
Intermediate Probability Theory for Biomedical Engineers - JohnD. Enderle
.pdf
BIVARIATE RANDOM VARIABLES 73
Solution. (a) We find
P (Ac ) = P (x = y) = px,y (1, 1) + px,y (3, 3) = 14 ;
hence, P (A) = 1 − P (Ac ) = 3/4. Let Dx,y denote the support set for the PMF px,y . Then
|
|
px,y (α, β) |
, (α, β) Dx,y ∩ {α = β} |
||
px,y| A(α, β | A) = |
|
P (A) |
|||
|
0, |
|
|
otherwise. |
|
The result is shown in graphical form in Fig. 4.13. |
|
||||
(b) We have |
|
|
|
|
|
|
py|x (β | 1) = |
|
px,y (1, β) |
, |
|
|
|
px (1) |
and
3 px (1) = Px,y (1, β) = Px,y (1, −1) + Px,y (1, 1) = 8 .
β
Consequently,
|
|
1/3, |
|
β = 1 |
|
px|y (β | 1) = 2/3, |
|
β = −1 |
|||
|
|
0, |
|
otherwise. |
|
|
|
b |
|
|
|
|
|
1 |
|
|
|
|
|
6 |
|
|
|
|
2 |
|
|
|
|
1 |
1 |
|
|
1 |
|
6 |
|
|
6 |
|
|
|
|
|
|
||
1 6 |
|
|
|
|
|
-1 |
|
1 |
2 |
3 |
a |
|
-1 |
1 |
|
|
|
|
3 |
|
|
|
|
|
|
|
|
|
FIGURE 4.13: Conditional PMF for Example 4.5.1a.

74INTERMEDIATE PROBABILITY THEORY FOR BIOMEDICAL ENGINEERS
(c)The support set for px,y is {(−1, 0), (−1, 1)}, and we find easily that P (B) = 1/4. Then
|
|
|
|
|
|
|
0.5, (α, β) = (−1, 0) |
||||||||||||||||
|
px,y|B (α, β | B) = 0.5, (α, β) = (−1, 1) |
||||||||||||||||||||||
|
|
|
|
|
|
|
|
0, |
|
|
|
otherwise. |
|||||||||||
Thus |
|
|
|
|
|
|
|
|
|
|
|
|
|
|
|
|
|
|
|
|
|
|
|
|
|
|
px|B |
(α |
| B |
) |
= |
1, |
|
|
α = −1 |
||||||||||||
|
|
|
|
|
0, |
|
|
otherwise, |
|||||||||||||||
and |
|
|
|
|
|
|
|
|
|
|
|
|
|
|
|
|
|
|
|
|
|
|
|
|
|
|
|
|
|
|
|
0.5, |
|
β = 0 |
|||||||||||||
|
|
py|B (β | B) = 0.5, |
|
β = 1 |
|||||||||||||||||||
|
|
|
|
|
|
|
|
0, |
|
otherwise. |
|||||||||||||
|
|
|
|
|
|
|
|
|
|
|
|
|
|
|
|
|
|
|
|
|
|
|
|
We conclude that x and y are conditionally independent, given B. |
|||||||||||||||||||||||
Example 4.5.2. Random variables x and y have joint PDF |
|
|
|
|
|||||||||||||||||||
fx,y |
(α, β) |
= |
0.25α(1 + 3β2), 0 < α < 2, 0 < β < 1 |
||||||||||||||||||||
|
|
|
0, |
|
|
|
|
|
|
|
|
|
otherwise. |
||||||||||
Find (a) P (0 < x < 1|y = 0.5) and (b) fx,y| A(α, β | A), where event A = {x + y ≤ 1}. |
|||||||||||||||||||||||
Solution. (a) First we find |
|
|
|
|
|
|
|
|
|
|
|
|
|
|
|
|
|
|
|
|
|
|
|
|
|
|
|
|
|
|
2 |
|
α 7 |
|
|
7 |
|
|
|
|
|||||||
|
|
|
fy (0.5) = |
|
|
|
|
|
|
|
|
||||||||||||
|
|
|
|
|
|
|
|
d α = |
|
|
. |
|
|
|
|||||||||
|
|
|
|
|
4 |
4 |
8 |
||||||||||||||||
|
|
|
|
|
|
|
0 |
|
|
|
|
|
|
|
|
|
|
|
|
|
|
|
|
Then for 0 < α < 2, |
|
|
|
|
|
|
|
|
|
|
|
|
