
Encyclopedia of SociologyVol._4
.pdf
SCIENTIFIC EXPLANATION
foundation for science (Keat and Urry, 1982, p. 16). One of the goals of science is to develop and test theories, although some scientists believe that science proceeds inductively, purely by amassing facts and building theories from the amassed data.
Scientific laws fall broadly into two types: deterministic laws and stochastic (probabilistic) laws. For deterministic laws, if the scientist knows the initial conditions and the forces acting on a system and those factors do not change, the state of the system can be determined for all times and places. Deterministic laws are the ideal of the Newtonian, or mechanistic, model of science. In this model, it is assumed that causes precede effects and that changes come only from the immediately preceding or present state, never from future states. It is assumed that if two systems are identical and are subject to the same initial conditions and forces, they will reach the same end point in the same way. Deterministic laws assume that it is possible to make a complete separation between the system and the environment and that the properties of the system arise from its smallest parts. The smallest parts of a system are those about which nothing can be determined except their location and direction. There is nothing in the parts themselves that influences the system, and all changes in the state of the system come from the forces acting on it. Deterministic laws are based on the assumption that the universe is regular and that connections between events are independent of time and space. The idea with a scientific explanation is that all other things being equal (ceteris paribus), identical circumstances lead to identical results.
Stochastic laws are expressed in terms of probability. For large or complex systems, it is not possible to identify precisely what state the system will be in at any given time but only to assess the probability of its being in a certain state. Quantum physics, chemistry, card games, and lotteries utilize stochastic laws. Those laws are stated in terms of probability over time and apply to classes of events rather than to specific instances. Most relationships in the social sciences are stated in stochastic terms because individual behavior is very difficult to predict. The use of probability does not mean that events are viewed as random, or uncaused, simply that the behavior of the individual elements of a system cannot be predicted with perfect accuracy.
Scientific theories are systematically linked to existing knowledge that is derived from other generally accepted theories. Each scientist builds on the work of other scientists, using tested theories to develop new theories. The scientific method is dedicated to changing theories, and scientific knowledge progresses through the challenge and revision of theories.
Often a new theory is preferred not because it is based on facts (data) that are different from those on which the old theory was based but because it provides a more comprehensive explanation of existing data. For example, Newton’s theory of the solar system superseded Kepler’s explanation of planetary motion because Newton’s theory included the theory of gravity (which predicted a gravitational attraction between all physical bodies in the universe) as well as the laws of motion. The two theories together provided many circumstances that could ‘‘test’’ the theory because they predicted not only where planets should be in relation to each other at given times but also phenomena such as falling apples and swinging pendulums. Newton’s theory was more comprehensive and more economical, and although it provided more opportunities for falsification than did Kepler’s (which made it more vulnerable), it also resisted falsification better and became the accepted scientific explanation (Chalmers 1982).
The premises, or propositions, in a scientific theory must lead logically to the conclusions. Scientific explanations show that the facts, or data, can be deduced from the general theory. Theories are tested by comparing what deduction says ‘‘should’’ hold if the theory is true with the state of affairs in the world (observations). The purpose of a theory is to describe, explain, and predict observations.
The classic example of deductive logic is the familiar syllogism ‘‘All men are mortal; Socrates is a man; therefore, Socrates is mortal.’’ Deductive conclusions include only the information included in the propositions. Thus, deductive reasoning can be logically correct but empirically incorrect. If a theory is based on empirically false premises, it probably will result in empirically false conclusions. A scientific test of the truth of the conclusions requires a comparison of the statements in the conclusion with actual states of affairs in the ‘‘real’’ world.
2465
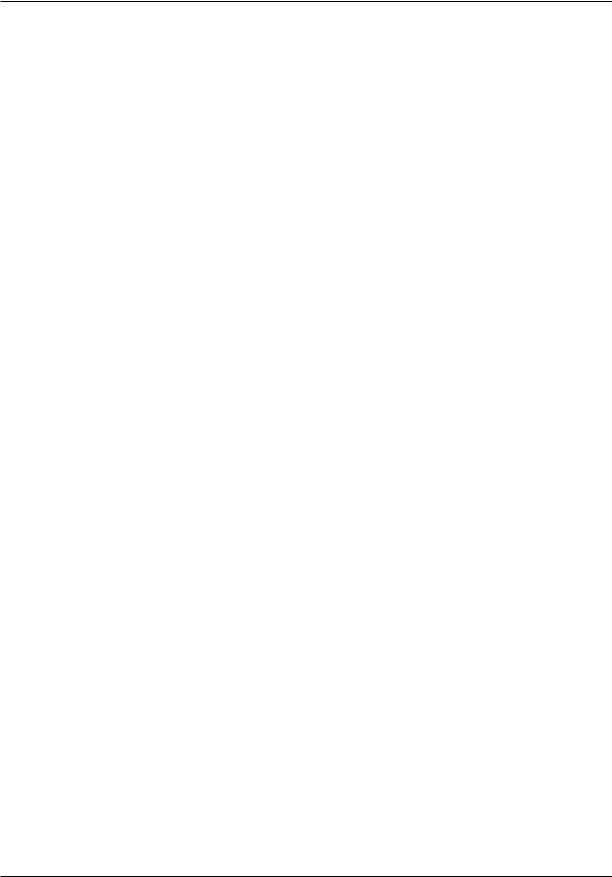
SCIENTIFIC EXPLANATION
Scientific explanations and theories are usually quite complex and thus often require more information than can be included in a deductively valid argument. Sometimes it is necessary to know that a conclusion is probably true, or at least justified, even if it does not follow logically from a set of premises and arguments (Giere 1984). Thus, there is a need for inductive logic, which is based on particular instances (facts or observations) and moves to general theories (laws).
Many sociologists and other scientists believe that scientific knowledge is produced mainly by induction (Glaser and Strauss 1967). For example, after one has observed many politicians, a theory might postulate that most politicians are crooked. Although this theory is based on many observations, its proof, or verification, would require observing every politician past, present, and future. Falsifying the theory would require finding a substantial number of politicians who were not crooked. The absolute and final verification of scientific theories is not possible. However, it should be possible to ‘‘falsify’’ any scientific theory by finding events or classes of events that do not support it (Stinchcombe 1987; Popper 1959).
Because inductive arguments are always subject to falsification, they are stated in terms of probabilities. Good inductive arguments have a high probability associated with their being true. This high probability comes from a large number of similar observations over time and in different circumstances. For example, although it is not absolutely certain that if someone in North America becomes a medical doctor, he or she will earn a high income, the evidence provided by observing doctors in many places and many times shows that a high probability can be assigned to the assertion that medical doctors earn high incomes.
