
Wooldridge_-_Introductory_Econometrics_2nd_Ed
.pdf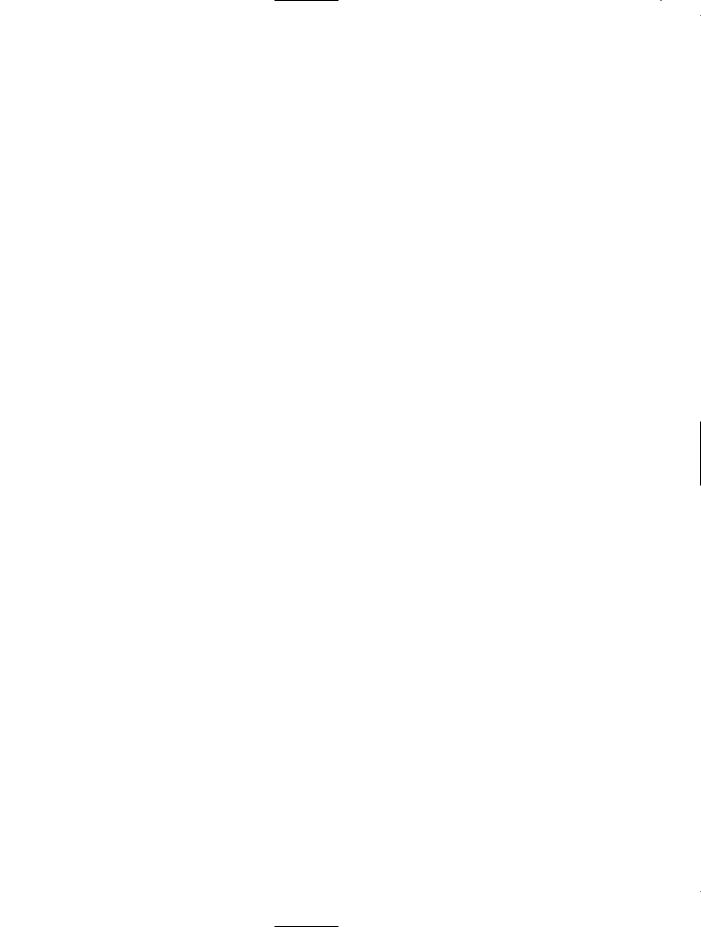
Chapter 2 |
The Simple Regression Model |
(i)What kinds of factors are contained in u? Are these likely to be correlated with level of education?
(ii)Will a simple regression analysis uncover the ceteris paribus effect of education on fertility? Explain.
2.2In the simple linear regression model y 0 1x u, suppose that E(u) 0. Letting 0 E(u), show that the model can always be rewritten with the same slope, but a new intercept and error, where the new error has a zero expected value.
2.3The following table contains the ACT scores and the GPA (grade point average) for 8 college students. Grade point average is based on a four-point scale and has been rounded to one digit after the decimal.
Student |
GPA |
ACT |
|
|
|
1 |
2.8 |
21 |
|
|
|
2 |
3.4 |
24 |
|
|
|
3 |
3.0 |
26 |
|
|
|
4 |
3.5 |
27 |
|
|
|
5 |
3.6 |
29 |
|
|
|
6 |
3.0 |
25 |
|
|
|
7 |
2.7 |
25 |
|
|
|
8 |
3.7 |
30 |
|
|
|
(i)Estimate the relationship between GPA and ACT using OLS; that is, obtain the intercept and slope estimates in the equation
GPˆA ˆ0 ˆ1ACT.
Comment on the direction of the relationship. Does the intercept have a useful interpretation here? Explain. How much higher is the GPA predicted to be, if the ACT score is increased by 5 points?
(ii)Compute the fitted values and residuals for each observation and verify that the residuals (approximately) sum to zero.
(iii)What is the predicted value of GPA when ACT 20?
(iv)How much of the variation in GPA for these 8 students is explained by ACT? Explain.
2.4The data set BWGHT.RAW contains data on births to women in the United States. Two variables of interest are the dependent variable, infant birth weight in ounces (bwght), and an explanatory variable, average number of cigarettes the mother smoked
61

Part 1 |
Regression Analysis with Cross-Sectional Data |
per day during pregnancy (cigs). The following simple regression was estimated using data on n 1388 births:
bwgˆht 119.77 0.514 cigs
(i)What is the predicted birth weight when cigs 0? What about when cigs 20 (one pack per day)? Comment on the difference.
