
- •1 Introduction
- •1.1 What makes eigenvalues interesting?
- •1.2 Example 1: The vibrating string
- •1.2.1 Problem setting
- •1.2.2 The method of separation of variables
- •1.3.3 Global functions
- •1.3.4 A numerical comparison
- •1.4 Example 2: The heat equation
- •1.5 Example 3: The wave equation
- •1.6 The 2D Laplace eigenvalue problem
- •1.6.3 A numerical example
- •1.7 Cavity resonances in particle accelerators
- •1.8 Spectral clustering
- •1.8.1 The graph Laplacian
- •1.8.2 Spectral clustering
- •1.8.3 Normalized graph Laplacians
- •1.9 Other sources of eigenvalue problems
- •Bibliography
- •2 Basics
- •2.1 Notation
- •2.2 Statement of the problem
- •2.3 Similarity transformations
- •2.4 Schur decomposition
- •2.5 The real Schur decomposition
- •2.6 Normal matrices
- •2.7 Hermitian matrices
- •2.8 Cholesky factorization
- •2.9 The singular value decomposition (SVD)
- •2.10 Projections
- •2.11 Angles between vectors and subspaces
- •Bibliography
- •3 The QR Algorithm
- •3.1 The basic QR algorithm
- •3.1.1 Numerical experiments
- •3.2 The Hessenberg QR algorithm
- •3.2.1 A numerical experiment
- •3.2.2 Complexity
- •3.3 The Householder reduction to Hessenberg form
- •3.3.2 Reduction to Hessenberg form
- •3.4 Improving the convergence of the QR algorithm
- •3.4.1 A numerical example
- •3.4.2 QR algorithm with shifts
- •3.4.3 A numerical example
- •3.5 The double shift QR algorithm
- •3.5.1 A numerical example
- •3.5.2 The complexity
- •3.6 The symmetric tridiagonal QR algorithm
- •3.6.1 Reduction to tridiagonal form
- •3.6.2 The tridiagonal QR algorithm
- •3.7 Research
- •3.8 Summary
- •Bibliography
- •4.1 The divide and conquer idea
- •4.2 Partitioning the tridiagonal matrix
- •4.3 Solving the small systems
- •4.4 Deflation
- •4.4.1 Numerical examples
- •4.6 Solving the secular equation
- •4.7 A first algorithm
- •4.7.1 A numerical example
- •4.8 The algorithm of Gu and Eisenstat
- •4.8.1 A numerical example [continued]
- •Bibliography
- •5 LAPACK and the BLAS
- •5.1 LAPACK
- •5.2 BLAS
- •5.2.1 Typical performance numbers for the BLAS
- •5.3 Blocking
- •5.4 LAPACK solvers for the symmetric eigenproblems
- •5.6 An example of a LAPACK routines
- •Bibliography
- •6 Vector iteration (power method)
- •6.1 Simple vector iteration
- •6.2 Convergence analysis
- •6.3 A numerical example
- •6.4 The symmetric case
- •6.5 Inverse vector iteration
- •6.6 The generalized eigenvalue problem
- •6.7 Computing higher eigenvalues
- •6.8 Rayleigh quotient iteration
- •6.8.1 A numerical example
- •Bibliography
- •7 Simultaneous vector or subspace iterations
- •7.1 Basic subspace iteration
- •7.2 Convergence of basic subspace iteration
- •7.3 Accelerating subspace iteration
- •7.4 Relation between subspace iteration and QR algorithm
- •7.5 Addendum
- •Bibliography
- •8 Krylov subspaces
- •8.1 Introduction
- •8.3 Polynomial representation of Krylov subspaces
- •8.4 Error bounds of Saad
