
Encyclopedia of SociologyVol._1
.pdf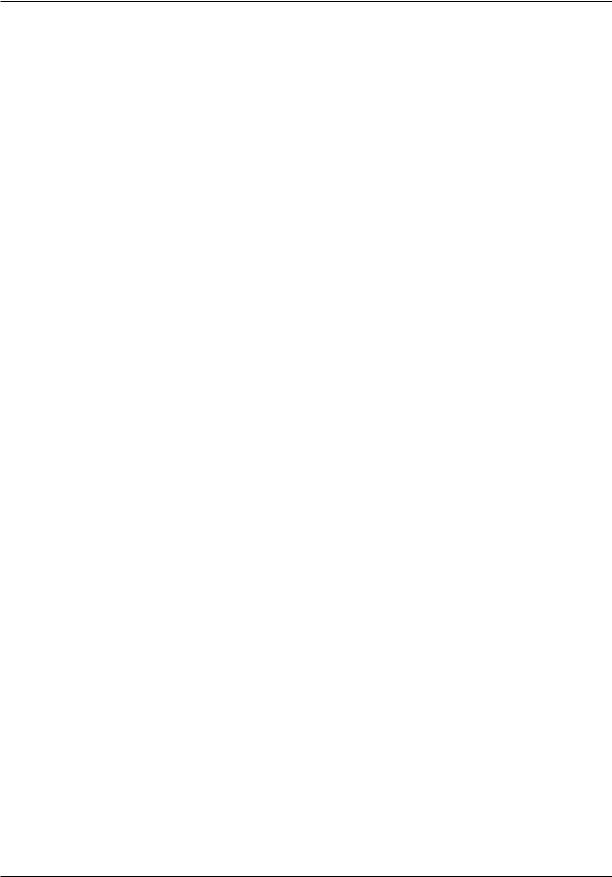
CORRELATION AND REGRESSION ANALYSIS
States because such coefficients are affected by the variances in the two populations.
The multiple correlation coefficient, R, is defined as the correlation between the observed values of Y and the values of Y predicted by the multiple regression equation. It would be unnecessarily tedious to calculate the multiple correlation coefficient in that way. The more convenient computational procedure is to compute R2 (for two predictors, and analogously for more than two predictors) by the following:
R2 = b*y1.2ry1 + b*y2.1ry2 |
(19) |
Like r2, R2 varies from 0 to 1.0 and indicates the proportion of variance in the criterion that is ‘‘explained’’ by the predictors. Alternatively stated, R2 is the percent of the possible reduction in prediction error (measured by the variance of actual values around predicted values) that is achieved by shifting from (a) as the prediction to
(b) the multiple regression values, Ŷ, as the prediction.
VARIETIES OF MULTIPLE REGRESSION
The basic concept of multiple regression has been adapted to a variety of purposes other than those for which the technique was originally developed. The following paragraphs provide a brief summary of some of these adaptations.
Dummy Variable Analysis. As originally conceived, the correlation coefficient was designed to describe the relationship between continuous, normally distributed variables. Dichotomized predictors such as gender (male and female) were introduced early in bivariate regression and correlation, which led to the ‘‘point biserial correlation coefficient’’ (Walker and Lev 1953). For example, if one wishes to examine the correlation between gender and income, one may assign a ‘‘0’’ to each instance of male and a ‘‘1’’ to each instance of female to have numbers representing the two categories of the dichotomy. The unstandardized regression coefficient, computed as specified above in equation 3, is then the difference between the mean income for the two categories of the dichotomous predictor, and the computational formula for r (equation 5), will yield the point biserial correlation coefficient, which can be interpreted much like any other r. It was then only a small step to the
inclusion of dichotomies as predictors in multiple regression analysis, and then to the creation of a set of dichotomies from a categorical variable with more than two subdivisions—that is, to dummy variable analysis (Cohen 1968; Bohrnstedt and Knoke 1988; Hardy 1993).
Religious denomination—e.g., Protestant, Catholic, and Jewish—serves as an illustration. From these three categories, one forms two dichotomies, called ‘‘dummy variables.’’ In the first of these, for example, cases are classified as ‘‘1’’ if they are
Catholic, and ‘‘0’’ otherwise (i.e., if Protestant or
Jewish). In the second of the dichotomies, cases are classified as ‘‘1’’ if they are Jewish, and ‘‘0’’ otherwise (i.e., if Protestant or Catholic). In this illustration, Protestant is the ‘‘omitted’’ or ‘‘reference’’ category (but Protestants can be identified as those who are classified ‘‘0’’ on both of the other dichotomies). The resulting two dichotomized ‘‘dummy variables’’ can serve as the only predictors in a multiple regression equation, or they may be combined with other predictors. When the dummy variables mentioned are the only predictors, the unstandardized regression coefficient for the predictor in which Catholics are classified ‘‘1’’ is the difference between the mean Y for Catholics and Protestants (the ‘‘omitted’’ or ‘‘reference’’ category). Similarly, the unstandardized regression coefficient for the predictor in which Jews are classified ‘‘1’’ is the difference between the mean Y for Jews and Protestants. When the dummy variables are included with other predictors, the unstandardized regression coefficients are the same except that the difference of each mean from the mean of the ‘‘reference’’ category has been statistically adjusted to control for each of the other predictors in the regression equation.
The development of ‘‘dummy variable analysis’’ allowed multiple regression analysis to be linked to the experimental statistics developed by R. A. Fisher, including the analysis of variance and covariance. (See Cohen 1968.)
Logistic Regression. Early students of correlation anticipated the need for a measure of correlation when the predicted or dependent variable was dichotomous. Out of this came (a) the phi coefficient, which can be computed by applying the computational formula for r (equation 5) to two dichotomies, each coded ‘‘0’’ or ‘‘1,’’ and (b) the tetrachoric correlation coefficient, which uses
454

CORRELATION AND REGRESSION ANALYSIS
information in the form of two dichotomies to estimate the Pearsonian correlation for the corresponding continuous variables, assuming the dichotomies result from dividing two continuous and normally distributed variables by arbitrary cutting points (Kelley 1947; Walker and Lev 1953; Carroll 1961).
