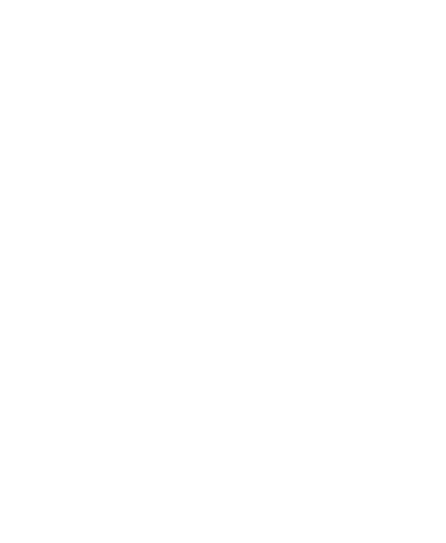
12.2 Interval Analysis |
331 |
of Example 12.4 with paths
(1)
@L0 : i = 0 n ≥ 0; @L1 : ?;
and
(2)
@L1 : ?; assume i < n; i := i + 1; @L1 : ?;
Initially,
µ(L0) νDI (i = 0 n ≥ 0) 0 ≤ i i ≤ 0 0 ≤ n .
After one iteration, following path (1), µ(L1) µ(L0).
Following path (2) on the next iteration yields
µ(L1) := µ(L1) DI spDI (µ(L1), assume i < n; i := i + 1) .
Currently µ(L1) 0 ≤ i i ≤ 0 0 ≤ n, so
sp(0 ≤ i i ≤ 0 0 ≤ n, assume i < n; i := i + 1)sp(0 ≤ i i ≤ 0 0 ≤ n, i := i + 1)
1 ≤ i i ≤ 1 0 ≤ n
Then the new µ(L1) is |
|
|
|
|
|
µ(L1) := µ(L1) DI spDI (· · · ) |
|
|
|
||
|
(0 ≤ i i ≤ 0 0 ≤ n) DI (1 ≤ i i ≤ 1 0 ≤ n) |
||||
|
0 ≤ i i ≤ 1 0 ≤ n |
|
|
|
|
Since the implication |
|
|
|
|
|
0 ≤ i i ≤ 1 0 ≤ n |
0 ≤ i i ≤ 0 0 ≤ n |
||||
| |
{z } |
| |
old{z( |
) |
} |
|
new µ(L1) |
|
µ L1 |
|
|
is invalid,
µ(L1) 0 ≤ i i ≤ 1 0 ≤ n
at the end of the iteration.
At the end of the kth iteration,
µ(L1) 0 ≤ i i ≤ k 0 ≤ n .

332 12 Invariant Generation
It is never the case that the implication
0 ≤ i i ≤ k 0 ≤ n 0 ≤ i i ≤ k − 1 0 ≤ n
is valid, so the main loop of AbstractForwardPropagate never finishes.
Hence, we need to define a widening operator DI . First, for any F DI,
F DI F and |
DI F F . |
Otherwise, let F, G DI |
be other than . For each variable v, suppose that |
F asserts v [ℓ1, u1] and G asserts v [ℓ2, u2]. Then F DI G asserts that v [ℓ, u], where
•ℓ = −∞ if ℓ2 < ℓ1, and otherwise ℓ = ℓ1;
•u = ∞ if u2 > u1, and otherwise u = u1.
Intuitively, F DI G drops bounds that grow from F to G. Since at most only twice as many finite bounds as variables exist, widening can only be applied a finite number of times before all bounds become stable.
Example 12.11. On the kth iteration (for some small k, say, k = 3) of the analysis in Example 12.10, compute
µ(L1) := µ(L1) DI (µ(L1) DI spDI (µ(L1), assume i < n; i := i + 1)) .
That is,
(0 ≤ i i ≤ k − 1 0 ≤ n) DI (0 ≤ i i ≤ k 0 ≤ n)0 ≤ i 0 ≤ n
because the upper bound on i increases from k − 1 to k. Then
µ(L1) 0 ≤ i 0 ≤ n .
