
- •CONTENTS
- •PREFACE
- •LIST OF FIGURES
- •INTRODUCTION
- •1.1 WHAT IS TIME?
- •1.2 SIMULATION
- •1.3 TESTING
- •1.4 VERIFICATION
- •1.6 USEFUL RESOURCES
- •2.1 SYMBOLIC LOGIC
- •2.1.1 Propositional Logic
- •2.1.2 Predicate Logic
- •2.2 AUTOMATA AND LANGUAGES
- •2.2.1 Languages and Their Representations
- •2.2.2 Finite Automata
- •2.3 HISTORICAL PERSPECTIVE AND RELATED WORK
- •2.4 SUMMARY
- •EXERCISES
- •3.1 DETERMINING COMPUTATION TIME
- •3.2 UNIPROCESSOR SCHEDULING
- •3.2.1 Scheduling Preemptable and Independent Tasks
- •3.2.2 Scheduling Nonpreemptable Tasks
- •3.2.3 Nonpreemptable Tasks with Precedence Constraints
- •3.2.5 Periodic Tasks with Critical Sections: Kernelized Monitor Model
- •3.3 MULTIPROCESSOR SCHEDULING
- •3.3.1 Schedule Representations
- •3.3.3 Scheduling Periodic Tasks
- •3.4 AVAILABLE SCHEDULING TOOLS
- •3.4.2 PerfoRMAx
- •3.4.3 TimeWiz
- •3.6 HISTORICAL PERSPECTIVE AND RELATED WORK
- •3.7 SUMMARY
- •EXERCISES
- •4.1 SYSTEM SPECIFICATION
- •4.2.1 Analysis Complexity
- •4.3 EXTENSIONS TO CTL
- •4.4 APPLICATIONS
- •4.4.1 Analysis Example
- •4.5 COMPLETE CTL MODEL CHECKER IN C
- •4.6 SYMBOLIC MODEL CHECKING
- •4.6.1 Binary Decision Diagrams
- •4.6.2 Symbolic Model Checker
- •4.7.1 Minimum and Maximum Delays
- •4.7.2 Minimum and Maximum Number of Condition Occurrences
- •4.8 AVAILABLE TOOLS
- •4.9 HISTORICAL PERSPECTIVE AND RELATED WORK
- •4.10 SUMMARY
- •EXERCISES
- •VISUAL FORMALISM, STATECHARTS, AND STATEMATE
- •5.1 STATECHARTS
- •5.1.1 Basic Statecharts Features
- •5.1.2 Semantics
- •5.4 STATEMATE
- •5.4.1 Forms Language
- •5.4.2 Information Retrieval and Documentation
- •5.4.3 Code Executions and Analysis
- •5.5 AVAILABLE TOOLS
- •5.6 HISTORICAL PERSPECTIVE AND RELATED WORK
- •5.7 SUMMARY
- •EXERCISES
- •6.1 SPECIFICATION AND SAFETY ASSERTIONS
- •6.4 RESTRICTED RTL FORMULAS
- •6.4.1 Graph Construction
- •6.5 CHECKING FOR UNSATISFIABILITY
- •6.6 EFFICIENT UNSATISFIABILITY CHECK
- •6.6.1 Analysis Complexity and Optimization
- •6.7.2 Timing Properties
- •6.7.3 Timing and Safety Analysis Using RTL
- •6.7.5 RTL Representation Converted to Presburger Arithmetic
- •6.7.6 Constraint Graph Analysis
- •6.8 MODECHART SPECIFICATION LANGUAGE
- •6.8.1 Modes
- •6.8.2 Transitions
- •6.9.1 System Computations
- •6.9.2 Computation Graph
- •6.9.3 Timing Properties
- •6.9.4 Minimum and Maximum Distance Between Endpoints
- •6.9.5 Exclusion and Inclusion of Endpoint and Interval
- •6.10 AVAILABLE TOOLS
- •6.11 HISTORICAL PERSPECTIVE AND RELATED WORK
- •6.12 SUMMARY
- •EXERCISES
- •7.1.1 Timed Executions
- •7.1.2 Timed Traces
- •7.1.3 Composition of Timed Automata
- •7.1.4 MMT Automata
- •7.1.6 Proving Time Bounds with Simulations
