
Теория информации / Cover T.M., Thomas J.A. Elements of Information Theory. 2006., 748p
.pdf17.8 ENTROPY POWER INEQUALITY AND BRUNN–MINKOWSKI INEQUALITY |
675 |
alternative proof of the entropy power inequality. We also show how the entropy power inequality and the Brunn – Minkowski inequality are related by means of a common proof.
We can rewrite the entropy power inequality for dimension n = 1 in a form that emphasizes its relationship to the normal distribution. Let X and Y be two independent random variables with densities, and let X and Y be independent normals with the same entropy as X and Y , respectively. Then 22h(X) = 22h(X ) = (2π e)σX2 and similarly, 22h(Y ) =
(2π e)σ 2 |
. Hence the entropy power inequality can be rewritten as |
|
Y |
|
|
|
22h(X+Y ) ≥ (2π e)(σX2 + σY2 ) = 22h(X +Y ), |
(17.89) |
since X and Y are independent. Thus, we have a new statement of the entropy power inequality.
Theorem 17.8.1 (Restatement of the entropy power inequality ) For two independent random variables X and Y ,
h(X + Y ) ≥ h(X + Y ), |
(17.90) |
where X and Y are independent normal random variables with h(X ) = h(X) and h(Y ) = h(Y ).
This form of the entropy power inequality bears a striking resemblance to the Brunn – Minkowski inequality, which bounds the volume of set sums.
Definition The set sum A + B of two sets A, B Rn is defined as the set {x + y : x A, y B}.
Example 17.8.1 The set sum of two spheres of radius 1 is a sphere of radius 2.
Theorem 17.8.2 (Brunn – Minkowski inequality) The volume of the set sum of two sets A and B is greater than the volume of the set sum of two spheres A and B with the same volume as A and B, respectively:
V (A + B) ≥ V (A + B ), |
(17.91) |
where A and B are spheres with V (A ) = V (A) and V (B ) = V (B).
The similarity between the two theorems was pointed out in [104]. A common proof was found by Dembo [162] and Lieb, starting from a
676 INEQUALITIES IN INFORMATION THEORY
strengthened version of Young’s inequality. The same proof can be used to prove a range of inequalities which includes the entropy power inequality and the Brunn – Minkowski inequality as special cases. We begin with a few definitions.
Definition Let f and g be two densities over Rn and let f g denote the convolution of the two densities. Let the Lr norm of the density be defined by
|
|
|
|
|
|
|
|
|
|
|
|
|
|
|
|
|
1 |
|
|
|||||
|
|
f |
|| |
r |
|
|
f r (x) dx r . |
|
(17.92) |
|||||||||||||||
|
|| |
|
|
|
= |
|
|
|
|
|
|
|
|
|
|
|
|
|
|
|
||||
Lemma 17.8.1 (Strengthened Young’s inequality ) |
For any two densi- |
|||||||||||||||||||||||
ties f and g over Rn, |
|
|
|
|
|
|
|
|
|
|
|
|
|
|
|
n |
|
|
|
|
|
|
|
|
f |
|
g |
|
r |
|
≤ |
|
|
CpCq |
|
2 |
|
f |
|
p |
g |
q , |
(17.93) |
||||||
|
|
|
|
|
|
|||||||||||||||||||
|| |
|
|| |
|
|
|
|
|
Cr |
|
|| |
|| |
|| |
|| |
|
||||||||||
where |
|
|
|
|
|
|
1 |
|
1 |
1 |
|
|
|
|
|
|
|
|
||||||
|
|
|
|
|
|
|
|
− 1 |
|
|
|
|
(17.94) |
|||||||||||
|
|
|
|
|
|
|
|
|
= |
|
+ |
|
|
|
|
|
|
|||||||
|
|
|
|
|
|
|
r |
p |
q |
|
|
|
|
|||||||||||
and |
|
|
|
|
|
|
|
|
1 |
|
|
|
|
|
|
|
|
|
|
|
|
|
|
|
|
|
|
|
|
|
|
|
|
|
|
|
|
1 |
|
1 |
|
|
|
|
|
||||
|
|
|
|
|
|
|
p p |
|
|
= 1. |
(17.95) |
|||||||||||||
|
Cp = |
|
|
|
|
|
|
, |
|
|
|
+ |
|
|
||||||||||
|
|
p |
1 |
|
|
|
p |
p |
|
|||||||||||||||
|
|
|
|
|
|
p |
|
|
|
|
|
|
|
|
|
|
|
Proof: The proof of this inequality may be found in [38] and [73].
We define a generalization of the entropy.
