
- •1 Introduction
- •1.1 What makes eigenvalues interesting?
- •1.2 Example 1: The vibrating string
- •1.2.1 Problem setting
- •1.2.2 The method of separation of variables
- •1.3.3 Global functions
- •1.3.4 A numerical comparison
- •1.4 Example 2: The heat equation
- •1.5 Example 3: The wave equation
- •1.6 The 2D Laplace eigenvalue problem
- •1.6.3 A numerical example
- •1.7 Cavity resonances in particle accelerators
- •1.8 Spectral clustering
- •1.8.1 The graph Laplacian
- •1.8.2 Spectral clustering
- •1.8.3 Normalized graph Laplacians
- •1.9 Other sources of eigenvalue problems
- •Bibliography
- •2 Basics
- •2.1 Notation
- •2.2 Statement of the problem
- •2.3 Similarity transformations
- •2.4 Schur decomposition
- •2.5 The real Schur decomposition
- •2.6 Normal matrices
- •2.7 Hermitian matrices
- •2.8 Cholesky factorization
- •2.9 The singular value decomposition (SVD)
- •2.10 Projections
- •2.11 Angles between vectors and subspaces
- •Bibliography
- •3 The QR Algorithm
- •3.1 The basic QR algorithm
- •3.1.1 Numerical experiments
- •3.2 The Hessenberg QR algorithm
- •3.2.1 A numerical experiment
- •3.2.2 Complexity
- •3.3 The Householder reduction to Hessenberg form
- •3.3.2 Reduction to Hessenberg form
- •3.4 Improving the convergence of the QR algorithm
- •3.4.1 A numerical example
- •3.4.2 QR algorithm with shifts
- •3.4.3 A numerical example
- •3.5 The double shift QR algorithm
- •3.5.1 A numerical example
- •3.5.2 The complexity
- •3.6 The symmetric tridiagonal QR algorithm
- •3.6.1 Reduction to tridiagonal form
- •3.6.2 The tridiagonal QR algorithm
- •3.7 Research
- •3.8 Summary
- •Bibliography
- •4.1 The divide and conquer idea
- •4.2 Partitioning the tridiagonal matrix
- •4.3 Solving the small systems
- •4.4 Deflation
- •4.4.1 Numerical examples
- •4.6 Solving the secular equation
- •4.7 A first algorithm
- •4.7.1 A numerical example
- •4.8 The algorithm of Gu and Eisenstat
- •4.8.1 A numerical example [continued]
- •Bibliography
- •5 LAPACK and the BLAS
- •5.1 LAPACK
- •5.2 BLAS
- •5.2.1 Typical performance numbers for the BLAS
- •5.3 Blocking
- •5.4 LAPACK solvers for the symmetric eigenproblems
- •5.6 An example of a LAPACK routines
- •Bibliography
- •6 Vector iteration (power method)
- •6.1 Simple vector iteration
- •6.2 Convergence analysis
- •6.3 A numerical example
- •6.4 The symmetric case
- •6.5 Inverse vector iteration
- •6.6 The generalized eigenvalue problem
- •6.7 Computing higher eigenvalues
- •6.8 Rayleigh quotient iteration
- •6.8.1 A numerical example
- •Bibliography
- •7 Simultaneous vector or subspace iterations
- •7.1 Basic subspace iteration
- •7.2 Convergence of basic subspace iteration
- •7.3 Accelerating subspace iteration
- •7.4 Relation between subspace iteration and QR algorithm
- •7.5 Addendum
- •Bibliography
- •8 Krylov subspaces
- •8.1 Introduction
- •8.3 Polynomial representation of Krylov subspaces
- •8.4 Error bounds of Saad
- •Bibliography
- •9 Arnoldi and Lanczos algorithms
- •9.2 Arnoldi algorithm with explicit restarts
- •9.3 The Lanczos basis
- •9.4 The Lanczos process as an iterative method
- •9.5 An error analysis of the unmodified Lanczos algorithm
- •9.6 Partial reorthogonalization
- •9.7 Block Lanczos
- •9.8 External selective reorthogonalization
- •Bibliography
- •10 Restarting Arnoldi and Lanczos algorithms
- •10.2 Implicit restart
- •10.3 Convergence criterion
- •10.4 The generalized eigenvalue problem
- •10.5 A numerical example