(ii)Does this simple regression necessarily capture a causal relationship between the child’s birth weight and the mother’s smoking habits? Explain.
2.5In the linear consumption function
coˆns ˆ0 ˆ1inc,
the (estimated) marginal propensity to consume (MPC) out of income is simply the slope, ˆ1, while the average propensity to consume (APC) is coˆns/inc ˆ0 /inc ˆ1. Using observations for 100 families on annual income and consumption (both measured in dollars), the following equation is obtained:
coˆns 124.84 0.853 inc
n 100, R2 0.692
(i)Interpret the intercept in this equation and comment on its sign and magnitude.
(ii)What is predicted consumption when family income is $30,000?
(iii)With inc on the x-axis, draw a graph of the estimated MPC and APC.
2.6 Using data from 1988 for houses sold in Andover, MA, from Kiel and McClain (1995), the following equation relates housing price (price) to the distance from a recently built garbage incinerator (dist):
log(prˆice) 9.40 0.312 log(dist)
n 135, R2 0.162
(i)Interpret the coefficient on log(dist). Is the sign of this estimate what you expect it to be?
(ii)Do you think simple regression provides an unbiased estimator of the ceteris paribus elasticity of price with respect to dist? (Think about the city’s decision on where to put the incinerator.)
(iii)What other factors about a house affect its price? Might these be correlated with distance from the incinerator?
2.7Consider the savings function
—
sav 0 1inc u, u inc e,
where e is a random variable with E(e) 0 and Var(e) 2e. Assume that e is independent of inc.
(i)Show that E(u inc) 0, so that the key zero conditional mean assump-
tion (Assumption SLR.3) is satisfied. [Hint: If e is independent of inc, then E(e inc) E(e).]
62
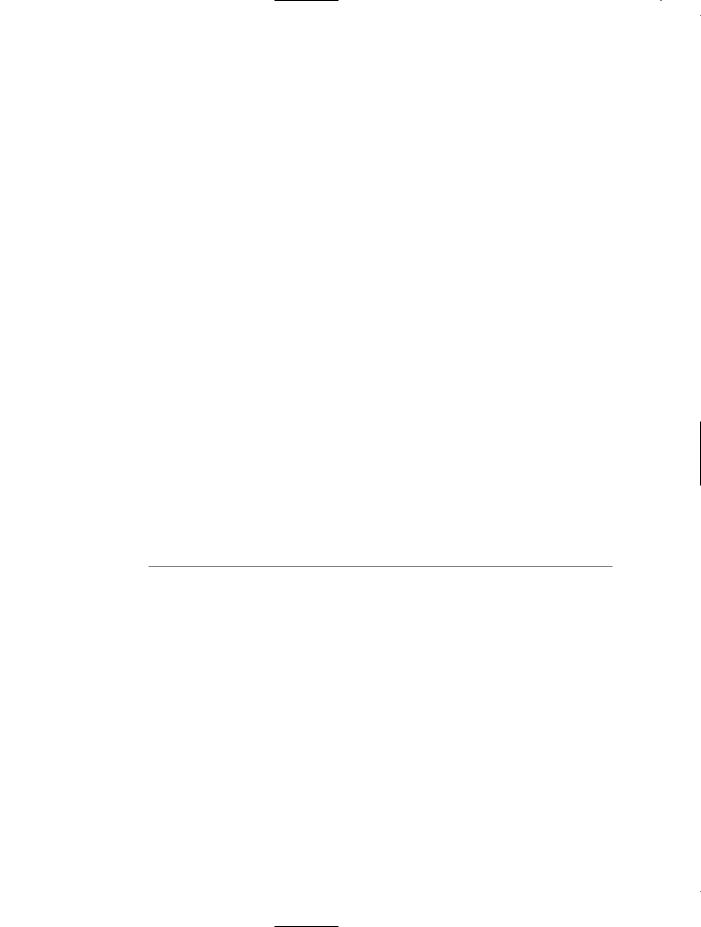
Chapter 2 |
The Simple Regression Model |
(ii)Show that Var(u inc) 2einc, so that the homoskedasticity Assumption SLR.5 is violated. In particular, the variance of sav increases with inc. [Hint: Var(e inc) Var(e), if e and inc are independent.]