Inductive arguments are not truth-preserving. Even with true premises, an inductive argument can have a false conclusion because the conclusions of inductive arguments generally contain more information or make wider generalizations than do the premises (Giere 1984). Science requires both deductive and inductive methods to progress. This progress is circular: Theories are developed and tested, and new data give rise to new theories, which then are tested (Wallace 1971).
Several steps are involved in testing scientific theories. Theories first must be expressed in both
abstract, verbal terms and concrete, operationalized terms. Concepts and constructs are rich, complex, abstract descriptions of the entity to be measured or studied. Concepts have nominal definitions (they are defined by using other words) and are specifically developed for scientific purposes. A variable is operationally defined to allow the measurement of one specific aspect of a concept. Operationalization is a set of instructions for how a researcher is going to measure the concepts and test the theory. These instructions should allow events and individuals to be classified unambiguously and should be precise enough that the same results will be achieved by anyone who uses them (Blalock 1979).
For example, one theory posits that the relationship between ‘‘anxiety’’ and test performance is curvilinear. This theory predicts that very little anxiety leads to poor performance on tests (as measured by grades), a medium amount of anxiety improves test performance, and very high anxiety causes poor test performance. If it were drawn on a graph, this curve would be an upside-down U. To test the theory, both anxiety and test performance must be measured as variables expressed in empirical terms. For an observation to be empirical means that it is, or hypothetically could be, experienced or observed in a way that can be measured in the same manner by others in the same circumstances.
As a concept, anxiety encompasses many different things. The measurement theory must specify whether anxiety will be measured as feelings, such as being tense, worried, or ‘‘uptight,’’ or as physical reactions, such as shortness of breath, heart palpitations, or sweaty palms. The researcher may decide to measure anxiety by asking subjects how worried or tense they felt before an examination. Racing hearts, sweating palms, and upset stomachs are part of the concept, but they are excluded from the operationalization. The researcher must decide whether this is or is not a valid (measures what it purports to measure) and reliable (obtains the same results on repeated tests) measure of anxiety, in part by comparing the results of the research to other research on anxiety and test performance. It is also necessary to strike a balance between the scope of the concept (the different things it refers to) and precision. The wider the scope of a concept, the more it can be generalized to other conditions and the fewer
2466
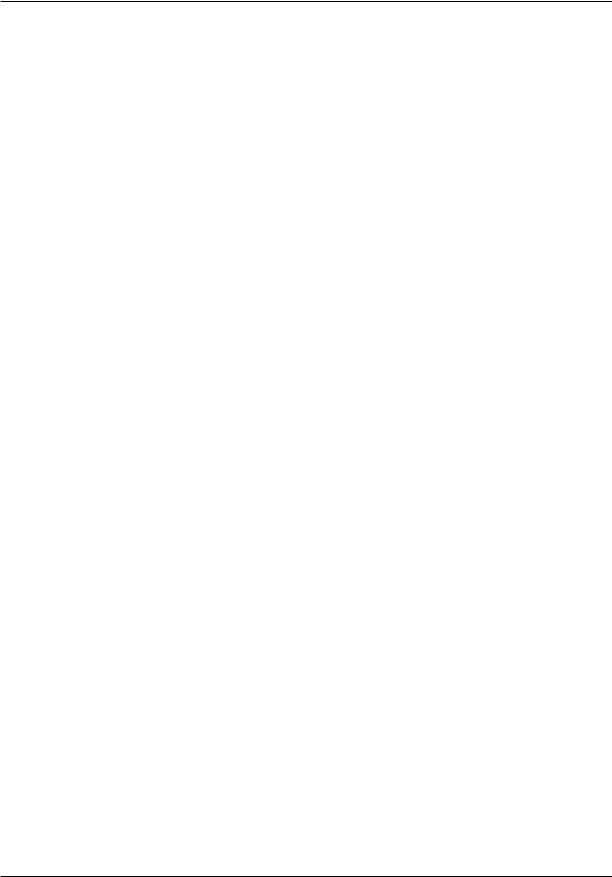
SCIENTIFIC EXPLANATION
conditions are required to construct a theory, making it more parsimonious. However, if the scope of a concept is too wide, the concept loses precision and becomes meaningless.
Scientific explanation involves the accurate and precise measurement of phenomena. Measurement is the assignment of symbols, usually numbers, to the properties of objects or events (Stevens 1951). The need for precise measurement has led to an emphasis on quantification. Some sociologists feel that some qualities and events that people experience defy quantification, arguing that numbers can never express the meaning that people’s behavior holds for them. However, mathematics is only a language, based on deductive logic, that expresses relationships symbolically. Assigning numbers to human experiences forces a researcher to be precise even when the concepts, such as ‘‘anxiety’’ and ‘‘job satisfaction,’’ are fuzzy.
Another important aspect of scientific explanations is that they attempt to be ‘‘objective.’’ In science this term has two broad meanings. First, it means that observers agree about what they have observed. For example, a group of scientists observing the behavior of objects when they are dropped would agree that they saw the objects ‘‘fall’’ to the ground. For this observation to be objective, (1) there must be an agreed-on method for producing it (dropping an object), (2) it must be replicable (more than one object is released, and they all ‘‘fall’’), and (3) the same results must occur regardless of who performs the operation and where it is performed (objects must behave the same way for all observers anywhere in the world). Scientific operations must be expressed clearly enough that other people can repeat the procedures. Only when all these conditions are met is it possible to say that an observation is objective. This form of objectivity is called ‘‘intersubjectivity’’ and it is crucial to scientific explanations.
The second use of the word ‘‘objective’’ in science means that scientific explanations are not based on the values, opinions, attitudes, or beliefs of the researcher. In other words, scientific explanations are ‘‘value-free.’’ A researcher’s values and interests may influence what kinds of things she or he chooses to study (i.e., why one person becomes a nuclear physicist and another becomes a sociologist), but once the problem for study is chosen the
scientist’s personal values and opinions do not influence the type of knowledge produced. The value-free nature of science is the goal of freeing scientific explanations from the influence of any individual or group’s biases and opinions.
The relationships in a theory state how abstract constructs are to be linked so that antecedent properties or conditions can be used to explain consequent ones. An antecedent condition may be seen as either necessary or sufficient to cause or produce a consequent condition. For example, higher social status may be seen as sufficient to increase the probability that farmers will adopt new farming techniques (innovation). It also could be argued that awareness and resources are necessary conditions for innovation. Without both, innovation is unlikely (Gartrell and Gartrell 1979).
