
- •CONTENTS
- •PREFACE
- •LIST OF FIGURES
- •INTRODUCTION
- •1.1 WHAT IS TIME?
- •1.2 SIMULATION
- •1.3 TESTING
- •1.4 VERIFICATION
- •1.6 USEFUL RESOURCES
- •2.1 SYMBOLIC LOGIC
- •2.1.1 Propositional Logic
- •2.1.2 Predicate Logic
- •2.2 AUTOMATA AND LANGUAGES
- •2.2.1 Languages and Their Representations
- •2.2.2 Finite Automata
- •2.3 HISTORICAL PERSPECTIVE AND RELATED WORK
- •2.4 SUMMARY
- •EXERCISES
- •3.1 DETERMINING COMPUTATION TIME
- •3.2 UNIPROCESSOR SCHEDULING
- •3.2.1 Scheduling Preemptable and Independent Tasks
- •3.2.2 Scheduling Nonpreemptable Tasks
- •3.2.3 Nonpreemptable Tasks with Precedence Constraints
- •3.2.5 Periodic Tasks with Critical Sections: Kernelized Monitor Model
- •3.3 MULTIPROCESSOR SCHEDULING
- •3.3.1 Schedule Representations
- •3.3.3 Scheduling Periodic Tasks
- •3.4 AVAILABLE SCHEDULING TOOLS
- •3.4.2 PerfoRMAx
- •3.4.3 TimeWiz
- •3.6 HISTORICAL PERSPECTIVE AND RELATED WORK
- •3.7 SUMMARY
- •EXERCISES
- •4.1 SYSTEM SPECIFICATION
- •4.2.1 Analysis Complexity
- •4.3 EXTENSIONS TO CTL
- •4.4 APPLICATIONS
- •4.4.1 Analysis Example
- •4.5 COMPLETE CTL MODEL CHECKER IN C
- •4.6 SYMBOLIC MODEL CHECKING
- •4.6.1 Binary Decision Diagrams
- •4.6.2 Symbolic Model Checker
- •4.7.1 Minimum and Maximum Delays
- •4.7.2 Minimum and Maximum Number of Condition Occurrences
- •4.8 AVAILABLE TOOLS
- •4.9 HISTORICAL PERSPECTIVE AND RELATED WORK
- •4.10 SUMMARY
- •EXERCISES
- •VISUAL FORMALISM, STATECHARTS, AND STATEMATE
- •5.1 STATECHARTS
- •5.1.1 Basic Statecharts Features
- •5.1.2 Semantics
- •5.4 STATEMATE
- •5.4.1 Forms Language
- •5.4.2 Information Retrieval and Documentation
- •5.4.3 Code Executions and Analysis
- •5.5 AVAILABLE TOOLS
- •5.6 HISTORICAL PERSPECTIVE AND RELATED WORK
- •5.7 SUMMARY
- •EXERCISES
- •6.1 SPECIFICATION AND SAFETY ASSERTIONS
- •6.4 RESTRICTED RTL FORMULAS
- •6.4.1 Graph Construction
- •6.5 CHECKING FOR UNSATISFIABILITY
- •6.6 EFFICIENT UNSATISFIABILITY CHECK
- •6.6.1 Analysis Complexity and Optimization
- •6.7.2 Timing Properties
- •6.7.3 Timing and Safety Analysis Using RTL
- •6.7.5 RTL Representation Converted to Presburger Arithmetic
- •6.7.6 Constraint Graph Analysis
- •6.8 MODECHART SPECIFICATION LANGUAGE
- •6.8.1 Modes
- •6.8.2 Transitions
- •6.9.1 System Computations
- •6.9.2 Computation Graph
- •6.9.3 Timing Properties
- •6.9.4 Minimum and Maximum Distance Between Endpoints
- •6.9.5 Exclusion and Inclusion of Endpoint and Interval
- •6.10 AVAILABLE TOOLS
- •6.11 HISTORICAL PERSPECTIVE AND RELATED WORK
- •6.12 SUMMARY
- •EXERCISES
- •7.1.1 Timed Executions
- •7.1.2 Timed Traces
- •7.1.3 Composition of Timed Automata
- •7.1.4 MMT Automata
- •7.1.6 Proving Time Bounds with Simulations
- •7.2.1 Untimed Traces
- •7.2.2 Timed Traces
- •7.3.1 Clock Regions
- •7.3.2 Region Automaton
- •7.4 AVAILABLE TOOLS
- •7.5 HISTORICAL PERSPECTIVE AND RELATED WORK
- •7.6 SUMMARY
- •EXERCISES
- •TIMED PETRI NETS
- •8.1 UNTIMED PETRI NETS
- •8.2 PETRI NETS WITH TIME EXTENSIONS
- •8.2.1 Timed Petri Nets
- •8.2.2 Time Petri Nets
- •8.3 TIME ER NETS
- •8.3.1 Strong and Weak Time Models
- •8.5.1 Determining Fireability of Transitions from Classes
- •8.5.2 Deriving Reachable Classes
- •8.6 MILANO GROUP’S APPROACH TO HLTPN ANALYSIS
- •8.6.1 Facilitating Analysis with TRIO
- •8.7 PRACTICALITY: AVAILABLE TOOLS
- •8.8 HISTORICAL PERSPECTIVE AND RELATED WORK
- •8.9 SUMMARY
- •EXERCISES
- •PROCESS ALGEBRA
- •9.1 UNTIMED PROCESS ALGEBRAS
- •9.2 MILNER’S CALCULUS OF COMMUNICATING SYSTEMS
- •9.2.1 Direct Equivalence of Behavior Programs
- •9.2.2 Congruence of Behavior Programs
- •9.2.3 Equivalence Relations: Bisimulation
- •9.3 TIMED PROCESS ALGEBRAS
- •9.4 ALGEBRA OF COMMUNICATING SHARED RESOURCES
- •9.4.1 Syntax of ACSR
