
- •Distribution Overview
- •Discrete Distributions
- •Continuous Distributions
- •Probability Theory
- •Random Variables
- •Transformations
- •Expectation
- •Variance
- •Inequalities
- •Distribution Relationships
- •Probability and Moment Generating Functions
- •Multivariate Distributions
- •Standard Bivariate Normal
- •Bivariate Normal
- •Multivariate Normal
- •Convergence
- •Statistical Inference
- •Point Estimation
- •Empirical distribution
- •Statistical Functionals
- •Parametric Inference
- •Method of Moments
- •Maximum Likelihood
- •Delta Method
- •Multiparameter Models
- •Multiparameter delta method
- •Parametric Bootstrap
- •Hypothesis Testing
- •Bayesian Inference
- •Credible Intervals
- •Function of parameters
- •Priors
- •Conjugate Priors
- •Bayesian Testing
- •Exponential Family
- •Sampling Methods
- •The Bootstrap
- •Rejection Sampling
- •Importance Sampling
- •Decision Theory
- •Risk
- •Admissibility
- •Bayes Rule
- •Minimax Rules
- •Linear Regression
- •Simple Linear Regression
- •Prediction
- •Multiple Regression
- •Model Selection
- •Non-parametric Function Estimation
- •Density Estimation
- •Histograms
- •Kernel Density Estimator (KDE)
- •Smoothing Using Orthogonal Functions
- •Stochastic Processes
- •Markov Chains
- •Poisson Processes
- •Time Series
- •Stationary Time Series
- •Estimation of Correlation
- •Detrending
- •ARIMA models
- •Causality and Invertibility
- •Spectral Analysis
- •Math
- •Gamma Function
- •Beta Function
- •Series
- •Combinatorics
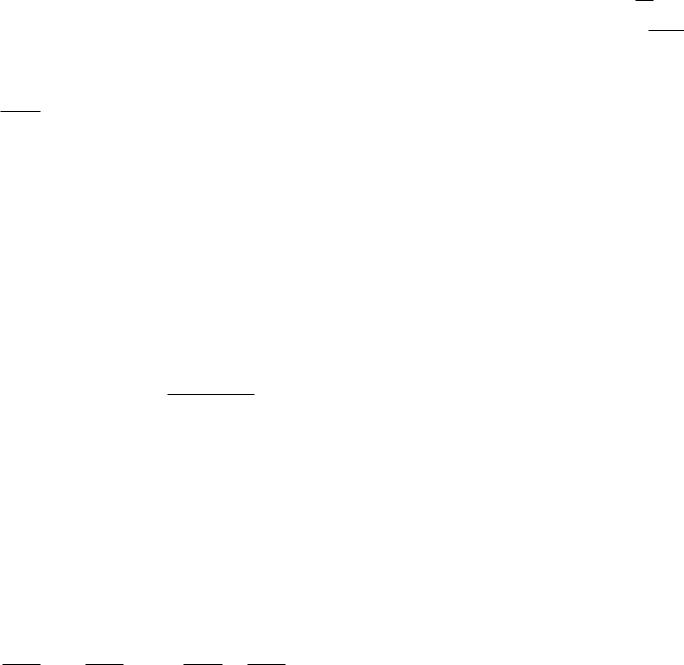
8Probability and Moment Generating Functions
GX(t) = E tX |
jtj < 1 |
1 (Xt)i |
|
1 E Xi |
|
|
i |
||
MX(t) = GX(et) = E eXt = E " |
|
|
# = |
|
|
|
t |
|
|
|
i! |
i=0 |
i! |
|
|
||||
|
|
i=0 |
|
|
|
|
|||
|
X |
|
X |
|
|
|
|
P [X = 0] = GX(0)P [X = 1] = G0X(0)
P [X = i] = G(Xi)(0) i!
E [X] = G0X(1 )
E Xk = MX(k)(0)
E |
X! |
= GX(k) |
(1 ) |
|
|
|
||
(X k)! |
|
|
|
|||||
V |
|
|
|
|
|
|
(1 ))2 |
|
[X] = G00 (1 ) + G0 |
(1 ) |
|
(G0 |
|||||
|
X |
X |
d |
X |
|
|||
GX(t) = GY (t) =) |
|
|
|
|
||||
X = Y |
|
|
9Multivariate Distributions
9.1Standard Bivariate Normal
Let X; Y N (0; 1) ^ X ?? Z where Y = X + p |
1 2 |
Z |
|
|||||||||
Joint density |
|
|
|
|
|
|
|
|
|
|
|
|
|
|
1 |
|
|
|
2 |
+ y2 |
|
2 xy |
|
||
f(x; y) = |
|
|
|
|
exp |
|
x |
|
|
|
||
|
|
|
|
|
2(1 |
2) |
||||||
2 |
1 |
|
2 |
|||||||||
Conditionals |
p |
|
|
|
|
|
|
|
|
|
||
(Y j X = x) N x; 1 2 |
|
and |
|
(X j Y = y) N y; 1 2 |
||||||||
Independence |
X ?? Y () = 0 |
|
|
|
||||||||
|
|
|
|
9.2Bivariate Normal
Let X N x; x2 and Y N y; y2 . |
|
|
exp |
|
|
|
|
|
|
||||||||
f(x; y) = |
1 |
|
|
|
|
z |
|
|
|
||||||||
|
|
|
|
|
|
2(1 |
2) |
|
|||||||||
2 x y 1 |
|
2 |
|
||||||||||||||
" |
x |
|
2 |
|
p |
2 |
|
|
|
|
|
y |
# |
||||
|
y |
|
|
x |
|
|
|||||||||||
z = |
x x |
|
|
+ |
y y |
|
|
|
2 |
x x |
|
|
y y |
|
|||
|
|
|
|
|
|
|
|
|
|
|
|
Conditional mean and variance
E [X j Y ] = E [X] + X (Y E [Y ])
Y
p
V [X j Y ] = X 1 2
9.3Multivariate Normal
Covariance matrix (Precision matrix 1) |
|
|
|
|||||
= |
0 |
V [X... |
1] |
... Cov [X... |
1; Xk]1 |
|||
|
BCov [Xk; X1] |
|
V [Xk] |
C |
||||
If X N ( ; ), |
@ |
|
|
|
|
A |
||
|
|
|
2 |
(x )T 1(x ) |
||||
fX(x) = (2 ) n=2 j j 1=2 exp |
||||||||
|
|
|
|
1 |
|
|
|
|
Properties
Z N (0; 1) ^ X = + 1=2Z =) X N ( ; )
X N ( ; ) =) 1=2(X ) N (0; 1)
X N ( ; ) =) AX N A ; A AT
X N ( ; ) ^ kak = k =) aT X N aT ; aT a
10 Convergence
Let fX1; X2; : : :g be a sequence of rv's and let X be another rv. Let Fn denote the cdf of Xn and let F denote the cdf of X.
Types of convergence
D
1. In distribution (weakly, in law): Xn ! X
lim Fn(t) = F (t) 8t where F continuous
n!1
P
2. In probability: Xn ! X
(8" > 0) lim P [jXn Xj > "] = 0
n!1
as
3. Almost surely (strongly): Xn ! X
P hn!1 Xn = Xi |
= |
P h |
! |
2 |
n!1 n |
i |
lim |
|
|
: lim X (!) = X(!) |
= 1 |
9