
Solid-Phase Synthesis and Combinatorial Technologies
.pdf5.2 FOCUSED LIBRARIES 173
information on the library components, compensating the synthetic efforts and the satisfactory analytical characterization (when). The library was prepared as discretes and was composed of 18 compounds (how). The synthetic scheme was composed of several SP steps with different complexities, and both noncommercial (A, B) and commercial (C) small monomer sets were used (what). Neither the time and effort required nor the chemicals used for the focused library synthesis represented a burdensome investment (how much).
5.2.3 Synthesis and Characterization
After having assessed the planned synthetic route on a single standard (when the focused library will be prepared in solution, this step is not necessary), the combinatorialization process takes place. This process is significantly less demanding than for primary libraries because of the reduced diversity of the monomers composing the sets, which typically show similar reactivity. It is therefore easier to find optimal reaction conditions, meaning the monomer rehearsal should be faster and produce only a few, if any, rejected monomers for the focused library synthesis. The high purity requirements for the focused library individuals will demand a meticulous characterization of any side reaction or side product arising in order to obtain pure final compounds in good yields. Particular attention will be devoted to automated or semiautomated purification techniques when solution-phase focused libraries are involved.
The synthesis of a model library is not strictly necessary because of the reduced complexity of a focused library, but it may be appropriate to prepare a few discretes with the very same equipment to be used for the focused library synthesis. The library is then prepared, purified when necessary, and fully characterized. The purity of all the compounds will be determined using the most appropriate analytical techniques and only after having satisfied all the selected criteria, the library individuals will be tested on the target, and the active molecules progressed as lead compounds for a specific application.
5.2.4 Scaffolds and Monomers
A focused library, even if much simpler in architecture when compared to a primary library, is a very valuable tool to speed up the discovery of a lead compound or its optimization. Its synthesis takes place when positive indications regarding the structure of its components are already known, so that the probability of finding relevant activities on a specific target is higher than for a primary library, where moderate activities can be expected at best. This justifies any effort for rationally selected monomers/scaffolds (see Section 5.4.2), which are useful to gather additional information on the target and to lead to the requisite activities. The selection of noncommercial monomers and/or novel scaffolds should actually be preferred in that the novelty embedded in the resulting structure provides an advantage over competitors interested in the same target.
174 SYNTHETIC ORGANIC LIBRARIES: LIBRARY DESIGN AND PROPERTIES
5.3 BIASED-TARGETED LIBRARIES: INFORMATION-RICH PRIMARY LIBRARIES
5.3.1 Properties
It could be safely assumed that nowadays diversity-inspired, pure primary libraries are not synthesized any more: in fact, although they can produce a large number of compounds their representation of the huge “chemical diversity space” remains necessarily limited, and the need to focus large libraries on “meaningful diversity” is more and more urgent.
A compromise between large unbiased and small focused libraries has become popular, especially for pharmaceutical applications. These biased-targeted libraries are not inspired by a precise structural information, but rather by general information regarding similar classes of targets (e.g., kinases or 7-transmembrane receptors) or by the desired activity-unrelated profile that a drug must possess (e.g., the molecular weight, the partition coefficient, the water solubility, and other physicochemical properties). Their main properties are listed in Fig. 5.6.
Biased-targeted libraries are similar to primary libraries in terms of their architecture and format. In fact, the reported primary libraries have often been designed taking into account some of the above-mentioned filters (information biased) and thus should actually be called pseudofocused libraries. They are tested on as many targets as possible, as for primary libraries, but the information gathered in terms of activity (valuable hits) should be more easily transformed into relevant active leads to be developed further.
BIASED-TARGETED LIBRARIES
-MEDIUM/LARGE (thousands to hundreds of thousands)
-FREQUENTLY ON SOLID PHASE
-FREQUENTLY AS POOLS
-<<1mg PER LIBRARY INDIVIDUAL
-INFORMATION-BIASED
-DIVERSITY-BASED
-TESTED ON MANY TARGETS
-DESIRED OUTCOME: VALUABLE HIT
Figure 5.6 Biased-targeted libraries: main features.
