
- •Preface
- •Biological Vision Systems
- •Visual Representations from Paintings to Photographs
- •Computer Vision
- •The Limitations of Standard 2D Images
- •3D Imaging, Analysis and Applications
- •Book Objective and Content
- •Acknowledgements
- •Contents
- •Contributors
- •2.1 Introduction
- •Chapter Outline
- •2.2 An Overview of Passive 3D Imaging Systems
- •2.2.1 Multiple View Approaches
- •2.2.2 Single View Approaches
- •2.3 Camera Modeling
- •2.3.1 Homogeneous Coordinates
- •2.3.2 Perspective Projection Camera Model
- •2.3.2.1 Camera Modeling: The Coordinate Transformation
- •2.3.2.2 Camera Modeling: Perspective Projection
- •2.3.2.3 Camera Modeling: Image Sampling
- •2.3.2.4 Camera Modeling: Concatenating the Projective Mappings
- •2.3.3 Radial Distortion
- •2.4 Camera Calibration
- •2.4.1 Estimation of a Scene-to-Image Planar Homography
- •2.4.2 Basic Calibration
- •2.4.3 Refined Calibration
- •2.4.4 Calibration of a Stereo Rig
- •2.5 Two-View Geometry
- •2.5.1 Epipolar Geometry
- •2.5.2 Essential and Fundamental Matrices
- •2.5.3 The Fundamental Matrix for Pure Translation
- •2.5.4 Computation of the Fundamental Matrix
- •2.5.5 Two Views Separated by a Pure Rotation
- •2.5.6 Two Views of a Planar Scene
- •2.6 Rectification
- •2.6.1 Rectification with Calibration Information
- •2.6.2 Rectification Without Calibration Information
- •2.7 Finding Correspondences
- •2.7.1 Correlation-Based Methods
- •2.7.2 Feature-Based Methods
- •2.8 3D Reconstruction
- •2.8.1 Stereo
- •2.8.1.1 Dense Stereo Matching
- •2.8.1.2 Triangulation
- •2.8.2 Structure from Motion
- •2.9 Passive Multiple-View 3D Imaging Systems
- •2.9.1 Stereo Cameras
- •2.9.2 3D Modeling
- •2.9.3 Mobile Robot Localization and Mapping
- •2.10 Passive Versus Active 3D Imaging Systems
- •2.11 Concluding Remarks
- •2.12 Further Reading
- •2.13 Questions
- •2.14 Exercises
- •References
- •3.1 Introduction
- •3.1.1 Historical Context
- •3.1.2 Basic Measurement Principles
- •3.1.3 Active Triangulation-Based Methods
- •3.1.4 Chapter Outline
- •3.2 Spot Scanners
- •3.2.1 Spot Position Detection
- •3.3 Stripe Scanners
- •3.3.1 Camera Model
- •3.3.2 Sheet-of-Light Projector Model
- •3.3.3 Triangulation for Stripe Scanners
- •3.4 Area-Based Structured Light Systems
- •3.4.1 Gray Code Methods
- •3.4.1.1 Decoding of Binary Fringe-Based Codes
- •3.4.1.2 Advantage of the Gray Code
- •3.4.2 Phase Shift Methods
- •3.4.2.1 Removing the Phase Ambiguity
- •3.4.3 Triangulation for a Structured Light System
- •3.5 System Calibration
- •3.6 Measurement Uncertainty
- •3.6.1 Uncertainty Related to the Phase Shift Algorithm
- •3.6.2 Uncertainty Related to Intrinsic Parameters
- •3.6.3 Uncertainty Related to Extrinsic Parameters
- •3.6.4 Uncertainty as a Design Tool
- •3.7 Experimental Characterization of 3D Imaging Systems
- •3.7.1 Low-Level Characterization
- •3.7.2 System-Level Characterization
- •3.7.3 Characterization of Errors Caused by Surface Properties