Relationships may be asymmetrical (the antecedent produces the effect) or symmetrical (both cause each other): Frustration may cause aggression, and aggression may cause frustration. Relationships may be direct, or positive (an increase in knowledge causes an increase in innovation), or negative (an increase in stress leads to a decrease in psychological well-being). They may be described as monotonic, linear, or curvilinear. Sociologists often assume that relationships are linear, partly because this is the simplest form of a relationship.
Relationships between variables are expressed by using a wide variety of mathematical theories, each of which has its own ‘‘language.’’ Algebra and calculus use the concepts of ‘‘greater than,’’ ‘‘less than,’’ and ‘‘equal to.’’ Set theory talks about things being ‘‘included in,’’ and graph theory uses ‘‘connectedness’’ or ‘‘adjacency between.’’ Markov chains attempt to identify a connectedness in time or a transition between states, and symbolic logic uses the terms ‘‘union’’ and ‘‘intersection’’ to talk about relationships.
Scientific explanation is also very explicit about the units to which relationships between propositions refer. Sociologists refer to a host of collectivities (cultures, social systems, organizations, communities), relationships (world systems, families), and parts of collectivities (social positions, roles). There is strength in this diversity of subject matter but also potential weakness in failing explicitly to define the unit of analysis. Some properties cannot be attributed to all units of analysis. For example, ‘‘income’’ is a concept that can apply to an individ-
2467
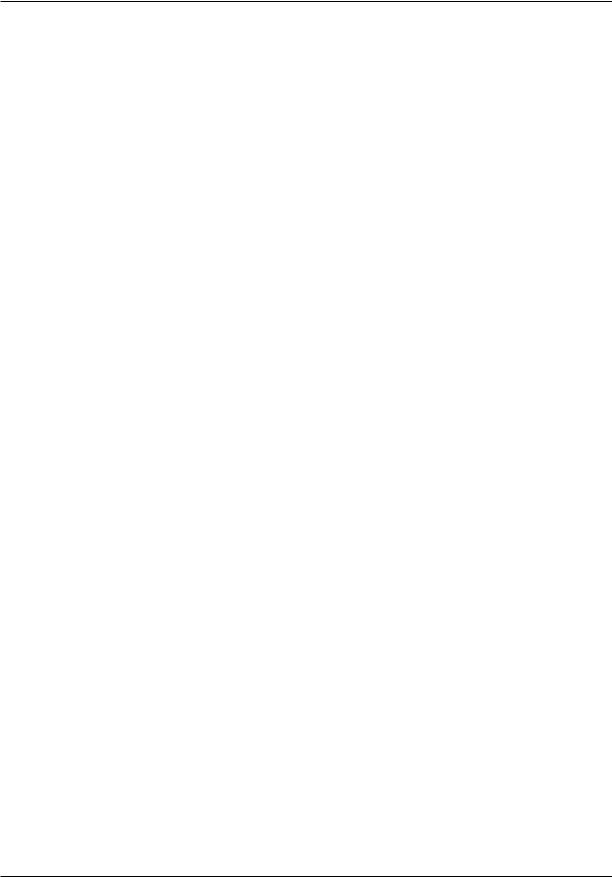
SCIENTIFIC EXPLANATION
ual or a group (e.g., ‘‘average’’ income), but ‘‘inequality’’ is always a property of an aggregate. The ‘‘ecological fallacy’’ (Hannan 1970) involves the incorrect attribution of properties of aggregates to individuals. Aggregation is not a matter of simple addition, and some relationships between subunits (homogeneity, complexity, inequality) have complicated aggregation algorithms. Care must be taken in switching units of reference from social collectivities to individuals. For example, communities with high divorce rates also may have high homicide rates, but this does not necessarily imply that divorced people kill one another or are more likely to be homicide victims or perpetrators.
To test theories, the relationships among concepts are stated as hypotheses, linking variables in an operationalized form. Since the existence of a relationship cannot be proved conclusively, a scientist instead tries to show that there is no relationship between the variables by testing hypotheses that are stated in the ‘‘null’’ form. In the test performance and anxiety example, a null hypothesis would state, ‘‘There is no curvilinear relationship between the number of correct responses on tests and the reported level of worry and tension.’’ If this hypothesis was rejected, that is, found to be highly unlikely, the researcher would have evidence to support the alternative hypothesis suggested by the theory: There is a curvilinear relationship between the variables.
Social scientists use a variety of methods to study human behavior, including experiments, surveys, participant observation, and unobtrusive measures. In essence, experiments try to identify causal sequences by determining the effect of an independent variable (the stimulus) on a dependent variable. Experiments require stringent conditions that often are difficult to fulfill with human beings, sometimes for ethical reasons but more often because there is a wide variation in individual responses to the same stimulus (Babbie 1989; Kerlinger 1973; Cook and Campbell 1979).
Social scientists have developed other research methods, such as surveys and field research, which allow them to produce scientific knowledge without resorting to experimental manipulation. Statistical analysis of survey data allows social scientists to examine complex problems in large populations by statistically controlling several variables that represent competing explanations (Blalock
1964). The distinctive characteristic of survey research is that the subjects of the study tell the scientist about themselves.
Social scientists also use qualitative methods such as participant observation to conduct research in the ‘‘field’’ where phenomena actually occur. Field research focuses on the empirical richness and complexity of the whole subject in order to understand what is subjectively meaningful. Participant observation proceeds inductively rather than deductively. The researcher observes and participates in order to understand (subjectively) and then attempts to externalize the observations by constructing categories of responses, or theory. In contrast to other research designs, participant observation deliberately does not attempt to control conditions; the researcher strives to obtain an unbiased picture of how the subjects see things in their natural setting (Whyte 1961). The emphasis is on the richness of subjects’ understanding of events and on subjectivity rather than on objectivity. Theory developed from this type of research is called grounded theory (Glaser and Strauss 1967). Unobtrusive methods such as content analysis focus on the study of artifacts (newspapers, homes), partly to overcome reactivity by subjects and biases on the part of the researcher.
CRITIQUES OF THE HYPOTHETICODEDUCTIVE MODEL OF SCIENCE
In the 1930s and 1940s, the dominant view of science was ‘‘radical positivism,’’ which viewed science as a process based only on inductive generalizations and empirical verification. Abstract theoretical concepts that could not be observed were considered literally meaningless. The revision of positivism in the 1950s (logical empiricism) recognized the importance of abstract concepts and theories but continued to insist that all scientific statements be subject to empirical falsification. In short, the empiricists persisted in their belief that ‘‘facts’’ were purely objective entities and that what was viewed as a fact did not depend on theory or theoretical concepts. However, theories play as large a role in scientific change and knowledge production as do empirical observations. In part, this internal confusion laid the groundwork for a wide range of critiques of both positivism and empiricism (Alexander 1982; Bernstein 1976).