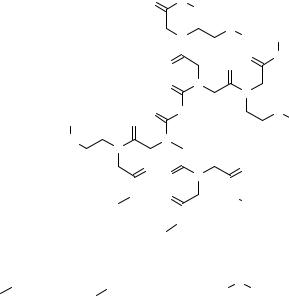
5.3 BIASED-TARGETED LIBRARIES: INFORMATION-RICH PRIMARY LIBRARIES 175
5.3.2 Rationale
•Why? A biased-targeted library is used as a source of relevant activities on various targets, and the availability of several robust and reliable HTS assays and the instrumentation allowing the successful synthesis and analytical characterization of the library are necessary, as mentioned for primary libraries. Moreover, some target-related or some physicochemical filters are introduced to select more valuable compounds. Knowledge of the appropriate selection techniques and the necessary equipment must be available (e.g., commercial computational databases and proprietary or published information on target classes; see Section 5.4.3).
•When? How? What? How Much? The answers to all these questions are identical to those given above for primary libraries and will not be commented upon further. It is worth saying that any generic information that can be transformed into a structural filter to select valuable library components should be applied whenever possible. The cost of the library synthesis in terms of time and effort will not vary significantly when compared to a primary library.
An example of a biased library, shown in Fig. 5.7, which was reported by Boger et al. (10), was designed to be a source of tools to probe protein–protein interactions (why). The effort required for the chemical assessment and for a satisfactory charac-
|
|
|
|
|
|
O |
H |
|
|
|
|
|
|
|
|
|
|
|
|
|
|
|
N |
|
|
|
|
|
|
|
|
||
1,596 compounds |
|
R1 |
|
|
H |
|
|
|
|
|||||||
|
|
|
|
N |
|
|
|
|
||||||||
|
168 pools |
|
|
|
N |
|
|
|
|
R2 |
|
|
R1 |
|||
|
|
|
|
|
|
|
|
|||||||||
|
|
|
|
|
|
|
|
|
|
|||||||
|
|
|
|
|
|
O |
|
|
O |
O |
|
|
NH |
|||
|
|
|
|
|
|
|
|
|||||||||
|
|
|
|
|
|
|
|
|
|
O |
|
|
|
|
||
|
|
|
|
|
|
O |
|
N |
|
|
|
|
N |
|
|
|
|
|
|
|
|
|
|
|
|
|
|
|
|
|
|
H |
|
|
|
|
|
|
|
O |
X |
|
|
|
|
|
|
|
||
|
|
|
|
|
|
|
|
|
|
|
|
|
N |
|||
|
R1 |
O |
|
|
|
|
|
|
|
|
|
R2 |
||||
|
|
|
|
|
|
|
|
|
|
|||||||
|
NH |
|
|
N |
|
|
|
|
|
|
|
O |
||||
|
|
|
|
N |
|
|
|
|
|
|
O |
|
|
|
||
|
|
|
|
|
|
|
|
|
|
|
|
|
||||
|
|
|
|
|
|
|
|
|
|
|
|
|
|
|
|
|
|
O |
|
|
O |
|
|
|
|
|
|
|
|
|
|||
|
|
|
|
|
|
|
|
|
|
|||||||
|
|
|
|
|
|
O |
|
N |
|
|
|
|
|
|
|
|
|
|
|
|
|
|
|
|
|
|
|
|
|
|
|
||
|
|
|
|
|
|
|
|
|
|
|
|
|
|
|
|
|
|
|
|
|
|
|
NH |
|
|
|
|||||||
|
|
|
|
NH O |
|
|
|
|
|
|
|
|
||||
|
|
|
|
R2 |
|
|
|
|
|
|
R2 |
|
|
|
||
|
|
|
|
|
|
|
|
|
|
|
|
|
||||
|
|
|
|
|
|
R1 |
|
NH |
|
|
|
|
|
|
|
|
|
|
|
|
|
|
|
|
|
|
|
|
|
|
|
|
|
NH2 |
|
R |
NH |
|
HOOC |
X |
|
|
|
|||||||
R1 |
|
2 |
|
|
|
COOH |
||||||||||
|
2 |
|
|
|
|
|
|
|
|
|
|
|
|
|
||
three monomers |
14 monomers |
|
|
38 monomers |
Figure 5.7 Example of a polyamide biased-targeted library.