- •9.4.2 Semantics of ACSR: Operational Rules
- •9.4.3 Example Airport Radar System
- •9.5 ANALYSIS AND VERIFICATION
- •9.5.1 Analysis Example
- •9.5.2 Using VERSA
- •9.5.3 Practicality
- •9.6 RELATIONSHIPS TO OTHER APPROACHES
- •9.7 AVAILABLE TOOLS
- •9.8 HISTORICAL PERSPECTIVE AND RELATED WORK
- •9.9 SUMMARY
- •EXERCISES
- •10.3.1 The Declaration Section
- •10.3.2 The CONST Declaration
- •10.3.3 The VAR Declaration
- •10.3.4 The INPUTVAR Declaration
- •10.3.5 The Initialization Section INIT and INPUT
- •10.3.6 The RULES Section
- •10.3.7 The Output Section
- •10.5.1 Analysis Example
- •10.6 THE ANALYSIS PROBLEM
- •10.6.1 Finite Domains
- •10.6.2 Special Form: Compatible Assignment to Constants,
- •10.6.3 The General Analysis Strategy
- •10.8 THE SYNTHESIS PROBLEM
- •10.8.1 Time Complexity of Scheduling Equational
- •10.8.2 The Method of Lagrange Multipliers for Solving the
- •10.9 SPECIFYING TERMINATION CONDITIONS IN ESTELLA
- •10.9.1 Overview of the Analysis Methodology
- •10.9.2 Facility for Specifying Behavioral Constraint Assertions
- •10.10 TWO INDUSTRIAL EXAMPLES
- •10.10.2 Specifying Assertions for Analyzing the FCE Expert System
- •Meta Rules of the Fuel Cell Expert System
- •10.11.1 General Analysis Algorithm
- •10.11.2 Selecting Independent Rule Sets
- •10.11.3 Checking Compatibility Conditions
- •10.12 QUANTITATIVE TIMING ANALYSIS ALGORITHMS
- •10.12.1 Overview
- •10.12.2 The Equational Logic Language
- •10.12.3 Mutual Exclusiveness and Compatibility
- •10.12.5 Program Execution and Response Time
- •10.12.8 Special Form A and Algorithm A
- •10.12.9 Special Form A
- •10.12.10 Special Form D and Algorithm D
- •10.12.11 The General Analysis Algorithm
- •10.12.12 Proofs
- •10.13 HISTORICAL PERSPECTIVE AND RELATED WORK
- •10.14 SUMMARY
- •EXERCISES
- •11.1 THE OPS5 LANGUAGE
- •11.1.1 Overview
- •11.1.2 The Rete Network
- •11.2.1 Static Analysis of Control Paths in OPS5
- •11.2.2 Termination Analysis
- •11.2.3 Timing Analysis
- •11.2.4 Static Analysis
- •11.2.5 WM Generation
- •11.2.6 Implementation and Experiment
- •11.3.1 Introduction
- •11.3.3 Response Time of OPS5 Systems
- •11.3.4 List of Symbols
- •11.3.5 Experimental Results
- •11.3.6 Removing Cycles with the Help of the Programmer
- •11.4 HISTORICAL PERSPECTIVE AND RELATED WORK
- •11.5 SUMMARY
- •EXERCISES
- •12.1 INTRODUCTION
- •12.2 BACKGROUND
- •12.3 BASIC DEFINITIONS
- •12.3.1 EQL Program
- •12.3.4 Derivation of Fixed Points
- •12.4 OPTIMIZATION ALGORITHM
- •12.5 EXPERIMENTAL EVALUATION
- •12.6 COMMENTS ON OPTIMIZATION METHODS
- •12.6.1 Qualitative Comparison of Optimization Methods
- •12.7 HISTORICAL PERSPECTIVE AND RELATED WORK
- •12.8 SUMMARY
- •EXERCISES
- •BIBLIOGRAPHY
- •INDEX
280 DESIGN AND ANALYSIS OF PROPOSITIONAL-LOGIC RULE-BASED SYSTEMS
10.6 THE ANALYSIS PROBLEM
The analysis problem is to decide whether a given real-time equational rule-based program meets the specified timing constraints as well as integrity constraints. Since the formulation of our problem is in terms of state-space graphs, our approach is compatible with the semantics of temporal logic: in spite of the many variations of temporal logic, their semantics are usually defined in terms of Kripke (state space) structures. Hence, the issue of verifying that an equational rule-based program meets a set of integrity constraints can be treated by appealing to temporal-logic techniques in a straightforward manner. Since the application of temporal logic to program verification is quite well understood, it suffices to note that we have integrated a model checker for the temporal-logic CTL [Clarke, Emerson, and Sistla, 1986] into our suite of tools, as was shown in the previous section. The focus of this chapter is on meeting timing constraints.
