
Ohrimenko+ / Ross S. A First Course in Probability_ Solutions Manual
.pdf
67.Let Fn denote the fortune after n gambles.
E[Fn] = E[E[Fn Fn−1]] = E[2(2p − 1)Fn−1p + Fn−1 − (2p − 1)Fn−1]
=(1 + (2p − 1)2)E[Fn− 1]
=[1 + (2p − 1)2]2E[Fn−2]
#
= [1 + (2p − 1)2]nE[F0]
68.(a) .6e−2 + .4e−3
(b).6e−2 23 +.4e−3 33 3! 3!
|
|
|
|
|
|
|
|
|
|
|
|
|
|
|
|
|
|
|
|
|
|
|
|
|
|
−2 |
|
|
−2 23 |
|
|
|
−3 −3 33 |
||||||||||||||||
|
|
|
|
|
|
|
P{3,0} |
|
|
.6e |
|
|
|
e |
|
|
|
|
|
+.4e |
|
e |
|
|
|
|
|
||||||||||||||||||||||
|
(c) |
P{3 0} = |
= |
|
|
|
|
|
|
|
3! |
|
|
|
3! |
|
|
||||||||||||||||||||||||||||||||
|
|
P{0} |
|
|
|
|
|
|
|
.6e−2 +.4e−3 |
|
|
|
|
|
|
|
|
|
||||||||||||||||||||||||||||||
|
|
|
|
|
|
|
|
|
|
|
|
|
|
|
|
|
|
|
|
|
|
|
|
|
|
|
|
|
|
|
|
||||||||||||||||||
|
|
∞ |
|
|
|
|
|
|
|
|
|
1 |
|
|
|
|
|
|
|
|
|
|
|
|
|
|
|
|
|
|
|
|
|
|
|
|
|
|
|
|
|
|
|
|
|
|
|
||
69. |
(a) |
|
∫e |
−x |
e |
−x |
dx |
|
|
|
|
|
|
|
|
|
|
|
|
|
|
|
|
|
|
|
|
|
|
|
|
|
|
|
|
|
|
|
|
|
|
|
|
||||||
|
|
|
= |
|
|
|
|
|
|
|
|
|
|
|
|
|
|
|
|
|
|
|
|
|
|
|
|
|
|
|
|
|
|
|
|
|
|
|
|
||||||||||
|
|
2 |
|
|
|
|
|
|
|
|
|
|
|
|
|
|
|
|
|
|
|
|
|
|
|
|
|
|
|
|
|
|
|
|
|
|
|
||||||||||||
|
|
0 |
|
|
|
|
|
|
|
|
|
|
|
|
|
|
|
|
|
|
|
|
|
|
|
|
|
|
|
|
|
|
|
|
|
|
|
|
|
|
|
|
|
|
|
|
|
|
|
|
|
∞ |
|
−x x3 |
|
|
−x |
|
1 |
∞ |
|
−y |
|
3 |
|
|
|
|
|
Γ(4) |
|
|
|
|
1 |
|
|
|
|
|
|||||||||||||||||||
|
(b) |
|
∫e |
|
|
|
e |
|
dx = |
|
|
|
|
∫e |
|
|
|
y |
dy = |
96 |
|
= |
|
|
|
|
|
|
|
|
|||||||||||||||||||
|
|
|
3! |
|
96 |
|
|
|
|
|
16 |
|
|
|
|
|
|||||||||||||||||||||||||||||||||
|
|
0 |
|
|
|
|
|
|
|
|
|
|
|
|
|
|
|
|
|
0 |
|
|
|
|
|
|
|
|
|
|
|
|
|
|
|
|
|
|
|
|
|
|
|
|
|
|
|
||
|
|
|
∞∫e−xe−x |
x3 |
|
e−xdx |
|
|
|
|
|
|
|
|
|
|
|
|
|
|
|
|
|
|
|
|
|
|
|
|
|
|
|
|
|
|
|||||||||||||
|
|
|
|
|
|
|
|
|
|
|
|
|
|
|
|
|
|
|
|
|
|
|
|
|
|
|
|
|
|
|
|
|
|
||||||||||||||||
|
(c) |
|
0 |
|
|
|
|
|
3 ! |
|
|
|
|
|
|
|
= |
|
2 |
= |
|
|
2 |
|
|
|
|
|
|
|
|
|
|
|
|
|
|
|
|
|
|
||||||||
|
|
|
|
∞ |
|
|
|
|
|
|
|
|
|
|
|
|
|
4 |
|
81 |
|
|
|
|
|
|
|
|
|
|
|
|
|
|
|
|
|
||||||||||||
|
|
|
|
|
∫e−xe−xdx |
|
3 |
|
|
|
|
|
|
|
|
|
|
|
|
|
|
|
|
|
|
