
- •Distribution Overview
- •Discrete Distributions
- •Continuous Distributions
- •Probability Theory
- •Random Variables
- •Transformations
- •Expectation
- •Variance
- •Inequalities
- •Distribution Relationships
- •Probability and Moment Generating Functions
- •Multivariate Distributions
- •Standard Bivariate Normal
- •Bivariate Normal
- •Multivariate Normal
- •Convergence
- •Statistical Inference
- •Point Estimation
- •Empirical distribution
- •Statistical Functionals
- •Parametric Inference
- •Method of Moments
- •Maximum Likelihood
- •Delta Method
- •Multiparameter Models
- •Multiparameter delta method
- •Parametric Bootstrap
- •Hypothesis Testing
- •Bayesian Inference
- •Credible Intervals
- •Function of parameters
- •Priors
- •Conjugate Priors
- •Bayesian Testing
- •Exponential Family
- •Sampling Methods
- •The Bootstrap
- •Rejection Sampling
- •Importance Sampling
- •Decision Theory
- •Risk
- •Admissibility
- •Bayes Rule
- •Minimax Rules
- •Linear Regression
- •Simple Linear Regression
- •Prediction
- •Multiple Regression
- •Model Selection
- •Non-parametric Function Estimation
- •Density Estimation
- •Histograms
- •Kernel Density Estimator (KDE)
- •Smoothing Using Orthogonal Functions
- •Stochastic Processes
- •Markov Chains
- •Poisson Processes
- •Time Series
- •Stationary Time Series
- •Estimation of Correlation
- •Detrending
- •ARIMA models
- •Causality and Invertibility
- •Spectral Analysis
- •Math
- •Gamma Function
- •Beta Function
- •Series
- •Combinatorics
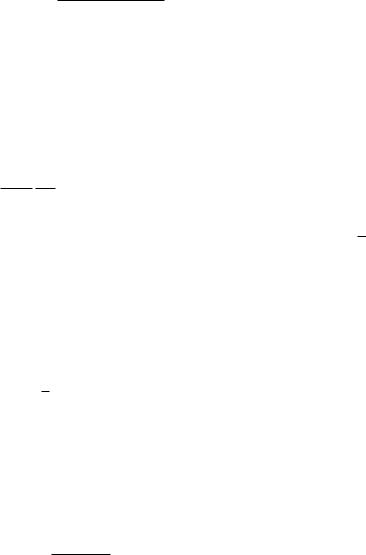
Training error
|
|
b |
|
|
n |
b |
|
|
|
|
|
|
|
||
|
|
|
Xi |
|
|
|
|
|
|
|
|||||
|
|
Rtr(S) = |
=1 |
(Yi(S) Yi)2 |
|
|
|
|
|
||||||
|
|
|
|
|
|
|
|
|
|
|
|
|
|
||
R2 |
|
|
|
|
|
|
|
|
|
|
|
|
|
|
|
|
|
rss(S) = 1 |
|
Rtr(S) = 1 |
|
in=1(Yi(S) |
|
|
)2 |
||||||
R2(S) = 1 |
|
|
Y |
||||||||||||
|
|
|
|
|
|
|
|
|
P in=1(Yi |
|
|
|
|
|
|
|
tss |
|
tss |
|
|
||||||||||
|
|
Y |
)2 |
||||||||||||
|
|
|
|
|
|
b |
|
|
|
b |
|
|
|
|
|
|
|
|
|
|
|
|
|
|
|
P |
|
|
|
|
|
The training error is a downward-biased estimate of the prediction risk.