- •7.2.1 Untimed Traces
- •7.2.2 Timed Traces
- •7.3.1 Clock Regions
- •7.3.2 Region Automaton
- •7.4 AVAILABLE TOOLS
- •7.5 HISTORICAL PERSPECTIVE AND RELATED WORK
- •7.6 SUMMARY
- •EXERCISES
- •TIMED PETRI NETS
- •8.1 UNTIMED PETRI NETS
- •8.2 PETRI NETS WITH TIME EXTENSIONS
- •8.2.1 Timed Petri Nets
- •8.2.2 Time Petri Nets
- •8.3 TIME ER NETS
- •8.3.1 Strong and Weak Time Models
- •8.5.1 Determining Fireability of Transitions from Classes
- •8.5.2 Deriving Reachable Classes
- •8.6 MILANO GROUP’S APPROACH TO HLTPN ANALYSIS
- •8.6.1 Facilitating Analysis with TRIO
- •8.7 PRACTICALITY: AVAILABLE TOOLS
- •8.8 HISTORICAL PERSPECTIVE AND RELATED WORK
- •8.9 SUMMARY
- •EXERCISES
- •PROCESS ALGEBRA
- •9.1 UNTIMED PROCESS ALGEBRAS
- •9.2 MILNER’S CALCULUS OF COMMUNICATING SYSTEMS
- •9.2.1 Direct Equivalence of Behavior Programs
- •9.2.2 Congruence of Behavior Programs
- •9.2.3 Equivalence Relations: Bisimulation
- •9.3 TIMED PROCESS ALGEBRAS
- •9.4 ALGEBRA OF COMMUNICATING SHARED RESOURCES
- •9.4.1 Syntax of ACSR
- •9.4.2 Semantics of ACSR: Operational Rules
- •9.4.3 Example Airport Radar System
- •9.5 ANALYSIS AND VERIFICATION
- •9.5.1 Analysis Example
- •9.5.2 Using VERSA
- •9.5.3 Practicality
- •9.6 RELATIONSHIPS TO OTHER APPROACHES
- •9.7 AVAILABLE TOOLS
- •9.8 HISTORICAL PERSPECTIVE AND RELATED WORK
- •9.9 SUMMARY
- •EXERCISES
- •10.3.1 The Declaration Section
- •10.3.2 The CONST Declaration
- •10.3.3 The VAR Declaration
- •10.3.4 The INPUTVAR Declaration
- •10.3.5 The Initialization Section INIT and INPUT
- •10.3.6 The RULES Section
- •10.3.7 The Output Section
- •10.5.1 Analysis Example
- •10.6 THE ANALYSIS PROBLEM
- •10.6.1 Finite Domains
- •10.6.2 Special Form: Compatible Assignment to Constants,
- •10.6.3 The General Analysis Strategy
- •10.8 THE SYNTHESIS PROBLEM
- •10.8.1 Time Complexity of Scheduling Equational
- •10.8.2 The Method of Lagrange Multipliers for Solving the
- •10.9 SPECIFYING TERMINATION CONDITIONS IN ESTELLA
- •10.9.1 Overview of the Analysis Methodology
- •10.9.2 Facility for Specifying Behavioral Constraint Assertions
- •10.10 TWO INDUSTRIAL EXAMPLES
- •10.10.2 Specifying Assertions for Analyzing the FCE Expert System
- •Meta Rules of the Fuel Cell Expert System
- •10.11.1 General Analysis Algorithm
- •10.11.2 Selecting Independent Rule Sets
- •10.11.3 Checking Compatibility Conditions
- •10.12 QUANTITATIVE TIMING ANALYSIS ALGORITHMS
- •10.12.1 Overview
- •10.12.2 The Equational Logic Language
- •10.12.3 Mutual Exclusiveness and Compatibility
- •10.12.5 Program Execution and Response Time
- •10.12.8 Special Form A and Algorithm A
- •10.12.9 Special Form A
- •10.12.10 Special Form D and Algorithm D