2468

SCIENTIFIC EXPLANATION
Reconstructed logic suggests that scientific knowledge can be accounted for by following formal rules of logic. The progress of knowledge is such that unscientific or prescientific explanations for phenomena are replaced successively by scientific explanations, which are ever closer approximations to the ‘‘truth.’’ It stresses that the knowledge produced by the scientific method is objective and value-free, corresponding to states of the world as it really is, not as it is seen by a particular group of people in a particular social and historical location.
However, the ‘‘facts’’ on which scientific explanations are based are not independent of ‘‘point of view’’ (Polanyi 1958; Hanson 1972). All scientific data are theoretically informed. What is ‘‘fact’’ and what is ‘‘theory’’ are what is convenient to the focus of scientific attention at a particular time. Because science is a social and cultural activity, it is grounded in an everyday, taken-for-granted reality. Scientists can perceive ‘‘facts’’ only in a particular social and cultural context. Observations take place in a cultural milieu that literally affects what the observer perceives, not just how it is interpreted. The totally objective, theory-free observation aspired to in science is not possible; to ‘‘see’’ something is always to see it ‘‘as’’ something. For example, to observe the medical ‘‘facts’’ in an x- ray, a physician must first learn what parts of the picture to ignore. The ‘‘fact’’ that objects ‘‘fall’’ to the ground is a fact only in a social context in which gravity is an accepted explanation for the behavior of falling objects. Scientific facts are constructed and developed through situated human labor; they do not have an independent, objective existence of their own (Fleck 1979).
Most twentieth-century philosophers of science have assumed that there is something called the scientific method that applies equally to all sciences and that sciences can be judged by their ability to adhere to that method. This is called the ‘‘unity of the sciences’’ model. However, the philosophy of science has ignored the actual behavior of scientists, concentrating instead an reconstructing the logic of science. The result has been an idealized and unrealistic picture of how scientific knowledge is produced. When the actual practice of scientists is observed, it is apparent that in different sciences, scientists reason in a wide variety of modes.
These different modes of reasoning were hidden by the philosophical approach of viewing scientific knowledge as resulting from the simple application of scientific logic to problems. Scientific knowledge is better seen as the outcome of an active, work-oriented process than as an uninvolved description of a ‘‘passive’’ natural world. This means that scientific knowledge production consists largely in activities in which scientists make decisions about how to proceed in different circumstances. This does not imply that scientific knowledge is ‘‘made up’’ and thus completely relative but instead, by looking at scientific practice rather than only scientific logic, that the view of science has shifted from science as a ‘‘representation’’ of nature to science as ‘‘action’’ or ‘‘work’’ (Knorr Cetina 1981).
The most definitive research into how the various sciences produce knowledge differently is represented by the work of the sociologist of science Knorr Cetina (1999). Knorr Cetina has examined the practical activity and ‘‘cultures of knowing’’ of two very different sciences: molecular biology and high-energy physics. She has focused on the ‘‘concrete, mundane, everyday practices of inquiring and concluding through which participants establish, for themselves and for others, knowledge claims’’ (1991, p. 108). Her research shows that what counts as ‘‘scientific method’’ differs radically between these two sciences. In other words, the cultural structure of scientific methodology varies from science to science (1991, p. 107).
Knorr Cetina demonstrates that the epistemic culture in a molecular biology laboratory is such that molecular biologists have to become ‘‘repositories of unconscious experience’’ and individual scientists have to develop an embodied sense of a reasonable response to different situations (1992, p. 119). A practicing molecular biologist literally becomes a measurement instrument. These scientists become highly skilled at seeing things others cannot see, and their bodies learn to perform delicate operations in loading gels and manipulating DNA that cannot be taught, only learned through experience. In their scientific work, individual molecular biologists often have to guess what procedure is best in a given situation. For this reason, the sense of what counts as a successful procedure depends heavily on an individual’s experience and the predictive ability ‘‘which indi-
2469
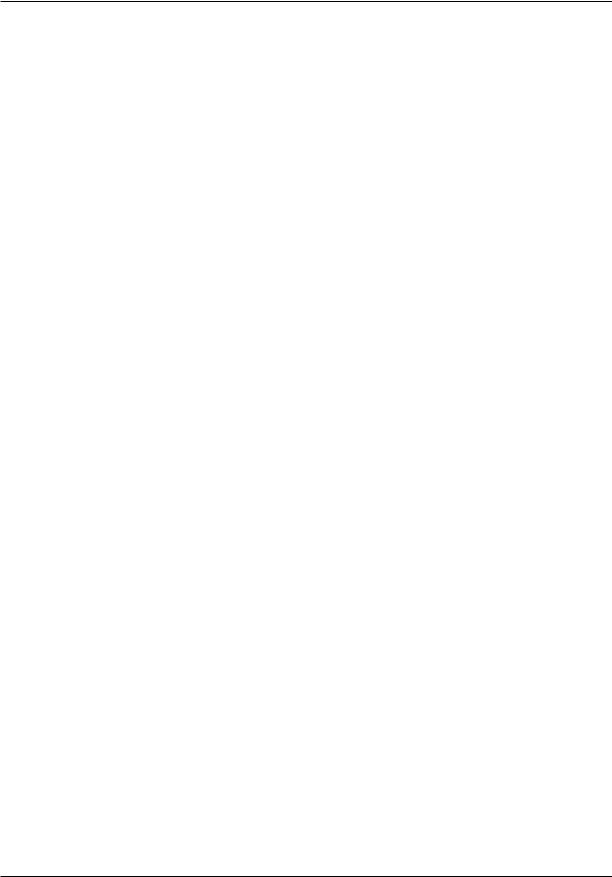
SCIENTIFIC EXPLANATION
viduals must somehow synthesize from features of their previous experience, and which remains implicit, embodied, and encapsulated within the person’’ (1992, p. 121). What counts as a successful procedure or as proper scientific method is implicit: It is a blend of the individual’s experience and the culture in the laboratory. Knorr Cetina calls this kind of reasoning ‘‘biographical’’ because ‘‘it is sustained by a scientist’s biographical archive and the store of his or her professional experience’’ (1991, p. 115).
In contrast to the highly individual and personalized culture of knowing in a molecular biology laboratory, high-energy physics laboratories are very different kinds of epistemic spaces. Their organization is best compared to that of a superorganism, such as highly organized colonies of bees, ants, or termites. High-energy physics involves more circularities and contingencies than does molecular biology; its experiments are long term and ‘‘supra-individual.’’
