
Young D.C. - Computational chemistry (2001)(en)
.pdf242 29 FORCE FIELD CUSTOMIZATION
U. Burkert, N. L. Allinger, Molecular Mechanics American Chemical Society, Washington (1982).
Some journal articles with general discussions are
M. Zimmer, Chem. Rev. 95, 2629 (1995).
B. P. Hay, Coord. Chem. Rev. 126, 177 (1993).
J. P. Bowen, N. L. Allinger, Rev. Comput. Chem. 2, 81 (1991).
J. R. Maple, U. Dinur, A. T. Hagler, Proc. Natl. Acad. Sci. USA 85, 5350 (1988). A. J. Hop®nger, R. A. Pearlstein, J. Comput. Chem. 5, 486 (1984).
A comprehensive listing of all published force ®eld parameters is
M. Jalaie, K. B. Lipkowitz, Rev. Comput. Chem. 14, 441 (2000).
E. Osawa, K. B. Lipkowitz, Rev. Comput. Chem. 6, 355 (1995).
Computational Chemistry: A Practical Guide for Applying Techniques to Real-World Problems. David C. Young Copyright ( 2001 John Wiley & Sons, Inc.
ISBNs: 0-471-33368-9 (Hardback); 0-471-22065-5 (Electronic)
30 Structure±Property Relationships
Structure±property relationships are qualitative or quantitative empirically de®ned relationships between molecular structure and observed properties. In some cases, this may seem to duplicate statistical mechanical or quantum mechanical results. However, structure-property relationships need not be based on any rigorous theoretical principles.
The simplest case of structure-property relationships are qualitative rules of thumb. For example, the statement that branched polymers are generally more biodegradable than straight-chain polymers is a qualitative structure±property relationship.
When structure-property relationships are mentioned in the current literature, it usually implies a quantitative mathematical relationship. Such relationships are most often derived by using curve-®tting software to ®nd the linear combination of molecular properties that best predicts the property for a set of known compounds. This prediction equation can be used for either the interpolation or extrapolation of test set results. Interpolation is usually more accurate than extrapolation.
When the property being described is a physical property, such as the boiling point, this is referred to as a quantitative structure±property relationship (QSPR). When the property being described is a type of biological activity, such as drug activity, this is referred to as a quantitative structure±activity relationship (QSAR). Our discussion will ®rst address QSPR. All the points covered in the QSPR section are also applicable to QSAR, which is discussed next.
30.1QSPR
The ®rst step in developing a QSPR equation is to compile a list of compounds for which the experimentally determined property is known. Ideally, this list should be very large. Often, thousands of compounds are used in a QSPR study. If there are fewer compounds on the list than parameters to be ®tted in the equation, then the curve ®t will fail. If the same number exists for both, then an exact ®t will be obtained. This exact ®t is misleading because it ®ts the equation to all the anomalies in the data, it does not necessarily re¯ect all the correct trends necessary for a predictive method. In order to ensure that the method will be predictive, there should ideally be 10 times as many test compounds as ®tted parameters. The choice of compounds is also important. For
243
244 30 STRUCTURE±PROPERTY RELATIONSHIPS
example, if the equation is only ®tted with hydrocarbon data, it will only be reliable for predicting hydrocarbon properties.
The next step is to obtain geometries for the molecules. Crystal structure geometries can be used; however, it is better to use theoretically optimized geometries. By using the theoretical geometries, any systematic errors in the computation will cancel out. Furthermore, the method will predict as yet unsynthesized compounds using theoretical geometries. Some of the simpler methods require connectivity only.
Molecular descriptors must then be computed. Any numerical value that describes the molecule could be used. Many descriptors are obtained from molecular mechanics or semiempirical calculations. Energies, population analysis, and vibrational frequency analysis with its associated thermodynamic quantities are often obtained this way. Ab initio results can be used reliably, but are often avoided due to the large amount of computation necessary. The largest percentage of descriptors are easily determined values, such as molecular weights, topological indexes, moments of inertia, and so on. Table 30.1 lists some of the descriptors that have been found to be useful in previous studies. These are discussed in more detail in the review articles listed in the bibliography.