- •10.6 Another numerical example
- •10.7 The Lanczos algorithm with thick restarts
- •10.8 Krylov–Schur algorithm
- •10.9 The rational Krylov space method
- •Bibliography
- •11 The Jacobi-Davidson Method
- •11.1 The Davidson algorithm
- •11.2 The Jacobi orthogonal component correction
- •11.2.1 Restarts
- •11.2.2 The computation of several eigenvalues
- •11.2.3 Spectral shifts
- •11.3 The generalized Hermitian eigenvalue problem
- •11.4 A numerical example
- •11.6 Harmonic Ritz values and vectors
- •11.7 Refined Ritz vectors
- •11.8 The generalized Schur decomposition
- •11.9.1 Restart
- •11.9.3 Algorithm
- •Bibliography
- •12 Rayleigh quotient and trace minimization
- •12.1 Introduction
- •12.2 The method of steepest descent
- •12.3 The conjugate gradient algorithm
- •12.4 Locally optimal PCG (LOPCG)
- •12.5 The block Rayleigh quotient minimization algorithm (BRQMIN)
- •12.7 A numerical example
- •12.8 Trace minimization
- •Bibliography
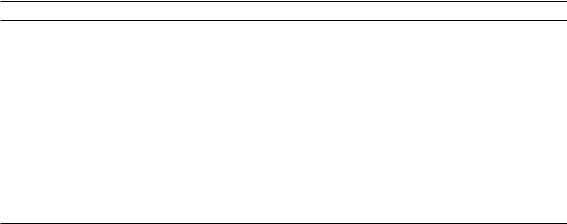
Chapter 10
Restarting Arnoldi and Lanczos algorithms
The number of iteration steps can be very high with the Arnoldi or the Lanczos algorithm. This number is, of course, not predictable. The iteration count depends on properties of the matrix, in particular the distribution of its eigenvalues, but also on the initial vectors.
High iteration counts entail a large memory requirement to store the Arnoldi/Lanczos vectors and a high amount of computation because of growing cost of the reorthogonalization.
The idea behind the implicitely restarted Arnoldi (IRA) and implicitely restarted Lanczos (IRL) algorithms is to reduce these costs by limiting the dimension of the search space. This means that the iteration is stopped after a number of steps (which is bigger than the number of desired eigenvalues), reduce the dimension of the search space without destroying the Krylov space tructure, and finally resume the Arnoldi / Lanczos iteration.
The implicitely restarted Arnoldi has first been proposed by Sorensen [7, 8]. It is implemented together with the implicitely restarted Lanczos algorithms in the software package ARPACK [4]. The ARPACK routines are the basis for the sparse matrix eigensolver eigs
in MATLAB.
10.1The m-step Arnoldi iteration
Algorithm 10.1 The m-step Arnoldi iteration
1: Let A Fn×n. This algorithm executes m steps of the Arnoldi algorithm.
2: q1 |
= x/ |
x |
; z = Aq1 |
; α1 |
= q z; |
|
|
|
|
k |
k |
|
|
1 |
|
3: r1 = w − α1q1; Q1 = [q1]; H1 = [α1]; |
|||||||
4: |
for j = 1, . . . , m − 1 do |
|
|
||||
5: |
|
βj := krj k; qj+1 = rj /βj ; |
Hj |
F(j+1)×j ; |
|||
|
|
|
|
|
|
||
6: |
|
Qj+1 := [Qj , qj+1]; |
Hˆj := βj ejT |
7:z := Aqj ;
8:h := Qj+1z; rj+1 := z − Qj+1h;
ˆ
9: Hj+1 := [Hj , h];
10: end for
We start with the Algorithm 10.1 that is a variant of the Arnoldi Algorithm 9.1. It
177

178 CHAPTER 10. RESTARTING ARNOLDI AND LANCZOS ALGORITHMS
executes just m Arnoldi iteration steps. We will now show how the dimension of the search space is reduced withouth losing the information regarding the eigenvectors one is looking for.
Remark 10.1. Step 8 in Algorithm 10.1 is classical Gram–Schmidt orthogonalization. As
rj+1 = z − Qj+1h = z − Qj+1Qj+1z,
we formally have Qj+1rj+1 = 0. However, classical Gram–Schmidt orthogonalization is faster but not so accurate as modified Gram–Schmidt orthogonalization [1]. So, often, Qj+1rj+1 is quite large. Therefore, the orthogonalization is iterated to get su cient orthogonality.