There are many types of timing constraints in real-time decision systems. The fundamental requirement is to be able to bound the response time of the decision system. We capture the response time of an equational rule-based program by the length of the monitor-decide cycle, that is, the time it takes for all the program variables to settle down to stable values. Technically, the analysis problem of interest is to decide whether a fixed point can always be reached from a launch state on any sufficiently long but finite path. In general, the analysis problem is undecidable if the program variables can have infinite domains, that is, no general procedure exists for answering all instances of the decision problem.
The undecidability result follows from the observation that any two-counter machine can be encoded by an equational rule-based program that uses only “+” and “−” as operations on integer variables and “>” and “=” as atomic predicates such that a two-counter machine accepts an input if and only if the corresponding equational rule-based program can reach a fixed point from an initial condition determined by the input to the two-counter machine. Since two-counter machines can simulate arbitrary Turing machines, our analysis problem is equivalent to the Turing machine halting problem. The formal proof of this result is straightforward but tedious and is omitted here. We illustrate the idea of the proof by exhibiting a twocounter machine (Figure 10.6) and the corresponding equational rule-based program. This two-counter machine accepts the integer input in its first register iff it is odd. The same input integer is used to initialize the variable c1 in the program below. The variables s and f are used to keep track of respectively the current state of the two-counter machine and whether the two-counter machine has entered an accepting state. Notice that the program below reaches a fixed point iff only rule 5 is enabled.
Equational rule-based program for simulating the two-counter machine of Figure 10.6.
initially: s = 1, c1 = INPUT, c2 = 0, f = 0
1.s := 2 ! c1 := c1 − 1! f := f + 1 IF s = 1 AND c1 > 0
2.[] s := 3 ! c1 := c1! f := f + 1 IF s = 1 AND c1 = 0
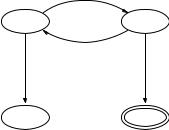
THE ANALYSIS PROBLEM |
281 |
+,*-1,*
P1 |
P2 |
|
+,*-1,* |
0,*0,* |
0,*0,* |
P3 |
P4 |
Figure 10.6 A two-counter machine for testing odd input.
3. [] s := 3 ! c1 := c1! f := f + 1 IF s = 3
4.[] s := 4 ! c1 := c1! f := f + 1 IF s = 2 AND c1 = 0
5.[] s := 4 ! c1 := c1! f := f IF s = 4
6.[] s := 1 ! c1 := c1 − 1! f := f + 1 IF s = 2 AND c1 > 0
10.6.1 Finite Domains
Even though the analysis problem is undecidable in general, it is trivially true that the analysis problem is decidable if all the variables of an equational rule-based program range over finite domains. In this case, the state-space graph of the program must be finite and can thus be analyzed by an algorithm that performs an exhaustive check on the finite graph. In section 10.5, we described a suite of tools for analyzing equational rule-based programs. The default approach is to generate the reachability graph from the initial (launch) state and use the model checker to determine whether a fixed point is always reachable on any path from the initial state. (Fixed points are expressed by an atomic predicate on a state that is true if and only if out-edges from the state are self-loops.) This approach is viable if the state-space graph is reasonably small, but in the worst case may require exponential computation time as a function of the number of variables in the program. More precisely, it can be shown that the computational complexity of the analysis problem restricted to finite graphs is PSPACE-complete.
It should be emphasized that in practice, it is often not necessary to check the complete state space to solve the analysis problem. Under appropriate conditions, efficient procedures exist that can be applied to reduce the size of the state space by a simple textual analysis of the program. In particular, rules of certain forms are always guaranteed to reach a fixed point in a finite number of iterations. As an example, one of these special forms4 which is especially useful, will be given below. First, some definitions are in order.