176 SYNTHETIC ORGANIC LIBRARIES: LIBRARY DESIGN AND PROPERTIES
terization was significant, but the successful preparation of a library aimed at a general class of biological targets more than accounted for this effort (when). The library was prepared as 168 pools in solution (1596 iminodiacetic acid diamide tetramers; how). The synthetic scheme was a mix of different reaction conditions in solution, and some biased monomer sets were used (what). These monomers were either commercially available or easily prepared from commercial precursors, while the library core was the commercially available iminodiacetic acid (how much).
5.3.3 Synthesis and Characterization
All the aspects, from the design of a biased-targeted library to its preparation, analytical characterization, and assay on various targets, are similar to the ones already described for primary libraries and will not be discussed further.
5.3.4 Scaffolds and Monomers
While the number of monomer sets employed for the synthesis of biased-targeted libraries is similar to that seen for primary libraries, some additional selection criteria are applied. If the library design is driven by structural information, the monomers will be chosen accordingly (e.g., hydrogen bond donors or acceptors and lipophilic pockets, to be placed in specific parts of the final library individuals). Furthermore, the scaffold, either as the starting point of the library synthesis or formed during the synthetic scheme, will need to satisfy the structural needs of the target biological class.
When the library individuals are filtered with physicochemical parameters, the nature of the scaffold becomes fundamental. As an example, if a maximum accepted value of log P (partition coefficient between n-octanol and water) of 4 is set as a limit, the use of a functionalized scaffold with log P = 6 will enormously limit the selection of monomers to highly hydrophilic structures, while the selection of a more appropriate scaffold would allow a higher degree of diversity while respecting the imposed filter. The same is true for monomers. For example, if an upper limit of 600 is imposed for the molecular weight (MW) of the final library components, the use of monomers with an MW higher than 250–300 will not be acceptable.
5.4 LIBRARY DESIGN VIA COMPUTATIONAL TOOLS
5.4.1 Primary Libraries
The use of computational tools to increase the quality of combinatorial libraries is becoming more and more popular. These methods can be applied to any library format, and their careful use may add significant value to the library. Their main application is the selection of individuals/monomers for a library from the many virtual monomers/final compounds theoretically available by using all the easily accessible monomers. We will examine in detail the usefulness of computational tools for each library format through the presentation of the main reported approaches by leading groups in
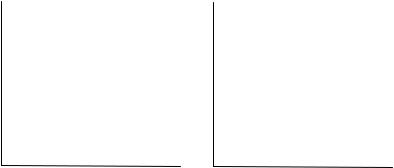
5.4 LIBRARY DESIGN VIA COMPUTATIONAL TOOLS 177
the field. These tools have also been widely used to determine the diversity of compound collections (11–14), either proprietary or commercial, and to select individuals to add to these collections to improve their representativity of chemical space (15–17). While the methods are the same, some typical implications for database selection will be discussed when appropriate.
Primary libraries are not inspired by any structural information, and their purpose is to contain the maximum of chemical diversity so as to function as a potential source of active compounds for many different applications. To consider a library size and its diversity as directly proportional entities is totally wrong, as the simple example in Fig. 5.8 shows. The library on the left, composed of 25 molecules, is much more “diverse” than the 50-member library on the right because it spans more of the chemical space reported in the figure. While the concept of chemical diversity is intuitive, in order to measure the diversity of library components and to select from them the most representative, we must define some key features and methods.