Definition The Renyi entropy hr (X) of order r is defined as
hr (X) = |
|
1 |
|
log |
f r (x) dx |
(17.96) |
1 |
− |
r |
||||
|
|
|
|
|
|
for 0 < r < ∞, r =1. If we take the limit as r → 1, we obtain the Shannon entropy function,
h(X) = h1(X) = − f (x) log f (x) dx. (17.97)
If we take the limit as r → 0, we obtain the logarithm of the volume of the support set,
h0(X) = log (µ{x : f (x) > 0}) . |
(17.98) |
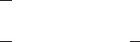
17.8 ENTROPY POWER INEQUALITY AND BRUNN–MINKOWSKI INEQUALITY |
677 |
Thus, the zeroth-order Renyi entropy gives the logarithm of the measure of the support set of the density f , and the Shannon entropy h1 gives the logarithm of the size of the “effective” support set (Theorem 8.2.2). We now define the equivalent of the entropy power for Renyi entropies.
Definition The Renyi entropy power Vr (X) of order r is defined as
|
|
|
|
|
|
|
|
|
2 r |
|
|
|
|
|
|
|
|
1 |
|
1 |
|
|
|
||
|
|
|
|
|
|
|
− n r , |
|
|
|
|
|
|
|
|
|
|
|
|
||||||
|
|
|
f r (x) dx |
0 < r |
≤ ∞ |
, r |
|
1 , r |
+ |
r |
|
|
= |
1 |
|||||||||||
V |
(X) |
|
|
|
|
2 |
|
|
|
r |
|
1 |
|
= |
|
|
|
|
|||||||
r |
|
|
exp [ |
n |
h(X)], |
|
|
|
|
|
|
|
|
|
|
|
|
|
|||||||
|
|
|
|
|
|
|
2 |
|
= |
|
|
|
|
|
|
|
|
|
|
|
|
|
|||
|
|
= |
|
|
|
|
|
|
|
|
|
|
|
|
|
|
|
|
|
|
|||||
|
|
|
|
µ({x : f (x) > 0}) n , |
r = 0 |
|
|
|
|
|
|
|
|
|
|
|
|
||||||||
|
|
|
|
|
|
|
|
|
|
|
|
|
|
|
|
|
|
|
|
|
|
|
|
|
|
|
|
|
|
|
|
|
|
|
|
|
|
|
|
|
|
|
|
|
|
|
|
|
(17.99) |
||
Theorem 17.8.3 |
|
For two independent random variables X and Y and |
|||||||||||||||||||||||
any 0 ≤ r < ∞ and any 0 ≤ λ ≤ 1, we have |
|
|
|
|
|
|
|
|
|
|
|
||||||||||||||
|
log Vr (X + Y ) ≥ λ log Vp(X) + (1 − λ) log Vq (Y ) + H (λ) |
|
|
||||||||||||||||||||||
|
|
|
|
|
|
|
|
|
|
1 r |
r |
λ(1 |
|
r) |
|
|
r |
, |
|
|
|
|
|
||
|
|
|
|
|
|
|
|
|
|
+ 1−+r H |
|
+1+r− |
− H |
|
|
|
(17.100) |
||||||||
|
|
|
|
|
|
|
|
|
1+r |
|
|||||||||||||||
where p = |
|
|
r |
|
|
, q = |
|
r |
|
and H (λ) = −λ log λ − (1 − λ) |
|||||||||||||||
|
(r+λ(1−r)) |
|
(r+(1−λ)(1−r)) |
log(1 − λ).