(iii)Provide a discussion that supports the assumption that the variance of savings increases with family income.
2.8Consider the standard simple regression model y 0 1x u under Assumptions SLR.1 through SLR.4. Thus, the usual OLS estimators ˆ0 and ˆ1 are unbiased for their respective population parameters. Let ˜1 be the estimator of 1 obtained by assuming the intercept is zero (see Section 2.6).
(i)Find E( ˜1) in terms of the xi, 0, and 1. Verify that ˜1 is unbiased for
1 when the population intercept ( 0) is zero. Are there other cases where ˜1 is unbiased?
(ii)Find the variance of ˜1. (Hint: The variance does not depend on 0.)
|
|
n |
n |
˜ |
ˆ |
2 |
|
(iii) Show that Var( 1) Var( 1). [Hint: For any sample of data, xi |
|||
|
|
i 1 |
i 1 |
(xi x¯)2, with strict inequality unless x¯ 0.]
(iv)Comment on the tradeoff between bias and variance when choosing between ˆ1 and ˜1.
2.9(i) Let ˆ0 and ˆ1 be the intercept and slope from the regression of yi on xi, using n observations. Let c1 and c2, with c2 0, be constants. Let ˜0 and ˜1 be the intercept and
slope from the regression c1yi on c2xi. Show that ˜1 (c1/c2) ˆ1 and ˜0 c1 ˆ0, thereby verifying the claims on units of measurement in Section 2.4. [Hint: To obtain ˜1, plug
the scaled versions of x and y into (2.19). Then, use (2.17) for ˜0, being sure to plug in the scaled x and y and the correct slope.]
(ii)Now let ˜0 and ˜1 be from the regression (c1 yi) on (c2 xi) (with no restriction on c1 or c2). Show that ˜1 ˆ1 and ˜0 ˆ0 c1 c2 ˆ1.
COMPUTER EXERCISES
2.10 The data in 401K.RAW are a subset of data analyzed by Papke (1995) to study the relationship between participation in a 401(k) pension plan and the generosity of the plan. The variable prate is the percentage of eligible workers with an active account; this is the variable we would like to explain. The measure of generosity is the plan match rate, mrate. This variable gives the average amount the firm contributes to each worker’s plan for each $1 contribution by the worker. For example, if mrate 0.50, then a $1 contribution by the worker is matched by a 50¢ contribution by the firm.
(i)Find the average participation rate and the average match rate in the sample of plans.
(ii)Now estimate the simple regression equation
praˆte ˆ0 ˆ1mrate,
and report the results along with the sample size and R-squared.
(iii)Interpret the intercept in your equation. Interpret the coefficient on mrate.
(iv)Find the predicted prate when mrate 3.5. Is this a reasonable prediction? Explain what is happening here.
63
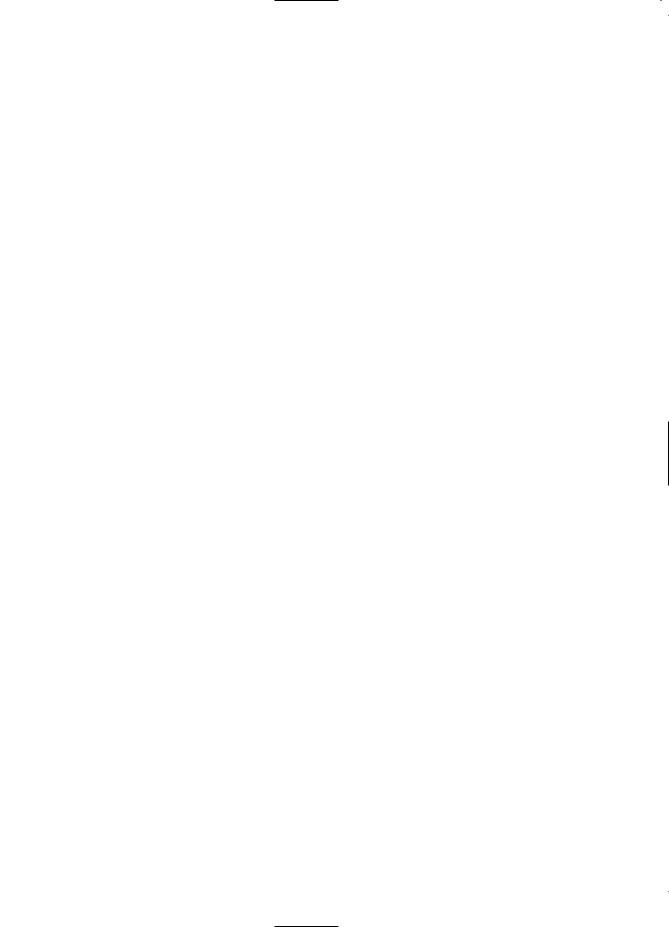
Part 1 |
Regression Analysis with Cross-Sectional Data |
(v)How much of the variation in prate is explained by mrate? Is this a lot in your opinion?
