
Введення в спеціальність / Додаткова література / data mining with ontologies
.pdfOntology-Based Construction of Grid Data Mining Workflows
Bernstein, A., Hill, S., & Provost, F. (2001). An intelligent assistant for the knowledge discovery process.InProceedingsoftheIJCAI-01Workshop on Wrappers for Performance Enhancement in KDD. Seattle, WA: Morgan Kaufmann.
Brezany,P., Tjoa,A.M.,Rusnak,M.,&Janciak,
I. (2003). Knowledge Grid support for treatment of traumatic brain injury victims. International Conference on Computational Science and its Applications. Montreal, Canada.
Brezany,P.,Janciak,I.,Woehrer,A.,&Tjoa,A.M.
(2004). GridMiner:AFrameworkforknowledge discovery on the Grid - from a vision to design and implementation. Cracow Grid Workshop, Cracow, Poland: Springer.
Brezany, P., Janciak, I., Kloner, C., & Petz, G.
(2006). Auriga—workflowengineforWS-I/WS- RFservices. Retrieved September 15, 2006, from http://www.Gridminer.org/auriga/
Bussler ,C., Davies, J., Dieter, F., & Studer , R.
(2004). The Semantic Web: Research and applications. In Proceedings of the 1st European SemanticWebSymposium,ESWS.LectureNotes in Computer Science, 3053. Springer.
Chapman, P., Clinton, J., Khabaza, T., Reinartz, T., & Wirth. R. (1999). The CRISP-DM process model.Technicalreport,CRISM-DMconsortium. Retrieved May 15, 2006, from http://www.crisp- dm.org/CRISPWP-0800.pdf
Christensen, E., Curbera, F., Meredith, G., &
Weerawarana, S. (2001). Web Services Description Language (WSDL) 1.1. Retrieved May 10, 2006, from http://www.w3.org/TR/wsdl
Data Mining Group. (2004). Predictive model markuplanguage. Retrieved May 10, 2006, from http://www.dmg.org/
Deelman,E.,Blythe,J.,Gil,Y.,&Kesselman,C. (2003). Workflow management in GriPhyN. The Grid Resource Management. The Netherlands: Kluwer.
Globus Alliance (2005). Globus Toolkit 4. http:// www.globus.org
Globus Alliance, IBM, & HP (2004). The WSResource framework. Retrieved May 10, 2006, from http://www.globus.org/wsrf/
Hornick, F. M., et al. (2005). Java data mining 2.0. Retrieved June 20, 2006, from http://jcp.org/ aboutJava/communityprocess/edr/jsr247/
Horrocks, I., Patel-Schneider, P. F., Boley, H., Tabet, S., Grosof, B., & Dean, M. (2004). SWRL: A SemanticWebrulelanguagecombiningOWLand RuleML. W3C Member Submission. Retrieved May 10, 2006, from http://www.w3.org/Submis- sion/2004/SUBM-SWRL-20040521
Kickinger, G., Hofer, J., Tjoa, A.M., & Brezany, P.(2003).WorkflowmManagementinGridMiner.
The3rdCracowGridWorkshop. Cracow, Poland: Springer.
Leymann, F. (2001). Web services flow language (WSFL1.0). Retrieved September 23, 2002, from www4.ibm.com/software/solutions/webservices/ pdf/WSFL.pdf
Majithia,S.,Walker,D.W.,&Gray,W.A.(2004).
Aframeworkforautomatedservicecomposition in service-oriented architectures (pp. 269-283). ESWS.
Martin, D., Paolucci, M., McIlraith, S., Burstein, M., McDermott, D., McGuinness, D., et al.(2004).
BringingsemanticstoWebservices:TheOWL-S approach. In Proceedings of the 1st International Workshop on Semantic Web Services and Web Process Composition. San Diego, California.
McBride, B. (2002). Jena: A Semantic Web toolkit. IEEE Internet Computing, November /December, 55-59.
Oinn, T. M., Addis, M., Ferris, J., Marvin, D.,
Senger, M., Greenwood, R. M., et al. (2004). Taverna: A tool for the composition and enactment of bioinformatics workflows. Bioinformatics, 20(17), 3045-3054.
0
Ontology-Based Construction of Grid Data Mining Workflows
Noy, N. F. , Sintek, M., Decker, S., Crubezy, M., Fergerson, R. W., & Musen, M.A. (2001). Creating Semantic Web contents with Protege-2000.
IEEE Intelligent Systems, 16(2), 60-71.