- •Bibliography
- •9 Arnoldi and Lanczos algorithms
- •9.2 Arnoldi algorithm with explicit restarts
- •9.3 The Lanczos basis
- •9.4 The Lanczos process as an iterative method
- •9.5 An error analysis of the unmodified Lanczos algorithm
- •9.6 Partial reorthogonalization
- •9.7 Block Lanczos
- •9.8 External selective reorthogonalization
- •Bibliography
- •10 Restarting Arnoldi and Lanczos algorithms
- •10.2 Implicit restart
- •10.3 Convergence criterion
- •10.4 The generalized eigenvalue problem
- •10.5 A numerical example
- •10.6 Another numerical example
- •10.7 The Lanczos algorithm with thick restarts
- •10.8 Krylov–Schur algorithm
- •10.9 The rational Krylov space method
- •Bibliography
- •11 The Jacobi-Davidson Method
- •11.1 The Davidson algorithm
- •11.2 The Jacobi orthogonal component correction
- •11.2.1 Restarts
- •11.2.2 The computation of several eigenvalues
- •11.2.3 Spectral shifts
- •11.3 The generalized Hermitian eigenvalue problem
- •11.4 A numerical example
- •11.6 Harmonic Ritz values and vectors
- •11.7 Refined Ritz vectors
- •11.8 The generalized Schur decomposition
- •11.9.1 Restart
- •11.9.3 Algorithm
- •Bibliography
- •12 Rayleigh quotient and trace minimization
- •12.1 Introduction
- •12.2 The method of steepest descent
- •12.3 The conjugate gradient algorithm
- •12.4 Locally optimal PCG (LOPCG)
- •12.5 The block Rayleigh quotient minimization algorithm (BRQMIN)
- •12.7 A numerical example
- •12.8 Trace minimization
- •Bibliography
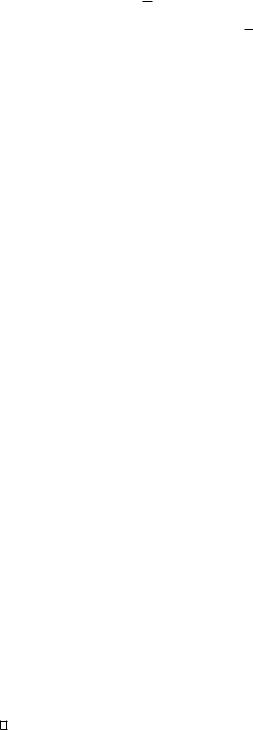
2.7. HERMITIAN MATRICES |
37 |
2.7Hermitian matrices
Definition 2.13 A matrix A Fn×n is Hermitian if
(2.22) |
A = A . |
The Schur decomposition for Hermitian matrices is particularly simple. We first note that A being Hermitian implies that the upper triangular Λ in the Schur decomposition A = UΛU is Hermitian and thus diagonal. In fact, because
Λ = Λ = (U AU) = U A U = U AU = Λ,
each diagonal element λi of Λ satisfies λi = λi. So, Λ has to be real. In summary have the following result.
Theorem 2.14 (Spectral theorem for Hermitian matrices) Let A be Hermitian. Then there is a unitary matrix U and a real diagonal matrix Λ such that
|
n |
|
Xi |
(2.23) |
A = UΛU = λiuiui . |
|
=1 |
The columns u1, . . . , un of U are eigenvectors corresponding to the eigenvalues λ1, . . . , λn. They form an orthonormal basis for Fn.
The decomposition (2.23) is called a spectral decomposition of A.
As the eigenvalues are real we can sort them with respect to their magnitude. We can, e.g., arrange them in ascending order such that λ1 ≤ λ2 ≤ · · · ≤ λn.
If λi = λj , then any nonzero linear combination of ui and uj is an eigenvector corresponding to λi,
A(uiα + uj β) = uiλiα + uj λj β = (uiα + uj β)λi.
However, eigenvectors corresponding to di erent eigenvalues are orthogonal. Let Au = uλ and Av = vµ, λ 6= µ. Then
λu v = (u A)v = u (Av) = u vµ,
and thus
(λ − µ)u v = 0,
from which we deduce u v = 0 as λ 6= µ.