- •3.7.4 Application-Based Characterization
- •3.8 Selected Advanced Topics
- •3.8.1 Thin Lens Equation
- •3.8.2 Depth of Field
- •3.8.3 Scheimpflug Condition
- •3.8.4 Speckle and Uncertainty
- •3.8.5 Laser Depth of Field
- •3.8.6 Lateral Resolution
- •3.9 Research Challenges
- •3.10 Concluding Remarks
- •3.11 Further Reading
- •3.12 Questions
- •3.13 Exercises
- •References
- •4.1 Introduction
- •Chapter Outline
- •4.2 Representation of 3D Data
- •4.2.1 Raw Data
- •4.2.1.1 Point Cloud
- •4.2.1.2 Structured Point Cloud
- •4.2.1.3 Depth Maps and Range Images
- •4.2.1.4 Needle map
- •4.2.1.5 Polygon Soup
- •4.2.2 Surface Representations
- •4.2.2.1 Triangular Mesh
- •4.2.2.2 Quadrilateral Mesh
- •4.2.2.3 Subdivision Surfaces
- •4.2.2.4 Morphable Model
- •4.2.2.5 Implicit Surface
- •4.2.2.6 Parametric Surface
- •4.2.2.7 Comparison of Surface Representations
- •4.2.3 Solid-Based Representations
- •4.2.3.1 Voxels
- •4.2.3.3 Binary Space Partitioning
- •4.2.3.4 Constructive Solid Geometry
- •4.2.3.5 Boundary Representations
- •4.2.4 Summary of Solid-Based Representations
- •4.3 Polygon Meshes
- •4.3.1 Mesh Storage
- •4.3.2 Mesh Data Structures
- •4.3.2.1 Halfedge Structure
- •4.4 Subdivision Surfaces
- •4.4.1 Doo-Sabin Scheme
- •4.4.2 Catmull-Clark Scheme
- •4.4.3 Loop Scheme
- •4.5 Local Differential Properties
- •4.5.1 Surface Normals
- •4.5.2 Differential Coordinates and the Mesh Laplacian
- •4.6 Compression and Levels of Detail
- •4.6.1 Mesh Simplification
- •4.6.1.1 Edge Collapse
- •4.6.1.2 Quadric Error Metric
- •4.6.2 QEM Simplification Summary
- •4.6.3 Surface Simplification Results
- •4.7 Visualization
- •4.8 Research Challenges
- •4.9 Concluding Remarks
- •4.10 Further Reading
- •4.11 Questions
- •4.12 Exercises
- •References
- •1.1 Introduction
- •Chapter Outline
- •1.2 A Historical Perspective on 3D Imaging
- •1.2.1 Image Formation and Image Capture
- •1.2.2 Binocular Perception of Depth
- •1.2.3 Stereoscopic Displays
- •1.3 The Development of Computer Vision
- •1.3.1 Further Reading in Computer Vision
- •1.4 Acquisition Techniques for 3D Imaging
- •1.4.1 Passive 3D Imaging
- •1.4.2 Active 3D Imaging
- •1.4.3 Passive Stereo Versus Active Stereo Imaging
- •1.5 Twelve Milestones in 3D Imaging and Shape Analysis
- •1.5.1 Active 3D Imaging: An Early Optical Triangulation System
- •1.5.2 Passive 3D Imaging: An Early Stereo System
- •1.5.3 Passive 3D Imaging: The Essential Matrix
- •1.5.4 Model Fitting: The RANSAC Approach to Feature Correspondence Analysis
- •1.5.5 Active 3D Imaging: Advances in Scanning Geometries
- •1.5.6 3D Registration: Rigid Transformation Estimation from 3D Correspondences
- •1.5.7 3D Registration: Iterative Closest Points
- •1.5.9 3D Local Shape Descriptors: Spin Images
- •1.5.10 Passive 3D Imaging: Flexible Camera Calibration
- •1.5.11 3D Shape Matching: Heat Kernel Signatures
- •1.6 Applications of 3D Imaging
- •1.7 Book Outline