Quinlan, R. (1993). C4.5: Programs for machine learning. San Mateo, CA: Morgan Kaufmann Publishers.
Sairafi, S., A., Emmanouil, F. S., Ghanem, M., Giannadakis, N., Guo, Y., Kalaitzopolous, D., et al. (2003). The design of discovery net: Towards open Grid services for knowledge discovery.
International Journal of High Performance Computing Applications, 17(3).
Seaborne,A.(2004).RDQL: Aquerylanguagefor RDF. Retrieved May 10, 2006, from http://www. w3.org/Submission/RDQL/
Sirin, E., & Parsia, B. (2004). Pellet: An OWL DL Reasoner, 3rd International Semantic Web Conference, Hiroshima, Japan. Springer.
Sirin, E.B. Parsia, B., & Hendler, J. (2004).
Filtering and selecting Semantic Web services with interactive composition techniques. IEEE Intelligent Systems, 19(4), 42-49.
SPARQL. Query Language for RDF, W3C Working Draft 4 October 2006. Retrieved October 8,
2006, from http://128.30.52.31/TR/rdf-sparql- query/
Thatte, S. (2001). XLANG: Web services for businessprocessdesign. Microsoft Corporation, Initial Public Draft.
Tsalgatidou, A., & Pilioura, T. (2002). An overview of standards and related technology in web services. Distributed and Parallel Databases. 12(3).
Witten, I.H., & Eibe, F. (2005). Data mining: Practicalmachinelearningtoolsandtechniques. (2nd ed.). San Francisco: Morgan Kaufmann.
World Wide Web Consortium. (2004). OWLWeb ontologylanguagesemanticsandabstractsyntax. W3C Recommendation 10 Feb, 2004.
additiOnal reading
For more information on the topics covered in this chapter, see http://www.Gridminer.org and also the following references:
Alesso, P. H., & Smith, F. C. (2005). Developing Semantic Web services. A.K. Peterson Ltd.
Antoniou,G.,&Harmelen,F.(2004).ASemantic Web primer. MIT Press.
Davies, J., Studer, R., & Warren P. (2006). SemanticWebtechnologies:Trendsandresearchin ontology-based systems. John Wiley & Sons.
Davies, N. J., Fensel, D., & Harmelen, F. (2003).
Towards the Semantic Web: Ontology-driven knowledge management. John Wiley & Sons.
Foster, I., & Kesselman, C. (1999). The Grid: Blueprint for a new computing infrastructure.
Morgan Kaufmann.
Fox,G.C.,Berman,F.,&Hey,A.J.G.(2003).Grid computing: Making the global infrastructure a reality. John Wiley & Sons.
Han,J.,&Kamber,M.(2000)Datamining:Concepts and techniques. Morgan Kaufmann.
Lacy, L.W. (2005). Owl: Representing information using the Web ontology language. Trafford Publishing.
Li, M., & Baker, M. (2005). The Grid: Core technologies. John Wiley & Sons.
Marinescu, D.C. (2002) Internet-basedworkflow management: Toward a Semantic Web. John
Wiley & Sons.
0
Ontology-Based Construction of Grid Data Mining Workflows
Matjaz,B.J.,Sarang,P.G.,& Mathew,B.(2006).
Business process execution language for Web services (2nd ed.). Packt Publishing.
Murch, R. (2004). Autonomic computing. Published by IBM Press.
Oberle, D. (2005). The semantic management of middleware. Springer.
Singh,M.P.,&Huhns,M.N.(2006).Service-ori- ented computing: Semantics, processes, agents.
John Wiley & Sons.
Sotomayor, B., & Childers, L. (2006). Globus Toolkit 4: Programming Java services. Morgan Kaufmann.
Stojanovic,Z.,&Dahanayake.A.(2005).Service orientedsoftwaresystemengineering:Challenges and practices. Idea Group Inc.
Taylor,I.J.,DeelmanE.,Gannon,D.B.,&Shields,
M. (2007). Workflows for e-science. Springer.
Zhong, N., Liu, J., & Yao, Y.(2003). Web intelligence. Springer.
Zhu, X., & Davidson, I. (2007). Knowledge discoveryanddatamining:Challengesandrealities.
Idea Group Inc.
Zhuge, H. (2004). The knowledge Grid. World
Scientific.