These early developments readily suggested use of a dichotomous predicted variable, coded ‘‘0’’ or ‘‘1,’’ as the predicted variable in a multiple regression analysis. The predicted value is then the conditional proportion, which is the conditional mean for a dichotomized predicted variable. But this was not completely satisfactory in some circumstances because the regression predictions are, under some conditions, proportions greater than
1 or less than 0. Logistic regression (Retherford
1993; Kleinman 1994; Menard 1995) is responsive to this problem. After coding the predicted variable ‘‘0’’ or ‘‘1,’’ the predicted variable is transformed to a logistic—that is, the logarithm of the ‘‘odds,’’ which is to say the logarithm of the ratio of the number of 1’s to the number of 0’s. With the logistic as the predicted variable, impossible regression predictions do not result, but the unstandardized logistic regression coefficients, describing changes in the logarithm of the ‘‘odds,’’ lack the intuitive meaning of ordinary regression coefficients. An additional computation is required to be able to describe the change in the predicted proportion for a given one-unit change in a predictor, with all other predictors in the equation held constant.
Path Analysis. The interpretation of multiple regression coefficients can be difficult or impossible when the predictors include an undifferentiated set of causes, consequences, or spurious correlates of the predicted variable. Path analysis was developed by Sewell Wright (1934) to facilitate the interpretation of multiple regression coefficients by making explicit assumptions about causal structure and including as predictors of a given variable only those variables that precede that given variable in the assumed causal structure. For example, if one assumes that Y is influenced by X1 and X2, and X1 and X2 are, in turn, both influenced by Z1,
Z2, and Z3, this specifies the assumed causal structure. One may then proceed to write multiple regression equations to predict X1, X2, and Y, including in each equation only those variables that come prior in the assumed causal order. For
example, the Z variables are appropriate predictors in the equation predicting X1 because they are assumed causes of X1. But X2 is not an appropriate predictor of X1 because it is assumed to be a spurious correlate of X1 (i.e., X1 and X2 are presumed to be correlated only because they are both influenced by the Z variables, not because one influences the other). And Y is not an appropriate predictor of X1 because Y is assumed to be an effect of X1, not one of its causes. When the assumptions about the causal structure linking a set of variables have been made explicit, the appropriate predictors for each variable have been identified from this assumed causal structure, and the resulting equations have been estimated by the techniques of regression analysis, the result is a path analysis, and each of the resulting coefficients is said to be a ‘‘path coefficient’’ (if expressed in standardized form) or a ‘‘path regression coefficient’’ (if expressed in unstandardized form).
If the assumed causal structure is correct, a path analysis allows one to ‘‘decompose’’ a correlation between two variables into ‘‘direct effects’’; ‘‘indirect effects’’; and, potentially, a ‘‘spurious component’’ as well (Land 1969; Bohrnstedt and
Knoke 1988; McClendon 1994).
For example, we may consider the correlation between the occupational achievement of a set of fathers and the occupational achievement of their sons. Some of this correlation may occur because the father’s occupational achievement influences the educational attainment of the son, and the son’s educational attainment, in turn, influences his occupational achievement. This is an ‘‘indirect effect’’ of the father’s occupational achievement on the son’s occupational achievement ‘‘through’’
(or ‘‘mediated by’’) the son’s education. A ‘‘direct effect,’’ on the other hand, is an effect that is not mediated by any variable included in the analysis.
Such mediating variables could probably be found, but if they have not been identified and included in this particular analysis, then the effects mediated through them are grouped together as the ‘‘direct effect’’—that is, an effect not mediated by variables included in the analysis. If the father’s occupational achievement and the son’s occupational achievement are also correlated, in part, because both are influenced by a common cause (e.g., a common hereditary variable), then that
455
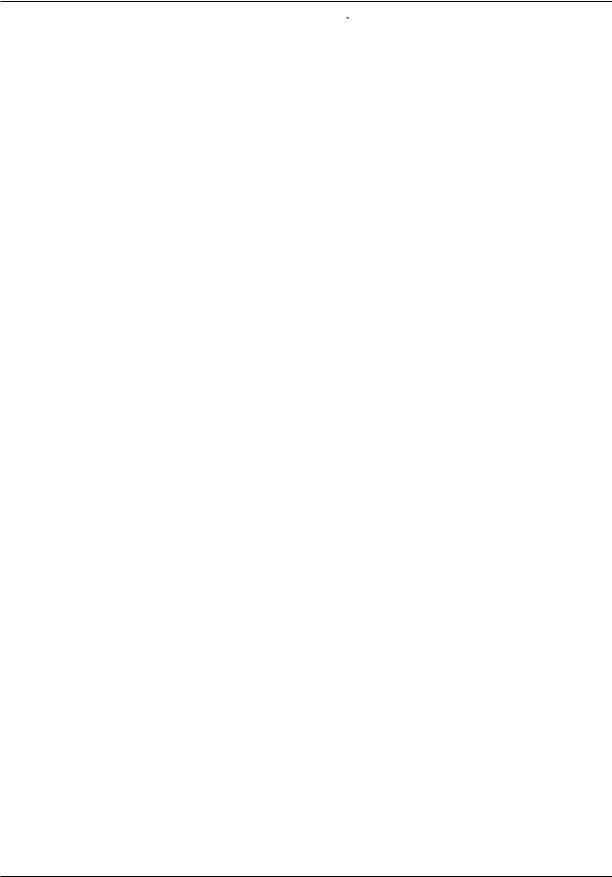
CORRELATION AND REGRESSION ANALYSIS
part of the correlation that is attributable to that common cause constitutes the ‘‘spurious component’’ of the correlation. If the variables responsible for the ‘‘spurious component’’ of a correlation have been included in the path analysis, the ‘‘spurious component’’ can be estimated; otherwise such a ‘‘spurious component’’ is merged into the ‘‘direct effect,’’ which, despite the connotations of the name, absorbs all omitted indirect effects and all omitted spurious components.
‘‘Stepwise’’ Regression Analysis. The interpretation of regression results can sometimes be facilitated without specifying completely the presumed causal structure among a set of predictors. If the purpose of the analysis is to enhance understanding of the variation in a single dependent variable, and if the various predictors presumed to contribute to that variation can be grouped, for example, into proximate causes and distant causes, a stepwise regression analysis may be useful. Depending on one’s primary interest, one may proceed in two different ways. For example, one may begin by regressing the criterion variable on the distant causes, and then, in a second step, introduce the proximate causes into the regression equation.