|
h |
i |
|
|
|
E Rbtr(S) < R(S) |
n |
|
|
bias(Rtr(S)) = E hRtr(S)i |
R(S) = 2 i=1 Cov hYi; Yii |
|||
b |
b |
|
X |
b |
Adjusted R2
R2(S) = 1 n 1 rss n k tss
Mallow's Cp statistic
Rb(S) = Rbtr(S) + 2kb2 = lack of t + complexity penalty
Akaike Information Criterion (AIC)
AIC(S) = `n(bS; 2 ) k bS
Bayesian Information Criterion (BIC)
BIC(S) = `n(bS; 2 ) k log n bS 2
Validation and training
|
|
|
m |
|
|
|
|
|
|
|
|
|
|
b |
|
|
Xi |
b |
|
i |
|
jf |
gj |
|
|
|
|
R |
V |
(S) = |
|
i |
m = |
|
4 |
|
2 |
||||
|
|
(Y |
(S) |
Y )2 |
|
validation data |
; often n or |
n |
|||||
|
|
|
=1 |
|
|
|
|
|
|
|
|
|
|
Leave-one-out cross-validation
RCV (S) = |
|
(Yi Y(i))2 = |
|
|
2 |
n |
n |
1i Uii(S) ! |
|||
|
X |
|
Xi |
Y |
Yi(S) |
b |
b |
|
b |
||
|
i=1 |
|
=1 |
|
|
U(S) = XS(XST XS) 1XS (\hat matrix")
19 Non-parametric Function Estimation
19.1Density Estimation
Estimate f(x), where f(x) = |
P |
[X |
2 |
A] = |
RA |
f(x) dx. |
|
||||
Integrated square error (ise) |
|
|
|
|
|
||||||
L(f; fn) = Z |
f(x) fn(x) |
|
2 dx = J(h) + Z f2(x) dx |
||||||||
Frequentist risk |
b |
|
|
|
b |
|
b2(x) dx + Z |
|
|||
|
R(f; fbn) = E hL(f; fbn)i = Z |
v(x) dx |
|||||||||
|
|
b(x) = E hfn(x)i f(x) |
|
||||||||
|
|
|
|
|
|
b |
|
|
i |
|
|
|
|
|
|
|
|
h |
|
|
|
v(x) = V fbn(x)
19.1.1Histograms
De nitions
Number of bins m
Binwidth h = m1
Bin Bj has j observations
De ne pj = j=n and pj = Bj f(u) du |
||||||||||||||||
b |
|
|
|
|
|
|
|
|
|
|
|
R |
|
|
|
|
Histogram estimator |
|
|
|
|
|
|
|
|
|
|
|
|||||
|
|
|
|
|
m |
|
pj |
|
|
|
|
|
|
|||
|
|
|
|
Xj |
|
|
|
|
|
|
|
|
||||
|
|
b |
b |
I(x 2 Bj) |
||||||||||||
|
|
|
pj |
|
||||||||||||
|
fn(x) = |
=1 |
|
h |
||||||||||||
E hfn(x)i = |
|
|
|
|
|
|
|
|
|
|||||||
|
|
|
|
|
|
|
|
|
|
|
|
|||||
|
h |
|
|
|
|
|
|
|
|
|
||||||
V h |
b |
i |
|
pj(1 |
|
|
pj) |
|
|
|
||||||
|
n |
|
|
2 |
|
nh2 |
|
|
|
|
|
|||||
|
f |
|
(x) = |
|
|
|
|
|
|
|
|
|
|
|
|
|
|
b |
|
|
h |
|
|
Z |
|
(f0(u)) |
2 |
1 |
|||||
R(fn; f) |
12 |
|
|
|
du + nh |
|||||||||||
|
|
b |
|
|
|
|
|
|
|
|
|
|
|
|
|
|
|
h = |
1 |
|
|
|
n1=3 |
||
|
|
|
||
R |
(fn; f) |
C |
||
|
||||
n2=3 |
||||
Cross-validation |