In high-energy physics (HEP) experiments, the work of producing knowledge is detached from the individual scientist and shifted to the group. These experiments can involve from 200 to 2,000 individuals from 200 different institutions around the world, all focused on a common goal, for up to twenty years (Knorr Cetina 1999, p. 160). Authorship belongs to the experiment as a whole; individual scientists feel that they are representatives of the whole, and there is a sense of collective responsibility among them. (Knorr Cetina 1995). Unlike the highly trained body and eyes of a molecular biologist, data interpretation in HEP is done not by individual scientists but by computers. In fact, individual scientists literally cannot run experiments. HEP experiments are huge, they take many years to run, and each experiment seeds new generations of experiments. High-energy physicists do not think in terms of individual achievements in months but of group successes over years and decades.
In HEP, forming a consensus about what counts as adequate scientific knowledge and the proper application of scientific method is very much a group process. In molecular biology, the group is involved in terms of the culture of the laboratory but each individual scientist is a highly skilled measuring instrument that makes most procedural decisions on his or her own. Thus, by examining
the organization of the laboratories and the working practices of the scientists in these two domains, Knorr Cetina has challenged the philosophical assumption of a unitary scientific method.
Science is now widely regarded as a social activity rather than an application of logic to nature. It is seen as an interplay between practical activity, empirical observations, and broad theoretical ‘‘paradigms’’ (Kuhn 1970; Fleck 1979). Paradigms dictate the valid questions for research as well as the range of possible answers and can be so powerful that contradictory data (anomalies) are explained away under the assumption that they can be brought into the theory at a later time. Confronted by contradictory empirical evidence that cannot be ignored, the adherents of a theory often develop ad hoc hypotheses and residual categories to account for anomalies. Thus, they encompass or explain observations that contradict their theories and often cling to those theories in dogmatic fashion. The reconstructed logic of science leads one to believe that theories would be rejected under those conditions.
However, sociological research has shown that ‘‘the data’’ do not and cannot speak for themselves and decide between competing scientific theories. Sometimes a theory wins out over its competitors because its survival is in the best interests of a group or researcher (Woolgar 1981; Shapin 1979). For example, when high-energy particle physicists were searching for the subatomic particles now known as quarks, two competing explanations were advanced: the ‘‘charm’’ and ‘‘color’’ theories. Both models were consistent with the data. The ultimate success of the charm model occurred because more people had an interest in seeing it succeed. Charm theorists were more successful in relating their theory to an existing body of practice and interests. The color theory was never empirically refuted but eventually ‘‘died’’ because its proponents were reduced to talking to themselves (Pickering 1982).
Part of the problem is that the decision about whether certain experiments or observations are critical to the proof or falsification of a theory is possible only after the fact, not before, and the possibility always exists that an experiment failed because it was not performed competently. It is difficult to establish the criteria for determining whether an experiment has been successful. To
2470
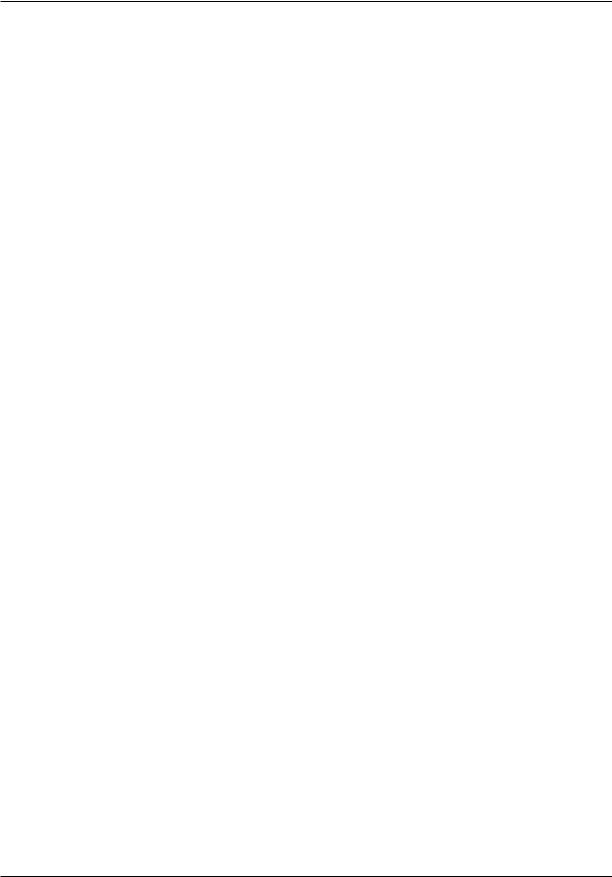
SCIENTIFIC EXPLANATION
know whether the experimental apparatus, the theory, and the competence of the researcher have combined to produce a successful experiment, it is necessary to know beforehand what the correct outcome is. However, the definition of a competently performed experiment is having a successful outcome, leading to the ‘‘experimenter’s regress’’ (Collins 1985).
The replication of results is an essential criterion for the stability of scientific knowledge, but scientific inquiry requires a high degree of tacit or personal knowledge (Polanyi 1958). This knowledge is by nature invisible, but its importance is strongly denied by a scientific community that bases its claims to validity on the potential for replication. Scientific developments often cannot be replicated unless there is direct, personal contact between the original researcher and the people attempting to do the replication. Few replications are possible using published results and procedures, and successful replication often rests on the original researcher’s tacit knowledge, which is not easily transferable (Collins 1985). To complicate matters, science reserves its highest rewards for original research rather than replication. As a consequence, there is little glory and less funding for replication, and the ‘‘replicability’’ requirement is reduced to demonstrating the possibility of replication.
Feminists have added their voice to critiques of science and the scientific method. The most successful feminist critiques of science are those identified as ‘‘feminist empiricist,’’ which attempt to restructure ‘‘bad science’’ and provide a more objective, gender-free knowledge (Harding 1986). Feminists have pointed out some androcentric (male-centered) categories in science and have identified the patriarchal social organization of ‘‘science as an institution.’’ Haraway has argued that there is no purely ‘‘objective’’ stance that can be taken; knowledge is always a ‘‘view’’ from somewhere (Haraway 1988). The concept of power based on gender has become a permanent category of analysis in feminist approaches (Smith 1987; Connel 1983).