While this new µ(L1) does not imply the previous one,
0 ≤ i 0 ≤ n 60 ≤ i i ≤ k − 1 0 ≤ n ,
one more iteration yields the same µ(L1), finishing the analysis. Thus,
0 ≤ i 0 ≤ n
is an inductive assertion at L1.
Unfortunately, the interval abstract domain is incapable of representing the more interesting invariant i ≤ n.
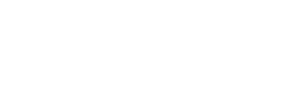
12.3 Karr’s Analysis |
333 |
12.3 Karr’s Analysis
Karr’s analysis discovers inductive assertions of the form
c0 + c1x1 + · · · + cnxn = 0 ,
for ci Z and program variables xi. Such assertions are called a ne assertions. They are useful for tracking the relationship among program variables and loop counters. Karr’s analysis can be implemented e ciently, with running time polynomial in the program size.
In this section, we present a simplified version of the analysis that Michael Karr originally proposed. In particular, our analysis ignores guards of loops and if statements. We use the notation and concepts from Section 8.2.
Example 12.12. Consider the loop
@L0 : ; i := 0;
j := 0; k := 0; while
@L1 : ? ( ) {
k := k + 1;
if ( ) i := i + 1; else j := j + 1;
}
The guard denotes nondeterministic choice: either branch can be taken. Karr’s analysis discovers the inductive invariant i + j = k at L1.
Step 1: Construct the domain DK.
DK consists of , , and conjunctions of literals of the form
c0 + c1x1 + · · · + cnxn = 0 ,
which define a ne spaces. An a ne space is a point, a line, a plane, etc. An a ne space can be specified by a set of equations
^
Ax = b , abbreviating ai1x1 + · · · + ainxn = bi ,
i
so that the space is given by points v satisfying Av = b; or by a finite set of points V = {v1, . . . , vk }, so that the space is given by
( |
) |
XX
a ne(V ) = |
λivi : λi = 1 , |
ii

334 12 Invariant Generation
that is, the set of a ne combinations of vectors in V . An a ne combination of vectors V is a weighted sum
X |
X |
λivi such that |
λi = 1 . |
i |
i |
For example, the a ne combination of two disjoint points is a line passing through both. These two representations are the constraint representation and the vertex representation, respectively.
Example 12.13. The a ne space represented in constraint form by
i + j = k |
|
|
|
|
|
|
|
|
|
|
|
|
||||
has vertex representation |
|
|
|
|
|
|
|
|
||||||||
0 , |
0 , |
|
1 |
, |
|
|
|
|
|
|
|
|||||
|
|
0 |
|
1 |
|
|
0 |
|
|
|
|
|
|
|
|
|
|
0 |
|
1 |
|
|
1 |
|
|
|
|
|
|
|
|
||
|
|
|
|
|
|
|
|
|
|
|
|
|
|
|||
|
|
|
|
|
|
|
|
|
|
|
|
|
|
T |
||
i + j = k. |
|
|
|
|
|
|
|
|
|
|
. Confirm that each vertex satisfies |
|||||
where vectors represent values for i j k |
|
|
||||||||||||||
The point |
1) |
|
|
|
|
|
|
|
|
|
|
|
||||
|
1 |
= ( |
|
0 |
+ (1) |
0 |
+ (1) |
1 |
||||||||
|
1 |
|
|
|
|
0 |
|
|
|
1 |
|
|
|
0 |
|
|
2 |
− 0 |
|
1 |
|
1 |
is in the a ne space because λ1 + λ2 + λ3 = −1 + 1 + 1 = 1: it is an a ne combination of the vertices.
The vertex representation is best suited for the version of Karr’s analysis that we present. Recall, though, that the abstract domain is really the set of ΣQ-formulae that are conjunctions of literals of the form
c0 + c1x1 + · · · + cnxn = 0 .
The vertex representation of domain elements is convenient for computation.
Step 2: Construct the map νDK .
This step is trivial, as this version of Karr’s analysis does not use information from annotations or assumption statements. Hence,
νDK (F ) = .