- •1.7.1 Part I: 3D Imaging and Shape Representation
- •1.7.2 Part II: 3D Shape Analysis and Processing
- •1.7.3 Part III: 3D Imaging Applications
- •References
- •5.1 Introduction
- •5.1.1 Applications
- •5.1.2 Chapter Outline
- •5.2 Mathematical Background
- •5.2.1 Differential Geometry
- •5.2.2 Curvature of Two-Dimensional Surfaces
- •5.2.3 Discrete Differential Geometry
- •5.2.4 Diffusion Geometry
- •5.2.5 Discrete Diffusion Geometry
- •5.3 Feature Detectors
- •5.3.1 A Taxonomy
- •5.3.2 Harris 3D
- •5.3.3 Mesh DOG
- •5.3.4 Salient Features
- •5.3.5 Heat Kernel Features
- •5.3.6 Topological Features
- •5.3.7 Maximally Stable Components
- •5.3.8 Benchmarks
- •5.4 Feature Descriptors
- •5.4.1 A Taxonomy
- •5.4.2 Curvature-Based Descriptors (HK and SC)
- •5.4.3 Spin Images
- •5.4.4 Shape Context
- •5.4.5 Integral Volume Descriptor
- •5.4.6 Mesh Histogram of Gradients (HOG)
- •5.4.7 Heat Kernel Signature (HKS)
- •5.4.8 Scale-Invariant Heat Kernel Signature (SI-HKS)
- •5.4.9 Color Heat Kernel Signature (CHKS)
- •5.4.10 Volumetric Heat Kernel Signature (VHKS)
- •5.5 Research Challenges
- •5.6 Conclusions
- •5.7 Further Reading
- •5.8 Questions
- •5.9 Exercises
- •References
- •6.1 Introduction
- •Chapter Outline
- •6.2 Registration of Two Views
- •6.2.1 Problem Statement
- •6.2.2 The Iterative Closest Points (ICP) Algorithm
- •6.2.3 ICP Extensions
- •6.2.3.1 Techniques for Pre-alignment
- •Global Approaches
- •Local Approaches
- •6.2.3.2 Techniques for Improving Speed
- •Subsampling
- •Closest Point Computation
- •Distance Formulation
- •6.2.3.3 Techniques for Improving Accuracy
- •Outlier Rejection
- •Additional Information
- •Probabilistic Methods
- •6.3 Advanced Techniques
- •6.3.1 Registration of More than Two Views
- •Reducing Error Accumulation
- •Automating Registration
- •6.3.2 Registration in Cluttered Scenes
- •Point Signatures
- •Matching Methods
- •6.3.3 Deformable Registration
- •Methods Based on General Optimization Techniques
- •Probabilistic Methods
- •6.3.4 Machine Learning Techniques
- •Improving the Matching
- •Object Detection
- •6.4 Quantitative Performance Evaluation
- •6.5 Case Study 1: Pairwise Alignment with Outlier Rejection
- •6.6 Case Study 2: ICP with Levenberg-Marquardt
- •6.6.1 The LM-ICP Method
- •6.6.2 Computing the Derivatives
- •6.6.3 The Case of Quaternions
- •6.6.4 Summary of the LM-ICP Algorithm
- •6.6.5 Results and Discussion
- •6.7 Case Study 3: Deformable ICP with Levenberg-Marquardt
- •6.7.1 Surface Representation
- •6.7.2 Cost Function
- •Data Term: Global Surface Attraction
- •Data Term: Boundary Attraction
- •Penalty Term: Spatial Smoothness
- •Penalty Term: Temporal Smoothness
- •6.7.3 Minimization Procedure
- •6.7.4 Summary of the Algorithm
- •6.7.5 Experiments
- •6.8 Research Challenges
- •6.9 Concluding Remarks
- •6.10 Further Reading
- •6.11 Questions
- •6.12 Exercises
- •References
- •7.1 Introduction
- •7.1.1 Retrieval and Recognition Evaluation