Comparison of the coefficients at each step will reveal the degree to which the effects of the distant causes are mediated by the proximate causes included in the analysis. Alternatively, one may begin by regressing the criterion variable on the proximate causes, and then introduce the distant causes into the regression equation in a second step. Comparing the coefficients at each step, one can infer the degree to which the first-step regression of the criterion variable on the proximate causes is spurious because of the dependence of both on the distant causes. A stepwise regression analysis may proceed with more than two stages if one wishes to distinguish more than two sets of predictors. One may think of a stepwise regression analysis of this kind as analogous to a path analysis but without a complete specification of the causal structure.
Nonadditive Effects in Multiple Regression.
In the illustrative regression equations preceding this section, each predictor has appeared only once, and never in a ‘‘multiplicative’’ term. We now consider the following regression equation, which includes such a multiplicative term:
Y = ay.12 + by1.2X1 + by2.1X2 + by.12X1X2 (20)
In this equation, Y is said to be predicted, not simply by an additive combination of X1 and X2 but also by their product, X1X2. Although it may not be intuitively evident from the equation itself, the presence of a multiplicative effect (i.e., the regression coefficient for the multiplicative term,
by.12, is not 0) implies that the effect of X1 on Y depends on the level of X2, and vice versa. This is
commonly called an interaction effect (Allison 1977;
Blalock 1979; Jaccard, Turrisi and Wan 1990; Aiken and West 1991; McClendon 1994). The inclusion of multiplicative terms in a regression equation is especially appropriate when there are sound reasons for assuming that the effect of one variable differs for different levels of another variable.
For example, if one assumes that the ‘‘return to education’’ (i.e., the annual income added by each additional year of schooling) will be greater for men than for women, this assumption can be explored by including all three predictors: education, gender, and the product of gender and education.
When product terms have been included in a regression equation, the interpretation of the resulting partial regression coefficients may become complex. For example, unless all predictors are ‘‘ratio variables’’ (i.e., variables measured in uniform units from an absolute 0), the inclusion of a product term in a regression equation renders the coefficients for the additive terms uninterpretable
(see Allison 1977).
SAMPLING VARIATION AND TESTS AGAINST THE NULL HYPOTHESIS
Descriptions based on incomplete information will be inaccurate because of ‘‘sampling variation.’’ Otherwise stated, different samplings of information will yield different results. This is true of sample regression and correlation coefficients, as it is for other descriptors. Assuming a random selection of observed information, the ‘‘shape’’ of the distribution of such sampling variation is often known by mathematical reasoning, and the magnitude of such variation can be estimated. For example, if the true correlation between X and Y is 0, a series of randomly selected observations will rarely yield a correlation that is precisely 0. Instead, the observed correlation will fluctuate around 0 in the
456
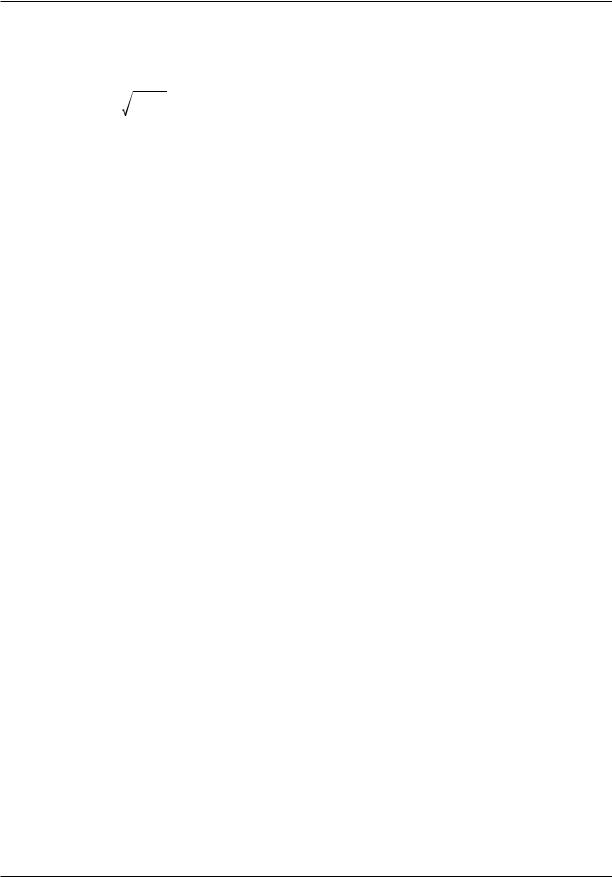
CORRELATION AND REGRESSION ANALYSIS
‘‘shape’’ of a normal distribution, and the standard deviation of that normal sampling distribu- tion—called the standard error of r—will be
σr = |
1 |
(21) |
|
||
|
N – 1 |
where σr = the standard error of r (i.e. the standard deviation of the sampling distribution of r, given that the true correlation is 0); and N = the sample size (i.e., the number of randomly selected cases used in the calculation of r). For example, if the true correlation were 0, a correlation coefficient based on a random selection of 400 cases will have a standard error of approximately 1/20 or .05. An observed correlation of .15 or greater in absolute value would thus be at least three standard errors away from 0 and hence very unlikely to have appeared simply because of random fluctuations around a true value of 0. This kind of conclusion is commonly expressed by saying that the observed correlation is ‘‘significantly different from 0’’ at a given level of significance (in this instance, the level of significance cited could appropriately be
.01). Or the same conclusion may be more simply
(but less precisely) stated by saying that the observed correlation is ‘‘significant.’’
The standard error for an unstandardized bivariate regression coefficient, and for an unstandardized partial regression coefficient, may also be estimated (Cohen and Cohen 1983; Kleinman,
Kupper and Muller 1988; Hamilton 1992; McClendon
1994; Fox 1997). Other things being equal, the standard error for the regression of the criterion on a given predictor will decrease as (1) the number of observations (N) increases; (2) the variance of observed values around predicted values decreases; (3) the variance of the predictor increases; and (4) the correlation between the predictor and other predictors in the regression equation decreases.