|
|
|
|
||||||||||||||||||||
|
|
|
|
|
|
|
|
|
|
|
|
|
|
|
|
|
|
|
|
|
|
|
|
|
|
|
|
|
|
|
|
|
|
|
|||||||||||||||
|
|
|
|
|
0 |
|
|
|
|
|
|
|
|
|
|
|
|
|
|
|
|
|
|
|
|
|
|
|
|
|
|
|
|
|
|
|
|
|
|
|
|
|
|
|
|
|
|
|
|
70. |
(a) |
|
∫1 |
pdp =1/ 2 |
|
|
|
|
|
|
|
|
|
|
|
|
|
|
|
|
|
|
|
|
|
|
|
|
|
|
|
|
|
|
|||||||||||||||
|
|
0 |
|
|
|
|
|
|
|
|
|
|
|
|
|
|
|
|
|
|
|
|
|
|
|
|
|
|
|
|
|
|
|
|
|
|
|
|
|
|
|
|
|
|
|
|
|
|
|
|
(b) |
|
∫1 |
p2dp =1/ 3 |
|
|
|
|
|
|
|
|
|
|
|
|
|
|
|
|
|
|
|
|
|
|
|
|
|
|
|
|
|
|
|||||||||||||||
|
|
0 |
|
|
|
|
|
|
|
|
|
|
|
|
|
|
|
|
|
|
|
|
|
|
|
|
|
|
|
|
|
|
|
|
|
|
|
|
|
|
|
|
|
|
|
|
|
|
|
|
|
|
|
|
|
|
|
|
1 |
|
|
|
|
|
|
|
|
|
|
|
|
|
|
|
|
|
|
|
|
1 |
|
n |
i |
|
|
|
|
|
|
n−i |
|
|
|
||||||
71. |
P{X = i} = ∫P{X = i |
|
p}dp = ∫ |
(1 |
− p) |
dp |
|||||||||||||||||||||||||||||||||||||||||||
|
i p |
|
|
|
|||||||||||||||||||||||||||||||||||||||||||||
|
|
|
|
|
|
|
|
|
|
|
|
|
|
||||||||||||||||||||||||||||||||||||
|
|
|
|
|
|
|
|
|
0 |
|
|
|
|
|
|
|
|
|
|
|
|
|
|
|
|
|
|
|
|
0 |
|
|
|
|
|
|
|
|
|
|
|
|
|
|
|
|
|
|
|
|
|
|
|
|
|
|
|
|
|
|
|
|
|
|
|
|
|
|
|
|
|
|
|
|
|
|
|
|
|
|
n i!(n −i)! |
=1/(n +1) |
|||||||||||||||||
|
|
|
|
|
|
|
|
|
|
|
|
|
|
|
|
|
|
|
|
|
|
|
|
|
|
|
|
= |
|
|
|
|
|
|
|
|
|
||||||||||||
|
|
|
|
|
|
|
|
|
|
|
|
|
|
|
|
|
|
|
|
|
|
|
|
|
|
|
|
|
|
|
|
1)! |
|
||||||||||||||||
|
|
|
|
|
|
|
|
|
|
|
|
|
|
|
|
|
|
|
|
|
|
|
|
|
|
|
|
|
|
|
i (n + |
|
|
|
|
|
|
|
|
118 |
Chapter 7 |

72. |
(a) |
P{N ≥ i} = ∫1 |
P{N ≥ i |
|
p}dp = ∫1 |
(1− p)i−1dp =1/i |
||
|
||||||||
|
|
|
|
|
|
|||
|
|
0 |
0 |
|
|
|
||
|
(b) P{N = i} = P{N ≥ i} − P{N ≥ i + 1} = |
1 |
|
|||||
|
i(i +1) |
|||||||
|
|
∞ |
|
|
∞ |
|
|
|
|
(c) |
E[N] = ∑P{N ≥ i} = ∑1/ i = ∞ . |
|
|
||||
|
|
i=1 |
|
|
i=1 |
|
|
|
73.(a) E[R] = E[E[R S]] = E[S] = µ
(b)Var(R S) = 1, E[R S] = S Var(R) = 1 + Var(S) = 1 + σ2
(c)fR(r) = ∫ fS (s)FR S (r s)ds
=C∫e−(s−µ)2 / 2σ 2 e−(r −s)2 / 2ds
|
|
|
|
|
µ + rσ |
2 |
|
|
|
σ |
2 |
2 |
|
|
|
∫ |
|
|
|
|
|
|
|
|
|
|
|||
= K |
exp − |
S − |
|
|
|
|
|
ds exp {−(ar |
+ br)} |
|||||
|
|
|
|
1+σ 2 |
|
1 |
+ |
σ2 |
|
|
||||
|
|
|
|
|
|
|
|
|
|
|
|
|
|
|
Hence, R is normal.