2.11The data set in CEOSAL2.RAW contains information on chief executive officers for U.S. corporations. The variable salary is annual compensation, in thousands of dollars, and ceoten is prior number of years as company CEO.
(i)Find the average salary and the average tenure in the sample.
(ii)How many CEOs are in their first year as CEO (that is, ceoten 0)? What is the longest tenure as a CEO?
(iii)Estimate the simple regression model
log(salary) 0 1ceoten u,
and report your results in the usual form. What is the (approximate) predicted percentage increase in salary given one more year as a CEO?
2.12 Use the data in SLEEP75.RAW from Biddle and Hamermesh (1990) to study whether there is a tradeoff between the time spent sleeping per week and the time spent in paid work. We could use either variable as the dependent variable. For concreteness, estimate the model
sleep 0 1totwrk u,
where sleep is minutes spent sleeping at night per week and totwrk is total minutes worked during the week.
(i)Report your results in equation form along with the number of observations and R2. What does the intercept in this equation mean?
(ii)If totwrk increases by 2 hours, by how much is sleep estimated to fall? Do you find this to be a large effect?
2.13Use the data in WAGE2.RAW to estimate a simple regression explaining monthly salary (wage) in terms of IQ score (IQ).
(i)Find the average salary and average IQ in the sample. What is the standard deviation of IQ? (IQ scores are standardized so that the average in the population is 100 with a standard deviation equal to 15.)
(ii)Estimate a simple regression model where a one-point increase in IQ changes wage by a constant dollar amount. Use this model to find the predicted increase in wage for an increase in IQ of 15 points. Does IQ explain most of the variation in wage?
(iii)Now estimate a model where each one-point increase in IQ has the same percentage effect on wage. If IQ increases by 15 points, what is the approximate percentage increase in predicted wage?
2.14For the population of firms in the chemical industry, let rd denote annual expenditures on research and development, and let sales denote annual sales (both are in millions of dollars).
(i)Write down a model (not an estimated equation) that implies a constant elasticity between rd and sales. Which parameter is the elasticity?
(ii)Now estimate the model using the data in RDCHEM.RAW. Write out the estimated equation in the usual form. What is the estimated elasticity of rd with respect to sales? Explain in words what this elasticity means.