By differentiating between ‘‘good science’’ and ‘‘bad science,’’ feminist empiricists strive to separate the wheat from the chaff by eradicating gender biases in the scientific process. The ultimate goal is to provide more objective, value-free knowl-
edge (Harding 1987). At the very least, feminist approaches often attempt to show the hidden biases in many scientific theories. The argument is that some types of knowledge are true only for certain social groups and do not reflect the experience of women, homosexuals, and many ethnic and racial groups, or other groups on the margins of society (Haraway 1988).
This perspective has had some success in the social sciences, perhaps because its revisions provide results that are intuitively appealing. By including categories that often are ignored, oppressed, and invisible to traditional sociology, feminist research gives a voice to what were previously ‘‘non-questions’’ under the mainstream, or as feminists call it, the male-stream model of science (Vickers 1982). For example, feminist research suggests that many women do not make a yes-or-no decision about having children but instead leave it to luck or time to decide. This type of decision-making behavior has implications for fertility and deserves the same theoretical status as the yes and no categories. However, a male-stream model of science that assumed that fertility decisions were the outcome of a series of rational costbenefit analyses was blind to this conceptualization (Currie 1988).
It is ironic that while feminist empiricist criticisms of ‘‘bad’’ science aspire to strengthen science, they ultimately subvert the understandings of science they attempt to reinforce: ‘‘If the concepts of nature, of dispassionate, value free, objective inquiry, and of transcendental knowledge are androcentric, white, bourgeois, and Western, then no amount of more rigorous adherence to scientific method will eliminate such bias, for the methods themselves reproduce the perspectives generated by these hierarchies and thus distort our understandings’’ (Harding 1987, p. 291).
Another critique of science comes from the hermeneutic, or interpretive, perspective, which takes issue with the positivist assumption that the concepts, categories, and methods used to describe the physical world are applicable to human behavior. Human studies proponents insist that the universal categories and objective arguments required for prediction and explanation in the natural sciences cannot be achieved in the social sciences. The proper subject matter of the social sciences is the internal, or subjective, meanings of
2471
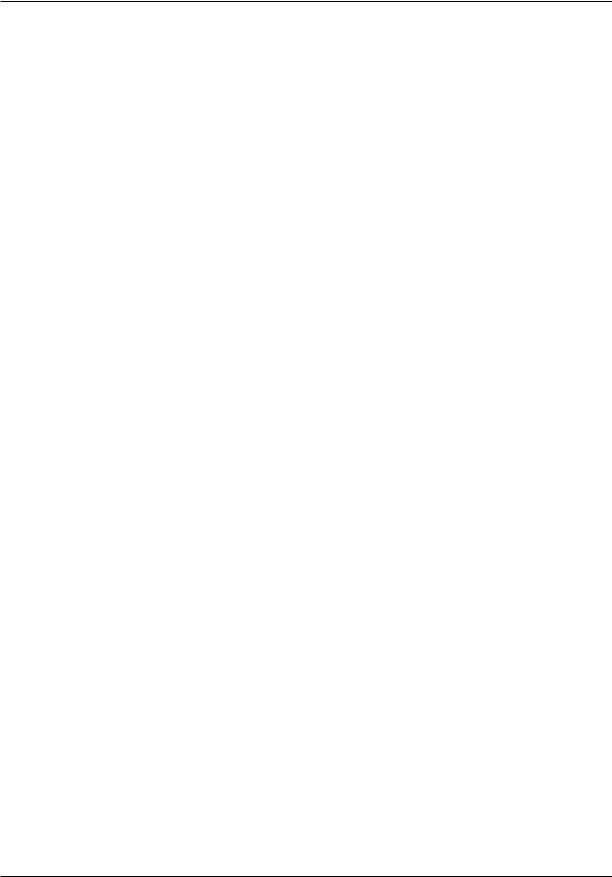
SCIENTIFIC EXPLANATION
human behavior that guide human action. Because these meanings are nonempirical and subjective rather than objective, they cannot meet the requirements for scientific explanation. Therefore, the goal of the social sciences is to understand rather than predict and explain human behavior (Hughes 1961; Habermas 1971; Gadamer 1976). Validation of interpretations is one of the biggest problems with the hermeneutic position because no firm ground exists from which to judge the validity of different interpretations of meaning and behavior. Hermeneutic explanations are ultimately subjective and in their extreme form focus solely on the explanation of individual, unique events (Alexander 1982).
The value-free nature of scientific knowledge also has been challenged by critical theory, which suggests that scientific knowledge is knowledge that is one-sided and specifically oriented to the domination and control of nature. This ‘‘interest’’ in domination and control does not lie in the application of scientific knowledge but is intrinsic to the knowledge itself. In contrast, communicative knowledge is knowledge that is oriented to reaching understanding and achieving human emancipation (Habermas 1984).
CONCLUSION
Although scientific explanation has been the subject of many critiques, it is still the most methodical, reliable form of knowledge. It is ironic that while the natural sciences are becoming less positivistic and are beginning to recognize nonempirical, subjective, and cultural influences on scientific knowledge, the social sciences continue to emphasize the refinement of methodology and measurement in an attempt to become more positivistic (Alexander 1982). The result is that in sociology, theoretical inquiry is increasingly divorced from empirical research. Paradoxically, this schism may be a source of strength if the two sides can learn to communicate. Sociology may be in a unique position to integrate critiques of the scientific model with ongoing empirical research, perhaps producing a hybrid that is neither relativistic nor positivistic.
REFERENCES
Alexander, Jeffrey C. 1982 Positivism, Presuppositions and Current Controversies. Berkeley: University of California Press.
Babbie, Earl 1989 The Practice of Social Research, 5th ed. Belmont, Calif: Wadsworth.
Bernstein, Richard J. 1976 The Restructuring of Political and Social Theory. Philadelphia: University of Pennsylvania Press.
Blalock, Hubert M. 1964 Causal Inferences in Non-Experi- mental Research. Chapel Hill: University of North Carolina Press.
———1979 Social Statistics, rev. 2nd ed. New York: McGraw-Hill.
Braithwaite, Richard Bevan 1968 Scientific Explanation.
London: Cambridge University Press.
Chalmers, A. F. 1982 What Is This Thing Called Science?
Milton Keynes, UK: Open University Press.
Cohen, M., and E. Nagel 1934 An Introduction to Logic and Scientific Method. New York: Harcourt.
Collins, H. M. 1985 Changing Order. Replication and Induction in Scientific Practice. Beverly Hills, Calif: Sage.
*Collins, H. M., and Pinch, T. 1998 The Golem: What You Should Know about Science. New York: Cambridge University Press.
Connell, R. W. 1983 Which Way Is Up? Boston: Allen and Unwin.
Cook, Thomas D., and Donald T. Campbell 1979 QuasiExperimentation: Design and Analysis Issues for Field Settings. Chicago: Rand McNally.