A possible modification of step 8 that incorporates a second iteration is
8: h := Q |
|
z; rj+1 := z |
− |
Qj+1h; |
|
|
|
|
|
|
|
|
|
|
|
|||||||||
j+1 |
|
|
|
|
|
|
|
|
|
|
|
|
|
|
|
|
|
|
|
|
|
|
||
c := Q |
rj+1; rj+1 |
:= rj+1 |
|
− |
Qj+1c; h = h + c; |
|
|
|||||||||||||||||
j+1 |
|
|
|
|
|
|
|
|
|
|
|
|
|
|
|
|
|
|
|
|
|
|||
Now we have, |
|
|
|
|
|
|
|
|
|
|
|
|
|
|
|
|
|
|
|
|
|
|
||
|
|
˜rj+1 = corrected rj+1 |
|
|
|
|
|
|
|
|
|
|
|
|||||||||||
|
|
= rj+1 − Qj+1 Qj+1rj+1 |
|
|
|
|
|
|
|
|||||||||||||||
|
|
|
|
|
|
|
|
j |
| |
|
|
{z |
|
} |
|
j+1 |
|
|
||||||
|
|
|
|
|
|
|
|
|
|
|
|
|
|
|||||||||||
|
|
= z |
|
Qj+1 Q +1z |
c |
Qj+1 Q rj+1 = z |
|
Qj+1(h + c) |
||||||||||||||||
|
|
− |
− |
− |
||||||||||||||||||||
|
|
|
|
|
| |
|
|
{z |
|
|
} |
|
|
|
| |
|
{z |
|
} |
|
hc
More iterations are possible but seldom necessary.
After the execution of Algorithm 10.1 we have the Arnoldi / Lanczos relation
|
AQm = QmHm + rmem, |
" |
# |
(10.1) |
Hm = |
|
|
available with |
|
|
|
|
rm = βmqm+1, |
kqm+1k = 1. |
|
If βm = 0 then R(Qm) is invariant under A, i.e., Ax R(Qm) for all x R(Qm). This lucky situation implies that σ(Hm) σm(A). So, the Ritz values and vectors are eigenvalues and eigenvectors of A.
What we can realistically hope for is βm being small. Then,
AQm − rmem = (A − rmqm)Qm = QmHm.
Then, R(Qm) is invariant under a matrix A + E, that di ers from A by a perturbation E with kEk = krmk = |βm|. From general eigenvalue theory we know that this in this situation well-conditioned eigenvalues of Hm are good approximations of eigenvalues of A.
In the sequel we investigate how we can find a q1 such that βm becomes small?
10.2Implicit restart
Let us start from the Arnoldi relation
(10.2) AQm = QmHm + rmem,
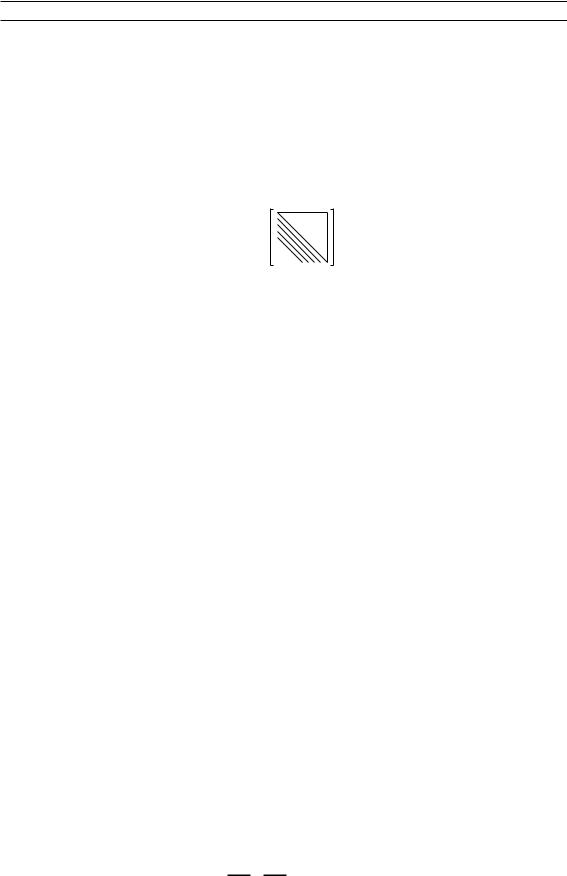
10.2. IMPLICIT RESTART |
179 |
Algorithm 10.2 k implicit QR steps applied to Hm
1:Hm+ := Hm.
2:for i := 1, . . . , k do
3: |
Hm+ := Vi Hm+Vi, where Hm+ − µiI = ViRi (QR factorization) |
4: |
end for |
that is obtained after calling Algorithm 10.1.
We apply k < m implicit QR steps to Hm with shifts µ1, . . . , µk, see Algorithm 10.2. Let V + := V1V2 · · · Vk. V + is the product of k (unitary) Hessenberg matrices whence it has k nonzero o -diagonals below its main diagonal.
Vm+ = |
. |
|
} |
|
k |
We define |
|
|
Qm+ := QmV +, Hm+ := (V +) HmV +. |
Then, from (10.2) we obtain |
|
|
AQmV + = QmV +(V +) HmV + + rmemV +, |
or |
|
(10.3) |
AQm+ = Qm+ Hm+ + rmemV +. |
As V + has k nonzero o -diagonals below the main diagonal, the last row of V + has the form
e |
V + = (0, . . . , 0, |
|
, . . . , |
|
), k + p = m. |
|||||||
m |
|
|
|
|
|
|
|
|
|
|||
|
| |
|
{z |
|
} | |
|
{z |
|
|
} |
|
|
|
|
p − 1 |
|
k + 1 |
|
We now simply discard the last k columns of (10.3).