64
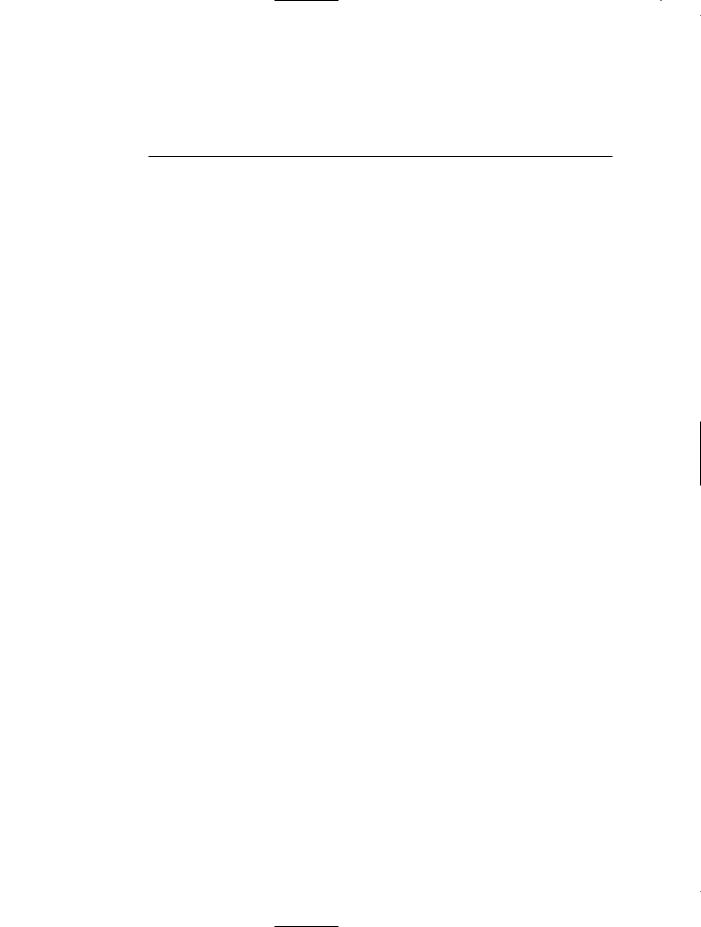
Chapter 2 The Simple Regression Model
A P P E N D I X |
2 A |
Minimizing the Sum of Squared Residuals
We show that the OLS estimates ˆ0 and ˆ1 do minimize the sum of squared residuals, as asserted in Section 2.2. Formally, the problem is to characterize the solutions ˆ0 andˆ1 to the minimization problem
n
min (yi b0 b1xi)2,
b0,b1 i 1
where b0 and b1 are the dummy arguments for the optimization problem; for simplicity, call this function Q(b0,b1). By a fundamental result from multivariable calculus (see Appendix A), a necessary condition for ˆ0 and ˆ1 to solve the minimization problem is that the partial derivatives of Q(b0,b1) with respect to b0 and b1 must be zero when eval-
uated at ˆ0, ˆ1: Q( ˆ0, ˆ1)/ b0 0 and Q( ˆ0, ˆ1)/ b1 0. Using the chain rule from calculus, these two equations become
n
2 (yi ˆ0 ˆ1xi) 0.
i 1
n
2 xi(yi ˆ0 ˆ1xi) 0.
i 1
These two equations are just (2.14) and (2.15) multiplied by 2n and, therefore, are solved by the same ˆ0 and ˆ1.
How do we know that we have actually minimized the sum of squared residuals? The first order conditions are necessary but not sufficient conditions. One way to verify that we have minimized the sum of squared residuals is to write, for any b0 and b1,
n |
ˆ |
ˆ |
|
ˆ |
|
|
|
ˆ |
|
|
|
|
|
|
|
|
b0) |
b1)xi) |
2 |
|
|
||||||||
Q(b0,b1) (yi 0 |
1xi |
( 0 |
( 1 |
|
|
|
||||||||
i 1 |
|
|
|
|
|
|
|
|
|
|
|
|
|
|
n |
ˆ |
|
|
ˆ |
|
|
|
|
|
|
|
|
|
|
|
|
|
b1)xi) |
2 |
|
|
|
|
|
|
||||
(uˆi ( 0 b0) |
( 1 |
|
|
|
|
|
|
|
|
|||||
i 1 |
|
|
|
|
|
|
|
|
|
|
|
|
|
|
n |
|
|
|
|
|
|
|
n |
|
|
|
|
|
n |
2 |
|
ˆ |
2 |
|
ˆ |
2 |
|
2 |
|
ˆ |
ˆ |
b1) xi, |
||
uˆi |
n( 0 b0) |
( 1 |
b1) |
|
xi |
2( 0 |
b0)( 1 |
|||||||
i 1 |
|
|
|
|
|
|
|
i 1 |
|
|
|
|
|
i 1 |
where we have used equations (2.30) and (2.31). The sum of squared residuals does not depend on b0 or b1, while the sum of the last three terms can be written as
n
[( ˆ0 b0) ( ˆ1 b1)xi]2,
i 1
as can be verified by straightforward algebra. Because this is a sum of squared terms, it can be at most zero. Therefore, it is smallest when b0 = ˆ0 and b1 = ˆ1.