0
Chapter XI
Ontology-Based Data
Warehousing and
Mining Approaches in
Petroleum Industries
Shastri L. Nimmagadda
Kuwait Gulf Oil Company, Kuwait
Heinz Dreher
Curtin University of Technology, Australia
aBstraCt
Several issues of database organization of petroleum industries have been highlighted. Complex geospatialheterogeneousdatastructurescomplicatetheaccessibilityandpresentationofdatainpetroleum industries.Objectivesofthecurrentresearcharetointegratethedatafromdifferentsourcesandconnect them intelligently. Data warehousing approach supported by ontology, has been described for effective dataminingofpetroleumdatasources.Petroleumontologyframework,narratingtheconceptualization ofpetroleumontologyandmethodologicalarchitecturalviews,hasbeendescribed.Ontology-baseddata warehousingwithfine-grainedmultidimensionaldatastructures,facilitatetominingandvisualizationof data patterns, trends, and correlations, hidden under massive volumes of data. Data structural designs and implementations deduced, through ontology supportive data warehousing approaches, will enable the researchers in commercial organizations, such as, the one of Western Australian petroleum industries, for knowledge mapping and thus interpret knowledge models for making million dollar financial decisions.
Overview
Data in major commercial petroleum industries arecomplexinnatureandoftenpoorlyorganized
and duplicated, and exist in different formats. Business, in these companies, is operated both in space and time. Due to the diverse nature of business products and operations in different
Copyright © 2008, IGI Global, distributing in print or electronic forms without written permission of IGI Global is prohibited.
Ontology-Based Data Warehousing and Mining Approaches in Petroleum Industries
geographic locations, these industries demand more accurate and precise information and data. Businesses operating in multiclient or multiuser environments with redundant data are prone to carry information with several ambiguities and anomalies.
With the widespread use of databases and explosive growth in their sizes, petroleum businesses face a problem of information overload.
Effectively utilizing these massive volumes of data is becoming a major challenge for this type of industry. Data searching becomes tedious when specific queries are made, due to the piling up of volumes of data and information accumulated in several places, such as Websites and Web servers.
In order to compete and increase profitability in world markets, it is vital for fast growing businesses to carry out mapping and integration of multioperational data structures. This can deliver accurate and precise information, which is crucial for elegant and economic decision support. Information in the form of knowledge or intelligence extracted from business data always adds value to the quality of decision-making. For the purpose of building knowledge from petroleum business data, an ontology approach that supports data warehousing, combined with data mining and visualization techniques, is a significant breakthrough. This chapter addresses issues of importing/exporting data from Web resources or data from offline sources, their logical and physical storage, and accessing, interpreting, and presenting the explored information.
Sedimentary basins, which are known to bear oil and natural gas deposits, may consist of several petroleum systems. We examine these systems as synonymouswithotherinformationsystems.Ontology is proposed for simplifying the complexity of petroleum exploration and production data of different petroleum systems. This has prompted the development of various conceptual models and translating them into logical data models by a multidimensional data mapping approach.
These logical data models will be converted into implementation models, using a contemporary
DBMS(forexample, Oracle),suchaswarehouse approach, proposed by us. Specific requests will bemadewithqueriesforlocatingaspecificpiece of data or information from these warehouses. Simple mining algorithms will be developed and used for extracting patterns, correlations and trends from petroleum data. These patterns and trends are interpreted for a meaningful geological knowledge.
intrOdUCtiOn
Large amounts of petroleum operational data are routinely collected and stored in the archives of many organizations. Much of the data archived for informational, as well as audit purposes, are still under-utilized or many personnel do not know what to do with them. However, by analyzing the petroleum data of one basin, it would be possible to discover exploration, drilling, and production patterns of other basins and use these patterns for future planning of various classes of drillable exploratory or development wells. Such an approach was not feasible until recently due to limitations in both hardware and software. In the recent past (Pujari, 2002) there has been a tremendous improvement in hardware—several
(gigabytes) GB of main memory, multi terabytes ofdiskspacewithmultiGHzofprocessingspeed on a PC. Thus, computer programs, which sift massive amounts of operational data recognize data patterns and provide hints to formulate hypotheses for tactical and strategic decision-mak- ing, can now be executed in a reasonable time. This has opened up a productive area of research to formulate appropriate algorithms for mining archival data to devise and test hypotheses. In this project, an array of ideas from computer science, information technology, statistics and management science are being applied for organizing
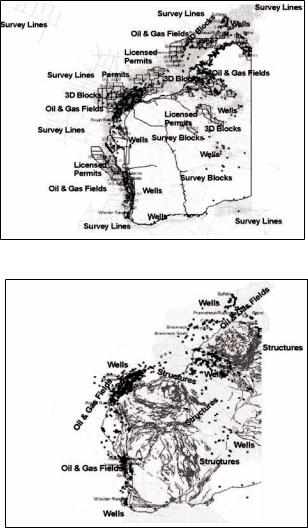
Ontology-Based Data Warehousing and Mining Approaches in Petroleum Industries
these heterogeneous petroleum exploration and production data for effective data mining and data visualization.