PROBLEMS IN REGRESSION ANALYSIS
Multiple regression is a special case of a very general and adaptable model of data analysis known as the general linear model (Cohen 1968; Fennessey 1968; Blalock 1979). Although the assumptions underlying multiple regression seem relatively demanding (see Berry 1993), the technique is remarkably ‘‘robust,’’ which is to say that the technique yields valid conclusions even when the
assumptions are met only approximately (Bohrnstedt and Carter 1971). Even so, restricted or biased samples may lead to conclusions that are misleading if they are inappropriately generalized. Furthermore, regression results may be misinterpreted if interpretation rests on an implicit causal model that is misspecified. For this reason it is advisable to make the causal model explicit, as in path analysis or structural equation modeling and to use regression equations that are appropriate for the model as specified. ‘‘Outliers’’ and ‘‘deviant cases’’ (i.e., cases extremely divergent from most) may have an excessive impact on regression coefficients, and hence may lead to erroneous conclusions. (See Berry and Feldman 1985; Fox 1991; Hamilton 1992.) A ubiquitous but still not widely recognized source of misleading results in regression analysis is measurement error (both random and non-random) in the variables (Stouffer
1936; Kahneman 1965; Gordon 1968; Bohrnstedt and Carter 1971; Fuller and Hidiroglou 1978; Berry and Feldman 1985). In bivariate correlation and regression, the effect of measurement error can be readily anticipated: on the average, random measurement error in the predicted variable attenuates (moves toward zero) the correlation coefficient (i.e., the standardized regression coefficient) but not the unstandardized regression coefficient, while random measurement error in the predictor variable will, on the average, attenuate both the standardized and the unstandardized coefficients. In multiple regression analysis, the effect of random measurement error is more complex. The unstandardized partial regression coefficient for a given predictor will be biased, not simply by random measurement error in that predictor, but also by other features. When random measurement error is entailed in a given predictor, X, that predictor is not completely controlled in a regression analysis. Consequently, the unstandardized partial regression coefficient for every other predictor that is correlated with X will be biased by random measurement error in X. Measurement error may be non-random as well as random, and anticipating the effect of non-random measurement error on regression results is even more challenging than anticipating the effect of random error. Non-random measurement errors may be correlated errors (i.e., errors that are correlated with other variables in the system being analyzed), and therefore they have the potential to distort greatly the estimates of both standardized and
457
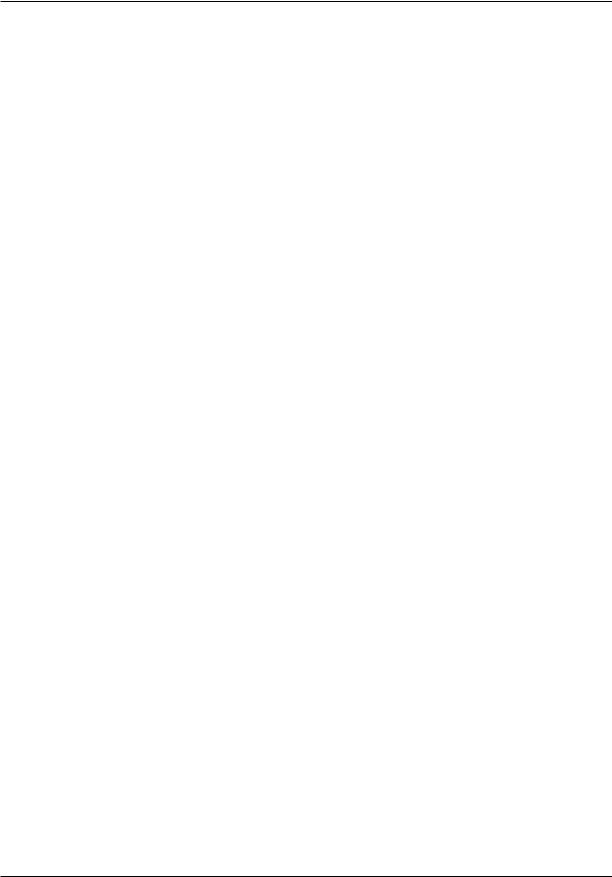
CORRELATION AND REGRESSION ANALYSIS
unstandardized partial regression coefficients. Thus, if the measures used in regression analysis are relatively crude, lack high reliability, or include distortions (errors) likely to be correlated with other variables in the regression equation (or with measurement errors in those variables), regression analysis may yield misleading results. In these circumstances, the prudent investigator should interpret the results with considerable caution, or, preferably, shift from regression analysis to the analysis of structural equation models with multiple indicators. (See Herting 1985; Schumacker and Lomax 1996.) This alternative mode of analysis is well suited to correct for random measurement error and to help locate and correct for nonrandom measurement error.
Fennessey, James 1968 ‘‘The General Linear Model: A New Perspective on Some Familiar Topics.’’ American Journal of Sociology 74:1–27.
Fox, John 1991 Regression Diagnostics. Newbury Park,
Calif.: Sage.
———, 1997 Applied Regression Analysis, Linear Models and Related Methods. Beverly Hills, Calif.: Sage.
Fuller, W. A., and M. A. Hidiroglou 1978 ‘‘Regression Estimates after Correcting for Attenuation.’’ Journal of the American Statistical Association 73:99–104.
Gordon, Robert 1968 ‘‘Issues in Multiple Regression.’’
American Journal of Sociology 73:592–616.
Hamilton, Lawrence C. 1992 Regression with Graphics: A Second Course in Applied Statistics. Pacific Grove, Calif.: Brookes-Cole.
Hardy, Melissa A. 1993 Regression with Dummy Variables. Newbury Park, Calif.: Sage.
REFERENCES
Agresti, Alan and Barbara Finlay 1997 Statistical Methods for the Social Sciences, 3rd ed. Upper Saddle River, N.J.: Prentice Hall.
Aiken, Leona S., and Stephen G. West 1991 Multiple Regression: Testing and Interpreting Interactions. Newbury Park, Calif.: Sage.
Allen, Michael Patrick 1997 Understanding Regression
Analysis. New York: Plenum Press.
Allison, Paul 1977 ‘‘Testing for Interaction in Multiple Regression.’’ American Journal of Sociology 83:144–153.
Berry, William D. 1993 Understanding Regression Assumptions. Newbury Park, Calif.: Sage.
———, and Stanley Feldman 1985 Multiple Regression in Practice. Beverly Hills, Calif.: Sage.