- •7.1.2 Chapter Outline
- •7.2 Literature Review
- •7.3 3D Shape Retrieval Techniques
- •7.3.1 Depth-Buffer Descriptor
- •7.3.1.1 Computing the 2D Projections
- •7.3.1.2 Obtaining the Feature Vector
- •7.3.1.3 Evaluation
- •7.3.1.4 Complexity Analysis
- •7.3.2 Spin Images for Object Recognition
- •7.3.2.1 Matching
- •7.3.2.2 Evaluation
- •7.3.2.3 Complexity Analysis
- •7.3.3 Salient Spectral Geometric Features
- •7.3.3.1 Feature Points Detection
- •7.3.3.2 Local Descriptors
- •7.3.3.3 Shape Matching
- •7.3.3.4 Evaluation
- •7.3.3.5 Complexity Analysis
- •7.3.4 Heat Kernel Signatures
- •7.3.4.1 Evaluation
- •7.3.4.2 Complexity Analysis
- •7.4 Research Challenges
- •7.5 Concluding Remarks
- •7.6 Further Reading
- •7.7 Questions
- •7.8 Exercises
- •References
- •8.1 Introduction
- •Chapter Outline
- •8.2 3D Face Scan Representation and Visualization
- •8.3 3D Face Datasets
- •8.3.1 FRGC v2 3D Face Dataset
- •8.3.2 The Bosphorus Dataset
- •8.4 3D Face Recognition Evaluation
- •8.4.1 Face Verification
- •8.4.2 Face Identification
- •8.5 Processing Stages in 3D Face Recognition
- •8.5.1 Face Detection and Segmentation
- •8.5.2 Removal of Spikes
- •8.5.3 Filling of Holes and Missing Data
- •8.5.4 Removal of Noise
- •8.5.5 Fiducial Point Localization and Pose Correction
- •8.5.6 Spatial Resampling
- •8.5.7 Feature Extraction on Facial Surfaces
- •8.5.8 Classifiers for 3D Face Matching
- •8.6 ICP-Based 3D Face Recognition
- •8.6.1 ICP Outline
- •8.6.2 A Critical Discussion of ICP
- •8.6.3 A Typical ICP-Based 3D Face Recognition Implementation
- •8.6.4 ICP Variants and Other Surface Registration Approaches
- •8.7 PCA-Based 3D Face Recognition
- •8.7.1 PCA System Training
- •8.7.2 PCA Training Using Singular Value Decomposition
- •8.7.3 PCA Testing
- •8.7.4 PCA Performance
- •8.8 LDA-Based 3D Face Recognition
- •8.8.1 Two-Class LDA
- •8.8.2 LDA with More than Two Classes
- •8.8.3 LDA in High Dimensional 3D Face Spaces
- •8.8.4 LDA Performance
- •8.9 Normals and Curvature in 3D Face Recognition
- •8.9.1 Computing Curvature on a 3D Face Scan
- •8.10 Recent Techniques in 3D Face Recognition
- •8.10.1 3D Face Recognition Using Annotated Face Models (AFM)
- •8.10.2 Local Feature-Based 3D Face Recognition
- •8.10.2.1 Keypoint Detection and Local Feature Matching
- •8.10.2.2 Other Local Feature-Based Methods
- •8.10.3 Expression Modeling for Invariant 3D Face Recognition
- •8.10.3.1 Other Expression Modeling Approaches
- •8.11 Research Challenges
- •8.12 Concluding Remarks
- •8.13 Further Reading
- •8.14 Questions
- •8.15 Exercises
- •References
- •9.1 Introduction
- •Chapter Outline
- •9.2 DEM Generation from Stereoscopic Imagery
- •9.2.1 Stereoscopic DEM Generation: Literature Review
- •9.2.2 Accuracy Evaluation of DEMs
- •9.2.3 An Example of DEM Generation from SPOT-5 Imagery
- •9.3 DEM Generation from InSAR
- •9.3.1 Techniques for DEM Generation from InSAR
- •9.3.1.1 Basic Principle of InSAR in Elevation Measurement
