
- •1 Introduction
- •1.1 What makes eigenvalues interesting?
- •1.2 Example 1: The vibrating string
- •1.2.1 Problem setting
- •1.2.2 The method of separation of variables
- •1.3.3 Global functions
- •1.3.4 A numerical comparison
- •1.4 Example 2: The heat equation
- •1.5 Example 3: The wave equation
- •1.6 The 2D Laplace eigenvalue problem
- •1.6.3 A numerical example
- •1.7 Cavity resonances in particle accelerators
- •1.8 Spectral clustering
- •1.8.1 The graph Laplacian
- •1.8.2 Spectral clustering
- •1.8.3 Normalized graph Laplacians
- •1.9 Other sources of eigenvalue problems
- •Bibliography
- •2 Basics
- •2.1 Notation
- •2.2 Statement of the problem
- •2.3 Similarity transformations
- •2.4 Schur decomposition
- •2.5 The real Schur decomposition
- •2.6 Normal matrices
- •2.7 Hermitian matrices
- •2.8 Cholesky factorization
- •2.9 The singular value decomposition (SVD)
- •2.10 Projections
- •2.11 Angles between vectors and subspaces
- •Bibliography
- •3 The QR Algorithm
- •3.1 The basic QR algorithm
- •3.1.1 Numerical experiments
- •3.2 The Hessenberg QR algorithm
- •3.2.1 A numerical experiment
- •3.2.2 Complexity
- •3.3 The Householder reduction to Hessenberg form
- •3.3.2 Reduction to Hessenberg form
- •3.4 Improving the convergence of the QR algorithm
- •3.4.1 A numerical example
- •3.4.2 QR algorithm with shifts
- •3.4.3 A numerical example
- •3.5 The double shift QR algorithm
- •3.5.1 A numerical example
- •3.5.2 The complexity
- •3.6 The symmetric tridiagonal QR algorithm
- •3.6.1 Reduction to tridiagonal form
- •3.6.2 The tridiagonal QR algorithm
- •3.7 Research
- •3.8 Summary
- •Bibliography
- •4.1 The divide and conquer idea
- •4.2 Partitioning the tridiagonal matrix
- •4.3 Solving the small systems
- •4.4 Deflation
- •4.4.1 Numerical examples
- •4.6 Solving the secular equation
- •4.7 A first algorithm
- •4.7.1 A numerical example
- •4.8 The algorithm of Gu and Eisenstat
- •4.8.1 A numerical example [continued]
- •Bibliography
- •5 LAPACK and the BLAS
- •5.1 LAPACK
- •5.2 BLAS
- •5.2.1 Typical performance numbers for the BLAS
- •5.3 Blocking
- •5.4 LAPACK solvers for the symmetric eigenproblems
- •5.6 An example of a LAPACK routines
- •Bibliography
- •6 Vector iteration (power method)
- •6.1 Simple vector iteration
- •6.2 Convergence analysis
- •6.3 A numerical example
- •6.4 The symmetric case
- •6.5 Inverse vector iteration
- •6.6 The generalized eigenvalue problem
- •6.7 Computing higher eigenvalues
- •6.8 Rayleigh quotient iteration
- •6.8.1 A numerical example
- •Bibliography
- •7 Simultaneous vector or subspace iterations
- •7.1 Basic subspace iteration
- •7.2 Convergence of basic subspace iteration
- •7.3 Accelerating subspace iteration
- •7.4 Relation between subspace iteration and QR algorithm
- •7.5 Addendum
- •Bibliography
- •8 Krylov subspaces
- •8.1 Introduction
- •8.3 Polynomial representation of Krylov subspaces
- •8.4 Error bounds of Saad
- •Bibliography
- •9 Arnoldi and Lanczos algorithms
- •9.2 Arnoldi algorithm with explicit restarts
- •9.3 The Lanczos basis
- •9.4 The Lanczos process as an iterative method
- •9.5 An error analysis of the unmodified Lanczos algorithm
- •9.6 Partial reorthogonalization
- •9.7 Block Lanczos
- •9.8 External selective reorthogonalization
- •Bibliography
- •10 Restarting Arnoldi and Lanczos algorithms
- •10.2 Implicit restart
- •10.3 Convergence criterion
- •10.4 The generalized eigenvalue problem
- •10.5 A numerical example
- •10.6 Another numerical example
- •10.7 The Lanczos algorithm with thick restarts