Several data mining tools are available to analyze, hypothesize, and discover relations among various data items, stored in data warehouses. So far, these tools have been successfully applied in manyfinanceandmarketingcompanies.However, these technologies are still under-utilized in the petroleumindustries;forexample,atypicaluser
forms a hypothesis about a relationship between data entities and verifies it or rejects it with a series of queries against the data. The petroleum businessanalystmighthypothesizeaboutanoilbearingsedimentarybasin:whetherthepossibility ofexploringoilorgascanbeinvestigated;where favorablestructuralsettingsandotheroil-playfac- tors exist. Data mining could be employed to test such hypotheses in different basins of similar oil plays.Similarly,knowingthatmultipayhorizons
Figure 1. Western Australian maps: (a) surveys, wells, permits and oil fields and (b) oil and gas fields associated with structures
(a)
(b)
Ontology-Based Data Warehousing and Mining Approaches in Petroleum Industries
of a particular geological formation belonging to particular geological age are producing oil from a drilled-well of a particular basin, what are the chances of producing oil from similar pay horizons, from the wells of other adjacent basins?
In our proposed case scenarios, for example, several oil, gas, and condensate fields are situated in a number of Western Australian basins. Wells, located on these petroleum prospects, are drilled based on the analysis of variety of surveys (geological, geophysical, and geochemical) and well-log data (petro-physical in nature). Their life span ranges between 5-15 or even more than 20 years in productive basins. Some wells cease to produce petroleum after a few days. Many wells are abandoned and/or suspended, due to the nonexistence of hydrocarbons and the poor commercial viability of some oil fields. Such actions result from poor knowledge and understanding of petroleum systems and interpretation of such ambiguous petroleum-plays. There could be multipay horizons in many wells in different basins. Oil-pay horizons interpreted in certain drilled wells in a basin may eventually be present in other wells of other basins. Petroleum-plays in such situations are complex to narrate. Quality of oil-play factors of a prospect in one basin may be poor, but it may be better in other basins. Analysis of petroleum system and its associated oil-playfactorsiscrucialtorealizationofthefull potential of petroleum prospects.
Problem Definition
In spite of major breakthroughs and advances in resourcestechnologies,identificationandtheprecise description of petroleum systems that narrate oil play factors remain unresolved issues of basins around the globe, including Western Australian basins. Numerous exploration and discovery findings and their associated articles have been published in journals. These published records cover different petroleum systems describing oil-
plays and represent a huge range of exploration and development findings of many basins.
Two major issues are adversely affecting the efficiencyandadvancementofpetroleumbusiness data research and petroleum exploration. These issues are (1) effective access to distributed unstructured information (both Web and off-line sources) and (2) lack of an existing infrastructure to support just-in-time (JIT) precise and accurate information access, and its retrieval. If the operational data are not appropriately integrated, information that is shared by various operational units may lead to failure of crucial operational activities. We attempt to use a systematic shared ontology, supporting data warehouse modeling, and data mining research to organize and store valuable petroleum industries data. Most of the published results are available on the Web through journal databases and resources databases in several formats and on software platforms, and thus amenable to this approach. Historical data are also available in hard copies. For example, historical exploration and production data (see Figure 1) in
Canning,Carnarvon,Bonaparte,Browse,Officer,
Eucla and Perth basins are available in different media and at times, these valuable data are not simply retrievable for computer analysis.
Understanding the prospect of a basin is a significantproblem.Dataintegrationandinformation sharing among different fields or prospects of different basins are key issues of the present problemdefinition.Littleattentionhasbeengiven tointegratingandorganizingthesehistoricaldata. Ontologies for structuring data warehouses and techniques for mining the warehoused data are needed for solving problems associated with data integrationandinformationsharing.Todate,there has been no systematic investigation of these data volumes using the proposed, focused technologies. Meticulous analysis of oil-plays of different petroleum systems of different fields associated with different basins is a much-needed research. Even without additional surveys or exploratory drilling, many more prospects can be explored or
Ontology-Based Data Warehousing and Mining Approaches in Petroleum Industries
discovered by data mining of warehoused existing exploration and production data. Unorganized volumes of massive stores of oil and gas business data hide undiscovered (unknown knowledge or intelligence) data patterns. Interpreting the patterns, correlations and trends among exploration, drilling and production data as well as their oilplayfactorsintomeaningfulscientificgeological and petroleum business information, is the goal of our current research.