- •9.3.1.2 Processing Stages of DEM Generation from InSAR
- •The Branch-Cut Method of Phase Unwrapping
- •The Least Squares (LS) Method of Phase Unwrapping
- •9.3.2 Accuracy Analysis of DEMs Generated from InSAR
- •9.3.3 Examples of DEM Generation from InSAR
- •9.4 DEM Generation from LIDAR
- •9.4.1 LIDAR Data Acquisition
- •9.4.2 Accuracy, Error Types and Countermeasures
- •9.4.3 LIDAR Interpolation
- •9.4.4 LIDAR Filtering
- •9.4.5 DTM from Statistical Properties of the Point Cloud
- •9.5 Research Challenges
- •9.6 Concluding Remarks
- •9.7 Further Reading
- •9.8 Questions
- •9.9 Exercises
- •References
- •10.1 Introduction
- •10.1.1 Allometric Modeling of Biomass
- •10.1.2 Chapter Outline
- •10.2 Aerial Photo Mensuration
- •10.2.1 Principles of Aerial Photogrammetry
- •10.2.1.1 Geometric Basis of Photogrammetric Measurement
- •10.2.1.2 Ground Control and Direct Georeferencing
- •10.2.2 Tree Height Measurement Using Forest Photogrammetry
- •10.2.2.2 Automated Methods in Forest Photogrammetry
- •10.3 Airborne Laser Scanning
- •10.3.1 Principles of Airborne Laser Scanning
- •10.3.1.1 Lidar-Based Measurement of Terrain and Canopy Surfaces
- •10.3.2 Individual Tree-Level Measurement Using Lidar
- •10.3.2.1 Automated Individual Tree Measurement Using Lidar
- •10.3.3 Area-Based Approach to Estimating Biomass with Lidar
- •10.4 Future Developments
- •10.5 Concluding Remarks
- •10.6 Further Reading
- •10.7 Questions
- •References
- •11.1 Introduction
- •Chapter Outline
- •11.2 Volumetric Data Acquisition
- •11.2.1 Computed Tomography
- •11.2.1.1 Characteristics of 3D CT Data
- •11.2.2 Positron Emission Tomography (PET)
- •11.2.2.1 Characteristics of 3D PET Data
- •Relaxation
- •11.2.3.1 Characteristics of the 3D MRI Data
- •Image Quality and Artifacts
- •11.2.4 Summary
- •11.3 Surface Extraction and Volumetric Visualization
- •11.3.1 Surface Extraction
- •Example: Curvatures and Geometric Tools
- •11.3.2 Volume Rendering
- •11.3.3 Summary
- •11.4 Volumetric Image Registration
- •11.4.1 A Hierarchy of Transformations
- •11.4.1.1 Rigid Body Transformation
- •11.4.1.2 Similarity Transformations and Anisotropic Scaling
- •11.4.1.3 Affine Transformations
- •11.4.1.4 Perspective Transformations
- •11.4.1.5 Non-rigid Transformations
- •11.4.2 Points and Features Used for the Registration
- •11.4.2.1 Landmark Features
- •11.4.2.2 Surface-Based Registration
- •11.4.2.3 Intensity-Based Registration
- •11.4.3 Registration Optimization
- •11.4.3.1 Estimation of Registration Errors
- •11.4.4 Summary
- •11.5 Segmentation
- •11.5.1 Semi-automatic Methods
- •11.5.1.1 Thresholding
- •11.5.1.2 Region Growing
- •11.5.1.3 Deformable Models
- •Snakes
- •Balloons
- •11.5.2 Fully Automatic Methods
- •11.5.2.1 Atlas-Based Segmentation
- •11.5.2.2 Statistical Shape Modeling and Analysis
- •11.5.3 Summary
- •11.6 Diffusion Imaging: An Illustration of a Full Pipeline