Blalock, Hubert M., Jr. 1979 Social Statistics, 2nd ed. New York: McGraw-Hill.
Bohrnstedt, George W., and T. Michael Carter 1971 ‘‘Robustness in Regression Analysis.’’ In Herbert L. Costner, ed., Sociological Methodology 1971. San Francisco: Jossey-Bass.
———, and David Knoke 1988 Statistics for Social Data Analysis, 2nd ed. Itasca, Ill.: F. E. Peacock.
Carroll, John B. 1961 ‘‘The Nature of the Data, or How to Choose a Correlation Coefficient.’’ Psychometrica 26:347–372.
Cohen, Jacob 1968 ‘‘Multiple Regression as a General Data Analytic System.’’ Psychological Bulletin 70:426–443.
———, and Patricia Cohen 1983 Applied Multiple Regression/Correlation Analysis for the Behavioral Sciences, 2nd ed. Hillsdale, N.J.: Lawrence Erlbaum Associates.
Heise, David R. 1969 ‘‘Problems in Path Analysis and Causal Inference.’’ In Edgar Borgatta and George W. Bohrnstedt, eds., Sociological Methodology 1969. San Francisco: Jossey-Bass.
Herting, Gerald 1985 ‘‘Multiple Indicator Models Using LISREL.’’ In H. M. Blalock, Jr., ed., Causal Models in the Social Sciences. New York: Aldine.
Jaccard, James, Robert Turrisi, and Choi K. Wan 1990
Interaction Effects in Multiple Regression. Newbury Park, Calif.: Sage.
Kahneman, D. 1965 ‘‘Control of Spurious Association and the Reliability of the Controlled Variable.’’ Psychological Bulletin 64:326–329.
Kelley, Truman Lee 1947 Fundamentals of Statistics. Cambridge, Mass.: Harvard University Press.
Kleinman, David G. 1994 Logistic Regression: A Self Learning Text. New York: Springer.
———, Lawrence L. Kupper, and Keith E. Muller 1988
Applied Regression Analysis and Other Multivariable Methods. Boston: PWS-Kent.
Land, Kenneth C. 1969 ‘‘Principles of Path Analysis.’’ In Edgar Borgatta and George W. Bohrnstedt, eds.,
Sociological Methodology 1969. San Francisco: Jossey-Bass.
McClendon, McKee J. 1994 Multiple Regression and Causal Analysis. Itasca, Ill.: F. E. Peacock.
Menard, Scott 1995 Applied Logistic Regression Analysis. Beverly Hills, Calif.: Sage.
Mueller, John H., Karl F. Schuessler, and Herbert L. Costner 1977 Statistical Reasoning in Sociology, 3rd ed. Boston: Houghton Mifflin.
Retherford, Robert D. and Minju Kim Choe 1993 Statistical Models for Causal Analysis. New York: Wiley.
458

COUNTERCULTURES
Schroeder, Larry P., David L. Sjoquist and Paula E. Stephan 1986 Understanding Regression Analysis: An Introductory Guide. Beverly Hills, Calif.: Sage.
Schumacker, Randall E., and Richard G. Lomax 1996 A Beginner’s Guide to Structural Equation Modeling. Mahwah, N.J.: Lawrence Erlbaum Associates.
Stouffer, Samuel A. 1936 ‘‘Evaluating the Effects of Inadequately Measured Variables in Partial Correlation Analysis.’’ Journal of the American Statistical Association 31:348–360.
Walker, Helen M. 1929 Studies in the History of Statistical Method. Baltimore: Williams and Wilkins.
———, and Joseph Lev 1953 Statistical Inference. New York: Holt.
Weisberg, Sanford 1985 Applied Linear Regression. New
York: Wiley.
Wright, Sewell 1934 ‘‘The Method of Path Coefficients.’’
The Annals of Mathematical Statistics 5:161–215.
HERBERT L. COSTNER
COUNTERCULTURES
The enclaves in which people of the modern era live no longer resemble the small, integrated, and homogeneous communities of earlier times; rather, these have been replaced by large societies that are complex and diverse in their composition. The United States, a prime exemplar, is composed of multiple smaller groups holding characteristics, beliefs, customs, and interests that vary from the rest of society. While there are many cultural universals binding such groups to the mainstream, they also exhibit significant cultural diversity. Some of these groups display no clear boundaries demarcating them from the rest of society and fail to achieve any degree of permanence. Yet those that do, and that also share a distinctive set of norms, values, and behavior setting them off from the dominant culture, are considered subcultures. Subcultures can be organized around age, ethnicity, occupation, social class, religion, or lifestyle and usually contain specific knowledge, expressions, ways of dressing, and systems of stratification that serve and guide its members (Thornton 1997). Distinctive subcultures within the United States include jazz musicians, gangs, Chicanos, gay, college athletes, and drug dealers. While it was once hypothesized that these subcultures would merge together in a ‘‘melting pot,’’ incorporating a mix
of the remnants of former subcultures (Irwin 1970), trends suggest that they resist total assimilation and retain their cultural diversity and distinct identity.
Some subcultures diverge from the dominant culture without morally rejecting the norms and values with which they differ. Others are more adamant in their condemnation, clearly conflicting with or opposing features of the larger society. Milton Yinger first proposed, in 1960, to call these contracultures, envisioning them as a subset of subcultures, specifically, those having an element of conflict with dominant norms, values, or both (Yinger 1960). Indeed, the feature he identified as most compelling about a contraculture is its specific organization in opposition to some cultural belief(s) or expression(s). Contracultures often arise, he noted, where there are conflicts of standards or values between subcultural groups and the larger society. Factors strengthening the conflict then strengthen the contracultural response. Contraculture members, especially from such groups as delinquent gangs, may be driven by their experiences of frustration, deprivation, or discrimination within society.