The cost of drilling a well varies from five to ten million dollars. Two key data structural scenarios are considered to improve the economics of petroleum reservoirs both at exploration and development stages involving (1) oil-play factors (reservoir, structure, source-maturity, seal, migration, timing of petroleum generation) have amajorroleinascertainingefficiencyofdifferent petroleumsystemsofWesternAustralianoil-bear- ingsedimentarybasinsnarratingtheirpotentiality (Figure 2a). (2) Exploration, drilling, production, and marketing data entities of Western Australian petroleum industry situation are considered for understanding the exploration and production system (which is again an information system) of each basin (Figure 2b).
related work
Research articles on ontology, data warehousing, and data mining have been examined for their feasibilityandapplicabilitytooilandgascompany operations. (Guan & Zhu, 2004; O’Leary, 2000;
Uschold, 1998) discuss concepts of ontology, and principles of conceptual modeling and ontology acquisition. Hadzic and Chang, (2005) (Jasper & Uschold, 1999; Shanks et al., 2003; Shanks et al., 2004) demonstrate ontology modeling, its validation, semantic conceptualization in various industry applications. Concepts of data warehousing, data modeling procedures for data warehouse design, and their applications in oil and gas industry scenarios are critically examined in
(Gornik,2000;Hofferetal.,2005;Nimmagadda
et al., 2005b; Nimmagadda & Rudra, 2005c; Nimmagadda & Rudra, 2005d; Rudra & Nimmagadda, 2005; Nimmagadda & Dreher, 2006).
They investigate issues of database structuring methodologies and multidimensionality and granularity of data structures in oil and gas exploration business applications. (Nimmagadda et al., 2005a) examine issues of ontology modeling approach in mapping complex oil and gas business data entities, described at various operational unit levels of a petroleum company. Pujari, (2002) and (Dunham, 2003) provide comprehensive insights of data mining technologies with different computing algorithms and applications of various company situations. Biswas et al., (1995), (Cheung et al., 2000; Guha et al., 1999; Huang, 1997; Matsuzawa & Fukuda, 2000; Ng & Han, 1994;Peietal.,2000;Ramkumar&Swami,1998; Yao&Zhong,2000;Yun&Chen,2000;Zhonget al., 1996) describe several data mining techniques such as clustering, associative rule mining and in constructing classifiers using decision tree structures.Oftenpetroleumdataarerepresented in spatio-temporal forms. Miller & Han, (2001), (Ott&Swiaczny,2001;Zhouetal.,1996)illustrate useofspatio-temporaldatasetsandorganizethem in a warehouse environment and explore these data using data mining procedures.
Longleyetal.,(2001)illustratethe21st century’s frontierareasofpetroleumprospectsinAustralian sedimentary basins. Telford et al., (1998) investigate critically the significance of geological, geophysical, and geochemical exploration and prospecting for petroleum deposits in different geologicalenvironments.Theyalsosignificantly investigate types of oil-play and their analysis. Huston et al., (2003) and Gilbert et al., (2004) discuss several burning issues of exploring and exploiting prospective reservoirs using data integration techniques. Several issues on reservoir, structural, and strati-structural plays under different geological settings have been described. DoddsandFletcher,(2004)describeissuesofrisks involved in drilling in explored, under-explored
Ontology-Based Data Warehousing and Mining Approaches in Petroleum Industries
and detailed exploration areas. Hori and Ohashi, |
|
networking) with complex data types, |
|
(2005) and Erdman and Rudi, (2001) demonstrate |
|
such as spatio-temporal forms. |
|
the use of XML technologies for preparing and |
|
° Handling numerous entities (of the or- |
|
delivering the explored data through Internet. |
|
der of 500) and attributes (of the order of |
|
Weimer and Davis, (1995) discuss different petro- |
|