(d)E[RS] = E[E[RS S]] = E[SE[R S]] = E[S 2] = µ2 + σ2
Cov (R, S) = µ2 + σ2 − µ2 = σ2
75.X is Poisson with mean λ = 2 and Y is Binomial with parameters 10, 3/4. Hence
(a)P{X + Y = 2} = P{X = 0)P{Y = 2} + P{X = 1}P{Y = 1} + P{X = 2}P{Y = 0}
= e−2 10 |
(3/ 4)2 |
(1/ 4)8 |
+ 2e−2 10 |
(3/ 4)(1/ 4)9 |
+ 2e−2 (1/ 4)10 |
2 |
|
|
1 |
|
|
(b)P{XY = 0} = P{X = 0} + P{Y = 0} − P{X = Y = 0}
=e−2 + (1/4)10 − e−2(1/4)10
(c)E[XY] = E[X]E[Y] = 2 10 34 = 15
Chapter 7 |
119 |

77.The joint moment generating function, E[etX+sY] can be obtained either by using
E[etX+sY] = ∫∫etX +sY f (x, y)dy dx
or by noting that Y is exponential with rate 1 and, given Y, X is normal with mean Y and variance 1. Hence, using this we obtain
E[etX+sY Y] = esYE[EtX Y] = esY eYt+t 2 / 2
and so
E[etX+sY] = et 2 / 2E[e(s+t)Y ]
= et 2 / 2 (1− s −t)−1 , s + t < 1
Setting first s and then t equal to 0 gives
E[etX] = et 2 / 2 (1−t)−1, t < 1
E[esY] = (1 − s)−1, s < 1
78.Conditioning on the amount of the initial check gives
E[Return] = E[Return A]/2 + E[Return B]/2
={AF(A) + B[1 − F(A)]}/2 + {BF(B) + A[1 − F(B)]}/2
={A + B + [B − A][F(B) − F(A)]}/2
> (A + B)/2
where the inequality follows since [B − A] and [F(B) − F(A) both have the same sign.
(b)If x < A then the strategy will accept the first value seen: if x > B then it will reject the first one seen; and if x lies between A and B then it will always yield return B. Hence,
E[Return of x-strategy] = |
B |
if A < x < B |
(A + B) / 2 |
otherwise |
(c)This follows from (b) since there is a positive probability that X will lie between A and B.
79.Let Xi denote sales in week i. Then
E[X1 + X2] = 80
Var(X1 + X2) = Var(X1) + Var(X2) + 2 Cov(X1, X2)
=72 + 2[.6(6)(6)] = 93.6
(a)With Z being a standard normal
|
+ X2 |
|
90 −80 |
|
P(X1 |
> 90) = P Z > |
|
|
|
|
||||
|
|
|
93.6 |
= P(Z > 1.034) ≈ .150
120 |
Chapter 7 |

(b)Because the mean of the normal X1 + X2 is less than 90 the probability that it exceeds 90 is increased as the variance of X1 + X2 increases. Thus, this probability is smaller when the correlation is .2.