Currie, Dawn 1988 ‘‘Re-Thinking What We Do and How We Do It: A Study of Reproductive Decisions.’’ Canadian Review of Sociology and Anthropology 25:231–253.
Fleck, Ludwik 1979 Genesis and Development of a Scientific Fact. Chicago: University of Chicago Press (originally published in German in 1935).
Gadamer, Hans-Georg 1976 Philosophical Hermeneutics.
Berkeley: University of California Press.
Gartrell, John W., and C. David Gartrell 1979 ‘‘Status, Knowledge, and Innovation: Risk and Uncertainty in Agrarian India.’’ Rural Sociology 44:73–94.
Giere, Ronald N. 1984 Understanding Scientific Reasoning, 2nd ed. New York: Holt, Rinehart and Winston.
Glaser, Barney G., and Anselm L. Strauss 1967 The Discovery of Grounded Theory. Chicago: Aldine.
(SEE ALSO: Causal Inference Models; Epistemology; Experiments; Measurement; Metatheory; Positivism; Quasi-Experi- mental Research Design; Reliability; Validity; Statistical Inference)
Habermas, Jurgen 1971 Knowledge and Human Interests.
Boston: Beacon Press.
——— 1984 The Theory of Communicative Action. Boston: Beacon Press.
2472
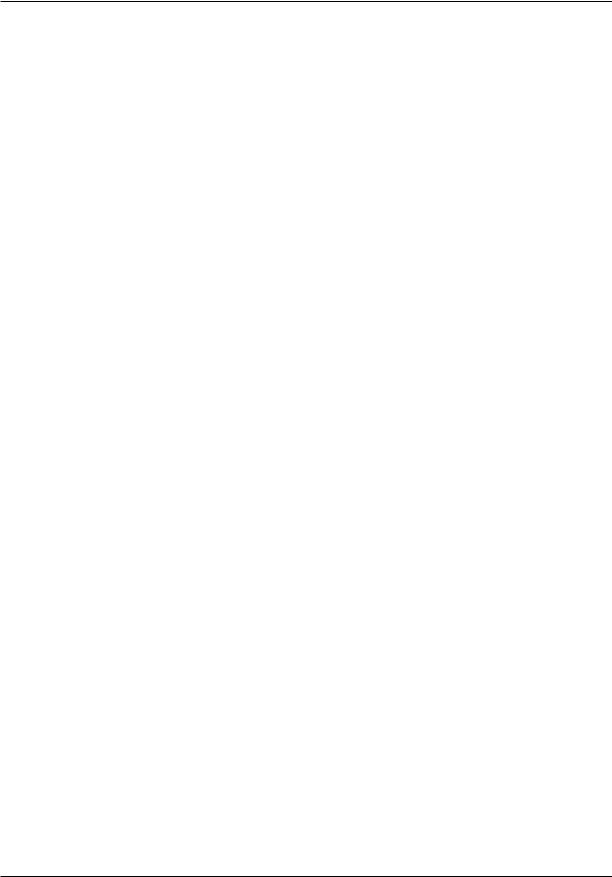
SECONDARY DATA ANALYSIS AND DATA ARCHIVES
Hannan, Michael T. 1970 Problems of Aggregation and Disaggregation in Sociological Research. Chapel Hill: University of North Carolina Press.
Hanson, Norbert R. 1972 Patterns of Discovery. Cambridge, UK: Cambridge University Press.
Haraway, Donna 1988 ‘‘Situated Knowledges: The Science Question in Feminism and the Privilege of Partial Perspective.’’ Feminist Studies 14:575–609.
Harding, Sandra 1986 The Science Question in Feminism. Ithaca, N.Y.: Cornell University Press.
——— 1987 ‘‘The Instability of the Analytical Categories of Feminist Theory.’’ In Sandra Harding and Jean F. O’Barr, eds., Sex and Scientific Inquiry. Chicago: University of Chicago Press.
Hempel, Carl 1966 Philosophy of Natural Science. Englewood
Cliffs, N.J.: Prentice-Hall.
Hughes, Stuart 1961 Consciousness and Society. New York:
Vintage.
Kaplan, Abraham 1964 The Conduct of Inquiry: Methodology for Behavioral Science. San Francisco: Chandler.
Keat, R., and J. Urry 1982 Social Theory as Science. London: Routledge and Kegan Paul.
Kerlinger, Fred N. 1973 Foundations of Behavioral Research, 2nd ed. New York: Holt, Rinehart and Winston.
Knorr Cetina, Karin 1981 The Manufacture of Knowledge. An Essay on the Constructivist and Contextual Nature of Science. Oxford, UK: Pergamon Press.
———1991 ‘‘Epistemic Cultures: Forms of Reason in Science’’ History of Political Economy, 23(1):105–122.
———1992 ‘‘The Couch, the Cathedral, and the Laboratory: On the Relationship between Experiment and Laboratory in Science.’’ In Andrew Pickering, ed., Science as Practice and Culture. Chicago: University of Chicago Press.
———1995 ‘‘How Superorganisms Change: Consensus Formation and the Social Ontology of High-Energy Physics Experiments.’’ Social Studies of Science 25:119–47.
———1999 Epistemic Cultures. How the Sciences Make Knowledge. Cambridge and London: Harvard University Press.
Pickering, Andrew 1982 ‘‘Interests and Analogies.’’ In B. Barnes and D. Edge, eds., Science in Context. Milton Keynes, UK: Open University Press.
Polanyi, Michael 1958 Personal Knowledge. Chicago: Uni-
versity of Chicago Press.
Popper, Karl R. 1959 The Logic of Scientific Discovery. London: Hutchinson.
Shapin, Steven 1979 ‘‘The Politics of Observation: Cerebral Anatomy and Social Interests in the Edinburgh Phrenology Disputes.’’ In Roy Wallis, ed., On the Margins of Science: The Social Construction of Rejected Knowledge. Monograph 27, 1979 Sociological Review.
Smith, Dorothy 1987 The Everyday World as Problematic. Boston: Northeastern University Press.
Stevens, S. S. 1951 ‘‘Mathematics, Measurement, and Psychophysics.’’ In S. S. Stevens, ed., Handbook of Experimental Psychology. New York: Wiley.
Stinchcombe, Arthur L. 1987 Constructing Social Theories. Chicago: University of Chicago Press.
Swinburne, R. G. 1964 ‘‘Falsifiability of Scientific Theories.’’ Mind, 73:434–436.