In summary, the eigenvectors corresponding to a particular eigenvalue λ form a subspace, the eigenspace {x Fn, Ax = λx} = N (A − λI). They are perpendicular to the eigenvectors corresponding to all the other eigenvalues. Therefore, the spectral decomposition (2.23) is unique up to ± signs if all the eigenvalues of A are distinct. In case of multiple eigenvalues, we are free to choose any orthonormal basis for the corresponding eigenspace.
Remark 2.4. The notion of Hermitian or symmetric has a wider background. Let hx, yi be an inner product on Fn. Then a matrix A is symmetric with respect to this inner product if hAx, yi = hx, Ayi for all vectors x and y. For the ordinary Euclidean inner product (x, y) = x y we arrive at the element-wise Definition 2.7 if we set x and y equal to coordinate vectors.
It is important to note that all the properties of Hermitian matrices that we will derive subsequently hold similarly for matrices symmetric with respect to a certain inner product.
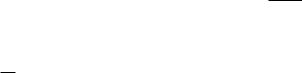
38 CHAPTER 2. BASICS
Example 2.15 We consider the one-dimensional Sturm-Liouville eigenvalue problem
(2.24) − u′′(x) = λu(x), 0 < x < π, u(0) = u(π) = 0,
that models the vibration of a homogeneous string of length π that is clamped at both ends. The eigenvalues and eigenvectors or eigenfunctions of (2.24) are
λk = k2, uk(x) = sin kx, k N.
Let u(in) denote the approximation of an (eigen)function u at the grid point xi,
π
ui ≈ u(xi), xi = ih, 0 ≤ i ≤ n + 1, h = n + 1.
We approximate the second derivative of u at the interior grid points by
(2.25) |
1 |
(−ui−1 |
+ 2ui − ui+1) = λui, 1 |
≤ i ≤ n. |
h2 |
Collecting these equations and taking into account the boundary conditions, u0 = 0 and un+1 = 0, we get a (matrix) eigenvalue problem
(2.26) |
|
|
Tnx = λx |
|
|
|
|
|
|
|
|
|
where |
|
−1 |
−2 |
−1 |
|
|
|
|
|
|
|
|
|
|
2 |
1 |
|
|
|
|
|
|
|
|
|
Tn := |
(n + 1)2 |
|
−1 |
. 2 −. |
1 . |
|
|
|
|
|
|
Rn×n. |
2 |
.. |
|
|
|||||||||
|
π |
|
|
.. .. |
|
|
|
|
||||
|
|
|
|
|
1 2 |
|
1 |
|
|
|
||
|
|
|
|
|
|
|
|
|
||||
|
|
|
|
|
|
|
|
|
|
|
|
|
|
|
|
|
− |
|
|
1 |
− |
2 |
|
|
|
|
|
|
|
|
− |
|
|
|
|
|||
|
|
|
|
|
|
|
|
|
|
|
The matrix eigenvalue problem (2.26) can be solved explicitly [3, p.229]. Eigenvalues and eigenvectors are given by
(n) |
|
(n + 1)2 |
|
4(n + 1)2 |
|
kπ |
|
|
|
||||
λk |
= |
|
|
|
(2 − 2 cos φk) = |
|
sin2 |
|
, |
|
|
||
|
π2 |
|
π2 |
2(n + 1) |
|
|
|||||||
(2.27) |
|
2 |
|
|
1/2 |
|
|
|
|
|
kπ |
||
(n) |
|
|
|
|
|
|
|
|
|||||
|
|
|
|
|
|
|
|
|
|||||
uk |
= |
|
|
[sin φk, sin 2φk, . . . , sin nφk]T , |
φk = |
|
. |
||||||
n + 1 |
n + 1 |
Clearly, λ(kn) converges to λk as n → ∞. (Note that sin ξ → ξ as ξ → 0.) When we identify
u(kn) with the piecewise linear function that takes on the values given by u(kn) at the grid points xi then this function evidently converges to sin kx.