- •11.6.1 From Scalar Images to Tensors
- •11.6.2 From Tensor Image to Information
- •11.6.3 Summary
- •11.7 Applications
- •11.7.1 Diagnosis and Morphometry
- •11.7.2 Simulation and Training
- •11.7.3 Surgical Planning and Guidance
- •11.7.4 Summary
- •11.8 Concluding Remarks
- •11.9 Research Challenges
- •11.10 Further Reading
- •Data Acquisition
- •Surface Extraction
- •Volume Registration
- •Segmentation
- •Diffusion Imaging
- •Software
- •11.11 Questions
- •11.12 Exercises
- •References
- •Index
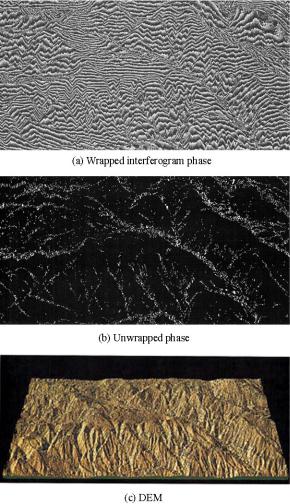
390 |
H. Wei and M. Bartels |
Fig. 9.10 InSAR generated DEMs from a pair of ERS-1 SAR images of a mountainous region of Sardinia, Italy. Figure courtesy of [37]
9.4 DEM Generation from LIDAR
9.4.1 LIDAR Data Acquisition
Airborne Laser Scanning (ALS) or LIght Detection And Ranging (LIDAR) has revolutionized topographic surveying for fast acquisition of high-resolution elevation data, which has enabled the development of highly accurate 3D products. LIDAR was developed by NASA in the 1970s [3]. Since the early 1990s, LIDAR has made significant contributions to many environmental, engineering and civil applications. It is being used increasingly by the public and commercial sectors [108] for forestry, archeology, 3D city map generation, flood simulations, coastal erosion monitoring,

9 3D Digital Elevation Model Generation |
391 |
Fig. 9.11 LIDAR acquisition principle
landslide prediction, corridor mapping and wave propagation models for mobile telecommunication networks.
From an airborne platform, a LIDAR system estimates the distance between the instrument and a point on the surface by measuring the time for a round-trip of a laser pulse, as illustrated in Fig. 9.11. Differential Global Positioning System (DGPS) and Inertial Navigation System (INS) complement the data with position and orientation, respectively [78]. The result is the collection of surface height information in the form of a dense 3D point cloud. LIDAR is an active sensor which relies on the amount of backscattered light. The portion of light returning back to the receiver depends on the scene surface type illuminated by the laser pulse. Consequently, there will be gaps and overlaps in the data which is therefore not homogeneously and regularly distributed [14]. Additionally, since LIDAR is an active sensor and is independent of external lighting conditions, it can be used at night, a great advantage compared to traditional stereo matching [93]. Typical flight heights range from 600 m to 950 m [14, 81], scan angles 7–23◦, wavelengths 1047–1560 nm, scan rates 13–653 Hz, and pulse rates 5–83 kHz [25, 78]. Average footprint sizes range typically from 0.4–1.0 m, depending on the flight height [20, 91]. Scan and pulse rates, in particular, have grown in recent years, leading to higher point densities as a function of flight height, velocity of the aircraft, scan angle, pulse rate and scan rate [10, 13].