- •10.12.11 The General Analysis Algorithm
- •10.12.12 Proofs
- •10.13 HISTORICAL PERSPECTIVE AND RELATED WORK
- •10.14 SUMMARY
- •EXERCISES
- •11.1 THE OPS5 LANGUAGE
- •11.1.1 Overview
- •11.1.2 The Rete Network
- •11.2.1 Static Analysis of Control Paths in OPS5
- •11.2.2 Termination Analysis
- •11.2.3 Timing Analysis
- •11.2.4 Static Analysis
- •11.2.5 WM Generation
- •11.2.6 Implementation and Experiment
- •11.3.1 Introduction
- •11.3.3 Response Time of OPS5 Systems
- •11.3.4 List of Symbols
- •11.3.5 Experimental Results
- •11.3.6 Removing Cycles with the Help of the Programmer
- •11.4 HISTORICAL PERSPECTIVE AND RELATED WORK
- •11.5 SUMMARY
- •EXERCISES
- •12.1 INTRODUCTION
- •12.2 BACKGROUND
- •12.3 BASIC DEFINITIONS
- •12.3.1 EQL Program
- •12.3.4 Derivation of Fixed Points
- •12.4 OPTIMIZATION ALGORITHM
- •12.5 EXPERIMENTAL EVALUATION
- •12.6 COMMENTS ON OPTIMIZATION METHODS
- •12.6.1 Qualitative Comparison of Optimization Methods
- •12.7 HISTORICAL PERSPECTIVE AND RELATED WORK
- •12.8 SUMMARY
- •EXERCISES
- •BIBLIOGRAPHY
- •INDEX
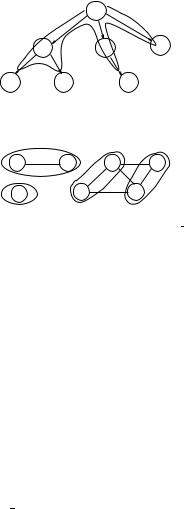
352 DESIGN AND ANALYSIS OF PROPOSITIONAL-LOGIC RULE-BASED SYSTEMS
|
1 1 |
|
|
|
1 |
|
|
|
6 |
4 |
|
|
|
|
3 1 |
3 2 |
|
7 1 |
|
2 |
3 |
|
|
|
1 2 |
2 2 |
|
5 |
4 1 |
Figure 10.18 Traversing orders by the subrule 11.
1 |
2 |
5 |
7 |
4 |
3 |
|
6 |
Figure 10.19 The ME graph of Program 3.
larly, N I12 = 1, N I21 = 5, N I22 = 1, N I31 = 7, N I32 = 2, N I41 = 1, N I51 = 1, N I61 = 1, and N I71 = 1.
Step 2 results in
N I1 = N I11 + N I12 = 7 + 1 = 8,
N I2 = N I21 + N I22 = 5 + 1 = 6,
N I3 = N I31 + N I32 = 7 + 1 = 8,
N I4 = N I41 = 1,
N I5 = N I51 = 1,
N I6 = N I61 = 1, and
N I7 = N I71 = 1.
The ME graph of Program 3 is then partitioned into four subgraphs by step 3, as shown in Figure 10.19. Finally, step 4 finds that
Tp = max(N I1, N I2) + max(N I3, N I5) + max(N I4) + max(N I6, N I7)
=8 + 8 + 1 + 1
=18.
10.12.11 The General Analysis Algorithm
In the previous sections, we showed the existence of two special forms and presented two algorithms to obtain upper bounds on the numbers of rule firings for programs in these two special forms. However, for any EQL program p, it is rarely the case that p as a whole is in a known special form. Instead, it is frequently found that p can
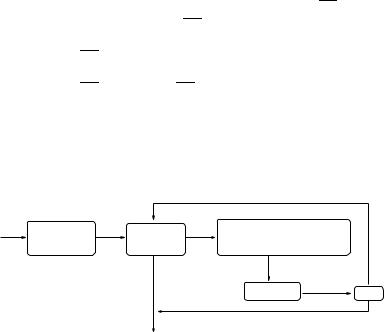
QUANTITATIVE TIMING ANALYSIS ALGORITHMS |
353 |
be partitioned into a hierarchy of sets of rules such that some of these sets are in a known special form. To exploit this property and to enhance the applicability of the two algorithms presented, the general analysis algorithm (GAA) has been developed and is reported in [Cheng and Wang, 1990].