|
|
|
|
|
|
|
|
|
|
|
|
|
|
fx|y (α | 0.5) = |
0.25α7/4 |
= |
|
α |
||||||||||||||||
|
|
|
|
|
|
|
|
, |
|
|
|||||||||||||
|
|
|
7/8 |
|
|
2 |
|||||||||||||||||
and |
|
|
|
|
|
|
|
|
|
|
|
|
|
|
|
|
|
|
|
|
|
|
|
|
P (0 < x < 1|y = 0.5) = |
0 α |
1 |
||||||||||||||||||||
|
1 |
|
d α = |
|
. |
||||||||||||||||||
|
2 |
4 |
|||||||||||||||||||||
(b) First, we find |
|
|
|
|
|
|
|
|
|
|
|
|
|
|
|
|
|
|
|
|
|
|
|
|
|
P (A) = |
α+β≤1 |
|
|
fx,y (α, β)d αdβ; |
|||||||||||||||||
|
|
|
|
|
|
|
|
|
|
|
|
|
|
|
|
|
|
|
|
|

|
|
|
|
|
|
|
|
|
|
|
BIVARIATE RANDOM VARIABLES |
75 |
|||
substituting, |
|
|
|
|
|
|
|
|
|
|
|
|
|
|
|
|
|
1 |
1−β |
α |
|
|
13 |
|
|
||||||
|
|
|
|
|
|
|
|
|
|||||||
P (A) = |
|
|
|
|
|
(1 + 3β2) d |
αdβ = |
|
. |
|
|||||
|
|
|
4 |
240 |
|
||||||||||
|
0 |
|
0 |
|
|
|
|
|
|
|
|
|
|
|
|
The support region for the PDF fx,y |
is |
|
|
|
|
|
|
|
|
|
|
|
|
||
R = {(α, β) : 0 < α < 2, 0 < β < 1}. |
|
||||||||||||||
Let B = R ∩ {α + β ≤ 1}. For all (α, β) B, we have |
|
|
|
|
|
|
|||||||||
|
|
|
|
|
|
fx,y (α, β) |
|
60 |
|
|
|
||||
fx,y| A(α, β | A) = |
|
|
|
|
= |
|
|
α(1 + 3β2), |
|
||||||
|
|
P (A) |
|
13 |
|
||||||||||
and fx,y| A(α, β | A) = 0, otherwise. We note that |
|
|
|
|
|
|
|
|
|||||||
B = {(α, β) : 0 < α ≤ 1 − β < 1}. |
|
||||||||||||||
Example 4.5.3. Random variables x and y have joint PDF |
|
||||||||||||||
fx,y |
(α, β) |
= |
6α, 0 < α < 1 − β < 1 |
|
|||||||||||
|
|
0, |
otherwise. |
|
Determine whether or not x and y are conditionally independent, given A = {ζ S : x ≥ y}.
Solution. The support region for fx,y is
R = {(α, β) : 0 < α < 1 − β < 1}; the support region for fx,y| A is thus
B = {(α, β) : 0 < α < 1 − β < 1, α ≥ β} = {0 < β ≤ α < 1 − β < 1}.
The support regions are illustrated in Fig. 4.14.
1 |
b |
|
|
1 |
|
|
|
||
0.5 |
|
|
|
0.5 |
|
R |
|
|
|
0 |
0.5 |
1 |
a |
0 |
b |
|
|
B |
|
|
0.5 |
1 |
a |
FIGURE 4.14: Support regions for Example 4.5.3.

76 INTERMEDIATE PROBABILITY THEORY FOR BIOMEDICAL ENGINEERS
|
|
|
b |
|
|
|
|
|
|
|
||
3 |
|
1 |
1 |
|
|
1 |
|
|||||
|
|
|
|
|
|
|
|
|
|
|
|
|
|
8 |
8 |
|
|
8 |
|
||||||
|
|
|
|
|
||||||||
2 |
|
1 |
1 |
|
|
|
|
|||||
|
|
|
|
|
|
|
|
|
|
|
|
|
|
8 |
4 |
|
|
|
|
||||||
|
|
|
|
|
|
|||||||
1 |
|
|
|
|
|
1 |
|
|
1 |
|
||
|
|
|
|
|
|
|
|
|
|
|
|
|
|
|
|
|
|
8 |
|
|
8 |
|
|||
|
|
|
|
|
|
|
|
|
||||
0 |
|
|
|
|
|
|
|
|
|
|
|
|
|
|
|
|
|
|
|
|
|
|
|
|
|
|
|
|
|
|
|
|
|
|
|
|
a |
|
1 |
|
2 |
|
3 |
FIGURE 4.15: PMF for Drill Problem 4.5.1.