A virtual library is defined as a computer-generated library containing all the compounds obtained by all the permutations of each monomer set composed of all the available monomers possessing the specific chemical functionalities. The available virtual monomers are gathered from commercial databases and sometimes from internal accessible collections. All the virtual library compounds are enumerated and the generated data are stored. This library undergoes a so-called virtual screening, that is, the characterization of each library individual and the selection of the components to be included in the actual library that will be synthesized. This computational screening requires the identification of the appropriate molecular descriptors that characterize a molecule and the determination of indices, or numerical values, that allow a comparison between the various molecules. Once the molecules have been characterized and compared in terms of the selected descriptors, selection methods are necessary to pick out of the virtual library members the actual library components that will be synthesized.
x |
|
x |
|
x |
x |
|
x |
x |
x |
|
|
|
|
x |
x |
x |
|
||||||
|
|
|
|
x |
x |
||||||
|
x |
|
x |
x |
x |
x |
x |
x |
|||
|
|
x |
|
||||||||
|
|
|
|
x |
x x |
x |
x |
x x |
|||
x |
x |
|
|
x |
|
x |
x x |
x x |
|||
|
|
x x |
x |
x |
|
||||||
|
|
|
|
x |
x |
x |
|
x x x |
|||
|
|
|
|
x |
x |
||||||
x |
|
x |
|
|
x x x |
x |
x |
x |
|
x x |
|
|
|
|
|
x |
x |
x |
|
x |
x x |
x |
|
x |
|
|
|
x |
|
|
x |
|
|
|
|
x |
|
|
x |
|
|
|
|
|
|
||
|
|
x |
x |
|
|
|
|
|
|
||
|
|
|
|
|
|
|
|
|
|||
|
|
|
|
|
|
|
|
|
|
|
xx
xx
CHEMICAL SPACE: |
CHEMICAL SPACE: |
diverse library |
focused library |
Figure 5.8 Chemical diversity in the chemical space.
178 SYNTHETIC ORGANIC LIBRARIES: LIBRARY DESIGN AND PROPERTIES
The selection of virtual monomers is made through the electronically accessible databases of chemicals and, where possible, proprietary collections of compounds. The final list will contain compounds that possess the specific functionality, and additional filters may be introduced to consider only reactive potential monomers (e.g., aromatic aldehydes where aliphatic ones are known to be problematic). This filtering function is driven by the chemical expertise and aims to remove inadequate reagents from the very beginning. Once the virtual monomer sets are prepared, the library is enumerated, that is, all the virtual components are prepared “on screen” (18, 19). The storage space required for the complete enumeration of a large virtual primary library is so huge that two more compact representations are normally used: Markush representations, which cover a library with common structural features, through either line notations (20–22) or connection tables (23–25), and reaction-based representations, which are commercialized by various companies (26–28). Both methods use the set of precursors/reactants/monomers and the generic reactions used to prepare the library to characterize the whole virtual set. Examples of both representations are shown in Fig. 5.9; a more detailed description of these representations can be found in the references mentioned above (18–28).
These molecules are then characterized through various molecular descriptors. Such a process can be performed on two different compound sets, productor reagent-based sets, as shown in the hypothetical example reported in Fig. 5.10. Considering a library where a common scaffold is decorated using three large monomer sets composed respectively of 2000, 1000, and 500 monomers just using commercial compounds, our virtual library would consist of 2000 × 1000 × 500 = 1 × 109 individuals. Thousands of candidates are commercially available for the most common monomers such as amines, carboxylic acids, alcohols, or aldehydes, and even bigger virtual libraries can be easily built. All of these virtual products should be enumerated and their properties/descriptors calculated using a product-based selection, requiring significant efforts for the management of such a large number of structures. An alternative is selection based on the structures of the reagents included in the monomer sets, which would involve only the evaluation of three sets totalling 3500 compounds. While it has been proven that the latter method is less accurate in generating diverse libraries (29), it can nevertheless produce a sound selection of the most diverse monomers in a specific class within a reasonable time and has been used mostly for large virtual diversity-based libraries (30). The accuracy lost in examining only the reactant space is more than compensated for by the reduced computing time required and by the effective reduction of each monomer set size.