Assume the program p can be partitioned into two sets, S and S = p − S, such that S is independent of S . Let tS denote the number of rule firings during the execution of S under the circumstances that S is regarded as an independent program. Similarly, let tS denote the number of rule firings during the execution of S under the circumstances that S is regarded as an independent program. According to the underlying execution model, the execution of the program p can be regarded as the execution of S, followed by the execution of S . Hence, the number of times that the rules in S are fired during the execution of p is equal to tS , and the number of times that the rules in S are fired during the same period of time is equal to tS . The number of rule firings during the execution of p is thus equal to the value of tS + tS . Hence, the value of an upper bound on tS plus the value of an upper bound on tS is an upper bound on the number of rule firings during the execution of p. If both tS and tS have finite upper bounds, a finite upper bound exists on the number of rule firings during the execution of p. On the other hand, if either tS or tS does not have a finite upper bound, a finite upper bound does not exist on the number of rule firings during the execution of p. Hence, p has a bounded response time if and only if both S and S have bounded response times. The GAA [Cheng et al., 1991], shown in Figure 10.20, exploits the above arguments and makes use of the HLD graph.
Suppose p is the program to be analyzed. First, the HLD graph G p is constructed. During the process of analysis, p and G p are modified to reflect the progress of analysis. In general, suppose p is the set of rules remaining to be analyzed at a point during analysis and G p is the corresponding HLD graph. In each iteration, GAA selects (and deletes) from p a subset of rules, S, corresponding to a vertex without an out-going edge in G p (and modifies G p accordingly), since the rules in this subset have the highest priorities among the remaining rules and the execution of this subset is not affected by the execution of other subsets.
S is then checked against known special forms to see if S is in a known special form. If it is, the corresponding response time algorithm is used to obtain an upper bound of S; otherwise, the aforementioned temporal-logic model checker and timing
PConstruct the HLD graph
HLD graph no Select an independent set S
empty?
no
yes
Exit?
yes
Exit
Figure 10.20 The general analysis algorithm.
354 DESIGN AND ANALYSIS OF PROPOSITIONAL-LOGIC RULE-BASED SYSTEMS
analyzer are used to conduct an exhaustive check on the state-space graph of S to obtain the upper bound. If S does not have a finite upper bound on the number of rule firings, neither does p; otherwise, the obtained upper bound is accumulated into a register. The analysis process is repeatedly applied to p until there is no rule left or one subset is found not to have a finite upper bound.
Although the GAA still needs to conduct exhaustive state-space graph checks in some cases, the sizes of state-space graphs checked in most cases have been effectively reduced with the help of special forms, resulting in an obvious reduction in the time spent in analyzing real-time rule-based systems. By using the GAA, we have successfully reduced the time needed to analyze the aforementioned real-life realtime rule-based systems. For example, the complete EQL program for the Cryogenic Hydrogen Pressure Malfunction Procedure of the Space Shuttle Vehicle Pressure Control System consists of 36 rules, 31 sensor input variables, and 32 non-input variables. It took two weeks for a set of tools relying mainly on an exhaustive state-space graph check to complete the analysis. However, GAA verified that this program can be partitioned into forward-independent subsets such that each of these subsets is in Special Form A. This fact contributes to the huge reduction in time needed—from two weeks to two seconds—for a set of tools employing GAA (equipped with Special Form A only) to complete the analysis.
Another advantage of GAA, in case the analyzed program is found not to have a finite upper bound, is that the subset that results in this property is isolated from the remaining rules, since GAA stops analyzing once it finds a subset without a finite upper bound.
10.12.12 Proofs
Proof of Theorem 5. Assume p is in Special Form A and r is a rule in p. Due to (A3) (i.e., L p ∩ Tp = ), the value of the enabling condition of r remains unchanged throughout the execution of p. Hence, r remains enabled/disabled throughout the execution of p if it is enabled/disabled at the invocation of p.
•If r is disabled at the invocation of p, it will not be fired during the execution of p. Hence, it will contribute 0 with respect to the number of rule firings.
•If r is enabled at the invocation, it may or may not be fired during the execution of p, depending on the progress of the system. If it is not fired during the exe-
cution of p, it will still contribute 0 with respect to the number of rule firings. If it is fired, it will not be fired again due to (A1), unless there is another rule r
firing later and assigning a distinct value to a variable in Lr ∩ Lr . However, due to (A2), r is disabled if it disagrees with r on the value assigned to a variable in Lr ∩ Lr . Hence, r will be fired at most once during the execution of p. It will contribute 1 with respect to the number of rule firings.
The above argument applies to each rule in p. Since there are n rules in p, obviously there are at most n rule firings during the execution of p. That is, T p = n.