For (α, β) B, |
|
|
|
|
|
|
|
|
|
|
|
|
|
|
|
|
|
|
|
|
|
|
|
fx,y| A(α, β | A) = |
|
fx,y (α, β) |
= |
|
6α |
||||||||||||||||||
|
|
|
|
|
|
|
. |
|
|
||||||||||||||
|
|
P (A) |
|
|
P (A) |
||||||||||||||||||
The conditional marginal densities are found by integrating |
|
fx,y| A: |
|||||||||||||||||||||
For 0 < β < 0.5, |
|
|
|
|
|
|
|
|
|
|
|
|
|
|
|
|
|
|
|
|
|
|
|
|
|
|
|
1 |
|
1−β |
|
|
|
|
|
|
|
3(1 − 2β) |
|
|
|||||||
fy| A( |
β |
| A) = |
|
|
|
6 |
α |
d |
α |
= |
|
. |
|||||||||||
P (A) |
|
|
|
|
|
||||||||||||||||||
|
|
|
|
|
|
|
|
P (A) |
|||||||||||||||
|
|
|
|
|
|
|
β |
|
|
|
|
|
|
|
|
|
|
|
|
|
|
|
|
For 0 < α < 0.5, |
|
|
|
|
|
|
|
|
|
|
|
|
|
|
|
|
|
|
|
|
|
|
|
|
|
|
|
1 |
|
|
α |
|
|
|
|
|
|
|
|
6α2 |
|||||||
|
|
|
|
|
|
|
|
|
|
|
|
|
|
|
|
||||||||
fx| A(α | A) = |
|
|
|
6α dβ = |
|
|
. |
|
|||||||||||||||
P (A) |
0 |
P (A) |
|||||||||||||||||||||
|
|
|
|
|
|
|
|
|
|
|
|
|
|
|
|
|
|
|
|
|
|
|
|
For 0.5 < α < 1, |
|
|
|
|
|
|
|
|
|
|
|
|
|
|
|
|
|
|
|
|
|
|
|
|
|
|
|
1 |
1−α |
|
|
|
|
|
|
6α(1 − α) |
|
||||||||||
|
α |
|
|
|
|
|
|
α |
|
α |
|
|
|
. |
|||||||||
fx| A( |
| A) = |
P (A) |
|
|
6 |
d |
= |
|
|||||||||||||||
|
0 |
|
|
|
|
|
|
P (A) |
|||||||||||||||
|
|
|
|
|
|
|
|
|
|
|
|
|
|
|
|
|
|
|
|
|
|
|
We conclude that since P (A) is a constant, the RVs x and y are not conditionally independent, given A.
Drill Problem 4.5.1. Random variables x and y have joint PMF shown in Fig. 4.15. Find (a) px (1), (b) py (2), (c ) px|y (1 | 2), (d ) py|x (3 | 1).
Answers: 1/2, 1/4, 3/8, 1/3.
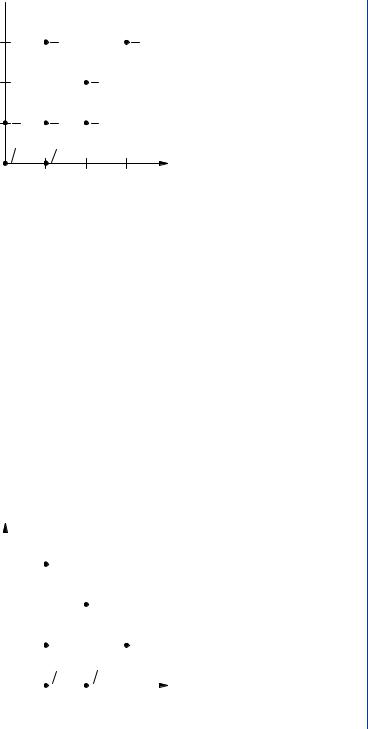
BIVARIATE RANDOM VARIABLES 77
b
3 |
1 |
|
|
1 |
8 |
|
|
8 |
|
|
|
|
||
2 |
|
|
1 |
|
|
|
8 |
|
|
|
|
|
|
|
1 |
1 |
|
1 |
|
1 |
8 |
|
8 |
|
8 |
|
|
||
1 8 |
1 8 |
|
|
a |
0 |
1 |
2 |
3 |
|
|
|
FIGURE 4.16: PMF for Drill Problem 4.5.2.