Vickers, Jill 1982 ‘‘Memoirs of an Ontological Exile: The Methodological Rebellions of Feminist Research.’’ In Angela Miles and Geraldine Finn, eds., Feminism in Canada: From Pressure to Politics. Montreal: Black Rose.
Wallace, Walter L. 1971 The Logic of Science in Sociology. Chicago: Aldine.
Whyte, William Foote 1961 Street Comer Society: The Social Structure of an Italian Slum, 2nd ed. Chicago: University of Chicago Press.
*Woolgar, Steve 1988 Science, the Very Idea. London, New York: Tavistock.
Woolgar, Steve 1981 ‘‘Interests and Explanation in the Social Study of Science.’’ Social Studies of Science 11:365–394.
LINDA DERKSEN
JOHN GARTRELL
Kuhn, Thomas 1970 The Structure of Scientific Revolutions, 2nd ed. Chicago: University of Chicago Press.
*Latour, Bruno 1987 Science in Action: How to Follow Scientists and Engineers through Society. Cambridge, Mass.: Harvard University Press.
———, and Steve Woolgar 1986 Laboratory Life. Princeton, N.J.: Princeton University Press.
Nagel, Ernest 1961 The Structure of Science: Problems in the Logic of Scientific Explanation. New York: Harcourt, Brace and World.
SCIENTIFIC METHOD
See Epistemology; Positivism; Scientific
Explanation.
SECONDARY DATA ANALYSIS AND DATA ARCHIVES
The creation and growth of publicly accessible data archives (or data banks) have revolutionized
2473
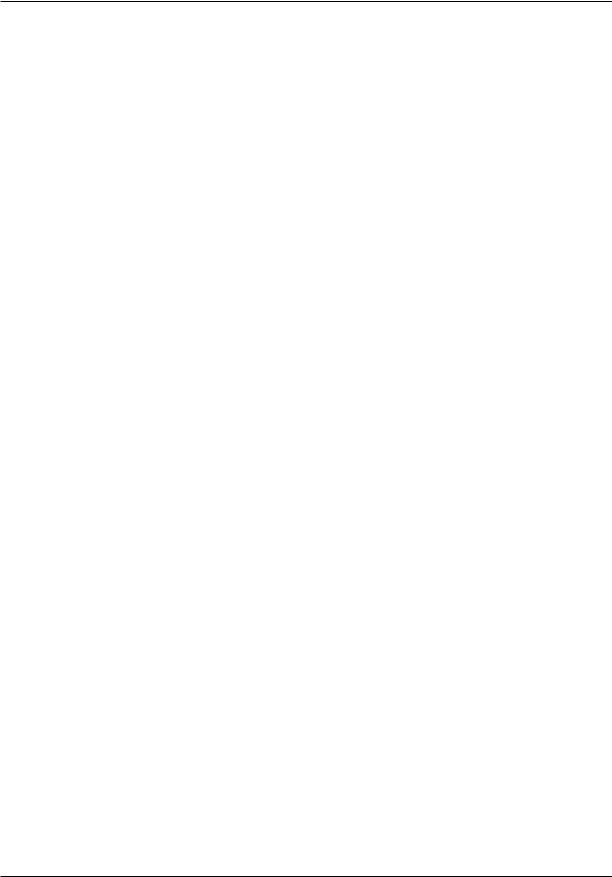
SECONDARY DATA ANALYSIS AND DATA ARCHIVES
the way sociologists conduct research. These resources have made possible a variety of secondary analyses, often utilizing the data in ways never anticipated by their creators. Traditionally, secondary data analysis involves the use of an available data resource by researchers to study a problem different from the one treated in the original analysis. For example, a researcher might have conducted a survey of workers’ reactions to technological change and analyzed those data to evaluate whether the workers welcomed or resisted such change in the workplace. As a matter of secondary interest, the researcher collects data on workers’ perceptions of the internal labor-market structures of their firms. She then lends those data to a colleague who studies the determinants of (workers’ perceptions of) job-ladder length and complexity in order to understand workers’ views on prospects for upward mobility in their places of employment. The latter investigation is a secondary analysis.
More recently, however, the definition of a secondary analysis has expanded as more data sets have been explicitly constructed with multiple purposes and multiple users in mind. The creators, or principal investigators, exercise control over the content of a data set but are responsive to a variety of constituencies that are likely to use that resource. The creators may undertake analyses of the data, addressing questions of intellectual interest to themselves while simultaneously releasing the data to the public or depositing the data resource in an archive. Data archives are depositories where data produced by a number of investigators are available for secondary analyses. The data bank generally takes responsibility for providing documentation on the data sets and other information needed for their use. The term also refers more generally to any source of data already produced that an investigator may uncover in the course of an investigation, such as government or business records housed in libraries. For example, the U.S. government archives thousands of government documents yearly in libraries around the world. The data in those documents cover a wide variety of topics and are often useful in sociological investigations. It remains the responsibility of the analyst to configure the data in a way that is useful to his or her investigation. This entry illustrates these expanded opportunities by describing one key data archive and indicating the extent and
breadth of data resources that this and other archives include. It then describes the process of conducting secondary analyses from resources such as these.
DATA ARCHIVES AND DATA SOURCES
One of the most important data archives for social scientists is the Interuniversity Consortium for Political and Social Research (ICPSR) at the University of Michigan, Ann Arbor. The ICPSR publishes an annual Guide to Resources and Services
(much of this description was taken from the 1996–1997 volume). Additional information is available at the ICPSR Web site (www.icpsr.umich.edu). The consortium was founded in 1962 as a partnership between the Survey Research Center at the University of Michigan and twenty-one U.S. universities. In 1997 the holdings included over 3,500 titles, some of them capturing several panels of data on the same respondents or several waves of data involving comparable information. These titles are available to researchers at member institutions. The consortium charges fees on a sliding scale to academic institutions for membership privileges; researchers whose institutions are not members can obtain data for a fee. In 1997, over four hundred institutions in the United States, Canada, and countries throughout the world were members. While ICPSR originated as a service to political analysts, it currently serves a broad spectrum of the social sciences, including economics, sociology, geography, psychology, and history as well, and its data resources have been used by researchers in education, social work, foreign policy, criminal justice, and urban affairs.
Although ICPSR provides training in research and statistical methods and helps members in the effective use of computing resources, its central function is the archiving, processing, and distribution of machine-readable data of interest to social scientists. Although data capturing elements of the U.S. political process are well represented in its holdings, data are available on consumer attitudes, educational processes and attainment, health care utilization, social indicators of the quality of American life, employment conditions, workers’ views on technology, and criminal behavior. The data come from over 130 countries, include both contemporary and historical censuses, and are not confined to the individual level but also provide
2474