Let p(λ) be a polynomial of degree d, p(λ) = α0 + α1λ + α2λ2 + · · · + αdλd. As
Aj = (UΛU )j = UΛj U we can define a matrix polynomial as |
U . |
|||||||
(2.28) |
p(A) = |
d |
αj Aj = |
d |
αj UΛj U = U |
d |
αj Λj |
|
|
|
X |
|
X |
|
X |
|
|
|
|
j=0 |
|
j=0 |
|
j=0 |
|
|
This equation shows that p(A) has the same eigenvectors as the original matrix A. The eigenvalues are modified though, λk becomes p(λk). Similarly, more complicated functions of A can be computed if the function is defined on spectrum of A.

2.7. HERMITIAN MATRICES |
|
|
39 |
Definition 2.16 The quotient |
|
|
|
ρ(x) := |
x Ax |
, |
x 6= 0, |
x x |
is called the Rayleigh quotient of A at x.
Notice, that ρ(xα) = ρ(x), α 6= 0. Hence, the properties of the Rayleigh quotient can be investigated by just considering its values on the unit sphere. Using the spectral decomposition A = UΛU , we get
|
|
|
|
|
|
|
|
n |
|
|
|
|
|
|
|
|
|
|
Xi |
|
|
|
|
|
x Ax = x UΛU x = λi|ui x|2. |
|||||||
P |
|
| |
n| |
|
|
|
|
=1 |
|
|
i=1 |
|
|
n |
|
≤ · · · ≤ |
n |
|
|||
|
|
i |
|
|
≤ |
|
|
|
||
Similarly, x x = n |
|
u x |
2 |
. With λ1 |
|
λ2 |
|
λn, we have |
||
|
|
|
X |
X |
|
|
Xi |
|ui x|2. |
||
|
|
λ1 |
|
|ui x|2 ≤ |
|
λi|ui x|2 ≤ λn |
||||
|
|
|
i=1 |
i=1 |
|
|
=1 |
|
||
So, |
|
|
|
|
|
|
|
|
|
|
|
|
|
λ1 ≤ ρ(x) ≤ λn, |
for all x 6= 0. |
As
ρ(uk) = λk,
the extremal values λ1 and λn are actually attained for x = u1 and x = un, respectively. Thus we have proved the following theorem.
Theorem 2.17 Let A be Hermitian. Then the Rayleigh quotient satisfies
(2.29) |
λ1 = min ρ(x), |
λn = max ρ(x). |
As the Rayleigh quotient is a continuous function it attains all values in the closed interval [λ1, λn].
The next theorem generalizes the above theorem to interior eigenvalues. The following theorems is attributed to Poincar´e, Fischer and P´olya.
Theorem 2.18 (Minimum-maximum principle) Let A be Hermitian. Then
(2.30) |
λp = |
min |
max ρ(Xx) |
|
|
X Fn×p,rank(X)=p x6=0 |
Proof. Let Up−1 = [u1, . . . , up−1]. For every Xnwith full rank we can choose x 6= 0 such |
|||
that U |
Xx = 0. Then 0 = z := Xx = |
i=p |
ziui. As in the proof of the previous |
p 1 |
6 |
|
|
theorem−we obtain the inequality |
P |
|
ρ(z) ≥ λp.
To prove that equality holds in (2.30) we choose X = [u1, . . . , up]. Then
− |
|
1 |
|
0 |
|
|
|
|
|
1 0 |
|
||
Up |
1Xx = |
|
... |
. |
|
x = 0 |
|
|
|
|
|
|
|
implies that x = ep, i.e., that z = Xx = up. So, ρ(z) = λp.