Drill Problem 4.5.2. Random variables x and y have joint PMF shown in Fig. 4.16. Event A = {ζ S : x + y ≤ 1}. Find (a) P (A), (b) px,y| A(1, 1 | A), (c ) px| A (1 | A), and (d) py| A(1 | A).
Answers: 0, 3/8, 1/3, 1/3.
Drill Problem 4.5.3. Random variables x and y have joint PMF shown in Fig. 4.17. Determine if random variables x and y are: (a) independent, (b) independent, given {y ≤ 1}.
Answers: No, No.
Drill Problem 4.5.4. The joint PDF for the RVs x and y is
|
2 |
α2β, 0 < α < 3, 0 < β < 1 |
||||||||||||||||||
fx,y (α, β) = 9 |
||||||||||||||||||||
0, |
|
|
|
|
|
|
otherwise. |
|||||||||||||
|
|
|
|
|
|
|
|
|
|
|
||||||||||
3 |
|
|
b |
1 |
|
|
|
|
|
|
|
|
|
|
|
|||||
|
|
|
|
|
|
|
|
|
|
|
|
|||||||||
|
|
|
|
|
|
|
|
|
|
|
|
|
|
|
||||||
|
|
|
|
|
|
|
|
|
|
|
|
|
|
|
|
|
|
|
||
|
|
|
|
8 |
|
|
|
|
|
|
|
|
|
|
|
|||||
|
|
|
|
|
|
|
|
|
|
|
|
|
|
|
|
|||||
2 |
|
|
|
|
|
|
|
|
|
1 |
|
|
|
|
|
|
||||
|
|
|
|
|
|
|
|
|
|
|
|
|
|
|
|
|
|
|
||
|
|
|
|
|
|
|
|
|
4 |
|
|
|
|
|
|
|||||
|
|
|
|
|
|
|
|
|
|
|
|
|
|
|
|
|||||
1 |
|
|
|
|
1 |
|
|
|
|
|
|
|
|
1 |
|
|
||||
|
|
|
|
|
|
|
|
|
|
|
|
|
|
|
|
|
|
|
||
|
|
|
|
8 |
|
|
|
|
|
|
|
|
8 |
|
|
|||||
|
|
|
|
|
|
|
|
|
|
|
|
|
|
|
||||||
|
|
|
|
|
|
|
1 4 |
|
|
1 8 |
|
|
|
|
|
|||||
|
|
|
|
|
|
|
|
|
|
|
|
|||||||||
0 |
|
|
|
|
|
|
|
|
|
|
|
|
|
|
|
|
|
a |
|
|
|
|
|
1 |
|
|
2 |
|
|
3 |
|
||||||||||
|
|
|
|
|
|
|
|
|
|
|
|
FIGURE 4.17: PMF for Drill Problem 4.5.3.

78 INTERMEDIATE PROBABILITY THEORY FOR BIOMEDICAL ENGINEERS
Find: (a) fx|y (1 | 0.5), (b) fy|x (0.5 | 1), (c )P (x ≤ 1, y ≤ 0.5 | x + y ≤ 1), and (d) P (x ≤ 1 | x + y ≤ 1).
Answers: 1/9, 1, 1, 13/16. |
|
|
|
|
|
|
|
|||
Drill Problem 4.5.5. |
The joint PDF for the RVs x and y is |
|||||||||
|
|
|
|
fx,y (α, β) = e −α−β u(α)u(β). |
||||||
Find: (a) fx|y (1 | 1), (b)Fx|y (1 | 1), (c )P (x ≥ 5 | x ≥ 1), and (d) P (x ≤ 0.5 | x + y ≤ 1). |
||||||||||
Answers: 1 |
− |
e −1, e −1 |
, e −4, |
1 − e −0.5 − 0.5e −1 |
. |
|
|
|
||
|
|
|
|
|
|
|||||
|
|
|
1 − 2e −1 |
|
|
|
||||
Drill Problem 4.5.6. |
The joint PDF for the RVs x and y is |
|||||||||
|
|
|
|
|
2β |
, 0 < β < √ |
|
< 1 |
||
|
|
|
|
|
α |
|||||
|
|
|
|
|
||||||
|
|
|
fx,y (α, β) = α |
otherwise. |
||||||
|
|
|
|
0, |
|
|||||
Find: (a) |
P (y ≤ 0.25 | x = 0.25), (b)P (y = 0.25 | x = 0.25), (c )P (x ≤ 0.25 | x ≤ 0.5), and |
|||||||||
(d) P (x ≤ 0.25 | x + y ≤ 1). |
|
|
|
|
|
|
|
Answers: 0, 1/2, 1/4, 0.46695.