The capability of the laser to penetrate gaps in vegetation allows the measurement of occluded ground. This is due to the laser’s large footprint relative to the vegetation gaps. At least the First Echo (FE) and the Last Echo (LE) can be recorded, as schematically depicted in Fig. 9.11. Ideally, the FE and LE are arranged in two different point clouds as subsets within LIDAR data [122] and clearly the differentiation of multiple echoes is a challenge. Modern LIDAR systems can record up to four echoes (or more if derived from full waveform datasets), while the latest survey techniques, such as low-level LIDAR, allow point densities up to 100 points per square meter [28]. The latest developments of the full waveform LIDAR have introduced a new range of applications. The recording of multiple echoes allows modeling of the vertical tree profile [14]. Acquired FE points mostly comprise canopies, sheds, roofs, dormers and even small details such as aerials or chimneys, whereas LEs are reflections of the ground or other lower surfaces after the laser has
392 |
|
H. Wei and M. Bartels |
Table 9.3 LIDAR versus aerial photogrammetry |
|
|
|
|
|
Property |
LIDAR |
Aerial photogrammetry |
|
|
|
Sensor |
Active |
Passive |
Georeferencing |
Direct |
Post-acquisition |
Point distribution |
Irregular |
Regular |
Vertical accuracy |
High |
Low |
Horizontal accuracy |
Low |
High |
Height information |
Directly from data |
Stereo matching required |
Degree of automation |
High |
Low |
Maintenance |
Intensive and expensive |
Inexpensive |
Life time |
Limited |
Long |
Contrast |
High |
Low |
Texture |
None |
Yes |
Color |
Monochrome |
Multispectral |
Shadows |
Minimal effect |
Clearly visual |
Ground captivity |
Vegetation gap penetration |
Wide open spaces required |
Breakline detection |
Difficult due to irregular sampling |
Suitable |
DTM generation source |
Height data |
Textured overlapping imagery |
Planimetry |
Random irregular point cloud |
Rich spatial context |
Land cover classification |
Unsuitable, fusion required |
Suitable |
|
|
|
penetrated gaps within the foliage. The occurrence of multiple echoes at the same geo-referenced point indicates vegetation but also edges of hard objects [10]. The difference between FE and LE therefore reveals, in the best case, building outlines and vegetation heights [138]. Table 9.3 summarizes the pros and cons of LIDAR versus traditional aerial photogrammetric techniques.
9.4.2 Accuracy, Error Types and Countermeasures
LIDAR is the most accurate remote sensing source for capturing height. It has revolutionized photogrammetry with regard to vertical accuracy, with values ranging between 0.10–0.26 m [3, 4, 34, 74, 104, 107, 167, 179]. Like any other physical measurements, LIDAR errors are composed of both systematic and random errors. Systematic errors are due to the setting of the equipment; generally they have a certain pattern and are therefore reproducible. Examples are systematic height shifts as a function of flight height, which are due to uncertainty of GPS, georeferencing and orientation. Time errors of assigning range and position are reported to be <10 μs [167]. Calibration can be carried out by using known mounting angles, the redundancy from overlapping (crossing) strips and ground truth (i.e. manually measured

9 3D Digital Elevation Model Generation |
393 |
GCPs which have been acquired in an accompanying field survey). Problems occur if the positions of GCPs and measured LIDAR points do not correspond with each other [137]. This can be solved using a high point density, acquired with a low altitude, low velocity and a steep scan angle.
In contrast, random errors are not reproducible and are thus more difficult to eliminate. Gross errors, blunders and ‘no data’ situations are due to a temporal malfunction of the LRF or occasional perturbations such as birds or low flying aircrafts [143]. Data voids are due to the irregular sampling nature of the LIDAR and have to be corrected using interpolation if required. The total number of gross errors and blunders may be small, but their overall impact on the data is strong. Kidner et al. [89] reported that local blunders can be large as 20 m and can be detected if the height of all neighbouring points is below a certain threshold, for example ≤5 m. Extreme blunders can be greater than 767 m [89] and can be removed by comparing the mean and standard deviation of the height differences of each LIDAR point in a defined neighborhood.
Point density and scan range per scanned line are a function of scan angle, however it is also known that the greater the scan angle the greater its elevation error [179]. The error caused by the scan angle becomes smaller when the flight height and pulse rate are lower [7]. A small scan angle ensures that terrain or object edges are resolved more clearly and vertical tree profiles can be sampled due to the fact that laser pulses reach lower tree branches [104]. In general, the smaller the scan angle, the higher the point density, the better the edges on man-made structure are sampled, and the better the laser can be pushed forward to lower branch levels of trees [138].