12.3 Karr’s Analysis |
335 |
Step 3: Define sp.
Let
spDK (F, assume c) F
for any F and c. That is, ignore assumption statements. Ignoring assumption statements is not a terrible loss in precision: at best, only a ne guards c : Ax = b could be interpreted within DK. Such guards are uncommon in practice.
Consider assignment xk := e, where e is an a ne expression
e0 + e1x1 + · · · + enxn ,
for ei Q. Construct the a ne transformation
|
1 |
1 |
|
|
|
|
|
|
0 |
|
|||
|
|
|
|
|
|
|
|
|
|
|
0 |
|
|
Ax + b : |
|
|
|
.. |
|
|
|
.. |
|
+ |
.. |
||
|
e |
|
e |
|
en |
. |
e |
|
|
||||
|
|
. |
|
x1 |
|
|
|||||||
|
|
|
|
|
|
|
|
|
. |
|
|||
|
1 |
|
2 |
|
|
|
|
|
0 |
||||
|
|
|
|
.· · · |
|
|
|
xn |
|
|
. |
|
|
|
|
|
|
. |
|
|
|
|
. |
|
|||
|
|
|
. |
|
|
|
|
. |
|||||
|
|
|
|
|
|
|
|
|
|
|
|
|
|
|
|
|
|
|
1 |
|
|
|
|
|
0 |
|
|
|
|
|
|
|
|
|
|
|
|
|
|||
|
|
|
|
|
|
|
|
|
|
|
|
|
|
where the row with e is the kth row, corresponding to xk , and the rest of the matrix is the identity matrix. Abbreviate this transformation with the notation [[xk := e]].
Now consider an a ne space F represented by a set of vertices VF . To compute the e ect of applying the assignment xk := e, apply [[xk := e]]:
[[xk := e]]F = [[xk := e]]a ne(VF ) = a ne{[[xk := e]]v : v VF } .
The transformed a ne space is given by applying [[xk := e]] to each of the vertices of VF . Then
spDK (F, xk := e) [[xk := e]]F
for a ne expression e.
Example 12.14. Consider the a ne space given by vertex representation
V = |
0 |
, |
|
|
|
|
|
1 |
|
|
|
|
1 |
|
|
||
|
|
|
|
|
|
|
|
|
|
T, and assignment i := 2i + j + 3. The assignment corre- |
|
for variables |
i j k |
|
|||
sponds to |
transformation |
||||
|
|
|
|
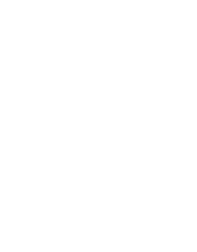
336 |
12 |
Invariant Generation |
|||||
|
2 1 0 |
i |
|
|
|
3 |
. |
0 1 0 |
j |
+ |
0 |
||||
|
0 0 1 |
k |
|
|
|
0 |
|
Then |
|
|
|
|
|
|
|
= |
|
|
. |
[[i := 2i + j + 3]]V = |
0 1 0 |
0 |
+ |
0 |
0 |
||||||
|
2 1 0 |
|
1 |
|
|
|
3 |
|
|
5 |
|
0 0 1 |
|
1 |
|
|
|
0 |
1 |
||||
|
|
|
|
|
|
|
|
|
|||
|
|
|
|
|
|
|
|
|
|
|
|
For assignments xk := e in which e is not an a ne expression, define the a ne hull DK of two a ne spaces F1 and F2 with vertex representations V and W , respectively, as
F1 DK |
F2 |
= |
|
λivi + µj wj : |
i |
λi + |
µj = 1 . |
|
|
|
i |
j |
j |
|
|
|
|
|
X |
X |
X |
X |
|
|
|
|
|
|
|
|
To implement this definition, simply let U = V W be the vertex representation of F1 DK F2. Then
( |
) |
XX
F1 DK F2 = a ne(U ) = |
λiui : λi = 1 , |
ii
which is equivalent to the definition in terms of V and W , as desired. For example, the a ne hull of two disjoint points is a line; and the a ne hull of a line and a point not on the line is a plane. Clearly, the a ne hull vastly overapproximates the union of two a ne spaces; however, it is the most precise a ne space that includes their union.