An important consequence of the minimum-maximum principle is the following

40 CHAPTER 2. BASICS
Theorem 2.19 (Monotonicity principle) Let q1, . . . , qp be normalized, mutually orthogonal vectors and Q := [q1, . . . , qp]. Let A′ := Q AQ Fp×p. Then the p eigenvalues
λ1′ ≤ · · · ≤ λp′ |
of A′ satisfy |
|
|
|
|
|
|
|
|
(2.31) |
λk ≤ λk′ , |
|
1 ≤ k ≤ p. |
|
|||||
Proof. Let w1, . . . , wp Fp be the eigenvectors of A′, |
|
|
|||||||
(2.32) |
A′w |
i |
= λ′ w |
, |
1 |
≤ |
i |
≤ |
p, |
|
|
i i |
|
|
|
|
with w wj = δij . Then the vectors Qw1, . . . , Qwp are normalized and mutually orthogonal. Therefore, we can construct a vector
|
|
|
x0 := a1Qw1 + · · · + akQwk, kx0k = 1, |
||||||||||||
that is orthogonal to the first k − 1 eigenvectors of A, |
|
|
|
||||||||||||
|
|
|
x x |
i |
= 0, |
|
1 |
≤ |
i |
≤ |
k |
− |
1. |
||
|
|
|
0 |
|
|
|
|
|
|
|
|||||
Then, with the minimum-maximum principle we have |
|
|
|
||||||||||||
λ |
k |
= |
min |
|
R(x) |
≤ |
R(x |
|
) = x Ax |
0 |
|||||
|
|
X=6 0 |
|
|
|
0 |
|
|
|
0 |
|
||||
|
|
|
X X1=···=X Xk−1=0 |
|
|
|
|
|
|
|
|
|
|
|
|
|
|
|
p |
|
|
|
k |
|
|
|
|
|
|
|
k |
|
|
= |
a¯iaj wi Q AQwj = |
i,j=1 |
a¯iaj λi′ δij = |a|i2λi′ ≤ λk′ . |
||||||||||
|
|
|
i,j=1 |
|
|
|
|
|
|
|
|
|
i=1 |
||
|
|
|
X |
|
|
|
X |
|
|
|
|
|
|
X |
|
The last inequality holds since kx0k = 1 implies |
|
|
ik=1|a|i2 = 1. |
||||||||||||
Remark 2.5. (The proof of this statement is an |
exercise) |
|
|
||||||||||||
|
P |
|
|
|
|
It is possible to prove the inequalities (2.31) without assuming that the q1, . . . , qp are orthonormal. But then one has to use the eigenvalues λ′k of
A′x = λ′Bx, B′ = Q Q,
instead of (2.32).
The trace of a matrix A Fn×n is defined to be the sum of the diagonal elements of a matrix. Matrices that are similar have equal trace. Hence, by the spectral theorem,
|
n |
n |
|
Xi |
X |
(2.33) |
trace(A) = |
aii = λi. |
|
=1 |
i=1 |
The following theorem is proved in a similar way as the minimum-maximum theorem.
Theorem 2.20 (Trace theorem)
(2.34) |
λ |
1 |
+ λ |
2 |
+ |
· · · |
+ λ |
p |
= |
min |
trace(X AX) |
|
|
|
|
|
|
X Fn×p,X X=Ip |
|
2.8Cholesky factorization
Definition 2.21 A Hermitian matrix is called positive definite (positive semi-definite) if all its eigenvalues are positive (nonnegative).

2.8. CHOLESKY FACTORIZATION |
41 |
For a Hermitian positive definite matrix A, the LU decomposition can be written in a particular form reflecting the symmetry of A.
Theorem 2.22 (Cholesky factorization) Let A Fn×n be Hermitian positive definite. Then there is a lower triangular matrix L such that
(2.35) |
A = LL . |
L is unique if we choose its diagonal elements to be positive.
Proof. We prove the theorem by giving an algorithm that computes the desired factorization.