- •10.8 Krylov–Schur algorithm
- •10.9 The rational Krylov space method
- •Bibliography
- •11 The Jacobi-Davidson Method
- •11.1 The Davidson algorithm
- •11.2 The Jacobi orthogonal component correction
- •11.2.1 Restarts
- •11.2.2 The computation of several eigenvalues
- •11.2.3 Spectral shifts
- •11.3 The generalized Hermitian eigenvalue problem
- •11.4 A numerical example
- •11.6 Harmonic Ritz values and vectors
- •11.7 Refined Ritz vectors
- •11.8 The generalized Schur decomposition
- •11.9.1 Restart
- •11.9.3 Algorithm
- •Bibliography
- •12 Rayleigh quotient and trace minimization
- •12.1 Introduction
- •12.2 The method of steepest descent
- •12.3 The conjugate gradient algorithm
- •12.4 Locally optimal PCG (LOPCG)
- •12.5 The block Rayleigh quotient minimization algorithm (BRQMIN)
- •12.7 A numerical example
- •12.8 Trace minimization
- •Bibliography

12.4. LOCALLY OPTIMAL PCG (LOPCG) |
229 |
Dividing the largest eigenvalue of A−1(A−λ1M) by the smallest positive gives the condition number
|
|
|
|
|
|
|
λ1 |
|
|
|
|
|
|
|
(12.35) |
κ1 := κ A−1(A |
λ1M) |
|
= |
1 − λn = λ2 λn − λ1 |
= λ2 κ0. |
||||||||
|
|
− |
|
|
|
|
|
|
|
|
|
|
|
|
|
R |
(u1) M |
|
1 − λ2 |
|
λn λ2 − λ1 |
λn |
|||||||
|
|
|
|
|
|
λ1 |
|
|
|
|
|
|
|
If λ2 λn then the condition number is much reduced. Further, κ1 is bounded independently of n,
(12.36) |
κ1 = |
1 − λ1/λn |
< |
1 |
. |
|
|
||||
|
|
1 − λ1/λ2 |
1 − λ1/λ2 |
So, with this particular preconditioner, κ1 does not dependent on the choice of the meshwidth h in the FEM application.
The previous discussion suggests to choose C in such way that C C A. C could, for
=
instance, be obtained form an Incomplete Cholesky decomposition. We make this choice in the numerical example below.
Notice that the transformation x −→ y = Cx need not be made explicitly. In partic-
˜ ˜
ular, the matrices A and M must not be formed. As with the preconditioned conjugate gradient algorithm for linear systems there is an additional step in the algorithm where the preconditioned residual is computed, see Fig. 12.1 on page 230.
12.4Locally optimal PCG (LOPCG)
The parameter δk in the RQMIN und (P)CG algorithms is determined such that
(12.37) |
ρ(xk+1) = ρ(xk + δkpk), pk = −gk + αkpk−1 |
is minimized. αk is chosen to make consecutive search directions conjugate. Knyazev [6] proposed to optimize both parameters, αk and δk, at once.
(12.38) |
ρ(xk+1) = |
min ρ(x |
k − |
δg |
k |
+ γp |
k−1 |
) |
δ,γ |
|
|
|
This results in potentially smaller values for the Rayleigh quotient, as
δ,γ |
k − |
δg |
k |
+ γp |
k−1 |
≤ |
δ |
xk − δ(gk − αkpk) . |
min ρ x |
|
|
|
|
min |
|
Hence, Knyazev coined the notion “locally optimal”.
ρ(xk+1) in (12.38) is the minimal eigenvalue of the 3 × 3 eigenvalue problem
|
|
xk |
|
− |
α |
|
|
xk |
|
− |
α |
|
|
pk−1 |
γ |
pk−1 |
γ |
||||||||
(12.39) |
|
−gk |
A[xk, |
|
gk, pk−1] β |
|
= λ |
−gk |
M[xk, |
|
gk, pk−1] β |
|
We normalize the eigenvector corresponding to the smallest eigenvalue such that its first component becomes 1,
[1, δk, γk] := [1, β/α, γ/α].
These values of δk and γk are the parameters that minimize the right hand side in (12.38). Then we can write
(12.40) xk+1 |
= xk − δkgk + γkpk−1 |
= xk + δk (−gk + (γk/δk)pk−1) |
= xk + δkpk. |
||||
|
|
| |
|
{z |
k |
} |
|
|
|
|
=:p |
|
|
We can consider xk+1 as having been obtained by a Rayleigh quotient minimization from xk along pk = −gk +(γk/δk)pk−1. Notice that this direction is needed in the next iteration step. (Otherwise it is not of a particular interest.)