Now define
spDK (F, xk := e) [[xk := 0]]F DK [[xk := 1]]F
when e is not an a ne expression. In the new a ne space, xk can have any value. Exercise 12.5 asks the reader to prove this claim.
Example 12.15. Consider a ne space given by vertex representation
V = |
0 |
, |
|
1 |
|
1 |
||
|
|
|
|
|
|
for variables i j k T, and non-a ne assignment i := f (i, j, k). Compute
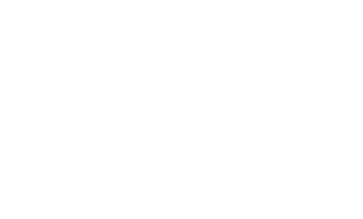
|
|
|
|
|
|
|
|
|
|
|
|
|
|
|
12.3 Karr’s Analysis 337 |
||||
[[i := 0]]V |
|
DK |
|
[[i := 1]]V |
|
|
0 |
|
|
|
0 |
|
0 |
||||||
= |
0 1 0 |
0 |
+ |
|
0 1 0 |
+ |
|||||||||||||
|
|
|
0 0 |
0 |
|
|
1 |
|
|
0 |
|
|
|
0 0 0 |
1 |
|
1 |
|
|
|
|
0 0 1 |
|
|
1 |
|
|
0 |
0 0 1 |
1 |
|
0 |
|||||||
|
|
|
|
|
|
|
|
|
|
|
|
|
|
|
|
|
|
||
|
|
|
0 |
|
|
|
|
1 |
|
|
|
|
|
|
|
|
|||
= |
|
0 |
|
0 |
|
|
|
|
|
|
|
|
|||||||
|
|
|
1 |
|
|
|
|
1 |
|
|
|
|
|
|
|
|
|
||
|
|
|
|
|
|
|
|
|
|
|
|
|
|
|
|
|
|
||
|
|
|
0 |
|
|
1 |
|
|
|
|
|
|
|
|
|
|
|
|
|
= |
|
0 |
, |
0 |
|
|
|
|
|
|
|
|
|
|
|
|
|||
|
|
|
1 |
|
|
|
1 |
|
|
|
|
|
|
|
|
|
|
|
|
The final set |
of vertices represents the set of states in which j = 0, k = 1, and |
||||||||||||||||||
|
|
|
|
|
|
|
|
|
|
|
|
|
|
|
|
|
|
|
|
i is any value. |
|
|
|
|
|
|
|
|
|
|
|
|
|
|
|
|
|
|
Step 4: Define a ne disjunction, DK .
The a ne hull DK , defined in Step 3, over-approximates disjunction.
Step 5: Define a ne implication checking.
For F1, F2 DK, whether F1 F2 is decidable. But a more e cient test relies on the vertex representations V and W of F1 and F2, respectively. For each v V , check if v a ne(W ): determine if there is a λ such that
X |
X |
v = λj wj and |
λj = 1 . |
j |
j |
In other words, determine if there is some a ne combination of the elements of W that equals v. More concisely, determine if there is some λ such that, for AW a matrix with columns w W ,
|
1T |
λ = |
|
1 |
|
; that is, |
1 |
|
λ = 1 and AW λ = v . |
|
AW |
|
|
v |
|
|
|
T |
|
This query can be decided e ciently using algorithms for solving linear equations, such as Gaussian elimination.
Then F1 F2 i for all v V , v a ne(W ).