QUANTITATIVE TIMING ANALYSIS ALGORITHMS |
355 |
Proof of Theorem 6. Assume p is in Special Form D and does not always reach a fixed point in a bounded number of rule firings. This means that an execution of p exists that does not terminate in any bounded number of rule firings. Since only a finite number of rules in p exist, a rule r1 must be firing infinitely often during this execution. According to the underlying execution model, r1 can be fired only if its firing changes the value of one variable in p. Since only a finite number of variables in Lr 1 exist, a variable x1 Lr 1 must be assigned a new value by a subrule ri1 infinitely often.
Based on the values of Rp ∩ L p and Tp ∩ L p , we divide the proof into four parts. To simplify the notations used next, the notation ri is used to stand for the specific subrule of the rule ri that assigns a value to a variable involved in the reasoning, unless otherwise stated. For example, without ambiguity, the subrule ri1 will be referred to as r1.
1.Rp ∩L p = and Tp ∩L p = . p is also a Special Form A program. According to Theorem 5, p always reaches a fixed point in a bounded number of rule firings.
2.Rp ∩ L p = and Tp ∩ L p = . Due to Rp ∩ L p = , r1 always assigns the constant value m1 to x1. r1 alone can change the value of x1 at most once if
another subrule does not exist that assigns a different value to x1. Hence, there must be a rule r1 with a subrule r1 that assigns a distinct value m1 to x1 and is fired infinitely often.
Due to (D1), r1 and r1 mutually exclude each other. There must be a vari-
able x2 whose value changes infinitely often and determines the enabledness |
|||||
of r1 |
and r1 . Now the argument applied to x1 also applies to x2. This means |
||||
|
|
|
and r2 |
|
|
there is a pair of subrules, r2 |
, assigning distinct values to x2 infinitely |
||||
|
2 |
|
|
1 |
|
often. One of them, say r |
, potentially enables r , while the other potentially |
||||
enables r1 . Hence, there exist the edges r2, r1 and r2 , r1 in the RD graph |
|||||
G R D |
. In addition, the argument applied to the pair of r1 |
and r1 also applies to |
|||
p |
|
|
|
|
|
the pair of r2 and r2 . We continue to apply the same arguments to the variables
and rules encountered.
Two paths, S = rk , rk−1, . . . , r2, r1 and S = rk , r(k−1)
will be found. However, since there are only a finite number of rules (and thus subrules) in p, eventually, for some k, both rk and rk will turn out to be subrules already encountered previously. One of three situations will happen:
(a)rk {rk−1, rk−2, . . . , r2, r1} and rk {r(k−1) , r(k−2) , . . . , r2 , r1 }.
Assume rk = ri and rk = r j . Then rk = ri , rk−1, . . . , ri+1, ri
(k−1) , . . . , r( j+1) , r j forms another
are respec-
tively contained in disjoint simple cycles and assign distinct values to xk , contradicting (D4).
(b)Both rk and rk belong to {rk−1, rk−2, . . . , r2, r1}. There are two cases to consider:
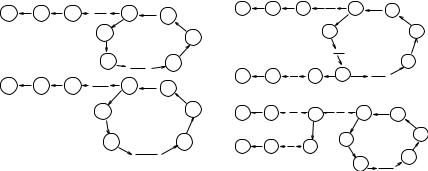
356 DESIGN AND ANALYSIS OF PROPOSITIONAL-LOGIC RULE-BASED SYSTEMS
S |
1 |
2 |
3 |
i |
|
|
|
|
k-1 |
S’ |
1’ |
2’ |
3’ |
j’ |
|
|
|
|
k-1’ |
i+1
j+1’
S
S’
S
S’
1 |
2 |
3 |
i |
i+1 |
|
|
k-1 |
|
|
1’ |
2’ |
k-1’ |
j |
|
|
|
(b) |
|
|
1 |
2 |
j |
i |
i+1 |
1’ |
2’ |
|
k-1 |
|
k-1’ |
|
|
(a) |
(c) |
|
Figure 10.21 Rule enabling patterns. |
• rk |
= ri |
and rk |
= r j , |
where j |
> i. In |
this case, |
rk = |
||||
ri , rk−1, . . . , r j = rk , . . . , ri forms a cycle C, as shown in |
|||||||||||
Figure 10.21(b). Hence, rk |
and rk are contained in C and respec- |
||||||||||
|
|
|
|
|
|
|
|
|
|
|
|
tively assign distinct values to xk , contradicting (D3). |
|
||||||||||
• rk |
= ri |
and rk |
= r j , |
where j |
< i. In |
this case, |
rk = |
||||
ri |
, rk−1, . . . , ri+1 |
, ri |
forms a cycle C, as |
shown in |
Figure |
||||||
|
|
|
|
|
j |
|
|
|
|
values |
to xk . |
10.21(c). ri and |
|
r respectively assign distinct |
|||||||||
Now the argument applied to the pair r1 and r1 |
also applies to |
||||||||||
the pair ri |
and r |
j |
|
|
|
|
|
|
|
|
|
|
. In addition, we know at this time that there is a |
||||||||||
|
|
|
j |
|
|
|
|
j |
, we conduct a |
||
path from ri to r |
. Hence, starting from ri and r |
new sequence of reasoning, resulting in another new sequence of reasoning.
