
- •Distribution Overview
- •Discrete Distributions
- •Continuous Distributions
- •Probability Theory
- •Random Variables
- •Transformations
- •Expectation
- •Variance
- •Inequalities
- •Distribution Relationships
- •Probability and Moment Generating Functions
- •Multivariate Distributions
- •Standard Bivariate Normal
- •Bivariate Normal
- •Multivariate Normal
- •Convergence
- •Statistical Inference
- •Point Estimation
- •Empirical distribution
- •Statistical Functionals
- •Parametric Inference
- •Method of Moments
- •Maximum Likelihood
- •Delta Method
- •Multiparameter Models
- •Multiparameter delta method
- •Parametric Bootstrap
- •Hypothesis Testing
- •Bayesian Inference
- •Credible Intervals
- •Function of parameters
- •Priors
- •Conjugate Priors
- •Bayesian Testing
- •Exponential Family
- •Sampling Methods
- •The Bootstrap
- •Rejection Sampling
- •Importance Sampling
- •Decision Theory
- •Risk
- •Admissibility
- •Bayes Rule
- •Minimax Rules
- •Linear Regression
- •Simple Linear Regression
- •Prediction
- •Multiple Regression
- •Model Selection
- •Non-parametric Function Estimation
- •Density Estimation
- •Histograms
- •Kernel Density Estimator (KDE)
- •Smoothing Using Orthogonal Functions
- •Stochastic Processes
- •Markov Chains
- •Poisson Processes
- •Time Series
- •Stationary Time Series
- •Estimation of Correlation
- •Detrending
- •ARIMA models
- •Causality and Invertibility
- •Spectral Analysis
- •Math
- •Gamma Function
- •Beta Function
- •Series
- •Combinatorics
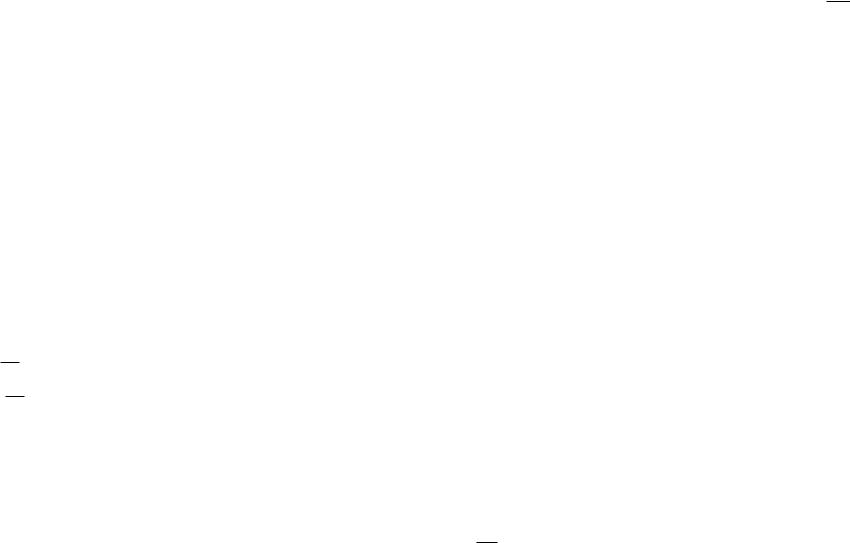
21.2Estimation of Correlation
Sample mean |
|
|
|
|
|
|
|
|
|
|
|
|
|
|
|
|
n |
|
|
|
|
|
|
|
|
|
|
|
|
|
|
|
|
|
|
|
1 |
|
|
|
|
|
|
|
|
|
|
|
|
|
|
|
|
|
|
|
|
|
|
|
Xt |
|
|
|
|
|
||
|
|
|
|
|
|
|
|
|
|
x = n |
|
|
|
|
|
|||||||
|
|
|
|
|
|
|
|
|
|
|
|
xt |
|
|
|
|
||||||
|
|
|
|
|
|
|
|
|
|
|
|
|
|
|
|
=1 |
|
|
|
|
|
|
Sample variance |
|
|
|
|
|
n |
|
|
|
|
|
jnj |
|
|
|
|
||||||
|
|
|
|
|
|
|
|
|
|
|
|
|
|
|
|
x(h) |
||||||
|
|
|
|
|
|
V [x] = n h= n 1 |
||||||||||||||||
|
|
|
|
|
|
|
1 |
|
X |
|
|
|
h |
|
|
|
|
|||||
|
|
|
|
|
|
|
|
|
|
|
|
|
|
|
|
|
|
|
|
|
|
|
Sample autocovariance function |
|
|
|
|
|
|
|
|
|
|
|
|
|
|
|
|
||||||
|
|
|
|
|
|
b |
1 n h |
|
|
|
|
|
|
|
|
|
|
|||||
|
|
|
|
|
|
|
|
|
Xt |
(xt+h x)(xt x) |
||||||||||||
|
|
|
|
|
|
(h) = |
n |
=1 |
||||||||||||||
Sample autocorrelation function |
|
|
|
|
|
|
|
|
|
|
|
|
|
|
|
|
||||||
|
|
|
|
|
|
|
|
|
|
(h) = |
|
(h) |
|
|
|
|
||||||
|
|
|
|
|
|
|
|