Multipath propagation causes backscattered laser light to travel a longer time. Consequently, some points will appear below the actual surface [91, 143]. Multipath effects of LE LIDAR data can be compensated for by exploiting the redundant information of FE and LE [166].
Finally, wind, atmospheric distortions and bright sunlight can all influence the accuracy of LIDAR data as the laser beam is narrow [12]. The best laser ranging conditions are achieved if the weather is dry, cool and clear [12]. Furthermore, water vapour and humidity such as rain and fog attenuate the power of the laser. This disturbing effect can be deliberately exploited for investigating clouds and aerosols in the atmosphere using ground-based LIDAR [77]. To generalize, studies have revealed that the greater slope, terrain roughness, amount of vegetation and the larger the flight velocity and height, the smaller the scan angle, the larger the random error will be [14, 74, 93, 179].
To reduce inaccuracies within the acquired data, error models are applied. A popular method to estimate the total error can be done by calculating the Root Mean Square Error (RMSE) of equal georeferenced GCPs and LIDAR points as defined in [74]:
|
= |
NGCP |
|
− |
|
|
|
RMSE |
|
|
1 |
NGCP(Xj |
|
GCPj )2 |
(9.22) |
|
|
|
|||||
|
|
|
|
j 1 |
|
|
|
|
|
|
|
|
|
|
|
|
|
|
|
= |
|
|
|
394 |
|
H. Wei and M. Bartels |
Table 9.4 Typical systematic and random errors in LIDAR point clouds |
||
|
|
|
Error (Type) |
Order |
Counter measure |
|
|
|
Height shift (Systematic) |
≤20 cm |
Ground control points |
GPS shift (Systematic) |
10 cm |
Differential GPS |
Georeferencing (Systematic) |
<10 μs |
Strip adjustment, redundancy, revisit |
Orientation (Systematic) |
<10 μs |
Strip adjustment, redundancy, revisit |
Mounting angles (Systematic) |
N/A |
Calibrate and recalculate |
Slope of terrain (Random) |
(15–20) cm |
Slope adaptive algorithm |
Gross errors/blunders (Random) |
>100 cm |
FE/LE redundancy, manual intervention |
Object roughness (Random) |
<10 cm |
Surveys in leaf-off season |
Multipath (Random) |
<10 cm |
Small scan angle, FE/LE redundancy |
Bright sun light (Random) |
N/A |
Surveys at night |
Atmosphere, weather (Random) |
N/A |
Avoid forest fires, clouds, fog |
Strong winds (Random) |
N/A |
Avoid rough weather |
|
|
|
where NGCP is the total number of GCPj and Xj the corresponding LIDAR points with j {1, 2, . . . , NGCP}. To break the absolute error down, mean height differences (systematic error) and standard deviation of difference (random error) can be calculated. Yu [179] observed that the random error decreases at greater vegetation penetration rates (i.e. the more open the space is), greater point densities and lower flight heights, while the systematic error remains stable at different flight heights. Even if there is no initial vertical error evident, a horizontal error still has an impact on the vertical error. Hodgson et al. [75] established a link between the terrain slope angle α and the horizontal displacement eh of the LIDAR point yielding the vertical error ev :
ev = eh tan(α) |
(9.23) |
where the horizontal error eh is dominated by the flight height h, which is a general rule of thumb [74]
eh ≈ 0.1 % h |
(9.24) |
Systematic and random errors can be corrected by GCPs, surveyed at the same time with LIDAR data. From another point of view, LIDAR itself can be used to provide GCPs for accuracy assessment of photogrammetric products as recently demonstrated by James et al. [84]. Systematic errors can either be eliminated by careful calibration or, if known, calculated and removed from the error budget. Random errors can only be estimated after the survey in a post-processing analysis. Table 9.4 summarizes all typical systematic and random errors and states countermeasures.