We continue to apply the same argument to pairs of subrules encountered. A sequence of paths, each of which connects a pair of subrules disagreeing on the expression assigned to a variable, will be found. Eventually, since only a finite number of rules in p exist, a rule previously encountered will be encountered again. A cycle containing rules disagreeing on the expression assigned to a variable will be found, contradicting (D3).
(c) Both rk and rk belong to {r(k−1) , r(k−2) , . . . , r2 , r1 }. With {r(k−1) , r(k−2) , . . . , r2 , r1 } replacing {rk−1, rk−2, . . . , r2, r1}, the argument
in the previous situation also applies to this situation. Hence, this situation will not happen, either.
Since none of the three situations can possibly happen, it is not possible for p not to reach a fixed point in a bounded number of rule firings if Rp ∩ L p = and Tp ∩ L p = .
3.Rp ∩ L p = and Tp ∩ L p = . Assume r1 is of the form x := f | · · · IF EC. Due to (D1) and Tp ∩ L p = , any rule disagreeing with r1 on the expression
QUANTITATIVE TIMING ANALYSIS ALGORITHMS |
357 |
assigned to x remains disabled throughout this execution of p. The fact that r1 assigns a new value to x infinitely often means that f changes its value infinitely often. Hence, f must be a function in at least one variable such that it can change its value, otherwise it is a constant expression and cannot change its value. Only two possible situations exist for f to be a function in variables. We now discuss both situations and prove they cannot occur.
(a) |
f is a function in x (i.e., r is of the form x := |
f (x) |
| |
EC). |
|
|
· · · IFRD |
||
|
However, this means that a one-vertex cycle C would exist in G p , if |
|||
|
such an r did exist. It is not possible to have a pair of subrules in C such |
|||
|
that they are compatible by mutual exclusiveness, since C contains only |
|||
|
one vertex. This would violate (D5). Hence, this situation cannot occur. |
(b) f is a function in a variable y that is different from the variable x (i.e., r is of the form x := f (y) | · · · IF EC). There is a subrule r1 of the form x := f (y) IF EC, and y (and thus f (y)) changes its value infinitely often. There must be a rule r2 (and a subrule r2) assigning a value to y and firing infinitely often. Now, the argument applied to r1 also applies to r2. We continue to apply the same argument to each rule encountered. Eventually, since there are only a finite number of rules in p, the subrules encountered form a cycle C in GRDp . Since the subrules in C can be fired one after another infinitely often, C is not a convergency cycle and all of the subrules in C are enabled at the same time, violating (D5). Hence, this situation cannot occur either.
Since neither of the two situations can possibly happen, it is not possible for p not to reach a fixed point in a bounded number of rule firings if Rp ∩ L p =
and Tp ∩ L p = . |
1 |
is |
|
|
|
· · · | |
|
1 := |
|
1 | · · · |
4. Rp ∩ L p = and Tp ∩ L p = . Assume r |
|
1 |
|
x |
f |
|||||
|
|
of the form |
|
|
|
|||||
IF EC, where f1 is an expression. The firing of r |
|
changes the value of x1 only |
if (1) the value of f1 has changed such that it is not equal to the old value of x1 or (2) a new value has been assigned to x1 by another rule, say r1 , such that the new value of x1 is not equal to the value of f1. There are two cases involving the value of f1: constant or not constant.
• f1 is a constant expression. This means r1 can change the value of x1 at most once if another rule does not exist assigning a distinct value to x1. Since r1 assigns a new value to x1 infinitely often, there must be another rule r1 assigning a distinct value to x1 infinitely often. r1 and r1 mutually exclude each other, due to (D1). Hence, a variable x2 exists whose value changes infinitely often and determines the enabledness of r1 and r1 . That, in turn, means there is a rule r2 that assigns a new value to x2 infinitely often and potentially enables r1. Hence, the ER edge r2, r1 exists in GRDp . Now, the argument applied to the pair of x1 and r1 also applies to the pair of x2 and r2.