Drill Problem 4.5.7. The joint PDF for the RVs x and y is
fx,y (α, β) = |
4αβ, |
0 < α < 1, 0 < β < 1 |
0, |
otherwise. |
Determine whether or not x and y are (a) independent, (b) independent, given A = {x + y ≥ 1}.
Answers: No, Yes.
4.6CONDITIONAL EXPECTATION
Conditional expectation is completely analogous to ordinary expectation, with the unconditional CDF replaced with the conditional version. In particular, the conditional expectation of g (x, y), given event A, is defined as
∞ ∞
E(g (x, y) | A) = |
g (α, β) d Fx,y|A(α, β | A) . |
(4.77) |
|
−∞ −∞ |
|
When the conditioning event A has zero probability, as when A = {x = 0} for continuous RVs, the conditional CDF, PMF, and PDF definitions of the previous sections are used.

BIVARIATE RANDOM VARIABLES 79
Definition 4.6.1. The conditional expectation of g (x, y), given y = β, is defined by
∞
E(g (x, y) | y = β) = g (α, β) d Fx|y (α | β). |
(4.78) |
|
−∞ |
|
|
In particular, the conditional mean of x, given y = β, is |
|
|
∞ |
∞ |
|
E(x | y = β) = α d Fx|y (α | β) = |
α fx|y (α | β) d α . |
(4.79) |
−∞ |
−∞ |
|
It is important to note that if the given value of y(β) is a constant, then E(x | y = β) is also a constant. In general, E(x | y = β) is a function of β. Once this function is obtained, one may substitute β = y(ζ ) and treat the result as a random variable; we denote this result as simply E(x | y). It is also important to note that conditional expectation, as ordinary expectation, is a linear operation.
Definition 4.6.2. The conditional mean of x, given y = β, is defined by |
|
||||||||
|
ηx|y=β = E(x|y = β), |
(4.80) |
|||||||
note that the RV ηx|y = E(x | y). The conditional variance of x, given y = β, is defined by |
|
||||||||
σx2|y=β = E((x − ηx|y )2|y = β). |
(4.81) |
||||||||
The RV σx2|y = E((x − ηx|y )2|y). |
|
|
|
|
|
|
|
|
|
Example 4.6.1. Random variable y has PMF |
|
|
|
|
|||||
|
|
|
0.25, |
α = 1 |
|
||||
py |
(α) |
= |
0.5, |
α = 2 |
|
||||
|
0 |
. |
25 |
, |
α |
= 3 |
|
||
|
|
|
|
|
|
|
|||
|
|
|
|
0, |
|
otherwise. |
|
||
Find the variance of y, given event A = {y odd}. |
|
|
|
||||||
Solution. We easily find P (A) = 0.5 so that |
|
|
|
|
|||||
|
|
|
|
|
0.5, |
α = 1 |
|
||
py| A(α | A) = 0.5, |
α = 3 |
|
|||||||
|
|
|
|
|
0, |
otherwise. |
|

80 INTERMEDIATE PROBABILITY THEORY FOR BIOMEDICAL ENGINEERS
Then
|
|
|
ηy| A = E(y| A) = |
|
|
α2 py|a (α| A) = 2 |
|
|
|
||||||||||||||||||||||
|
|
|
|
|
|
|
|
|
|
|
|
|
|
|
|
|
α |
|
|
|
|
|
|
|