If we consider the example of Fig. 5.10, when the reagents are selected to produce a 20 × 10 × 10 = 2000-membered library we end up with combined monomer sets composed of 40, rather than 3500, individuals (Fig. 5.11, top). If the 2000 most diverse library components are selected from the product space (Fig. 5.11, bottom), it may be that a significant fraction, or even all, the virtual monomers from one set must be used to prepare them, so that the library cost, in terms of both reagents and human effort, is not significantly reduced. This selection problem was noticed (31) during the selection from a virtual library of the 1600 most diverse amides, which were found to contain 137 amine and 146 carboxylic acid building blocks (19,992 possible combi-
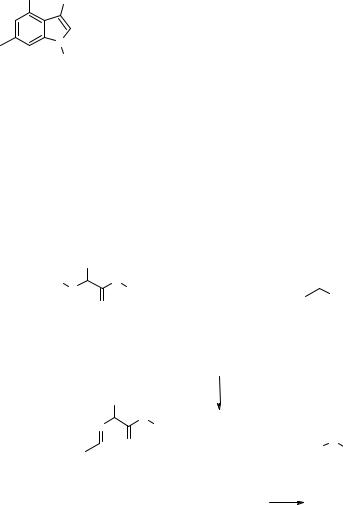
5.4 LIBRARY DESIGN VIA COMPUTATIONAL TOOLS 179
Markush Representation
R4 |
R |
3 |
|
R1 = H, Me, Et, n-Pr, cHex, Bn, Phet, Ph, 4-MeOPh, 4-MePh |
||
|
|
|
R2 |
R2 |
= H, Cl, Br, Me, Ph, CHO, COOH, COOMe, CONH2, CONMe2 |
|
R |
N |
|
R3 |
= H, Cl, Br, I, OMe, OBn, NMe2, NBn2, Me, Et |
||
|
|
|||||
5 |
R1 |
|
|
|||
|
R4 |
= H, Cl, Br, I, Me, CN, COOH, COOMe, CONH2, CONMe2 |
||||
100,000-membered |
||||||
|
|
|||||
indole library |
|
|
R5 |
= H, Cl, Br, Me, Ph, CN, NO2, COOMe, CF3, COPh |
Line Notations: scaffold identification: 1H-Indole12346, then descriptors for 5 randomization points:
[10R1]1.[10R2]2.[10R3]3.[10R4]4.[10R5]6, then monomer definitions: Me=C, Et=CC, CF3=C(F)(F)F and so on.
Reaction-based Representation
|
|
R1 |
|
O |
|||
H |
O |
|
|||||
|
|
|
|
||||
N |
H |
R2 |
|
|
H |
||
|
|
||||||
|
|
|
|
|
|||
H |
O |
||||||
|
|
|
|
||||
[H:1][N:2]([H:3])[C:4]([*:5])[C:6](=[O:7])[O:8][H:9]. |
[O:10]=[C:11]([H:12])[*:13]>> |
|
R1 |
|
N |
O |
|
H |
|
|
|
O |
O |
R2 |
|
H H |
[*:13][C:11]([H:12])=[N:2][C:4]([*:5])[C:6](=[O:7])[O:8][H:9]. |
[H:1][O:10][H:3] |
* = Randomization point; >> =
Figure 5.9 Representation of chemical libraries: Markush and reaction-based approaches.
nations). Such a problem disappears when considering selections among commercial/proprietary databases where the virtual compounds to be ordered/withdrawn for a specific assay are structurally unrelated and need not be synthesized.