• f1 is not a constant expression. f1 must be a variable expression either in x1 or not x1.
358 DESIGN AND ANALYSIS OF PROPOSITIONAL-LOGIC RULE-BASED SYSTEMS
i. |
f1 |
is an expression in x1 |
(i.e., r1 is of the form · · · | x1 := |
||||||||
|
f (x |
1) |
| · · · IF EC). |
However, this means that there would be a |
|||||||
|
|
|
|
RD |
|
||||||
|
one-vertex VM cycle in G p , if such an r did exist. As mentioned |
||||||||||
|
earlier, this would violate (D5). Hence, this situation will not |
||||||||||
|
occur. |
|
|
|
|
|
|
|
|||
ii. |
f1 |
is |
not |
an expression |
in x1. f1 must be |
an expression in |
|||||
|
a |
variable |
different |
from |
x1, say x2, (i.e., r1 is of the form |
||||||
|
· ·1 |
· |
|
| |
x1 |
:= f (x2) |
| · · · IF EC). Hence, |
there is a subrule |
|||
|
r |
|
of |
the |
form x1 := f (x2) IF EC, and the |
value of x2 (and |
|||||
|
|
|
|
|
|
|
|
|
|
|
|
thus f (x2)) changes infinitely often. A rule r2 may exist that
assigns a new value to x2 and fires infinitely often. Hence, there is a VM edge r2, r1 in GRDp . Now, the argument ap-
plied to the pair of r1 and x1 also applies to the pair of r2 and x2.
We continue to apply the argument above to each rule and variable pair encountered. A path of ER edges or VM edges or both will be found. Eventually, since only a finite number of rules exist in p, a rule and variable pair that has been encountered previously will be encountered again. Hence, a cycle C will be found. One of three situations will happen (to simplify the explanation, if a variable x is assigned a value by a subrule in C, we say x is in C):
(a)C is an EV cycle. However, the existence of an EV cycle would violate (D2). Thus, this situation will not occur.
(b)C is an ER cycle. For each pair of ri and xi in C, the value of fi assigned to xi by ri is a constant. This means that xi is also assigned
a distinct value infinitely often by another rule ri that enables r(i−1) . Hence, the ER edge ri , r(i−1) also exists in GRDp . A path consisting
of ri , r(i−1) , . . . , r2 , r1 in GRDp shall also exist. Since there are only a finite number of rules in p, eventually we will end up with the same
situation as we did in part (2) of this proof. That means this situation will not occur either.
(c)C is a VM cycle. For each variable xi in C, xi gets a new value because the expression f (xi+1) assigned to xi by ri changes infinitely often. Since the subrules in C can be fired one after another in the order they
appear in C for an unlimited number of times, it is obvious that C is
not a convergent cycle. In addition, for each subrule ri in C, ri must be enabled at the time when it is ri ’s turn to be fired. If all of the subrules in C can be enabled at the same time, then (D5) is violated. On the other hand, if all of the subrules (vertices) contained in C cannot be
enabled at the same time, there must be at least one disabled rule at any
moment. Assume rk is disabled at some moment during the execution considered. Hence, there must be a subrule, say g1, whose firing will enable rk . There are two cases to consider:
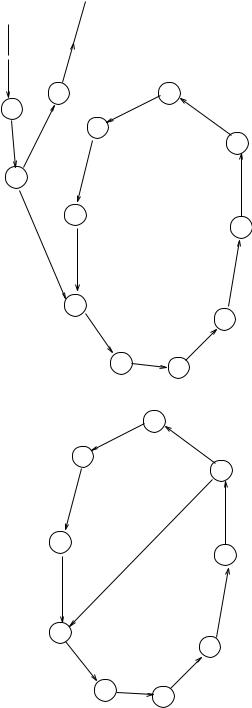
g1
ER
VM
ri+2
VM
i+1 r
VM
i r
VM
VM
|
VM |
|
ri+2 |
VM |
|
i+1 |
ER |
r |
|
VM
i r
VM
VM
VM
|
VM |
|
(b) |
|
VM |
VM |
VMcycles. |
10.22 |
|
VM |
Figure |
|
g1 |
|
VM |
|
(a) |
|
VM |
VM
359