Example 12.16. Consider a ne space F1 given by vertex representation
1
V1 = 1
2
and a ne space F2 given by vertex representation
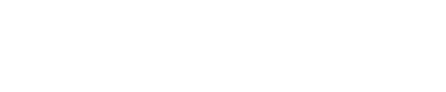
338 |
|
12 Invariant Generation |
|
|
|
|
|
|
|||||||||
V2 = |
0 |
, |
0 , |
1 . |
|
|
|
|
|||||||||
|
|
|
0 |
|
|
|
1 |
|
0 |
|
|
|
|
|
|
|
|
|
|
|
0 |
|
|
1 |
|
1 |
|
|
|
|
|
|
|||
|
|
|
|
|
|
|
|
|
|
|
|
|
|||||
|
|
|
|
|
|
|
1 |
F2 |
|
|
|
|
|
|
|
||
Is the implication F |
+ (1) |
valid? Yes, as |
|
||||||||||||||
|
1 |
|
= ( |
1) |
0 |
|
0 |
|
+ (1) |
|
1 |
|
|
||||
|
1 |
|
|
|
|
0 |
|
|
|
|
1 |
|
|
|
0 |
|
|
2 |
− 0 |
|
1 |
|
1 |
|
|||||||||||
and −1 + 1 + 1 = 1, so the vertex is in a ne(V2). |
|
Step 6: Define a ne widening.
With each growth of a µ(L), its dimension increases by at least 1 by definition of the a ne hull. As each µ(L) can be at most n-dimensional, for n the number of program variables, the procedure AbstractForwardPropagate terminates even without the use of widening. Hence, we do not define a widening operator.
Example 12.17. Consider the loop
@L0 : ; i := 0;
j := 0; k := 0; while
@L1 : ? ( ) {
k := k + 1;
if ( ) i := i + 1; else j := j + 1;
}
which has three basic paths:
(1)
@L0 : ; i := 0;
j := 0; k := 0; @L1 : ?;
which is summarized by transformation
τ1 : |
0 0 0 |
j |
+ |
0 |
, |
|
0 0 0 |
i |
|
0 |
|
|
0 0 0 k |
|
0 |
|
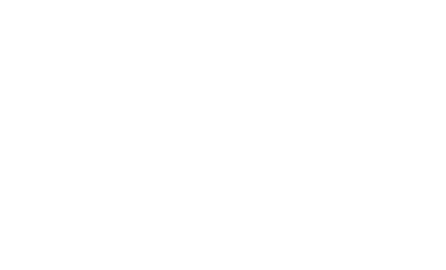
12.3 Karr’s Analysis |
339 |
(2)
@L1 : ?;
k := k + 1; i := i + 1; @L1 : ?;
which is summarized by transformation
τ2 |
: I |
j |
+ |
0 |
||
|
|
|
i |
|
|
1 |
|
|
|
|
|
||
|
|
|
k |
|
|
1 |
where, recall, I is the identity matrix, and
(3)
@L1 : ?;
k := k + 1; j := j + 1; @L1 : ?;
which is summarized by transformation
i0
τ3 : I j + 1 .
k1
Initially, µ(L0) , so its vertex representation is the set of unit vectors
0 |
, |
1 |
, |
0 |
, |
0 |
, |
|
|
|
|
|||
1 |
|
|
0 |
|
0 |
|
0 |
|
|
|
|
|
|
|
0 |
|
0 |
|
1 |
|
0 |
|
|
|
|
|
|
||
|
|
|
|
|
|
|
|
|
|
|
|
|
||
while µ(L |
1) , represented |
by |
|
. Then |
|
|
||||||||
|
|
|
|
|
0 . |
|||||||||
µ(L1) := µ(L1) |
DK |