Once the descriptors have been computed, is necessary to decide which ones will be used. This is usually done by computing correlation coe½cients. Correlation coe½cients are a measure of how closely two values (descriptor and property) are related to one another by a linear relationship. If a descriptor has a correlation coe½cient of 1, it describes the property exactly. A correlation coe½cient of zero means the descriptor has no relevance. The descriptors with the largest correlation coe½cients are used in the curve ®t to create a property prediction equation. There is no rigorous way to determine how large a correlation coe½cient is acceptable.
Intercorrelation coe½cients are then computed. These tell when one descriptor is redundant with another. Using redundant descriptors increases the amount of ®tting work to be done, does not improve the results, and results in unstable ®tting calculations that can fail completely (due to dividing by zero or some other mathematical error). Usually, the descriptor with the lowest correlation coe½cient is discarded from a pair of redundant descriptors.
A curve ®t is then done to create a linear equation, such as
Property ˆ c0 ‡ c1d1 ‡ c2d2 ‡ |
…30:1† |
where ci are the ®tted parameters and di the descriptors. Most often, the equation being ®tted is a linear equation like the one above. This is because the use of correlation coe½cients and linear equations together is an easily automated process. Introductory descriptions cite linear regression as the algorithm for determining coe½cients of best ®t, but the mathematically equivalent matrix leastsquares method is actually more e½cient and easier to implement. Occasionally, a nonlinear parameter, such as the square root or log of a quantity, is used. This is done when a researcher is aware of such nonlinear relationships in advance.

30.1 QSPR 245
TABLE 30.1 Common Molecular Descriptors
Constitutional Descriptors
Molecular weight
Number of atoms of various elements
Number of bonds of various orders
Number of rings
Topological Descriptors
Weiner index
Randic indices
Kier and Hall indices
Information content
Connectivity index
Balaban index
Electrostatic Descriptors
Partial charges
Polarity indices
Topological electronic index
Multipoles
Charged partial surface areas
Polarizability
Anisotropy of polarizability
Geometrical Descriptors
Moments of inertia
Molecular volume
Molecular surface areas
Shadow indices
Taft steric constant
Length, width, and height parameters
Shape factor
Quantum Chemical Descriptors
Net atomic charges
Bond orders
HOMO and LUMO energies
FMO reactivity indices
Refractivity
Total energy
Ionization potential
Electron a½nity
Energy of protonation
Orbital populations
Frontier orbital densities
Superdelocalizabilities
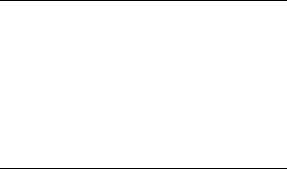
246 30 STRUCTURE±PROPERTY RELATIONSHIPS
TABLE 30.1 (Continued)
Quantum Chemical Descriptors
Sum of the squared atomic charge densities
Sum of the absolute values of charges
Absolute hardness
Statistical Mechanical Descriptors
Vibrational frequencies
Rotational enthalpy and entropy
Vibrational enthalpy and entropy
Translational enthalpy and entropy
The process described in the preceding paragraphs has seen widespread use. This is partly because it has been automated very well in the more sophisticated QSPR programs.
It is possible to use nonlinear curve ®tting (i.e., exponents of best ®t). Nonlinear ®tting is done by using a steepest-descent algorithm to minimize the deviation between the ®tted and correct values. The drawback is possibly falling into a local minima, thus necessitating the use of global optimization algorithms. Automated algorithms for determining which descriptors to include in a nonlinear ®t are possible, but there is not yet a consensus as to what technique is best. This approach can yield a closer ®t to the data than multiple linear techniques. However, it is less often used due to the large amount of manual trial-and-error work necessary. Automated nonlinear ®tting algorithms are expected to be included in future versions of QSPR software packages.