Proof: If we take the logarithm of Young’s inequality (17.93), we obtain
1 |
|
|
|
1 |
|
|
1 |
|
|
|
|
|
|
|
|
|
|
|
|
|
|
|
|||||||
|
|
log Vr (X |
+ Y ) ≥ |
|
log Vp(X) + |
|
log Vq (Y ) + log Cr |
|
|
|
|||||||||||||||||||
|
r |
p |
q |
|
|
|
|||||||||||||||||||||||
|
|
|
|
|
|
|
|
|
|
|
|
− log Cp − log Cq . |
|
|
(17.101) |
||||||||||||||
Setting λ |
|
r /p |
and using (17.94), we have 1 |
|
|
λ |
|
r /q |
, p |
|
|
r |
|
||||||||||||||||
= |
− |
= |
= r+λ(1−r) |
||||||||||||||||||||||||||
and q = |
r |
|
. Thus, (17.101) becomes |
|
|
|
|
|
|
||||||||||||||||||||
r+(1−λ)(1−r) |
|
|
|
|
r |
|
|
|
|
|
|||||||||||||||||||
|
|
|
|
|
|
|
|
|
|
|
|
|
|
|
|
|
|
|
|
|
|
|
|
|
|||||
log Vr (X + Y ) ≥ |
λ log Vp(X) + (1 − λ) log Vq (Y ) + |
|
log r − log r |
||||||||||||||||||||||||||
r |
|||||||||||||||||||||||||||||
|
|
|
|
|
|
|
r |
|
r |
|
|
|
r |
|
|
|
|
r |
|
|
|
|
|
||||||
|
|
|
|
|
− |
|
log p + |
|
log p − |
|
log q + |
|
log q |
|
|
|
|||||||||||||
|
|
|
|
|
p |
p |
q |
q |
(17.102) |
||||||||||||||||||||
|
|
|
|
|
|
|
|
|
|
|
|
|
|
|
|
|
|
|
|
|
|
|
|
|
|
= λ log Vp(X) + (1 − λ) log Vq (Y )
+r log r − (λ + 1 − λ) log r r
− r log p + λ log p − r log q + (1 − λ) log q p q
(17.103)
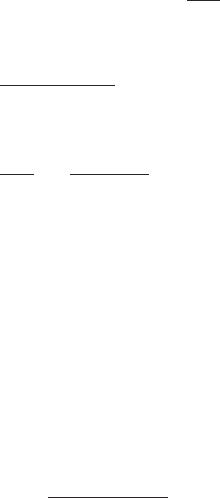
17.9 INEQUALITIES FOR DETERMINANTS |
679 |
The general theorem unifies the entropy power inequality and the Brunn – Minkowski inequality and introduces a continuum of new inequalities that lie between the entropy power inequality and the Brunn – Minkowski inequality. This further strengthens the analogy between entropy power and volume.
17.9INEQUALITIES FOR DETERMINANTS
Throughout the remainder of this chapter, we assume that K is a nonnegative definite symmetric n × n matrix. Let |K| denote the determinant of
K.
We first give an information-theoretic proof of a result due to Ky Fan [199].
Theorem 17.9.1 log |K| is concave.
Proof: Let X1 and X2 be normally distributed n-vectors, Xi N(0, Ki ), i = 1, 2. Let the random variable θ have the distribution
Pr{θ = 1} = λ, |
(17.111) |
Pr{θ = 2} = 1 − λ |
(17.112) |
for some 0 ≤ λ ≤ 1. Let θ , X1, and X2 be |
independent, and let Z = |
Xθ . Then Z has covariance KZ = λK1 + (1 − λ)K2. However, Z will not be multivariate normal. By first using Theorem 17.2.3, followed by Theorem 17.2.1, we have
1 |
log(2π e)n|λK1 |
+ (1 − λ)K2| ≥ h(Z) |
|
|
|
(17.113) |
|||
|
2 |
|
|
|
|||||
|
|
|
≥ h(Z|θ ) |
|
|
|
(17.114) |
||
|
|
|
1 |
log(2π e)n|K1 |
| |
||||
|
|
|
= λ |
|
|||||
|
|
|
2 |
||||||
|
|
|
+ (1 − |
1 |
log(2π e)n|K2|. |
||||
|
|
|
λ) |
|
|||||
|
|
|
2 |
||||||
Thus, |
|
|
|
|
|
|
|
||
|
|
|λK1 + (1 − λ)K2| ≥ |K1|λ|K2|1−λ, |
(17.115) |
||||||
as desired. |
|
|
|
|
|
|
|
We now give Hadamard’s inequality using an information-theoretic proof [128].