(c)In this case,
P(X1 + X2 > 90) = |
|
90 −80 |
|
P Z > |
|
|
|
|
|||
|
|
72 + 2[.2(6)(6)] |
|
|
|
|
= P(Z > 1.076) ≈ .141
Chapter 7 |
121 |

Theoretical Exercises
1.Let µ = E[X]. Then for any a
E[(X − a)2 = E[(X − µ + µ − a)2]
=E[(X − µ)2] + (µ − a)2 + 2E[(x − µ)(µ − a)]
=E[(X − µ)2] + (µ − a)2 + 2(µ − a)E[(X − µ)]
=E[(X − µ)2 + (µ − a)2
2. |
E[ X − a = ∫(a − x) f (x)dx + ∫(x − a) f (x)dx |
||||||
|
|
x<a |
x>a |
|
|
||
|
= aF(a) − ∫xf (x)dx + ∫xf (x)dx − a[1− F(a)] |
||||||
|
|
|
x<a |
x>a |
|
|
|
|
Differentiating the above yields |
|
|
|
|
||
|
derivative = 2af(a) + 2F(a) − af(a) − af(a) − 1 |
||||||
|
Setting equal to 0 yields that 2F(a) = 1 which establishes the result. |
||||||
3. |
E[g(X, Y)] = |
∞∫P{g(X ,Y ) > a}da |
|
|
|
|
|
|
|
0 |
|
|
|
|
|
|
|
∞ |
|
|
|
g (x, y) |
|
|
= |
∫ |
∫∫ f (x, y)dydxda = ∫∫ |
|
∫daf (x, y)dydx |
||
|
|
0 |
x, y: |
|
|
0 |
|
|
|
g (x, y)>a |
|
|
|
|
|
|
|
|
|
|
= ∫∫g(x, y)dydx |
||
4. |
g(X) = g(µ) + g′(µ)(X − µ) + g′′(µ) |
|
(X − µ)2 |
||||
|
|
|
+ … |
||||
2 |
|
||||||
|
|
|
|
|
|
≈ g(µ) + g′(µ)(X − µ) + g′′(µ) (X − µ)2 2
Now take expectations of both sides.
5.If we let Xk equal 1 if Ak occurs and 0 otherwise then
n |
|
X = ∑Xk |
|
k =1 |
|
Hence, |
|
n |
n |
E[X] = ∑E[Xk ] = ∑P(Ak ) |
|
k =1 |
k =1 |
But |
|
n |
n |
E[X] = ∑P{X ≥ k} = ∑P(Ck ) . |
|
k =1 |
k =1 |
122 |
Chapter 7 |
6. X = ∞∫X (t)dt and taking expectations gives
0
E[X] = ∞∫E[X (t)] dt = ∞∫P{X > t}dt
00
7.(a) Use Exercise 6 to obtain that
E[X] = ∞∫P{X > t}dt ≥ ∞∫P{Y > t}dt = E[Y]
00
(b)It is easy to verify that
X+ ≥st Y+ and Y− ≥ st X−
Now use part (a).
8. Suppose X ≥st Y and f is increasing. Then P{f(X) > a} = P{X > f −1(a)}
≥ P{Y > f −1(a)} since x ≥st Y = P{f(Y) > a}
Therefore, f(X) ≥st f(Y) and so, from Exercise 7,
E[f(X)] ≥ E[f(Y)].
On the other hand, if E[f(X)] ≥ E[f(Y)] for all increasing functions f, then by letting f be the increasing function
f(x) = |
1 |
if x > t |
0 |
otherwise |
then
P{X > t} = E[f(X)] ≥ E[f(Y)] = P{Y > t}
and so X >st Y.
9. |
Let |
|
|
|
Ij = |
1 |
if a run of size k begins at the jth flip |
|
|
otherwise |
|
|
|
0 |
Then
n−k +1
Number of runs of size k = ∑I j
j=1
Chapter 7 |
123 |

|
|
|
|
|
|
|
|
n−k +1 |
|
|
|
|
|
|
|
E[Number of runs of size k = E |
∑I j |
|
|
|
|
||||||
|
|
|
|
|
|
|
|
|
j =1 |
|
|
|
|
|
|
|
|
|
|
|
|
|
|
|
|
|
|
|
|
|
|
|
|
|
|
|
|
|
n−k |
|
|
|
|
|
|
|
|
|
|
= P(I1 = 1) + ∑P(I j =1) + P(In−k +1 =1) |
|||||
|
|
|
|
|
|
|
|
|
|
|
j =2 |
|
|
|
|
|
|
|
|
|
|
= pk(1 − p) + (n − k − 1)pk(1 − p)2 + pk(1 − p) |
|||||
10. |
1 = |
n |
|
n |
|
n |
|
n |
|
|
|
n |
|
E ∑Xi |
∑Xi = ∑E Xi |
∑Xi |
= nE |
X1 |
∑Xi |
||||||||
|
|
1 |
|
1 |
|
1 |
|
1 |
|
|
|
1 |
|
|