65
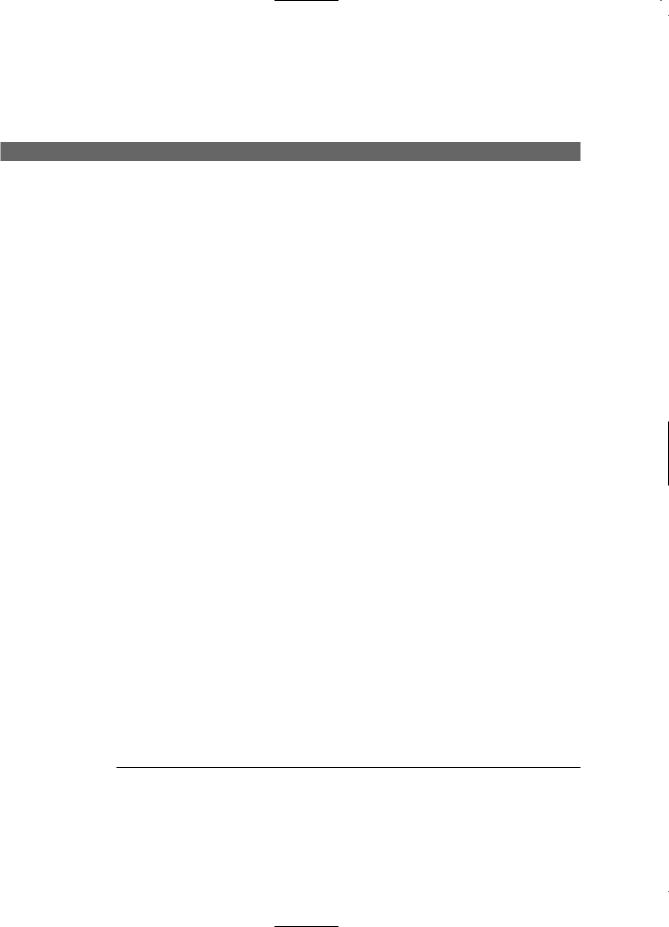
C h a p t e r Three
Multiple Regression Analysis:
Estimation
In Chapter 2, we learned how to use simple regression analysis to explain a dependent variable, y, as a function of a single independent variable, x. The primary drawback in using simple regression analysis for empirical work is that it is very difficult to draw ceteris paribus conclusions about how x affects y: the key assumption, SLR.3—that all other factors affecting y are uncorrelated with x—is often unrealistic. Multiple regression analysis is more amenable to ceteris paribus analysis because it allows us to explicitly control for many other factors which simultaneously affect the dependent variable. This is important both for testing economic theories and for evaluating policy effects when we must rely on nonexperimental data. Because multiple regression models can accommodate many explanatory variables that may be correlated, we can
hope to infer causality in cases where simple regression analysis would be misleading. Naturally, if we add more factors to our model that are useful for explaining y, then
more of the variation in y can be explained. Thus, multiple regression analysis can be used to build better models for predicting the dependent variable.
An additional advantage of multiple regression analysis is that it can incorporate fairly general functional form relationships. In the simple regression model, only one function of a single explanatory variable can appear in the equation. As we will see, the multiple regression model allows for much more flexibility.
Section 3.1 formally introduces the multiple regression model and further discusses the advantages of multiple regression over simple regression. In Section 3.2, we demonstrate how to estimate the parameters in the multiple regression model using the method of ordinary least squares. In Sections 3.3, 3.4, and 3.5, we describe various statistical properties of the OLS estimators, including unbiasedness and efficiency.
The multiple regression model is still the most widely used vehicle for empirical analysis in economics and other social sciences. Likewise, the method of ordinary least squares is popularly used for estimating the parameters of the multiple regression model.
3.1 MOTIVATION FOR MULTIPLE REGRESSION
The Model with Two Independent Variables
We begin with some simple examples to show how multiple regression analysis can be used to solve problems that cannot be solved by simple regression.