|
τ1µ(L0) = |
|
||||||||||
|
|
|
|
|
|
|
|
|
|
|
|
|
0 |
|
|
|
|
|
|
|
|
|
|
|
0 |
||||
|
|
|
|
|
|
|
|
|
|
|
|
|
|
|
For the next iteration, consider the two transitions τ2 and τ3:
µ(L1) := µ(L1) |
DK τ2µ(L1) = 0 |
τ2 |
0 |
|||||
|
|
0 |
|
|
0 |
|
||
0 |
0 |
|||||||
|
|
|
|
|
|
|
|
|
|
|
0 |
|
|
|
|
||
|
= |
0 |
, |
0 |
. |
|
||
|
|
0 |
|
|
1 |
|
|
|
|
|
|
1 |
|
||||
|
|
|
|
|
|
|
||
|
|
|
|
|
|
|
|
Next,
340 |
|
12 Invariant Generation |
|
|
|
|
|
|
|
|
0 τ3 |
0 |
, τ3 |
0 |
||||||||||||||||||||||||
|
µ(L1) := µ(L1) |
DK τ3µ(L1) = 0 |
, |
|||||||||||||||||||||||||||||||||||
|
|
|
|
|
|
|
|
|
|
|
|
|
|
|
|
|
|
|
|
0 |
|
1 |
|
|
|
|
|
0 |
|
|
1 |
|
||||||
|
|
|
|
|
|
|
|
|
|
|
|
|
|
|
|
|
|
0 |
|
1 |
|
|
|
0 |
|
|
1 |
|||||||||||
|
|
|
|
|
|
|
|
|
|
|
|
|
|
|
|
|
|
|
|
|
|
|
|
|
|
|
|
|
|
|
|
|||||||
|
|
|
|
|
|
|
|
|
|
|
|
|
|
|
|
|
|
|
|
|
|
|
|
|
|
|
|
|
|
|
|
|
|
|
||||
|
|
|
|
|
|
|
|
|
|
|
|
|
|
|
|
|
= 0 |
, |
0 , |
1 . |
|
|
|
|
||||||||||||||
|
|
|
|
|
|
|
|
|
|
|
|
|
|
|
|
|
|
|
|
0 |
|
1 |
|
|
|
|
|
0 |
|
|
|
|
|
|
||||
|
|
|
|
|
|
|
|
|
|
|
|
|
|
|
|
|
|
|
0 |
|
1 |
|
|
|
1 |
|
|
|
|
|
||||||||
|
|
|
|
|
|
|
|
|
|
|
|
|
|
|
|
|
|
|
|
|
|
|
|
|
|
|
|
|
|
|
|
|
||||||
to |
|
|
|
|
|
as |
|
|
|
T |
|
|
|
|
|
|
|
|
|
|
|
|
|
|
|
|
T |
|
|
|
|
|
|
|
||||
|
|
|
|
|
|
|
|
|
|
|
|
|
|
|
|
|
|
|
|
|
|
|
. Note that τ3 is applied |
|||||||||||||||
The new vertex |
|
0 1 1 |
|
|
is obtained from τ3 |
0 0 0 |
|
|||||||||||||||||||||||||||||||
|
|
|
|
|
T |
|
|
|
|
|
|
|
|
|
|
|
|
|
|
|
|
|
|
|
|
|
|
|
|
|
|
|
|
|
|
|
|
|
|
1 0 1 |
|
well; however |
|
|
+ (1) |
|
+ (1) |
|
|
, |
|
|
|
|
|
|
|
||||||||||||||||||||
|
τ3 |
|
0 |
|
= |
1 |
|
= ( |
|
1) |
|
0 |
0 |
1 |
|
|
|
|
|
|
|
|||||||||||||||||
|
|
|
1 |
|
|
|
1 |
|
|
|
|
|
|
|
0 |
|
|
|
|
|
1 |
|
|
|
|
|
|
0 |
|
|
|
|
|
|
|
|
|
|
|
|
1 |
2 |
|
− 0 |
|
|
|
1 |
|
|
|
1 |
|
|
|
|
|
|
|
|
|||||||||||||||||
and −1 + 1 + 1 = 1. Hence, |
1 1 2 |
|
T is redundant. |
|
|
|
|
|
|
|
|
|
|
|||||||||||||||||||||||||
|
On the next iteration, |
we obtain convergence. For |
|
|