|
|
|
|
|
||
and |
|
|
|
|
|
|
|
|
|
|
|
|
|
|
|
|
|
|
|
|
|
|
|
|
|
|
|
|
|
|
|
|
|
|
|
|
|
E(y2| A) = |
α |
α2 py| A(α| A) = 5. |
|
|
|
|
|
||||||||||||||||||
|
|
|
|
|
|
|
|
|
|
|
|
|
|
|
|
|
|
|
|
|
|
|
|
|
|
|
|
|
|
|
|
Finally, |
|
|
|
|
|
|
|
|
|
|
|
|
|
|
|
|
|
|
|
|
|
|
|
|
|
|
|
|
|
|
|
|
|
σy2| A = E(y2| A) − E2(y| A) = 5 − 4 = 1. |
|
|
|||||||||||||||||||||||||||
Example 4.6.2. Random variables x and y have joint PDF |
|
|
|
|
|
|
|
|
|||||||||||||||||||||||
|
|
fx,y |
(α, β) |
|
= |
2, α > 0, 0 < β < 1 − α |
|
|
|
||||||||||||||||||||||
|
|
|
|
|
|
|
|
0, |
|
|
|
|
otherwise. |
|
|
|
|
|
|
||||||||||||
Find ηx|y = E(x|y), E(ηx|y ), and E(x). |
|
|
|
|
|
|
|
|
|
|
|
|
|
|
|
|
|
||||||||||||||
Solution. We first find the marginal PDF |
|
|
|
|
|
|
|
|
|
|
|
|
|
|
|
|
|
||||||||||||||
|
|
|
|
|
|
|
∞ |
|
|
|
|
|
|
|
|
1−β |
|
|
|
|
|
|
|
|
|||||||
|
fy (β) = |
|
|
|
fx,y (α, β) d α = |
|
|
|
2 d α = 2(1 − β), |
|
|||||||||||||||||||||
|
|
|
|
|
|
−∞ |
|
|
|
|
|
|
|
0 |
|
|
|
|
|
|
|
|
|
|
|
||||||
for 0 < β < 1. Then for 0 < β < 1, |
|
|
|
|
|
|
|
|
|
|
|
|
|
|
|
|
|
|
|
||||||||||||
|
|
|
|
|
|
|
|
|
fx,y (α, β) |
|
1 |
|
|
|
, 0 < α < 1 − β |
|
|||||||||||||||
fx|y ( |
|
|
) |
|
|
|
|
|
|
|
|
|
|
|
|
||||||||||||||||
α |
β |
= |
|
= |
|
|
1 − |
|
β |
|
|||||||||||||||||||||
|
|
fy (β) |
|
|
|
|
|||||||||||||||||||||||||
| |
|
|
|
|
|
|
|
|
|
|
otherwise. |
|
|||||||||||||||||||
|
|
|
|
|
|
|
|
|
|
|
|
|
|
|
|
0, |
|
|
|
|
|||||||||||
Hence, for 0 < β < 1, |
|
|
|
|
|
|
|
|
|
|
|
|
|
|
|
|
|
|
|
|
|
|
|
|
|
|
|
|
|
|
|
|
|
|
|
|
|
|
|
|
|
|
|
|
|
|
1−β |
|
|
|
|
|
1 − β |
|
|
|
|
||||||
|
|
|
E(x | y = β) = |
|
|
|
α |
|
d α = |
. |
|
|
|
||||||||||||||||||
|
|
|
|
|
|
β |
|
|
|
|
|||||||||||||||||||||
|
|
|
|
|
|
|
|
|
|
|
|
|
|
|
0 |
|
1 − |
|
|
|
|
|
|
2 |
|
|
|
|
|
||
We conclude that |
|
|
|
|
|
|
|
|
|
|
|
|
|
|
|
|
|
|
|
|
|
|
|
|
|
|
|
|
|
|
|
|
|
|
|
|
|
|
|
|
η |
x|y |
= E(x | y) = |
|
|
1 − y |
. |
|
|
|
|
|
|
|
|||||||||
|
|
|
|
|
|
|
|
|
2 |
|
|
|
|
|
|
|
|||||||||||||||
|
|
|
|
|
|
|
|
|
|
|
|
|
|
|
|
|
|
|
|||||||||||||
Now, |
|
|
|
|
|
|
|
|
|
|
|
|
|
|
|
|
|
|
|
|
|
|
|
|
|
|
|
|
|
|
|
η |
x|y ) |
= E |
|
|
1 − y |
|
= |
|
1 |
1 − β |
|
2(1 − |
β |
)d |
β |
= |
1 |
= E(x) |
. |
||||||||||||
|
|
|
|
|
3 |
||||||||||||||||||||||||||
E( |
|
|
|
|
2 |
|
|
|
|
0 |
2 |
|
|
|
|
|
|
|
|
|
|
|
|
|
|
|
|
|
|
|
|
|
|
|
|
|
|
|
|
|
BIVARIATE RANDOM VARIABLES |
81 |
|||||||||||||
Example 4.6.3. |