estimate of |
E |
||
b |
|
|
||
JCV (h) = |
Z |
fn2(x) dx |
||
b |
|
b |
|
|
|
(f0(u))2 du! |
1=3 |
|
|
|
|
|
|
|
||||||
|
|
|
6 |
|
|
|
|
|
|
|
|
|
|
|
|
|
|
|
|
|
|
|
|
|
|
|
|
|
|
|
|
|
|
|
|
R |
|
3 |
|
2=3 |
|
|
|
|
|
|
|
1=3 |
|
|
|
|
C = |
|
|
|
Z (f0(u))2 du |
|
|
||||||||
|
|
4 |
|
|
|
|||||||||||
[J(h)] |
|
|
|
|
|
|
|
|
|
|
|
|
|
|||
2 |
|
n |
b |
|
|
|
|
|
|
2 |
|
|
n + 1 |
m |
||
|
Xi |
|
|
|
|
|
|
|
|
|
|
|
|
X |
||
n |
=1 |
f( i)(Xi) = |
(n |
|
1)h |
(n |
|
1)h |
j=1 |
|||||||
|
|
|
|
|
|
|
|
|
|
|
|
|
|
p2 bj
20
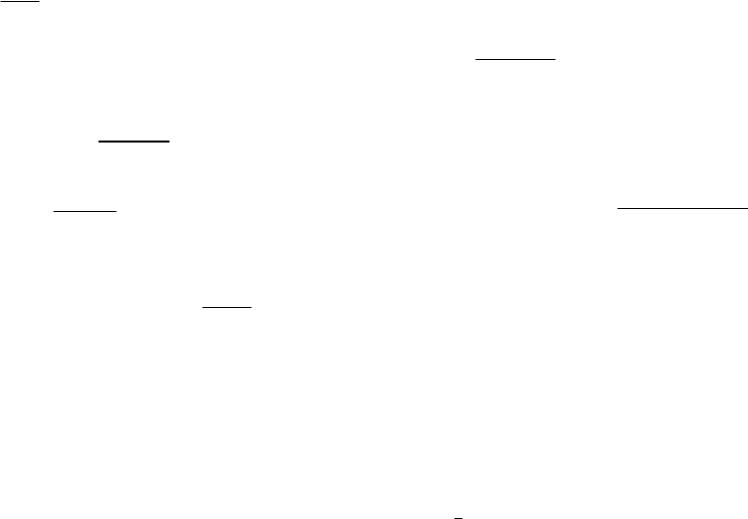
19.1.2Kernel Density Estimator (KDE)
Kernel K
K(x) 0
R
K(x) dx = 1
R
xK(x) dx = 0
R x2K(x) dx K2 > 0
KDE |
|
|
|
|
|
|
|
|
|
|
|
|
|
|
|
|
|
|
|
|
|
|
|
|
|
|
|
|
f |
(x) = |
1 |
n |
1 |
K |
|
x Xi |
|
|
|
|
|
|
|
|
|
|
|
|
|
|
|||||||
|
|
|
|
|
|
|
|
|
|
|
|
|
|
|
|
|
|
|
|
|||||||||
n |
|
n i=1 h |
|
|
|
h |
|
|
|
|
|
|
|
|
|
|
|
|
|
|||||||||
b |
|
1 |
|
|
X |
|
|
|
|
|
|
|
1 |
|
|
|
|
|
|
|
|
|
|
|
||||
R(f; fn) |
|
(h K)4 Z (f00(x))2 dx + |
|
|
|
Z K2(x) dx |
|
|
|
|
|
|
||||||||||||||||
4 |
nh |
|
|
|
|
|
|
|||||||||||||||||||||
|
b |
c 2=5c2 1=5c3 1=5 |
|
2 |
|
; c2 = Z |
|
2 |
|
|
= Z |
|
2 |
|
||||||||||||||
|
h = |
1 |
|
|
|
|
|
|
|
c1 = K |
K |
(x) dx; c3 |
(f00(x)) |
|
dx |
|||||||||||||
|
|
|
|
|
n1=5 |
|
|
|
|
|
|
|||||||||||||||||
R (f; fn) = n4=5 |
c4 |
= 4( K2 )2=5 Z K2(x) dx |
4=5 |
Z (f00)2 dx |
1=5 |
|
|
|||||||||||||||||||||
|
|
|
|
|
|
|||||||||||||||||||||||
|
b |
c4 |
|
|
|
5 |
|
|
|
|
|
|
|
|
|
|
|
|
|
|
|
|||||||
|
|
|
|
|
|
|
|
|
|
|
|
|
|
|
|
|
|
|
|
|
|
|
|
|
|
|
||
|
|
|
|
|
|
|
|
|
| |
|
|
|
|
|
|
|
} |
|
|
|
|
|
||||||
|
|
|
|
|
|
|
|
|
|
|
|
C |
(K) |
|
|
|
|
|
|
|
||||||||
|
|
|
|
|
|
|
|
|
|
|
|
|
|
{z |
|
|
|
|
|
|
|
k-nearest Neighbor Estimator |
|
|
|
|
|
|
|
|
||||||||||||
1 |
|
X |
|
|
|
|
|
|
|
|
where Nk(x) = fk values of x1; : : : ; xn closest to xg |
|||||||||
b |
|
|
|
|
Yi |
|
|
|
||||||||||||
|
|
|
|
|
|
|||||||||||||||
r(x) = k |
|
|
|
|
|
|
||||||||||||||
|
|
i:xi2Nk(x) |
|
|
|
|
|
|
|
|
|
|
|
|
|
|
||||
Nadaraya-Watson Kernel Estimator |
|
|
|
|
|
|||||||||||||||
|
|
b |
|