Hence, |
|
|
|
|
|
|
|
|
|
|
|
|
|
|
|
k |
|
n |
|
= k / n |
|
|
|
|
|
|
|
|
E ∑Xi |
∑Xi |
|
|
|
|
|
|
|
|||
|
|
|
1 |
|
1 |
|
|
|
|
|
|
|
|
11. |
Let |
|
|
|
|
|
j never occurs |
|
|
|
|
|
|
|
|
Ij = 1 |
outcome |
|
|
|
|
|
|
||||
|
|
|
0 |
|
otherwise |
|
|
|
|
|
|
|
|
|
|
r |
|
|
|
|
r |
|
|
|
|
|
|
|
Then X = ∑I j |
and E[X] = ∫(1− p j )n |
|
|
|
|
|
||||||
|
|
1 |
|
|
|
|
j =1 |
|
|
|
|
|
|
12.Let
|
Ij = |
1 |
success on trial j |
||
|
|
otherwise |
|||
|
|
0 |
|||
|
|
n |
|
|
n |
|
E ∑I j = |
∑Pj independence not needed |
|||
|
|
1 |
|
|
1 |
|
|
|
n |
|
n |
|
Var |
∑I j |
= ∑pj (1− pj ) independence needed |
||
|
|
|
1 |
|
1 |
13. |
Let |
|
|
|
|
|
Ij = 1 |
record at j |
|||
|
|
0 |
otherwise |
E ∑n I j = ∑n E[I1 1
Var ∑n I j = ∑n
1 1
n |
|
|
|
|
|
n |
|
j ] = ∑P{X j |
is largest of X1,..., X j } = ∑1/ j |
||||||
1 |
|
|
|
|
|
1 |
|
n |
1 |
|
|
1 |
|
||
Var(I j ) = ∑ |
|
− |
|
||||
|
|
|
|||||
|
j |
1 |
j |
|
|||
1 |
|
|
|
|
124 |
Chapter 7 |

|
n |
n |
|
1 i is success |
|
15. |
µ = ∑pi |
by letting Number = ∑Xi |
where |
||
Xi = |
|||||
|
i=1 |
i=1 |
|
0 - - - |
n
Var(Number) = ∑pi (1− pi )
i=1
maximization of variance occur when pi ≡ µ/n
minimization of variance when pi = 1, i = 1, …, [µ], p[µ]+1 = µ − [µ]
To prove the maximization result, suppose that 2 of the pi are unequal—say pi ≠ pj. Consider a new p-vector with all other pk, k ≠ i, j, as before and with pi = p j = . Then in the variance formula, we must show
|
p |
+ p |
j |
|
|
p |
+ p |
j |
|
|
|
i |
|
− |
i |
|
≥ pi(1 − pi) + pj(1 − pj) |
||||
|
|
|
|
|
|
|||||
2 |
|
2 |
|
1 |
|
2 |
|
|
||
|
|
|
|
|
|
|
|
|
or equivalently,
pi2 + p2j − 2 pi p j = ( pi − p j )2 ≥ 0.
The maximization is similar.
16.Suppose that each element is, independently, equally likely to be colored red or blue. If we let Xi equal 1 if all the elements of Ai are similarly colored, and let it be 0 otherwise, then
∑ir=1 Xi is the number of subsets whose elements all have the same color. Because
r |
|
r |
r |
|
|
|
|
||||
E ∑Xi |
= ∑E[Xi ]= ∑2(1/ 2) |
|
Ai |
||
|
|||||
i=1 |
|
i=1 |
i=1 |
|
|
it follows that for at least one coloring the number of monocolored subsets is less than or equal to ∑ir=1(1/ 2) Ai −1
17.Var(λX1 + (1−λ)X2 )= λ2σ12 + (1−λ)2σ22
d |
( ) = 2λσ12 |
− 2(1−λ)σ22 |
|
σ 2 |
|
|
= 0 λ = |
2 |
|||
dλ |
σ12 +σ22 |
||||
|
|
|
As Var(λX1 + (1 − λ)X2) = E[(λX1 + (1−λ)X2 − µ)2 ]we want this value to be small.
Chapter 7 |
125 |
18.(a. Binomial with parameters m and Pi + Pj.
(b)Using (a) we have that Var(Ni + Nj) = m(Pi + Pj)(1 − Pi − Pj) and thus m(Pi + Pj)(1 − Pi − Pj) = mPi(1 − Pi) + mPj(1 − Pj) + 2 Cov(Ni, Nj) Simplifying the above shows that
Cov(Ni, Nj) = −mPiPj.