Monomer selection may be significantly assisted by a different perspective, that is the computational evaluation of the reactivity of each potential monomer belonging to a virtual set. In fact, it may well happen that a suitable, diversity-adding selected monomer has a poor reactivity in the library reaction scheme that will eventually

180 SYNTHETIC ORGANIC LIBRARIES: LIBRARY DESIGN AND PROPERTIES
|
|
|
X |
|
|||||
|
|
R |
|
|
|
|
R1 |
R |
|
|
|
1 |
|
|
|
|
|
||
|
|
|
|
|
|
|
|
2 |
|
SCAFFOLD + |
R2 |
Y |
SCAFFOLD |
||||||
|
|
|
|
|
|||||
W |
|||||||||
|
|
R |
R3 |
||||||
|
|
|
|
|
|
|
|||
|
|
3 |
|
|
|
|
|
|
|
|
|
|
|
|
|
|
|
1,000,000,000 |
|
|
|
|
|
|
|
|
virtual library components |
||
X |
Y |
|
|
W |
|
||||
R1 |
R2 |
|
R3 |
|
|||||
2000 |
1000 |
|
500 |
|
|
|
|
||
monomers |
monomers |
|
monomers |
|
PRODUCT-BASED SELECTION: 1,000,000,000 generated and characterized structures
REAGENT-BASED SELECTION: 3500 characterized structures
Figure 5.10 Virtual libraries: product-based and reagent-based selection methods.
surface during the library synthesis; the a priori selection of a similar, better reacting monomer would be highly beneficial and would save time, efforts and money. Horvath (32) has reported a reactivity prediction model for any chemical transformation, and has successfully applied it to the selection of carboxylic acids for the synthesis of an amide library; 100 out of the virtual 150 monomers were selected according to their reactivity, and the error of predicted versus experimental reactivity in the acylation for the acids was on average less than 10% and never more than 20%.
Examples of library selections based on virtual products, rather than monomers, have been reported, especially when the computational burden is reduced by fixing the scaffold orientation and optimizing only the randomization points (33). From now on we will not mention specifically if selections are performed on virtual sets of reagents or products, providing that the reader remembers throughout the rest of this section the relevance of this issue and its dependence on project-related factors (number, availability of hardware/software, and so on), rather than on dogmatic assumptions.
The selection among virtual monomers, or products, is made by considering their properties as determined by a set of molecular descriptors, and then selecting the most diverse representatives. Many descriptors have been reported (34), and they can be grouped into three main classes: one-, two-, and three-dimensional (1D, 2D, and 3D) descriptors. One-dimensional descriptors are represented by a single value integer, as for the so-called topological indices (35–37, 11), which characterize the bonding pattern of a molecule, or by a real value calculated for global molecular properties (38, 39, 30), such as molecular weight, lipophilicity, and solvation energy. Their calculation is easy and fast, thus reducing the time required for the virtual screening.
Two-dimensional descriptors are normally represented by linear bit strings indicating the presence or the absence of some properties in the molecule: Examples include structural fragments (structural keys; 40, 41, 36), specific atom paths with predefined
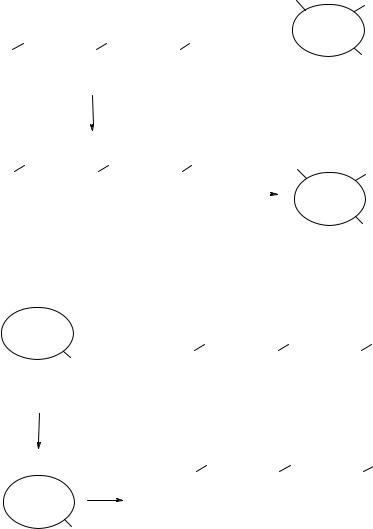
|
5.4 |
LIBRARY DESIGN VIA COMPUTATIONAL TOOLS 181 |
|||||
|
|
|
|
|
R1 |
R2 |
|
|
|
|
|
|
|
||
X |
Y |
W |
|
|
SCAFFOLD |
|
|
|
|
|
|
||||
R1 |
R2 |
R3 |
|
|
|
R3 |
|
2000 virtual |
1000 virtual |
500 virtual |
1,000,000,000 |
||||
|
|||||||
monomers |
monomers |
monomers |
|
virtual library components |
|||
|
REAGENT-BASED |
|
|
|
|
||
|
SELECTION |
|
|
|
|
||
|
|
|
|
|
|
|
|
X |
Y |
W |
|
|
R1 |
|
|
R1 |
R2 |
R3 |
|
|
R2 |
||
|
|
|
|||||
20 real |
10 real |
10 real |
|
|
SCAFFOLD |
|
|
|
|
|
|||||
monomers |
monomers |
monomers |
|
|
|
||
|
|
|
|
||||
|
|
|
|
|
|
R3 |
|
|
|
|
2000 |
||||
|
|
|
|
library components
R1 R2
SCAFFOLD
R3
1,000,000,000 virtual library components
PRODUCT-BASED
SELECTION
R1 R2
SCAFFOLD
R3
2000 library components
X |
Y |
W |
R1 |
R2 |
R3 |
2000 virtual |
1000 virtual |
500 virtual |
monomers |
monomers |
monomers |
X |
Y |
W |
R1 |
R2 |
R3 |
up to 2000 |
up to 1000 |
up to 500 |
real monomers |
real monomers |
real monomers |
|
|
|
Figure 5.11 Product-based and reagent-based selection methods: impact on monomer selection.