The validation of the prediction equation is its performance in predicting properties of molecules that were not included in the parameterization set. Equations that do well on the parameterization set may perform poorly for other molecules for several di¨erent reasons. One mistake is using a limited selection of molecules in the parameterization set. For example, an equation parameterized with organic molecules may perform very poorly when predicting the properties of inorganic molecules. Another mistake is having nearly as many ®tted parameters as molecules in the test set, thus ®tting to anomalies in the data rather than physical trends.
The development of group additivity methods is very similar to the development of a QSPR method. Group additivity methods can be useful for properties that are additive by nature, such as the molecular volume. For most properties, QSPR is superior to group additivity techniques.
Other algorithms for predicting properties have been developed. Both neural network and genetic algorithm-based programs are available. Some arguments can be made for the use of each. However, none has yet seen widespread use. This may be partially due to the greater di½culty in interpreting the chemical information that can be gained in addition to numerical predictions. Neural
30.3 3D QSAR |
247 |
networks are generally known to provide a good interpolation of data, but rather poor extrapolation.
30.2QSAR
QSAR is also called traditional QSAR or Hansch QSAR to distinguish it from the 3D QSAR method described below. This is the application of the technique described above to biological activities, such as environmental toxicology or drug activity. The discussion above is applicable but a number of other caveats apply; which are addressed in this section. The following discussion is oriented toward drug design, although the same points may be applicable to other areas of research as well.
In order to parameterize a QSAR equation, a quanti®ed activity for a set of compounds must be known. These are called lead compounds, at least in the pharmaceutical industry. Typically, test results are available for only a small number of compounds. Because of this, it can be di½cult to choose a number of descriptors that will give useful results without ®tting to anomalies in the test set. Three to ®ve lead compounds per descriptor in the QSAR equation are normally considered an adequate number. If two descriptors are nearly collinear with one another, then one should be omitted even though it may have a large correlation coe½cient.
In the case of drug design, it may be desirable to use parabolic functions in place of linear functions. The descriptor for an ideal drug candidate often has an optimum value. Drug activity will decrease when the value is either larger or smaller than optimum. This functional form is described by a parabola, not a linear relationship.
The advantage of using QSAR over other modeling techniques is that it takes into account the full complexity of the biological system without requiring any information about the binding site. The disadvantage is that the method will not distinguish between the contribution of binding and transport properties in determining drug activity. QSAR is very useful for determining general criteria for activity, but it does not readily yield detailed structural predictions.
30.33D QSAR
For drug design purposes, it is desirable to construct a method that will predict the molecular structures of candidate compounds without requiring knowledge of the binding-site geometry. 3D QSAR has been fairly successful in ful®lling these criteria. It is similar to QSAR in that property descriptors, statistical analysis, and ®tting techniques are used. Beyond that, the two computations are signi®cantly di¨erent.
Like QSAR, molecular structures must be available for compounds that
248 30 STRUCTURE±PROPERTY RELATIONSHIPS
have known quantitatively de®ned activities. The ®rst step is then to align the molecular structures. This alignment is based on the fact that all have a drug activity due to docking at a particular site. Alignment algorithms rotate and translate a molecule within the Cartesian coordinate space until it matches the location and rotation of another molecule as well as possible. This can be as simple as aligning the backbones of similar molecules or as complex as a sophisticated search and optimization scheme. For conformationally ¯exible compounds, both alignment and conformation must be addressed. Typically, the most rigid molecule in the set is the one to which the others are aligned. There are automated routines for ®nding the conformer of best alignment, or this can be done manually.
Once the molecules are aligned, a molecular ®eld is computed on a grid of points in space around the molecule. This ®eld must provide a description of how each molecule will tend to bind in the active site. Field descriptors typically consist of a sum of one or more spatial properties, such as steric factors, van der Waals parameters, or the electrostatic potential. The choice of grid points will also a¨ect the quality of the ®nal results.