|
|
|
|
|
|
||||||||||||||||||||||||||||
|
|
|
|
|
|
= ( |
1) |
|
|
|
|
|
|
|
|
|
|
|
|
|
||||||||||||||||||
|
τ2 |
|
0 |
|
= |
0 |
|
, |
τ2 |
0 |
= |
0 |
0 |
+ (2) |
0 |
, |
|
|
|
|||||||||||||||||||
|
|
|
0 |
|
|
|
1 |
|
|
|
|
|
|
1 |
|
|
|
2 |
|
|
|
|
|
0 |
|
|
|
|
|
|
1 |
|
|
|
|
|
||
and |
0 |
1 |
|
|
|
1 |
2 |
|
− 0 |
|
|
|
1 |
|
|
|
|
|||||||||||||||||||||
|
|
|
= |
|
|
|
|
|
|
|
|
|
+ (1) |
|
+ (1) |
|
|
, |
|
|
|
|
|
|
|
|||||||||||||
|
τ2 |
1 |
1 |
= ( |
|
1) |
0 |
0 |
1 |
|
|
|
|
|
|
|
||||||||||||||||||||||
|
|
|
0 |
|
|
|
1 |
|
|
|
|
|
|
|
0 |
|
|
|
|
|
1 |
|
|
|
|
|
|
0 |
|
|
|
|
|
|
|
|
|
|
|
|
1 |
2 |
|
− 0 |
|
|
|
1 |
|
|
|
1 |
|
|
|
|
|
|
|
|
|||||||||||||||||
so that τ2 does not modify µ(L1). Additionally, |
|
|
|
|
|
|
|
|
|
|
|
|
, |
|||||||||||||||||||||||||
|
τ3 |
|
0 |
|
= |
1 |
|
, |
τ3 |
|
0 |
= |
1 |
= ( |
1) |
0 |
+ (1) |
0 |
+ (1) |
1 |
||||||||||||||||||
|
|
|
0 |
|
|
|
0 |
|
|
|
|
|
|
1 |
|
|
|
1 |
|
|
|
|
|
0 |
|
|
|
|
|
|
1 |
|
|
|
0 |
|
||
and |
0 |
1 |
|
|
|
1 |
2 |
|
− 0 |
|
|
|
1 |
|
1 |
|||||||||||||||||||||||
|
|
|
= |
|
|
|
|
|
|
|
|
|
+ (2) |
|
|
|
|
|
|
|
|
|
|
|
|
|
|
|
|
|
|
|||||||
|
τ3 |
1 |
2 |
= ( |
|
1) |
0 |
1 |
, |
|
|
|
|
|
|
|
|
|
|
|
|
|
|
|
||||||||||||||
|
|
|
0 |
|
|
|
0 |
|
|
|
|
|
|
|
0 |
|
|
|
|
|
0 |
|
|
|
|
|
|
|
|
|
|
|
|
|
|
|
|
|
|
|
1 |
2 |
|
− 0 |
|
|
|
1 |
|
|
|
|
|
|
|
|
|
|
|
|
|
|
|
|
|||||||||||||
so that τ3 does not modify µ(L1), either. |
|
|
|
|
|
|
|
|
|
|
|
|
|
|
|
|
|
|||||||||||||||||||||
|
Hence, the final vertex representation of µ(L1) is |
|
|
|
|
|
|
|
|
|
||||||||||||||||||||||||||||
|
V = 0 |
, |
|
0 |
, |
1 . |
|
|
|
|
|
|
|
|
|
|
|
|
|
|
|
|
|
|
|
|
|
|||||||||||
|
|
|
|
0 |
|
|
1 |
|
|
0 |
|
|
|
|
|
|
|
|
|
|
|
|
|
|
|
|
|
|
|
|
|
|
|
|||||
|
|
|
0 |
|
|
1 |
|
|
1 |
|
|
|
|
|
|
|
|
|
|
|
|
|
|
|
|
|
|
|
|
|
|
|||||||
|
|
|
|
|
|
|
|
|
|
|
|
|
|
|
|
|
|
|
|
|
|
|
|
|
|
|
|
|
|
|
|
|
|
|
||||
|
|
|
|
|
|
|
|
|
|
|
|
|
|
|
|
|
|
|
|
|
|
|
|
|
|
|
|
|
|
|
|
|
|
|
|
To obtain the constraint representation of this a ne space, solve