Yinger’s conceptualization, although abstract and academic at first, came to enjoy widespread popularity with the advent of the 1960s and the student movement. Here was the kind of contraculture he had forecast, and his ideas were widely applied to the trends of the time, albeit under another label. Most analysts of contracultures preferred the term counterculture, and this soon overtook its predecessor as the predominant expression. In 1969 historian Theodore Roszak published his The Making of a Counter Culture, claiming that a large group of young people (ages fifteen to thirty) had arisen who adamantly rejected the technological and scientific outlook characteristic of Western industrialized culture, replacing this, instead, with a humanistic/mysticist alternative. In a more recent update, Roszak (1995) reflected back on that time, further locating the counterculture phenomenon as an historical aberration that arose out of the affluence of post-World War II America. Kenneth Keniston (1971) described this counterculture as composed of distinct subgroups (radicals, dropouts, hippies, drug users, communards, or those living in communes) rising from the most privileged children of the world’s wealthiest nation. Jack Douglas (1970) also discussed the social,
459

COUNTERCULTURES
political, and economic background to this movement and its roots in members’ entrenchment in the welfare state and the existing youth and student cultures. While this movement was clearly political as well, Douglas outlined some of its social dimensions, including rejection of the workaday world and its idealization of leisure, feeling, openness, and antimaterialism. Richard Flacks (1971) and Fred Davis (1971) followed with descriptions of the counterculture’s overarching lifestyles, values, political beliefs, and ideologies. Ralph Turner
(1976), Nathan Adler (1972), and Erik Erikson
(1968) explored the social psychological implications of this counterculture, positing, respectively, a transformation in the self from ‘‘institution’’ to ‘‘impulse,’’ the rise of an antinomian personality, where individuals oppose the obligatoriness of the moral law, and the formation of the negative identity. John Rothchild and Susan Berns Wolf (1976) documented the vast extension of countercultural outposts around the country and their innovations in child rearing. Charles Reich (1970) emphatically stated that this counterculture, consisting mostly of students, was being reinforced by merging with nonstudent youth, educated labor, and the women’s movement, already effecting a major transformation in Western laws, institutions, and social structure. There was strong belief that this movement would significantly and permanently alter both society and its consciousness (Wuthnow 1976). After researching one commune in depth, Berger (1981) later mused about the survival of the counterculture, acknowledging its failure to meet earlier expectations, yet examining how its ideals and values become incorporated into the mainstream culture (cf. Spates 1976).
Other subcultural analysts noted more broadly that these groups are typically popular among youth, who have the least investment in the existing culture, and that, lacking power within society, they are likely to feel the forces of social control swiftly moving against them, from the mass media to police action. Countercultures were further differentiated from subcultures by the fact that their particular norms and values, were not well integrated into the dominant culture, generally known among group members, and other mainstream subcultures.
Yinger reclaimed theoretical command of the counterculture concept with his reflective expansions on the term in a presidential address for the
American Sociological Association (Yinger 1977) and a book that serves as the definitive statement on the topic (1982). He asserted the fundamental import of studying these sharp contradictions to the dominant norms and values of a society as a means of gaining insight into social order.
Countercultures, through their oppositional culture (polarity, reversal, inversion, and diametric opposition), attempt to reorganize drastically the normative bases of social order. These alternatives may range from rejecting a norm or value entirely to exaggerating its emphasis in their construction of countervalues. As a result, some countercultures fade rapidly while others become incorporated into the broader cultural value system. Examples of countercultural groups would include the 1960s student counterculture (in both its political and social dimensions); youth gangs (especially delinquent groups); motorcycle gangs (such as the Hell’s Angels); revolutionary groups (the Weathermen of the Students for a Democratic Society, the Black
Panthers, millenialists [Williams 1996]); terrorist organizations (such as the Symbionese Liberation
Army); extremist racist groups (the Ku Klux Klan, skinheads [Baron 1997; Hamm 1995; Young and
Craig 1997] , the Aryan Nation); survivalists (Branch
Davidians); punkers; bohemian Beats; ‘‘straight edgers;’’ Rainbow family; Earth First! (Lange 1990;
Short 1991) and some extreme religious sects (such as the Amish and the Hare Krishnas [see
Saliba 1996]).
VARIETIES OF COUNTERCULTURES
Yinger believed that countercultural groups could take several forms. The radical activist counterculture ‘‘preaches, creates, or demands new obligations’’ (Yinger 1977, p. 838). They are intimately involved with the larger culture in their attempts to transform it. Members of the communitarian utopian counterculture live as ascetics, withdrawing into an isolated community forged under the guidelines of their new values. Mystical countercultures search for the truth and for themselves, turning inward toward consciousness to realize their values. Theirs is more a disregard of society than an effort to change it. These three forms are not necessarily intended to describe particular groups. Rather, they are ideal types, offered to shed insights into characteristics or tendencies groups may combine or approximate in their formation.
Hippie communities or bohemian Beat groups
460

COUNTERCULTURES
combined the mystical and utopian features of countercultures in their withdrawal from conventional society and their search for a higher transcendence. Revolutionary youth gangs, such as the
1960s radicals, the Hell’s Angels, and the punkers, fuse the mystical search for new experiences and insights (often through drug use) with an activist attack on the dominant culture and its institutional expressions. Survivalists, Amish, and Hare Krishnas fuse the radical critique of conventional values and lifestyles with a withdrawal into an isolated and protected community.
Countercultures can be differentiated by their primary breaks with the dominant culture. Some take odds with its epistemology, or the way society contends that it knows the truth. Hippies and other mystics, for example, have tended to seek insight in homespun wisdom, meditation, sensory deprivation, or drugs, rejecting the rationality of science and technology. Others assert an alternative system of ethics, or the values pursued in defining good or striving for the good life. Some, like skinheads or KKK members, may be quite conservative in their definition of the good life; others are libertarian, advocating for people to
‘‘do their own thing.’’ Still other countercultures offer alternative aesthetic standards by which fashion, taste, and beauty are judged. Punk or acid rock musical movements, performance or postmodernist art movements, and bohemian or hippie fashion movements were all aesthetic statements that incorporated a radical rejection of the standards of conventional taste and its connection to conventional values. Thus, entire countercultural movements may be based on their advocacy of these competing beliefs.