1000),mappingandmodelinghundreds |
|
leum systems of the USA, Middle-East, Russia, |
|
of tables is a tedious process. |
|
and South America narrating stratigraphic and |
|
° Data integration (sometimes among |
|
structural plays. Johnston, (2004) discusses issues |
|
10-15 operational centers) of enormous |
|
oftime-lapse4-Dtechnologythathelpminimize |
|
amount of multidisciplinary data is a |
|
drillingdryholesandreservoirmodeluncertainty. |
|
serious business in large oil and gas |
|
Aside from brief discussions on ontology issues |
|
companies. Sharing of data in a mul- |
|
in oil companies (Meersman, 2004), there is no |
|
ticlient environment must be a prereq- |
|
concrete literature available on ontology applica- |
|
uisite for carrying out the successful |
|
tion in oil and gas industries as on today. |
|
exploration and production business. |
|
major issues of petroleum industries |
|
° Managing large volumes of databases |
|
|
in petroleum industries is one of the |
||
|
|
|
major issues today. As business grows, |
Fast tracking and developing infrastructure for |
|
data volumes associated with petro- |
|
accessing accurate and precise petroleum data |
|
leum industries get accumulated in |
|
from multiple sources, the first author, with his |
|
unmanageable way, especially in the |
|
vast work experience and knowledge of major |
|
knowledge domain. |
|
oil companies and discussions with national and |
• |
Petroleum data mining and knowledge |
|
international operators in Western Australia and |
|
building: |
|
abroad, has identified the following major issues |
|
° Knowledge-building from these mas- |
|
to be resolved: |
|
sive data structures is an intricate issue. |
|
|
|
|
With increasing volumes of periodic |
• |
Petroleum data management: |
|
data, there is difficulty in understand- |
|
° Massive storage devices are needed to |
|
ing or retrieving knowledge. At times, |
|
store volumes of data (of the order of |
|
many operational units are forced to |
|
10 GB for each basin) to describe the |
|
integrateandsharevolumesofmultidis- |
|
numberofpetroleumsystemsinseveral |
|
ciplinary data without prior knowledge |
|
basins. Data pertaining to a range of |
|
of a petroleum system in a basin. In |
|
factors across several basins amount |
|
otherwords,expensivefieldoperations |
|
to several gigabytes in size; these fac- |
|
(e.g., drilling, oil field monitoring, |
|
tors include reservoir, structure, seal, |
|
production casing) are carried out in |
|
source, migration, and deposition |
|
many basins without prior knowledge |
|
timing. The petroleum data include |
|
or outcome of operations. |
|
surveys, wells, permits, and produc- |
|
° More petroleum resources can be ex- |
|
tion data. Large upstream integrated |
|
plored and exploited based on existing |
|
petroleum companies (exploration, |
|
exploration and production data. |
|
drilling, production, and marketing) |
|
° Inconsistency among petroleum meta- |
|
are unable to manage multifaceted |
|
dataandbasincharacteristicsaffectsthe |
|
data comprising of heterogeneous data |
|
economicsinvolvedinplanningandde- |
|
structures (relational, hierarchical, and |
|
veloping drilling programs. Unknown |
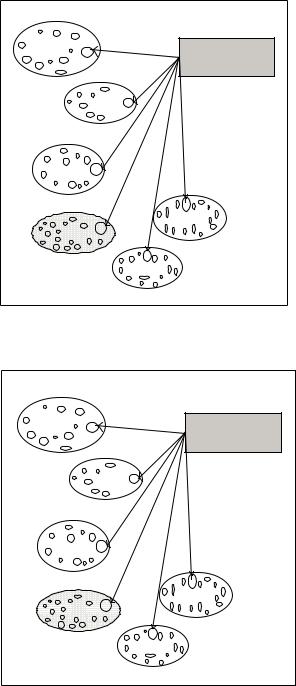
Ontology-Based Data Warehousing and Mining Approaches in Petroleum Industries
Figure 2. (a) Classifying the similarity of oil-play data properties for constructing a petroleum system; Figure 2. (b) classifying petroleum exploration and production attributes for constructing E&P systems
|
entities/Objects |
|
|
reservoir |
petroleum system |
|
|
(r,sm,st,sl,mn,tm) |
|
source |
explored entity/Object |
|
|
|
entities/Objects |
maturity |
|
structure |
|
|
|
|
|
|
seal |
timing |
|
|
|
|
migration |
|
|
entities/Objects |
|
|
(a) |
|
|
entities/Objects |
|
|
reservoir |
petroleum system |
|
|
(r,sm,st,sl,mn,tm) |
|
source |
explored entity/Object |
|
|
|
entities/Objects |
maturity |
|
structure |
|
|
|
|
|
|
|
timing |
seal
migration
entities/Objects
(b)