AQ+m(:, 1 : p) = Q+mHm+(:, 1 : p) + rmemV +(:, 1 : p)
= Qm+ (:, 1 : p)Hm+(1 : p, 1 |
: p) + hp++1,p qp++1ep + vm,p+ rmep |
|||||||||
+ |
+ |
| |
|
{z |
|
} |
+ |
|
+ |
|
|
|
|
|
|||||||
|
|
|
βp+ |
|
|
|
|
|||
= Qm(:, 1 : p)Hm(1 : p, 1 |
|
+ |
|
|
|
|
|
|
||
: p) + (qp+1hp+1,p + rmvm,p) ep. |
||||||||||
|
|
| |
|
|
|
|
|
{z |
|
} |
|
|
|
|
|
|
|
|
r+p
In Algorithm 10.3 we have collected what we have derived so far. We have however left open in step 3 of the algorithm how the shifts µ1, . . . , µk should be chosen. In ARPACK [4], all eigenvalues of Hm are computed. Those k eigenvalues that are furthest away from some target value are chosen as shifts. We have not specified how we determine convergence, too.
One can show that a QR step with shift µi transforms the vector q1 in a multiple of (A − µiI)q1. In fact, a simple modification of the Arnoldi relation (10.2) gives
(A − µiI)Qm = Qm (|Hm {z− µiI}) +rmem = QmV1R1 + rmem. V1R1
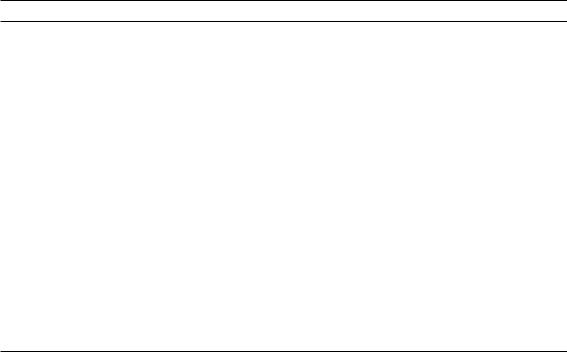
180 CHAPTER 10. RESTARTING ARNOLDI AND LANCZOS ALGORITHMS
Algorithm 10.3 Implicitely restarted Arnoldi (IRA)
1:Let the Arnoldi relation AQm = QmHm + rmem be given.
2:repeat
3:Determine k shifts µ1, . . . , µk;
4:v := em;
5:for i = 1, . . . , k do
6:Hm − µiI = ViRi; /* QR factorization */
7: |
Hm := Vi HmVi; Qm := QmVi; |
8:v := v Vi;
9:end for
10:rp := q+p+1βp+ + rmvm,p+ ;
11:Qp := Qm(:, 1 : p); Hp := Hm(1 : p, 1 : p);
12:Starting with
AQp = QpHp + rpep
execute k additional steps of the Arnoldi algorithm until
AQm = QmHm + rmem.
13: until convergence
Comparing the first columns in this equation gives
(A − µiI)q1 = q(1)1 V1e1r11 + 0 = q(1)1 r11.
By consequence, all k steps combined give
Yk
q1 ←− Ψ(A)q1, Ψ(λ) = (λ − µi).
i=1
If µi were an eigenvalue of A then (A − µiI)q1 removes components of q1 in the direction of the corresponding eigenvector. More general, if µi is close to an eigenvalue of A then (A−µiI)q1 will have only small components in the direction of eigenvectors corresponding to nearby eigenvalues. Choosing the µi equal to Ritz values far away from the desired part of the spectrum thus enhances the desired component. Still there is the danger that in each sweep on Algorithm 10.3 the same undesired Ritz values are recovered. Therefore, other strategies for choosing the shifts have been proposed [2]. Experimental results indicate however, that the original strategy chosen in ARPACK mostly works best.
10.3Convergence criterion
Let Hms = sϑ with ksk = 1. Let xˆ = Qms. Then we have as earlier
(10.4) |
kAxˆ − ϑxˆk = kAQms − QmHmsk = krmk|ems| = βm|ems|. |
In the Hermitian case, A = A , the Theorem 9.1 of Krylov–Bogoliubov provides an interval that contains an eigenvalue of A. In the general case, we have
(10.5) |
(A + E)xˆ = ϑxˆ, E = |
r |
m |
q |
, |
k |
E |
k |
= |
r |
mk |
= β |
m |
. |
|
|
− |
m |
|
|
|
k |
|
|