COUNTERCULTURES AND SOCIAL
CHANGE
Due to their intense opposition to the dominant culture, countercultures are variously regarded as ‘‘engines of social change, symbols and effects of change, or mere faddist epiphenomena’’ (Yinger
1982, p. 285). Examining these in reverse order, countercultures are often considered mutations of the normative social order, encompassing such drastic lifestyle changes that they invoke deep ambivalence and persecution. Most major countercultural mutations appear in the form of
religious movements. Other countercultures arise out of underlying or developing societal stress: rapid political or economic change; demographic transformations in the population (age, gender, location); a swift influx of new ideas; drastic escalation or diminishment of hopes or aspirations; weakening of ties to primary support circles (families, neighborhoods, work groups); and the erosion of meaning in the deepest symbols and rituals of society. These factors are then augmented by communication among people sharing such experiences or beliefs, leading them to coalesce into normatively and ideologically integrated groups. Countercultures can also precipitate social change if the norms and values they champion are incorporated into the mainstream. In commenting on the 1960s student movement, Chief Justice Warren Burger of the United States Supreme Court stated that ‘‘the turbulent American youth, whose disorderly acts [I] once ‘resented,’ actually had pointed the way to higher spiritual values’’ (cited in Yinger 1977, p. 848). Lasting influence may not always result from major countercultural movements, as witnessed by the rapid erosion in influence of Mao’s cultural revolution after his death, yet it is possible. This occurs through a cultural dialectic, wherein each existing system, containing antithetical, contradictory ideas, gives rise to the oppositional values of a counterculture. These are ultimately incorporated into a future new order.
COUNTERCULTURE CASE STUDIES
While the student movement of the 1960s was undoubtedly the largest and most influential counterculture to arise in the United States, a review of three more contemporary American countercultures may yield further insight into the parameters and character of these movements. Let us focus on the Hare Krishnas, punks, and survivalists.
The Hare Krishna movement, also known as the International Society for Krishna Consciousness (ISKCON), is one of the religious movements that became popular in the United States during the great ‘‘cult’’ period of the 1970s (Judah 1974; Rochford 1985). Its rise after the decline of the 1960s student movement is not coincidental, for many people who were former hippies or who were influenced by or seeking the ideals and values of the 1960s turned toward new religions (Tipton
461
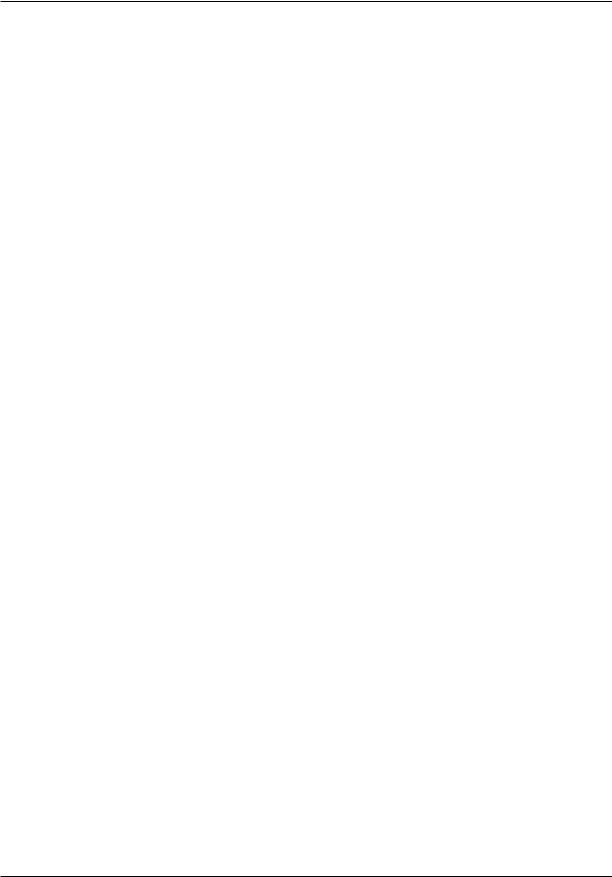
COUNTERCULTURES
1982) in search of the same features of community, idealism, antimaterialism, mysticism, transcendence to a higher plane, and ‘‘a spiritual way of life, which stands outside the traditional institutions found in America’’ (Rochford 1985, p. 44).
Its primary values conflicting with mainstream culture include the rejection of (1) material success through competitive labor; (2) education to promote that end; (3) possessions for sense gratification; (4) authority favoring the status quo; (5) imperialistic aggression; and (6) the hypocrisy of racial discrimination (Judah 1974, p. 16). After the death of its American spiritual master, Srila Prabhupada, in 1977, however, the movement peaked and became more commercialized, transferring its emphasis from self-expression and uniqueness perpetuation of the sect, thereby becoming more of a mass phenomenon.
In contrast to the religious and value components of the Hare Krishnas’ rejection of mainstream culture, the punk or punk rock counterculture of the late 1970s and early 1980s was more of a style movement (Hebdige 1981). As Fox (1987, p. 349) has noted, ‘‘The punks created a new aesthetic that revealed their lack of hope, cynicism, and rejection of societal norms.’’ This was expressed in both their appearance and their lifestyle. The punk belief system was antiestablishment and anarchistic, celebrating chaos, cynicism, and distrust of authority. Punks disdained the conventional system, with its bureaucracies, power structures, and competition for scarce goods (Fox 1987). Members lived outside the system, unemployed, in old abandoned houses or with friends, and engaged in heavy use of drugs such as heroin and glue. Hardcore commitment was usually associated with semipermanent alteration of members’ appearance through tattoos, shaven heads, or Mohawk hairstyles (Brake 1985). The musical scene associated with punks was contrary to established tastes as well and often involved self-abandonment characterized by ‘‘crash dancing’’ (Street 1986).
In contrast to the hippies, Krishnas, and punks, the survivalist counterculture was grounded in exaggeration of right-wing beliefs and values. While some of the former groups preached love, survivalists were characterized by hate. Formed out of extremist coalition splinter groups such as neoNazis, the KKK, the John Birch Society, fundamentalist Mormon Freemen, the White Aryan Resistance, and tax protesters from Posse Comitatus,
survivalists drew on long-standing convictions that an international conspiracy of Jews was taking over everything from banking, real estate, and the press to the Soviet Politburo, and that the white race was being ‘‘mongrelized’’ by civil-rights legislation. A cleansing nuclear war or act of God, with ‘‘secular’’ assistance, would soon bring the Armageddon, eradicating the ‘‘Beast’’ in their midst (Coates 1987). Members thus set about producing and distributing survivalist literature, stockpiling machine guns, fuel, food, and medical supplies on remote farms and in underground bunkers, joining survivalist retreat groups, and attending survivalist training courses (Peterson 1984). Within their retreat communities they rejected the rationalization, technologization, secularization, and commodification of society, creating an environment of creative self-expression where an individual could accomplish meaningful work with a few simple tools. In their withdrawn communities and ‘‘utopian’’ future scenario, men would reclaim their roles as heads of the family; women would regain mastery over crafts and nurturance. Theirs is thus a celebration of fantasy and irrationality (Mitchell, n.d.). Yet while they isolate themselves in countercultures composed of like-minded individuals, they try to influence mainstream society through activism in radical right-wing politics as well. Their actions and beliefs, although rejecting the directions and trends in contemporary society, arise out of and represent frustrations felt by embattled segments of the Moral Majority (mainly fundamentalist Christian, white groups).