230 CHAPTER 12. RAYLEIGH QUOTIENT AND TRACE MINIMIZATION
function [x,rho,log] = rqmin1(A,M,x,tol,C)
%RQMIN1 |
[x,rho] = rqmin1(A,M,x0,tol,C) |
%cg-Rayleigh quotient minimization for the computation
% of the smallest eigenvalue of A*x = lambda*M*x,
%A and M are symmetric, M spd. x0 initial vector
%C’*C preconditioner
%tol: convergence criterium:
%||2*(C’*C)\(A*x - lam*M*x)|| < tol
%PA 16.6.2000
u = |
M*x; |
|
|
|
q = |
sqrt(x’*u); |
|
|
|
x = |
x/q; u = u/q; |
|
|
|
v = |
A*x; |
|
|
|
rho |
= |
x’*v; |
|
|
k = |
0; g = x; gnorm = 1; |
log=[]; |
% Initializations |
|
while |
gnorm > tol, |
|
|
|
k |
= |
k + 1; |
|
|
galt = g; |
|
|
||
if exist(’C’), |
|
|
||
|
g |
= 2*(C\(C’\(v - rho*u))); |
% preconditioned gradient |
|
else |
|
|
|
|
|
g |
= 2*(v - rho*u); |
% gradient |
|
end |
|
|
|
|
if k == 1, |
|
|
||
|
p |
= -g; |
|
|
else |
|
|
|
|
|
p |
= -g + (g’*M*g)/(galt’*M*galt)*p; |
||
end |
|
|
|
[qq,ll] = eig([x p]’*[v A*p],[x p]’*[u M*p]); [rho,ii] = min(diag(ll));
delta = qq(2,ii)/qq(1,ii);
x = x + delta*p; u = M*x;
q = sqrt(x’*u);
x = x/q; u = u/q; v = A*x;
gnorm = norm(g);
if nargout>2, log = [log; [k,rho,gnorm]]; end end
Figure 12.1: MATLAB code RQMIN: Rayleigh quotient minimization
12.4. LOCALLY OPTIMAL PCG (LOPCG) |
231 |
function [x,rho,log] = lopcg(A,M,x,tol,C) %RQMIN1 [x,rho] = lopcg(A,M,x0,tol,C)
%Locally Optimal Proconditioned CG algorithm for
% computing the smallest eigenvalue of A*x = lambda*M*x,f
%where A and M are symmetrisch, M spd.
%x0 initial vektor
%C’*C preconditioner
%tol: stopping criterion:
%(C’*C)\(A*x - lam*M*x) < tol
%PA 2002-07-3
n = size(M,1); u = M*x;
q = sqrt(x’*u); x = x/q; u = u/q; v = A*x;
rho = x’*v;
k = 0; |
gnorm = 1; |
log=[]; % initializations |
||
while gnorm > tol, |
|
|
||
k = k |
+ |
1; |
|
|
g = v |
- |
rho*u; |
% |
gradient |
gnorm |
= |
norm(g); |
|
|
if exist(’C’), |
|
|
||
g = |
(C\(C’\g)); |
% |
preconditioned gradient |
|
end |
|
|
|
|
if k == |
1, p = zeros(n,0); end |
aa = [x -g p]’*[v A*[-g p]]; aa = (aa+aa’)/2; mm = [x -g p]’*[u M*[-g p]]; mm = (mm+mm’)/2; [qq,ll] = eig(aa,mm);
[rho,ii] = min(diag(ll)); delta = qq(:,ii);
p = [-g p]*delta(2:end); x = delta(1)*x + p;
u = M*x;
q = sqrt(x’*u);
x = x/q; u = u/q; v = A*x;
if nargout>2, log = [log; [k,rho,gnorm]]; end end
Figure 12.2: MATLAB code LOPCG: Locally Optimal Preconditioned Conjugate Gradient algorithm
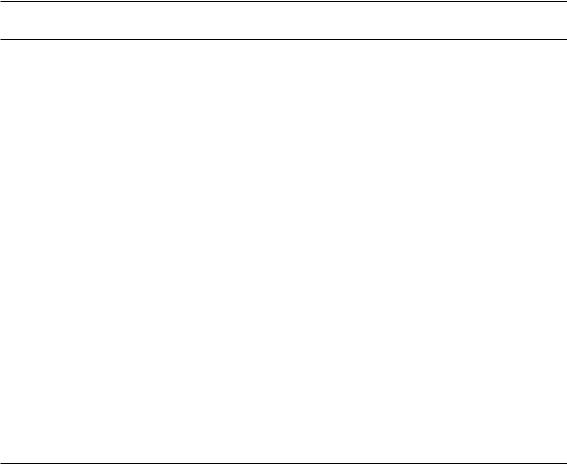
232 CHAPTER 12. RAYLEIGH QUOTIENT AND TRACE MINIMIZATION
12.5The block Rayleigh quotient minimization algorithm (BRQMIN)
The above procedures converge very slowly if the eigenvalues are clustered. Hence, these methods should be applied only in blocked form.