Find the conditional variance of y, given A = {x ≤ 0.75}, where |
|
|||||||||||||||||||||||||||||||||||||
|
|||||||||||||||||||||||||||||||||||||||
|
|
|
|
fx,y (α, β) = 1.5(α2 + β2)(u(α) − u(α − 1))(u(β) − u(β − 1)). |
|
||||||||||||||||||||||||||||||||||
Solution. First, we find |
|
|
|
|
|
|
|
|
|
|
|
|
|
|
|
|
|
|
|
|
|
|
|
|
|
|
|
|
|
|
|
|
|||||||
|
|
|
|
|
|
|
|
|
|
1 |
0.75 |
|
|
|
|
|
|
|
|
|
|
|
|
75 |
|
|
|
|
|
|
|||||||||
|
|
|
|
|
|
|
|
|
|
|
|
|
|
|
|
|
|
|
|
|
|
|
|
|
|
|
|
|
|
|
|||||||||
|
|
|
|
|
|
|
|
P (A) = |
|
1.5(α2 |
+ |
β2) d αdβ = |
|
|
|
|
|
, |
|
|
|
||||||||||||||||||
|
|
|
|
|
|
|
|
|
128 |
|
|||||||||||||||||||||||||||||
|
|
|
|
|
|
|
|
|
|
0 |
0 |
|
|
|
|
|
|
|
|
|
|
|
|
|
|
|
|
|
|
|
|
|
|
|
|
|
|
|
|
so that |
|
|
|
|
|
|
|
|
|
|
|
|
|
|
|
|
|
|
|
|
|
|
|
|
|
|
|
|
|
|
|
|
|
|
|
|
|
|
|
|
|
|
(α, β |
|
A) |
|
|
fx,y (α, β) |
|
|
64 |
(α2 + β2), 0 < α < 0.75, 0 < β < 1 |
|
||||||||||||||||||||||||||
f |
, |
y| A |
| |
= |
|
= |
|
|
|
|
|
||||||||||||||||||||||||||||
|
P (A) |
|
|
|
|||||||||||||||||||||||||||||||||||
|
x |
|
|
|
|
|
|
25 |
|
|
0, |
|
|
|
|
|
|
|
|
|
|
|
|
otherwise. |
|
||||||||||||||
|
|
|
|
|
|
|
|
|
|
|
|
|
|
|
|
|
|
|
|
|
|
|
|
|
|
|
|
|
|
|
|
||||||||
Then for 0 < β < 1, |
|
|
|
|
|
|
|
|
|
|
|
|
|
|
|
|
|
|
|
|
|
|
|
|
|
|
|
|
|
|
|
|
|
||||||
|
|
|
|
|
|
fy| A(β | A) = |
|
0.75 64 |
|
α2 |
|
|
|
β2 d |
9 |
|
|
|
48 |
β2. |
|
||||||||||||||||||
|
|
|
|
|
|
|
|
|
|
+ |
|
α = |
|
|
|
+ |
|
|
|
|
|||||||||||||||||||
|
|
|
|
|
|
0 |
|
25 |
25 |
25 |
|
||||||||||||||||||||||||||||
Consequently, |
|
|
|
|
|
|
|
|
|
|
|
|
|
|
|
|
|
|
|
|
|
|
|
|
|
|
|
|
|
|
|
|
|
|
|
|
|||
|
|
|
|
|
|
|
|
|
|
|
1 |
|
9 |
|
|
48 |
|
|
|
|
|
|
|
66 |
|
|
|
|
|
|
|||||||||
|
|
|
|
|
|
|
|
|
|
|
|
|
|
|
|
|
|
|
|
|
|
|
|
|
|
|
|
||||||||||||
|
|
|
|
|
|
|
E(y | A) = |
β |
|
|
|
+ |
|
|
β2 |
d α = |
|
|
|
, |
|
||||||||||||||||||
|
|
|
|
|
|
|
|
25 |
25 |
100 |
|
||||||||||||||||||||||||||||
|
|
|
|
|
|
|
|
|
|
|
0 |
|
|
|
|
|
|
|
|
|
|
|
|
|
|
|
|
|
|
|
|
|
|
|
|
|
|
|
|
and |
|
|
|
|
|
|
|
|
|
|
|
|
|
|
|
|
|
|
|
|
|
|
|
|
|
|
|
|
|
|
|
|
|
|
|
|
|
|
|
|
|
|
|
|
|
|
|
|
|
|
1 |
|
9 |
|
|
|
48 |
|
|
|
|
|
|
378 |
|
|
|
||||||||||||
|
|
|
|
|
|
|
|
|
|
|
|
|
|
|
|
|
|
|
|
|
|
|
|
|
|||||||||||||||
|
|
|
|
|
|
|
E(y2 | A) = |
β2 |
|
|
|
+ |
|
|
β2 |
d α = |
|
|
|
. |
|
|
|||||||||||||||||
|
|
|
|
|
|
|
25 |
|
25 |
750 |
|
|
|||||||||||||||||||||||||||
|
|
|
|
|
|
|
|
|
|
|
0 |
|
|
|
|
|