Scholarly treatment of counterculture movements is not limited to the United States. In the field of new social movements research, many European scholars have looked at organizations that are designed to mobilize forces against nationalistic cultures. These studies, ranging in topics from nuclear weapons, ecology, squatters’ rights, gays, women, and other countercultural groups (i.e., Autonomen or terrorist organizations), have explored the common denominators inherent in all new social movements. Using quantitative data from protest events collected from newspaper sources in France, the Netherlands, Germany, and Switzerland, Hanspeter Kriesi and others (1995) outlined the ‘‘new cleavage’’ that exists in these Western European societies.
Countercultures thus stand on the periphery of culture, spawned by and spawning social trends
462

COUNTERCULTURES
and changes [by their opposition to dominant culture]. As Yinger (1977, p. 850) noted, ‘‘Every society gets the countercultures it deserves, for they do not simply contradict, they also express the situation from which they emerge. . . .
Countercultures borrow from the dominant culture even as they oppose it.’’
(SEE ALSO: Alternative Lifestyles; Social Movements; Student
Movements)
REFERENCES
Adler, Nathan 1972 The Underground Stream: New Life Styles and the Antinomian Personality. New York: Harper.
Baron, Stephen W. 1997 ‘‘Canadian Male Street Skinheads: Street Gang or Street Terrorists?’’ Canadian Review of Sociology and Anthropology 34:151–174.
Berger, Bennett 1981 The Survival of a Counterculture. Berkeley, Calif.: University of California Press.
Brake, Michael 1985 Comparative Youth Culture: The Sociology of Youth Culture and Subcultures in America, Britain, and Canada. London: Routledge.
Coates, James 1987 Armed and Dangerous. New York: Hill and Wang.
Davis, Fred 1971 On Youth Subcultures: The Hippie Variant. New York: General Learning Press.
Douglas, Jack D. 1970 Youth in Turmoil. Chevy Chase, Md.: National Institute of Mental Health.
Erikson, Erik 1968 Identity: Youth and Crisis. New York: W. W. Norton.
Flacks, Richard 1971 Youth and Social Change. Chicago:
Markham.
Fox, Kathryn J. 1987 ‘‘Real Punks and Pretenders: The Social Organization of a Counterculture.’’ Journal of Contemporary Ethnography 16:344–370.
Hamm, Mark 1993 American Skinheads. Westport, Conn.:
Praeger.
Hebdige, Dick 1981 Subcultures: The Meaning of Style. New York: Methuen.
Irwin, John 1970 ‘‘Notes on the Present Status of the Concept Subculture.’’ In D.O. Arnold, ed., The Sociology of Subcultures. Berkeley, Calif.: Glendessary Press.
Judah, J. Stillson 1974 Hare Krishna and the Counterculture. New York: Wiley.
Keniston, Kenneth 1971 Youth and Dissent: The Rise of a New Opposition. New York: Harcourt Brace Jovanovich.
Kriesi, Hanspeter, Ruud Koopmans, Jan Willem Dyvendak, and Marco G. Giugni 1995 New Social Movements in
Western Europe. Minneapolis: University of Minnesota Press.
Lange, Jonathan I. 1990 ‘‘Refusal to Compromise: The Case of Earth First!’’ Western Journal of Communication 54:473–494.
Mitchell, Richard n.d. Dancing at Armageddon: Doomsday and Survivalists in America. Unpublished paper, Oregon State University.
———1991 ‘‘Secrecy and Disclosure in Fieldwork.’’ In W. Shaffir and R. Stebbins, eds., Experiencing Fieldwork. Newbury Park, Calif.: Sage.
Peterson, Richard G. 1984 ‘‘Preparing for Apocalypse: Survivalist Strategies.’’ Free Inquiry in Creative Sociology 12:44–46.
Reich, Charles A. 1970 The Greening of America. New York: Random House.
Rochford, E. Burke 1985 Hare Krishna in America. New Brunswick, N.J.: Rutgers University Press.
Roszak, Theodore 1969 The Making of a Counter Culture. New York: Doubleday.
——— 1995 ‘‘Introduction to the 1995 Edition.’’ In Theodore Roszak The Making of a Counter Culture. Berkeley, Calif.: University of California Press.
Rothchild, John, and Susan Berns Wolf 1976 The Children of the Counter-Culture. New York: Doubleday.
Saliba, John A. Understanding New Religious Movements. Grand Rapids, Mich.: William B. Eerdmans Press.
Short, Brant 1991 ‘‘Earth First! And the Rhetorics of Moral Confrontation.’’ Communication Studies 42:172– 198.
Spates, James L. 1976 ‘‘Counterculture and Dominant Culture Values: A Cross-National Analysis of the Underground Press and Dominant Culture Magazines.’’ American Sociological Review 41:868–883.
Street, J. 1986 Rebel Rock: The Politics of Popular Music. Oxford: Basil Blackwell.
Thornton, Sarah 1997 ‘‘General Introduction.’’ In Ken Gelder and Sarah Thornton, eds., The Subcultures Reader. London: Routledge.
Tipton, Steven M. 1982 Getting Saved from the Sixties. Berkeley, Calif.: University of California Press.
Turner, Ralph 1976 ‘‘The Real Self: From Institutions to Impulse.’’ American Journal of Sociology 81:989–1016.
Williams, Rhys H. ‘‘Politics, Religion and the Analysis of Culture.’’ Theory and Society 25:883–900.
Wuthnow, Robert 1976 The Consciousness Revolution. Berkeley, Calif.: University of California Press.
Yinger, J. Milton 1960 ‘‘Contraculture and Subculture.’’
American Sociological Review 25: 625–635.
463