The ®eld points must then be ®tted to predict the activity. There are generally far more ®eld points than known compound activities to be ®tted. The least-squares algorithms used in QSAR studies do not function for such an underdetermined system. A partial least squares (PLS) algorithm is used for this type of ®tting. This method starts with matrices of ®eld data and activity data. These matrices are then used to derive two new matrices containing a description of the system and the residual noise in the data. Earlier studies used a similar technique, called principal component analysis (PCA). PLS is generally considered to be superior.
The model obtained from the PLS algorithm gives two pieces of information on various regions of space. The ®rst is how well the activity correlates to that region in space. The second is whether the functional group at that point should be electron-donating, electron-withdrawing, bulky, and so forth according to the choice of ®eld parameters. This site description is called a pharmacophore in drug design work.
An examination of the plotted data reveals signi®cant structural information, such as the fact that an electron-donating group should be a certain distance from a withdrawing group, and so on. Further examination of relative magnitudes can give an indication as to precisely which group might be best. Unknown compounds may then be run through the same analysis to obtain a quantitative prediction of their drug activities.
Ideally, the results should be validated somehow. One of the best methods for doing this is to make predictions for compounds known to be active that were not included in the training set. It is also desirable to eliminate compounds that are statistical outliers in the training set. Unfortunately, some studies, such as drug activity prediction, may not have enough known active compounds to make this step feasible. In this case, the estimated error in prediction should be increased accordingly.
BIBLIOGRAPHY 249
30.4COMPARATIVE QSAR
Comparative QSAR is a ®eld currently under development by several groups. Large databases of known QSAR and 3D QSAR results have been compiled. Such a database can be used for more than simply obtaining literature citations. The analysis of multiple results for the same or similar systems can yield a general understanding of the related chemistry as well as providing a good comparison of techniques.
30.5RECOMMENDATIONS
Floppy molecules present some additional di½culty in applying QSAR/QSPR. They are also much more di½cult to work with in 3D QSAR. With QSAR/ QSPR, this problem can be avoided by using only descriptors that do not depend on the conformation, but the accuracy of results may su¨er. For more accurate QSPR, the lowest-energy conformation is usually what should be used. For QSAR or 3D QSAR, the conformation most closely matching a rigid molecule in the test set should be used. If all the molecules are ¯oppy, ®nding the lowest-energy conformer for all and looking for some commonality in the majority might be the best option.
QSPR and QSAR are useful techniques for predicting properties that would be very di½cult to predict by any other method. This is a somewhat empirical or indirect calculation that ultimately limits the accuracy and amount of information which can be obtained. When other means of computational prediction are not available, these techniques are recommended for use. There are a variety of algorithms in use that are not equivalent. An examination of published results and tests of several techniques are recommended.
BIBLIOGRAPHY
Introductory descriptions are in
A.K. RappeÂ, C. J. Casewit, Molecular Mechanics across Chemistry University Science Books, Sausalito (1997).
A.R. Leach Molecular Modelling Principles and Applications Longman, Essex (1996).
G.H. Grant, W. G. Richards, Computational Chemistry Oxford, Oxford (1995).
Books about QSAR/QSPR are
L.B. Kier, L. H. Hall, Molecular Structure Description: The Electrotopological State
Academic Press, San Diego (1999).
Topological Indices and Related Descriptors in QSAR and QSPR J. Devillers, A. T. Balaban, Eds., Gordon and Breach, Reading (1999).
3D QSAR in Drug Design H. Kubinyi, Y. C. Martin, G. Folker, Eds., Kluwer, Norwell MA (1998). (3 volumes)
250 30 STRUCTURE±PROPERTY RELATIONSHIPS
J.Devillers, Neural Networks in QSAR and Drug Design Academic Press, San Diego (1996).