|
|
n |
K |
x hxi |
|
|
|
|
|
|
||||||
|
|
|
Xi |
|
|
|
|
|
|
|||||||||||
|
|
r(x) = |
|
|
|
|
wi(x)Yi |
|
|
|
|
|
|
|||||||
|
|
|
|
|
=1 |
|
|
K |
|
|
|
|
|
|
|
|
||||
|
|
wi(x) = |
|
|
|
|
|
|
2 [0; 1] |
|
|
|
|
|||||||
|
|
|
|
|
n |
|
|
x xj |
|
|
|
2 |
||||||||
|
|
|
|
|
|
|
4j=1 |
h |
|
4 |
|
|
|
|||||||
|
|
|
|
|
|
hP |
|
|
|
|
|
|
|
|
|
|
|
|||
|
|
|
|
|
|
Z |
x2K2(x) dx Z r00 |
|
|
f (x) |
dx |
|||||||||
R(rn; r) |
|
|
|
(x) + 2r0 |
(x) |
0 |
||||||||||||||
4 |
|
f(x) |
||||||||||||||||||
|
|
b |
|
|
|
|
|
2 |
|
K2(x) dx |
|
|
|
|
|
|
||||
|
|
|
|
+ Z |
c1 |
|
|
Rnhf(x) |
|
dx |
|
|
|
|
|
|||||
|
|
|
h |
|
|
|
|
|
|
|
|
|
|
|
|
|
||||
|
|
|
n1=5 |
|
|
|
|
|
|
|
|
|
|
|
||||||
R (r |
; r) |
|
|
|
c2 |
|
|
|
|
|
|
|
|
|
|
|
|
|||
n4=5 |
|
|
|
|
|
|
|
|
|
|
||||||||||
|
|
bn |
|
|
|
|
|
|
|
|
|
|
|
Epanechnikov Kernel |
(0 |
|
|
|
otherwisej j |
|||||||
|
|
K(x) = |
|
|
||||||||
|
|
|
|
|
|
|
|
3 |
|
x < p |
|
|
|
|
|
|
|
|
4p |
5(1 x2=5) |
5 |
||||
Cross-validation estimate of E [J(h)] |
|
|
|
|
|
|
||||||
JCV (h) = Z |
fn2 |
|
2 n |
|
|
1 |
n n |
|||||
(x) dx n i=1 f( i)(Xi) hn2 |
i=1 j=1 K |
|||||||||||
b |
b |
|
|
X b |
|
|
|
|
|
XX |
Cross-validation estimate of E [J(h)]
n |
n |
|
|
|
|
|
|
|
|
|
|
JCV (h) = (Yi r( i)(xi))2 = |
X |
|
(Yi |
r(xi))2 |
|
|
|
||||
|
|
|
|
|
2 |
||||||
Xi |
|
|
|
|
K(0) |
|
|
|
|
||
b |
|
1 |
|
|
|
b |
|
|
|
|
|
|
|
n |
K |
x |
xj |
! |
|
||||
|
|
|
|
|
|||||||
b |
i=1 |
|
j=1 |
|
h |
|
|||||
=1 |
|
|
P |
|
|
|
|
|
|
||
|
|
|
|
|
|
|
|
19.3Smoothing Using Orthogonal Functions
|
h |
|
nh |
1 |
J |
|
|
Xi Xj |
+ |
2 |
K(0) |
Approximation |
|
|
|
|
|
X X
r(x) = j j(x) j j(x)
Z
K (x) = K(2)(x) 2K(x) K(2)(x) = K(x y)K(y) dy
19.2Non-parametric Regression
Estimate f(x) where f(x) = E [Y j X = x]. Consider pairs of points (x1; Y1); : : : ; (xn; Yn) related by
Yi = r(xi) + i
E [ i] = 0
V [ i] = 2
j=1 |
|
i=1 |
|
|
|
Multivariate regression |
|
|
|
|
|
Y = + |
|
|
|
||
where i = i and |
= |
0 0(...x1) |
... |
J (...x1) |
1 |
|
|
B 0(xn) |
|
J (xn)C |
|
|
|
@ |
|
A |
Least squares estimator
b = ( T ) 1 T Y
n1 T Y (for equally spaced observations only)
21