Longsine and McCormick [8] suggested several variants for blocking Algorithm 12.1. See [1] for a recent numerical investigation of this algorithm.
12.6The locally-optimal block preconditioned conjugate gradient method (LOBPCG)
In BRQMIN the Rayleigh quotient is minimized in the 2q-dimensional subspace generated by the eigenvector approximations Xk and the search directions Pk = −Hk + Pk−1Bk, where the Hk are the preconditioned residuals corresponding to Xk and Bk is chosen such that the block of search directions is conjugate. Instead, Knyazev [6] suggests that the space for the minimization be augmented by the q-dimensional subspace R(Hk). The resulting algorithm is deemed ‘locally-optimal’ because ρ(x) is minimized with respect to all available vectors.
Algorithm 12.2 The locally-optimal block preconditioned conjugate gradient method (LOBPCG) for solving Ax = λMx with preconditioner N of [1]
1: |
Choose random matrix X0 Rn×q with X0T MX0 = Iq. Set Q := [ ]. |
|
2: |
Compute (X0T AX0)S0 = S0Θ0 |
/* (Spectral decomposition) */ |
|
where S0T S0 = Iq, Θ0 = diag(ϑ1, . . . , ϑq), |
ϑ1 ≤ . . . ≤ ϑq. |
3: X0 := X0S0; R0 := AX0 − MX0Θ0; P0 := [ ]; k := 0.
4:while rank(Q) < p do
5:Solve the preconditioned linear system NHk = Rk
6:Hk := Hk − Q(QT MHk).
7: |
A := [Xk, Hk, Pk]T A[Xk, Hk, Pk]. |
|
|
|
|
|
|
|
|
|||||||||||||||||||||
8: |
M := [X |
|
, H |
|
, P |
]T M[X |
, H |
, P |
]. |
|
|
|
|
|
|
|||||||||||||||
|
e |
|
|
|
k |
|
k |
|
|
k |
|
|
|
|
|
k |
|
k |
k |
|
|
|
|
|
|
≤ |
≤ |
|||
|
|
|
|
|
k |
|
eek |
|
|
fe e k |
|
|
|
|
|
|
|
|
|
|||||||||||
9: |
Compute AS |
|
|
= MS |
Θ |
|
|
|
|
|
|
|
/* (Spectral decomposition) */ |
|||||||||||||||||
|
f |
|
|
T |
|
|
|
k |
|
|
|
|
k |
e |
k |
|
|
|
|
|
|
|
|
|
|
|
||||
|
k |
|
ek |
|
|
fe |
|
= I3q, |
|
|
= diag(ϑ1, . . . , ϑ3q), ϑ1 |
|
. . . ϑ3q. |
|||||||||||||||||
|
where S |
|
|
MS |
|
Θ |
|
|
||||||||||||||||||||||
10: |
S |
|
:= S |
|
[e1, . . . , eq], |
Θ := diag(ϑ1, . . . , ϑq). |
|
|
|
|||||||||||||||||||||
11: |
P |
|
|
:= [H |
, P |
] S |
|
; |
X |
|
|
:= X |
S |
|
+ P |
|
. |
|
|
|||||||||||
12: |
|
k+1 |
e |
|
|
|
k |
|
|
|
k |
|
|
k,2 |
|
|
|
k+1 |
|
|
k |
|
k,1 |
|
k+1 |
|
|
|
||
|
Rk+1 := AXk+1 − MXk+1Θk. |
|
|
|
|
|
|
|
|
|||||||||||||||||||||
13: |
k := k + 1. |
|
|
|
|
|
|
|
|
|
|
|
|
|
|
|
|
|
|
|
|
|
|
|||||||
14: |
for i = 1, . . . , q do |
|
|
|
|
|
|
|
|
|
|
|
|
|
|
|
||||||||||||||
15: |
|
/* (Convergence test) */ |
|
|
|
|
|
|
|
|
|
|
||||||||||||||||||
16: |
|
if kRkeik < tol then |
|
|
|
|
|
|
|
|
|
|
|
|
|
|||||||||||||||
17: |
|
|
Q := [Q, Xkei]; |
|
Xkei := t, with t a random vector. |
18:M-orthonormalize the columns of Xk.
19:end if
20:end for
21:end while
If dj = [dT1j , dT2j , dT3j ]T , dij Rq, is the eigenvector corresponding to the j-th eigenvalue of (12.1) restricted to R([Xk, Hk, Pk−1]), then the j-th column of Xk+1 is the corresponding