lengths (hashed fingerprints; 42–45), and intermolecular interactions (binding properties; 46–48). Their calculation is relatively fast, and when chosen for a monomer/product selection, they do not represent a bottleneck for the project. An example of 2D descriptors is reported in Fig. 5.12.
Three-dimensional descriptors represent spatial relationships, such as distances and angles, between key functionalities in a structure, and they are encoded by linear bit
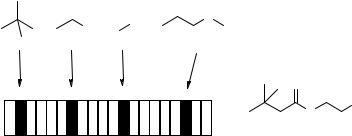
182 SYNTHETIC ORGANIC LIBRARIES: LIBRARY DESIGN AND PROPERTIES
C |
|
O |
|
|
||
C C |
|
|
|
N C Cl C |
C |
N C |
C C |
|
|
C |
C
2D-bin string for
O
Cl
N
H
Figure 5.12 Two-dimensional molecular descriptors: an example.
strings, where a range is first defined (e.g., 2–10 Å for a distance or 30°–90° for an angle) and then a certain increment defines the bin width (e.g., 1 Å or 5°). These distances and angles are measured between atom pairs, or between functionalities, and define the so-called pharmacophores (threeor four-point pharmacophores), which are stored and used to compare molecules in terms of diversity or similarity. Examples of 3D descriptors are reported in Fig. 5.13. Many different research groups have defined different sets of 3D descriptors/pharmacophores (49–62), and all of them have been used to select diverse sets of compounds.
A recent method called 4D-QSAR (63) builds and optimizes a set of relevant 3D-pharmacophores for a specific target and effectively samples the available conformational space and identifies a sound QSAR in terms of binding mode of each compound examined. The method has been successfully validated in the design and virtual screening of libraries aimed towards the thromboxane A2 receptor (64) and glycogen phosphorylase b (65, 66); both examples have produced virtual hits which, once prepared and tested on the target, were confirmed as novel bioactive compounds.
While a 3D descriptor should represent the 3D interaction between a molecule and a biological target more accurately, the time required to define the 3D descriptors for a large virtual set of structures is significantly higher than for 1D or 2D descriptors, and often this cannot be tolerated by the project. Three-dimensional descriptors can be obtained from a fixed low-energy conformation for a given structure (rigid descriptors) or by the same distances/angles obtained on a number of conformations for the same structure (flexible descriptors). The former are easily calculated in a timely manner but are significantly less information rich than the latter. An example (41) showed how 2D descriptors can perform significantly better than rigid 3D descriptors in separating biologically active molecules from inactives, thus hopefully leading to a good compound selection; other comparisons between descriptors and descriptor classes have also been recently reported (67–70).
The selection of the most appropriate descriptors out of this wide range of choices is often driven by project-related parameters (number of virtual library components or monomers) or by the available computational equipment (software and hardware). It is important to avoid the use of different descriptors that describe the same property or are biased by similar contributions because this would increase their weight in the