|
|
b |
|
|
|
|
|
b |
|
|
|
|
|
|
|
|
|
|
|
|
|
|
|
|
|
|
|
|
|
(0) |
|
|
|
|
|||
Sample cross-variance function |
|
|
|
|
|
|
|
|
b |
|
|
|
|
|
||||||||
|
|
|
|
|
|
b |
|
1 n h |
|
|
|
|
|
|
|
|
||||||
|
|
|
|
|
|
|
|
|
|
Xt |
|
|
|
|
|
|
|
|
||||
|
|
|
|
|
|
xy(h) = |
n |
=1 (xt+h x)(yt |
y |
) |
||||||||||||
Sample cross-correlation function |
|
|
|
|
|
|
|
|
|
|
|
|
|
|||||||||
|
|
|
|
|
|
|
|
|
|
|
|
|
|
|
|
|
xy(h) |
|
|
|
|
|
|
|
|
|
|
|
xy(h) = |
|
|
|
|
b |
|
|
|
|
|
||||||
|
|
|
|
|
|
|
|
|
|
|
|
|
|
|
|
|||||||
|
|
|
|
|
|
|
|
|
x(0) y(0) |
|||||||||||||
|
|
|
|
|
|
b |
|
|
|
|
|
|
|
|
|
|||||||
Properties |
|
|
|
|
|
|
|
|
|
|
|
|
pb |
b |
|
|
|
|
||||
|
1 |
|
|
|
|
|
|
|
|
|
|
|
|
|
|
|
|
|
|
|
||
x(h) = |
p |
|
|
|
if xt is white noise |
|
|
|
|
|
|
|
|
|
|
|||||||
n |
|
|
|
|
|
|
|
|
|
|
|
|||||||||||
b |
1 |
|
|
|
|
|
|
|
|
|
|
|
|
|
|
|
|
|
|
|||
xy(h) = |
p |
|
if xt or yt is white noise |
|
|
|
|
|
||||||||||||||
n |
|
|
|
|
|
|||||||||||||||||
b |
|
|
|
|
|
|
|
|
|
|
|
|
|
|
|
|
|
|
|
|
|
|
21.3Non-Stationary Time Series
Classical decomposition model
xt = t + st + wt
t = trend
st = seasonal component
wt = random noise term
21.3.1Detrending
Least squares
1.Choose trend model, e.g., t = 0 + 1t + 2t2
2.Minimize rss to obtain trend estimate bt = b0 + b1t + b2t2
3.Residuals , noise wt
Moving average
The low-pass lter vt is a symmetric moving average mt with aj = 2k1+1 :
|
|
|
|
|
1 |
|
k |
|
|
|
|
|
vt = |
|
xt 1 |
|
|
|
|
|
|
|
|
|
||
|
|
|
|
2k + 1 |
|
|||
|
|
|
|
|
|
|
i= k |
|
|
|
|
|
|
|
|
X |
|
|
|
1 |
|
k |
|
|
|
|
|
If |
|
i= k wt j 0, a linear trend function t = 0 |
+ 1t passes |
||||
2k+1 |
P without distortion
Di erencing
t = 0 + 1t =) rxt = 1
21.4ARIMA models
Autoregressive polynomial |
|
|
|
|
|
|
|
||||
|
(z) = 1 1z pzp |
|
z 2 C ^ p 6= 0 |
||||||||
Autoregressive operator |
|
|
|
|
|
|
|
|
|||
|
|
|
(B) = 1 1B pBp |
||||||||
Autoregressive model order p, AR (p) |
|
|
|
|
|
||||||
|
xt = 1xt 1 + + pxt p + wt () (B)xt = wt |
||||||||||
AR (1) |
|
|
|
|
|
|
|
|
|
|
|
|
|
|
k 1 |
|
|
k!1;j j<1 |
1 |
|
|
|
|
k |
|
|
Xj |
j |
|
|
j |
||||
xt = (xt k) + |
(wt j) |
= |
X |
||||||||
|
|
(wt j) |
|||||||||
|
|
|
=0 |
|
|
|
j=0 |
||||
E [xt] = |
j=0 (E [wt j]) = 0 |
| |
|
{z |
|
} |
|||||
|
|
||||||||||
|
1 |
|
|
|
|
|
linear process |
||||
(h) = |
j |
|
|
|
|
|
|
|
|
|
|
P |
t+h t |
|
1 2 |
|
|
|
|
|
|
||
Cov [x |
|
; x ] = |
w2 h |
|
|
|
|
|
|
||
|
|
|
|
|
|
|
|
(h) = ((0)h) = h
(h) = (h 1) h = 1; 2; : : :
24