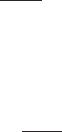

17.10 INEQUALITIES FOR RATIOS OF DETERMINANTS |
683 |
Translating this to determinants, one obtains
lim |
|
n| |
1 |
lim |
|Kn| |
|
(17.135) |
K |
n |
. |
|||||
n→∞ |
| |
|
= n→∞ |Kn−1| |
|
|
||
Theorem 17.9.7 (Minkowski inequality [390] ) |
|
|
|||||
|K1 + K2|1/n ≥ |K1|1/n + |K2|1/n. |
(17.136) |
Proof: Let X1, X2 be independent with Xi N(0, Ki ). Noting that X1 + X2 N(0, K1 + K2) and using the entropy power inequality (Theorem 17.7.3) yields
(2π e) K1 |
+ |
K2 |
1/n |
= |
2 n2 h(X1 + X2) |
(17.137) |
| |
|
| |
2 n2 h(X1) + 2 n2 h(X2) |
|
||
|
|
|
|
≥ |
(17.138) |
=(2π e)|K1|1/n + (2π e)|K2|1/n. (17.139)
17.10INEQUALITIES FOR RATIOS OF DETERMINANTS
We now prove similar inequalities for ratios of determinants. Before developing the next theorem, we make an observation about minimum mean- squared-error linear prediction. If (X1, X2, . . . , Xn) N(0, Kn), we know that the conditional density of Xn given (X1, X2, . . . , Xn−1) is univariate
normal with mean linear in X |
, X |
, . . . , X |
n−1 |
and conditional variance |
||||||||||
2 |
2 |
1 |
2 |
|
|
|
ˆ |
2 |
over all |
|||||
σn |
. Here σn is the minimum mean squared error E(Xn − Xn) |
|
||||||||||||
|
|
|
ˆ |
based on X1, X2, . . . , Xn−1. |
|
|
||||||||
linear estimators Xn |
|
|
||||||||||||
Lemma 17.10.1 |
σn2 = |Kn|/|Kn−1|. |
|
|
|
|
|
|
|||||||
Proof: Using the conditional normality of Xn, we have |
|
|
||||||||||||
|
1 |
log 2π eσn2 = h(Xn|X1, X2, . . . , Xn−1) |
|
|
(17.140) |
|||||||||
|
|
|
|
|
||||||||||
|
|
2 |
|
|
||||||||||
|
|
|
= h(X1, X2, . . . , Xn) − h(X1, X2, . . . , Xn−1) (17.141) |
|||||||||||
|
= |
1 |
log(2π e)n|Kn| − |
1 |
log(2π e)n−1|Kn−1| |
|
(17.142) |
|||||||
|
|
2 |
2 |
|
||||||||||
|
= |
1 |
log 2π e|Kn|/|Kn−1|. |
|
(17.143) |
|||||||||
|
|
|
|
|||||||||||
|
|
2 |
|

684 INEQUALITIES IN INFORMATION THEORY
Minimization of σn2 over a set of allowed covariance matrices {Kn} is aided by the following theorem. Such problems arise in maximum entropy spectral density estimation.
Theorem 17.10.1 |
(Bergstrøm [42] ) log(|Kn|/|Kn−p|) is |
concave |
in Kn. |
|
|
Proof: We remark |
that Theorem 17.9.1 cannot be used |
because |
log(|Kn|/|Kn−p|) is the difference of two concave functions. Let Z = Xθ , where X1 N(0, Sn), X2 N(0, Tn), Pr{θ = 1} = λ = 1 − Pr{θ = 2}, and let X1, X2, θ be independent. The covariance matrix Kn of Z is given by
Kn = λSn + (1 − λ)Tn. |
(17.144) |
The following chain of inequalities proves the theorem:
|
1 |
log(2π e)p|Sn|/|Sn−p |
|
1 |
log(2π e)p|Tn|/|Tn−p| |
|||||||
λ |
|
| + (1 − λ) |
|
|||||||||
2 |
2 |
|||||||||||
|
|
(a) |
|
|
|
|
|
|
|
|
|
|
|
|
= λh(X1,n, X1,n−1, . . . , X1,n−p+1|X1,1, . . . , X1,n−p) |
|
|||||||||
|
|
|
+ (1 − λ)h(X2,n, X2,n−1, . . . , X2,n−p+1|X2,1, . . . , X2,n−p) |
|||||||||
|
|
|
|
|
|
|
|
|
|
|
|
(17.145) |
|
|
= h(Zn, Zn−1, . . . , Zn−p+1|Z1, . . . , Zn−p, θ ) |
(17.146) |
|||||||||
|
|
(b) |
|
|
|
|
|
|
|
|
|
(17.147) |
|
|
≤ h(Zn, Zn−1, . . . , Zn−p+1|Z1, . . . , Zn−p) |
||||||||||
|
|
(c) |
|
1 |
log(2π e)p |
|Kn| |
|
, |
|
|
(17.148) |
|
|
|
≤ |
2 |
|Kn−p| |
|
|
||||||
|
|
|
|
|
|
|
|
|||||
where |
|
(a) follows |
from |
h(Xn, Xn−1, . . . , Xn−p+1|X1, . . . , Xn−p) = |
||||||||
h(X1, . . . , Xn) − h(X1, . . . , Xn−p), (b) follows from the |
conditioning |
lemma, and (c) follows from a conditional version of Theorem 17.2.3.
Theorem 17.10.2 (Bergstrøm [42] ) |Kn|/|Kn−1| is concave in Kn.
Proof: Again we use the properties of Gaussian random variables. Let us assume that we have two independent Gaussian random n-vectors, X N(0, An) and Y N(0, Bn). Let Z = X + Y. Then
1 |
log 2π e |
|An |
+ Bn| |
(a) |
h(Z |
|
Z |
|
, Z |
|
, . . . , Z |
) (17.149) |
|
2 |
|An−1 + Bn−1| |
= |
n| |
n−1 |
n−2 |
||||||||
|
|
|
|
1 |
|