C. Hansch, A. Leo, Exploring QSAR American Chemical Society, Washington (1995). L. B. Kier, L. H. Hall, Molecular Connectivity in Structure-Activity Analysis Research
Studies Press, Chichester (1986).
L. B. Kier, L. H. Hall, Molecular Connectivity in Chemistry and Drug Research Academic Press, San Diego (1976).
Review articles are
D. Ivanciuc, Encycl. Comput. Chem. 1, 167 (1998).
V. Venkatasubramanian, a. Sundaram, Encycl. Comput. Chem. 2, 1115 (1998). G. Jones, Encycl. Comput. Chem. 2, 1127 (1998).
D. Ivanciuc, A. T. Balaban, Encycl. Comput. Chem. 2, 1169 (1998). J. Shorter, Encycl. Comput. Chem. 4, 1487 (1998).
P. C. Jurs, Encycl. Comput. Chem. 1, 2320 (1998).
M. Randic, Encycl. Comput. Chem. 5, 3018 (1998).
S.Profeta, Jr., Kirk-Othmer Encyclopedia of Chemical Technology Supplement J. I. Kroschwitz (Ed.) 315, John Wiley & Sons, New York (1998).
G. A. Arteca, Rev. Comput. Chem. 9, 191 (1996).
M. Karelson, V. S. Lobanov, A. R. Katritzky, Chem. Rev. 96, 1027 (1996).
A.R. Katritzky, V. S. Lobanov, M. Karelson, Chem. Soc. Rev. 24, 279 (1995).
B.W. Clare, Theor. Chim. Acta 87, 415 (1994).
L. H. Hall, L. B. Kerr, Rev. Comput. Chem. 2, 367 (1991).
I. B. Bersuker, A. S. Dimoglo, Rev. Comput. Chem. 2, 423 (1991). S. P. Gupta, Chem. Rev. 87, 1183 (1987).
3D QSAR reviews are
H. Kubinyi, Encycl. Comput. Chem. 1, 448 (1998).
T. I. Oprea, C. L. Waller, Rev. Comput. Chem. 11, 127 (1997).
G.Greco, E. Novellino, Y. C. Martin, Rev. Comput. Chem. 11, 183 (1997).
Comparative QSAR reviews are
H.Gao, J. A. Katzenellenbogen, R. Garg, C. Hansch, Chem. Rev. 99, 723 (1999).
C.Hansch, G. Gao, Chem. Rev. 97, 2995 (1997).
C.Hansch, D. Hoekmen, H. Gao, Chem. Rev. 96, 1045 (1996).
Many resources are listed at the web site of The QSAR and Modelling Society
http://www.pharma.ethz.ch/qsar
QSAR applications in various ®elds
J. Devillers, Encycl. Comput. Chem. 2, 930 (1998).
H. Kubinyi, Encycl. Comput. Chem. 4, 2309 (1998).
BIBLIOGRAPHY 251
F. Leclerc, R. Cedergren, Encycl. Comput. Chem. 4, 2756 (1998).
QSAR in Environmental Toxicology-IV Elsevier, Amsterdam (1991).
Practical Applications of Quantitative Structure-Activity Relationships (QSAR) in Environmental Chemistry and Toxicology W. Karcher, J. Devillers, Eds., Kluwer, Dordrecht (1990).
QSAR in Environmental Toxicology K. L. E. Kaiser, Ed., D. Reidel Publishing, Dordrecht (1989).
QSAR in Environmental Toxicology-II D. Reidel Publishing, Dordrecht (1987).
QSAR in Drug Design and Toxicology D. Hadzi, B. Jerman-BlazÏicÏ, Eds., Elsevier, Amsterdam (1987).
QSAR and Strategies in the Design of Bioactive Compounds J. K. Seydel, Ed., VCH, Weinheim (1985).
An article listing many descriptors is
M. Cocchi, M. C. Menziani, F. Fanelli, P. G. de Benedetti, J. Mol. Struct. (Theochem) 331, 79 (1995).