Since A is positive definite, we have a11 = e1Ae1 |
> 0. Therefore we can form the |
|||||||||||||||||||||||||
matrix |
|
|
|
|
|
|
|
|
|
|
|
|
|
|
|
|
|
|
|
|
|
|
|
|
|
|
|
|
|
|
(1) |
|
|
|
|
|
|
|
a21 |
1 |
|
|
|
|
|
|
|||||||
|
|
|
|
|
|
|
|
|
|
|
|
|
|
|
|
|
|
|
||||||||
|
|
|
|
|
1 |
|
|
|
|
|
√a1,1 |
|
|
|
|
|
|
|||||||||
|
|
l21 |
|
|
|
|
|
|
|
|
|
|
|
|
|
|
|
|||||||||
|
|
|
l(1) |
|
|
|
|
|
|
|
|
|
√a11 |
|
|
|
|
|
|
|
|
|||||
|
|
|
|
11 |
|
|
|
|
|
|
|
|
.. |
|
|
|
|
|
|
|
. |
|
|
|||
L1 |
= .. |
|
|
|
.. |
. |
|
= |
|
|
|
|
... |
|
|
|
||||||||||
|
|
. |
|
|
|
|
|
|
. |
|
|
|
|
|
|
|
|
|
|
|||||||
|
|
l |
|
|
|
|
|
|
1 |
|
|
|
|
|
|
|
|
|
|
|
|
1 |
|
|
|
|
|
|
|
|
|
|
|
|
|
|
|
|
|
|
|
|
|
|
|
|
|||||||
|
|
n1 |
|
|
|
|
|
|
√a1,1 |
|
|
|
|
|
|
|||||||||||
|
|
|
|
|
|
|
|
|
|
|
|
|
|
|
|
|
|
|
|
|
||||||
|
|
|
|
|
|
|
|
|
|
|
|
|
|
an1 |
|
|
|
|
|
|
|
|
||||
|
|
|
|
(1) |
|
|
|
|
|
|
|
|
|
|
|
|
|
|
|
|
|
|||||
We now form the matrix |
|
|
|
|
0 a22 − |
|
|
|
|
|
|
|
|
|
− |
|
|
|
|
|
||||||
|
|
1 |
|
|
1 |
|
0 |
a11 |
|
|
|
. . . a2n |
0 |
a11 |
|
|
||||||||||
|
1 |
|
|
1 |
|
|
|
1 |
|
|
|
|
|
|
|
. . . |
|
|
|
|
|
|
||||
|
|
|
|
|
.. |
|
|
|
.. |
|
|
|
|
... |
|
|
.. |
|
|
|
||||||
|
|
|
|
|
|
|
|
0 a |
|
|
a21a12 |
|
|
|
|
|
a21a1n |
|
|
|||||||
A1 = L− AL− |
|
= |
|
|
an1a12 . . . a |
|
|
an1a1n |
. |
|||||||||||||||||
|
|
. |
|
|
|
. |
|
|
|
|
|
|
|
|
|
. |
|
|
|
|||||||
|
|
|
|
|
|
|
|
|
|
n2 |
|
|
a11 |
|
|
|
|
|
|
nn |
|
|
a11 |
|
|
|
This is the first step of the algorithm. Since positive definiteness is preserved by a congruence transformation X AX (see also Theorem 2.24 below), A1 is again positive definite. Hence, we can proceed in a similar fashion factorizing A1(2:n, 2:n), etc.
Collecting L1, L2, . . . , we obtain
I = L−n 1 · · · L−2 1L−1 1A(L1)−1(L2)−1 · · · (Ln)−1
or
(L1L2 · · · Ln)(Ln · · · L2L1) = A.
which is the desired result. It is easy to see that L1L2 · · · Ln is a triangular matrix and that
· · · |
|
l11(1) |
|
|
|
|
|
|
|
|
|
|
|
|
|||
|
l21(1) |
l22(2) |
|
|
|
|
||
|
.. .. .. .. |
. |
|
|
||||
L1L2 Ln = |
. . . |
|
|
|||||
l31(1) |
l32(2) |
l33(3) |
|
lnn |
|
|||
|
l |
|
l |
l . . . |
|
|||
|
|
|
|
|
|
|
|
|
|
|
|
(1) |
(2) |
(3) |
|
(n) |
|
|
|
|
n1 |
n2 |
n3 |
|
|
|
Remark 2.6. When working with symmetric matrices, one often stores only half of the matrix, e.g. the lower triangle consisting of all elements including and below the diagonal. The L-factor of the Cholesky factorization can overwrite this information in-place to save memory.
Definition 2.23 The inertia of a Hermitian matrix is the triple (ν, ζ, π) where ν, ζ, π is the number of negative, zero, and positive eigenvalues.