|
|
|
|
|
|
|
|
|
|
|
|
|
|
|
|
|
|
|
|
|
|
|
Finally, |
|
|
|
|
|
|
|
|
|
|
|
|
|
|
|
|
|
|
|
|
|
|
|
|
|
|
|
|
|
|
|
|
|
|
|
|
|
|
|
|
|
|
|
|
|
|
|
|
σy2| A = E(y2 | A) − E2 |
|
|
|
|
513 |
|
|
|
|
|
|
|
|
|
||||||||||||||||
|
|
|
|
|
|
|
|
|
(y | A) = |
|
. |
|
|||||||||||||||||||||||||||
|
|
|
|
|
|
|
|
|
7500 |
||||||||||||||||||||||||||||||
There are many applications of conditional expectation. One important use is to simplify |
|||||||||||||||||||||||||||||||||||||||
calculations involving expectation, as by applying the following theorem. |
|
||||||||||||||||||||||||||||||||||||||
Theorem 4.6.1. Let x and y be jointly distributed RVs. Then |
|
|
|
|
|
|
|
|
|
||||||||||||||||||||||||||||||
|
|
|
|
|
|
|
|
|
E(g (x, y)) = E(E(g (x, y) | y)) |
(4.82) |
|||||||||||||||||||||||||||||
Proof. Note that |
|
|
|
|
|
|
|
|
|
|
|
|
|
|
|
|
|
|
|
|
|
|
|
|
|
|
|
|
|
|
|
|
|
|
|
||||
|
|
|
|
|
|
|
|
|
d Fx,y (α, β) = d Fx|y (α | β) dFy (β). |
|
|
|
|
|
|
|
|
|
|||||||||||||||||||||
|
|
|
|
|
|
|
|
|
|
|
|
|
|
|
|
|

82 INTERMEDIATE PROBABILITY THEORY FOR BIOMEDICAL ENGINEERS
Special cases of this are
fx,y (α, β) = fx|y (α | β) fy (β)
and
px,y (α, β) = px|y (α | β) py (β).
We thus have
∞ |
∞ |
|
E(g (x, y)) = |
g (α, β) d Fx|y (α | β) dFy (β). |
|
−∞ |
−∞ |
|
Hence |
|
|
|
∞ |
|
E(g (x, y)) = E(g (x, y) | y = β) dFy (β), |
|
|
|
−∞ |
|
from which the desired result follows. |
|
|
The conditional mean estimate is one of the most important applications of conditional |
||
expectation. |
|
|
Theorem 4.6.2. Let x and y be jointly distributed RVs with σx2 < ∞. The function g |
which |
|
minimizes E((x − g (y))2) is |
|
|
|
g (y) = E(x | y). |
(4.83) |
Proof. We have |
|
|
E((x − g (y))2 | y) = E((x − ηx|y + ηx|y − g (y))2 | y)
=σx2|y + 2E((x − ηx|y )(ηx|y − g (y))|y) + (ηx|y − g (y))2
=σx2|y + (ηx|y − g (y))2.
The choice g (y) = ηx|y is thus seen to minimize the above expression, applying the (unconditional) expectation operator yields the desired result.
The above result is extremely important: the best minimum mean square estimate of a quantity is the conditional mean of the quantity, given the data to be used in the estimate. In many cases, the conditional mean is very difficult or even impossible to compute. In the important Gaussian case (discussed in a later chapter) the conditional mean turns out to be easy to find. In fact, in the Gaussian case, the conditional mean is always a linear function of the given data.