19.Cov(X + Y, X − Y) = Cov(X, X) + Cov(X, −Y) + Cov(Y, X) + Cov(Y, −Y)
=Var(X) − Cov(X, Y) + Cov(Y, X) − Var(Y)
=Var(X) − Var(Y) = 0.
20.(a) Cov(X, Y Z)
=E[XY − E[X Z]Y − XE[Y Z] + E[X Z]E[Y Z] [Z]
=E[XY Z] − E[X Z] E[Y Z] − E[X Z]E[Y Z] + E[X Z]E[Y Z]
=E[XY Z] − E[X Z]E[Y Z]
where the next to last equality uses the fact that given Z, E[X Z] and E[Y Z] can be treated as constants.
(b) From (a)
E[Cov(X, Y Z)] = E[XY] − E[E[X Z]E[Y Z]]
On the other hand,
Cov(E[X Z], E[Y Z] = E[E[X Z]E[Y Z]] − E[X]E[Y]
and so |
|
E[Cov(X, Y Z)] + Cov(E[X Z], E[Y Z]) |
= E[XY] − E[X]E[Y] |
|
= Cov(X, Y) |
(c) Noting that Cov(X, X Z) = Var(X Z) we obtain upon setting Y = Z that Var(X) = E[Var(X Z)] + Var(E[X Z])
21.(a) Using the fact that f integrates to 1 we see that
c(n, i) ≡ ∫1 xi−1(1− x)n−i dx = (i − 1)!(n − i)!/n!. From this we see that
0
E[X(i)] = c(n + 1, i + 1)/c(n, i) = i/(n + 1)
E[X(2i) ] = c(n + 2, i + 2)/c(n, i) = |
i(i +1) |
|
(n + 2)(n +1) |
||
|
126 |
Chapter 7 |
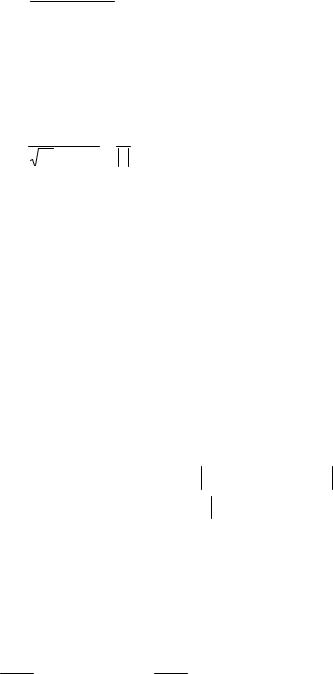
and thus
i(n +1−i) Var(X(i)) = (n +1)2 (n + 2)
(b) The maximum of i(n + 1 − i) is obtained when i = (n + 1)/2 and the minimum when i is either 1 or n.
22.Cov(X, Y) = b Var(X), Var(Y) = b2 Var(X)
ρ(X ,Y ) = b Var(X ) |
= |
b |
b2 Var(X ) |
|
b |
26.Follows since, given X, g(X) is a constant and so
E[g(X)Y X] = g(X)E[Y X]
27.E[XY] = E[E[XY X]]
=E[XE[Y X]]
Hence, if E[Y X] = E[Y], then E[XY] = E[X]E[Y]. The example in Section 3 of random variables uncorrelated but not independent provides a counterexample to the converse.
28.The result follows from the identity
E[XY] = E[E[XY X]] = E[XE[Y X]] which is obtained by noting that, given X, X may be
treated as a constant.
29.x = E[X1 + … + Xn X1 + … + Xn = x] = E[X1 ∑Xi = x]+... + E[Xn ∑Xi = x]
=nE[X1 ∑Xi = x]
Hence, E[X1 X1 + … + Xn = x] = x/n
30. |
E[NiNj Ni] = NiE[Nj Ni] = Ni(n − Ni) |
p j |
since each of the n − Ni trials no resulting in |
1− p |
|||
|
|
i |
|
outcome i will independently result in j with probability pj/(1 − pi). Hence,
E[NiNj] = 1−p jpi (nE[Ni ] − E[Ni2 ])= 1−p jpi [n2 pi − n2 pi2 − npi (1− pi )]
= n(n − 1)pi pj
and
Cov(Ni, Nj) = n(n − 1)pi pj − n2pi pj = −npi pj
Chapter 7 |
127 |