4It is unnecessary for all the rules of a program to be in a special form in order to be able to reduce the state space. Techniques exist that can be applied recursively to fragments of a program and the result can be used to transform the whole program into a simpler one.

282 DESIGN AND ANALYSIS OF PROPOSITIONAL-LOGIC RULE-BASED SYSTEMS
We defined earlier three sets of variables for an equational rule-based program. They are repeated below for convenience.
L = {v | v is a variable appearing in LHS}
R = {v | v is a variable appearing in RHS}
T = {v | v is a variable appearing in EC}
Let T = {v1, v2, . . . , vn } and let v¯ be the vector v1, v2, . . . , vn . With this definition, each test (enabling condition) in a program can be viewed as a function f (v)¯ from the space of v¯ to the set {true, false}. Let fa be the function corresponding to the test a and let Va be the subset of the space of v¯ for which the function fa maps to true. We say that two tests a and b are mutually exclusive iff the subsets Va and Vb of the corresponding functions fa and fb are disjoint. Obviously, if two tests are mutually exclusive, only one of the corresponding rules can be enabled at a time.
For some rules, it is straightforward to determine if two tests are mutually exclusive. For example, consider tests of the form:
C1 AND C2 AND · · · AND Cm ,
where each Ci is a predicate of the form
variable relational operator constant
For a test a of this form, it is easy to see that the subset Va of the space of v for which fa maps to true can be expressed as the cross product:
Va,1 × Va,2 × · · · × Va,n
such that fa (v)¯ maps to true iff the ith component of v¯ is in Va,i , for i = 1, . . . , n. Note that if the variable vk does not appear in test a, then Va,k is the entire domain of vk . To verify that two tests a and b are mutually exclusive, it suffices to find at least one variable vi for which Va,i ∩ Vb,i = . If no such vi is found, the two tests are not mutually exclusive.
Let Lx denote the set of variables appearing in the left-hand-side (LHS) of rule x. Two rules a and b are said to be compatible iff at least one of the following conditions holds:
(CR1) Test a and test b are mutually exclusive (CR2) La ∩ Lb =
(CR3) Suppose La ∩ Lb = . Then for every variable v in La ∩ Lb, the same expression must be assigned to v in both rule a and rule b.
We are now ready to give a special form of rules for which the analysis problem can be solved efficiently.
THE ANALYSIS PROBLEM |
283 |
10.6.2Special Form: Compatible Assignment to Constants, L and T Disjoint
A set of rules are said to be in special form if all three of the following conditions hold.
1.Constant terms are assigned to all the variables in L, that is, R = .
2.All of the rules are compatible pairwise.
3.L ∩ T = .
We claim that an equational rule-based program whose rules are in special form will always reach a fixed point in a finite number of iterations. The fact that L and T are disjoint means that the logical value of every test will remain constant throughout an invocation once all sensor readings have been taken and assigned to the input variables. Thus condition (3) implies that a rule is either enabled or disabled throughout an invocation of the program. So we need only to focus on the set of rules that are enabled. If condition CR1 holds for every pair of rules, then at most one of the rules is enabled at any invocation, and since assignments are always to constants, the program will reach a fixed point in one iteration. If condition CR2 holds, then every variable appears at most once in LHS of the enabled rules. Hence, one constant at most can be assigned to any particular variable, and the program must reach a fixed point after all the enabled rules have been fired. If two or more enabled rules can be fired that assign to the same variable, condition CR3 guarantees that they will assign the same value to the variable, and again the program must reach a fixed point after all the rules have been fired. Obviously, the number of iterations before reaching a fixed point is bounded by the number of rules in the program. (This assumes that the scheduler must not execute a rule more than once if no variable will be changed by executing the rule.) Tighter bounds are possible by taking into account rules whose tests are mutually exclusive.
To illustrate the application of the special form, consider the programs in examples 3–5 below. In example 3, even though the two tests in this program are not mutually exclusive because (b = c = true) is true in both test 1 and test 2, the fact that all LHS variables are distinct makes the rules compatible (condition CR2 is satisfied) and thus is sufficient to guarantee that this program will reach a fixed point in a finite number of iterations.
In example 4, test 1 and test 3 are not mutually exclusive. However, rule 1 and rule 3 are compatible by condition CR2. Rule 2 and rule 3 are compatible because their tests are mutually exclusive, and so are rule 1 and rule 2. Thus, this program will reach a fixed point in finite time.
Finally, consider example 5. Note that the same value (the constant true) is assigned to the variable a1 that appears in the LHS of both rules 1 and 2. Hence, condition CR3 is satisfied and thus the rules are compatible; hence, this program is guaranteed to reach a fixed point in finite time.