66
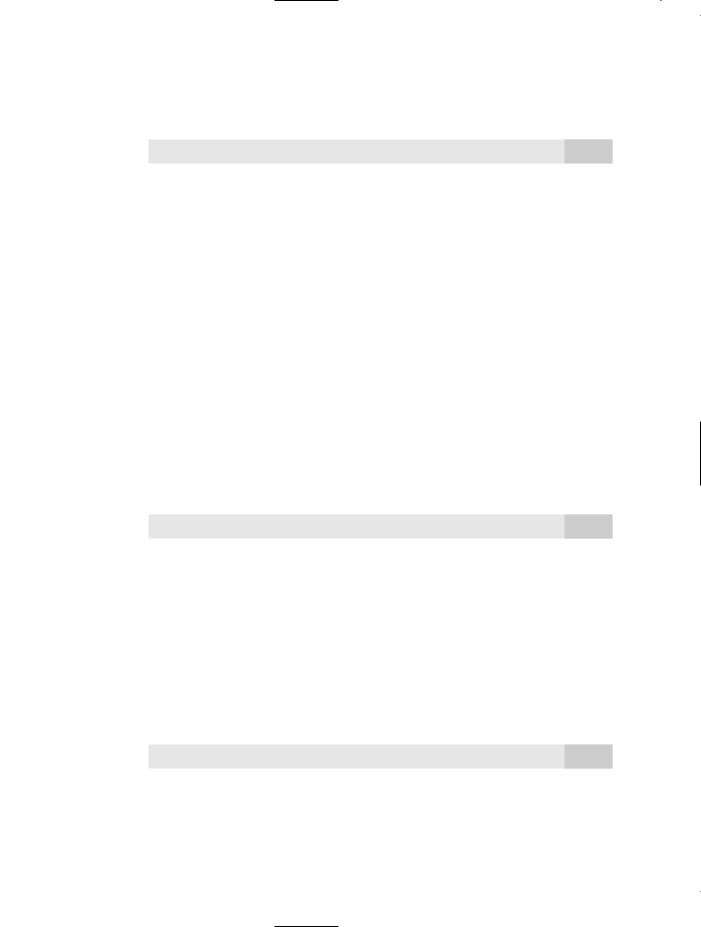
Chapter 3 |
Multiple Regression Analysis: Estimation |
The first example is a simple variation of the wage equation introduced in Chapter 2 for obtaining the effect of education on hourly wage:
wage 0 1educ 2exper u, |
(3.1) |
where exper is years of labor market experience. Thus, wage is determined by the two explanatory or independent variables, education and experience, and by other unobserved factors, which are contained in u. We are still primarily interested in the effect of educ on wage, holding fixed all other factors affecting wage; that is, we are interested in the parameter 1.
Compared with a simple regression analysis relating wage to educ, equation (3.1) effectively takes exper out of the error term and puts it explicitly in the equation. Because exper appears in the equation, its coefficient, 2, measures the ceteris paribus effect of exper on wage, which is also of some interest.
Not surprisingly, just as with simple regression, we will have to make assumptions about how u in (3.1) is related to the independent variables, educ and exper. However, as we will see in Section 3.2, there is one thing of which we can be confident: since (3.1) contains experience explicitly, we will be able to measure the effect of education on wage, holding experience fixed. In a simple regression analysis—which puts exper in the error term—we would have to assume that experience is uncorrelated with education, a tenuous assumption.
As a second example, consider the problem of explaining the effect of per student spending (expend) on the average standardized test score (avgscore) at the high school level. Suppose that the average test score depends on funding, average family income (avginc), and other unobservables:
avgscore 0 1expend 2avginc u. |
(3.2) |
The coefficient of interest for policy purposes is 1, the ceteris paribus effect of expend on avgscore. By including avginc explicitly in the model, we are able to control for its effect on avgscore. This is likely to be important because average family income tends to be correlated with per student spending: spending levels are often determined by both property and local income taxes. In simple regression analysis, avginc would be included in the error term, which would likely be correlated with expend, causing the OLS estimator of 1 in the two-variable model to be biased.
In the two previous similar examples, we have shown how observable factors other than the variable of primary interest [educ in equation (3.1), expend in equation (3.2)] can be included in a regression model. Generally, we can write a model with two independent variables as
y 0 1x1 2x2 u, |
(3.3) |
where 0 is the intercept, 1 measures the change in y with respect to x1, holding other factors fixed, and 2 measures the change in y with respect to x2, holding other factors fixed.
67
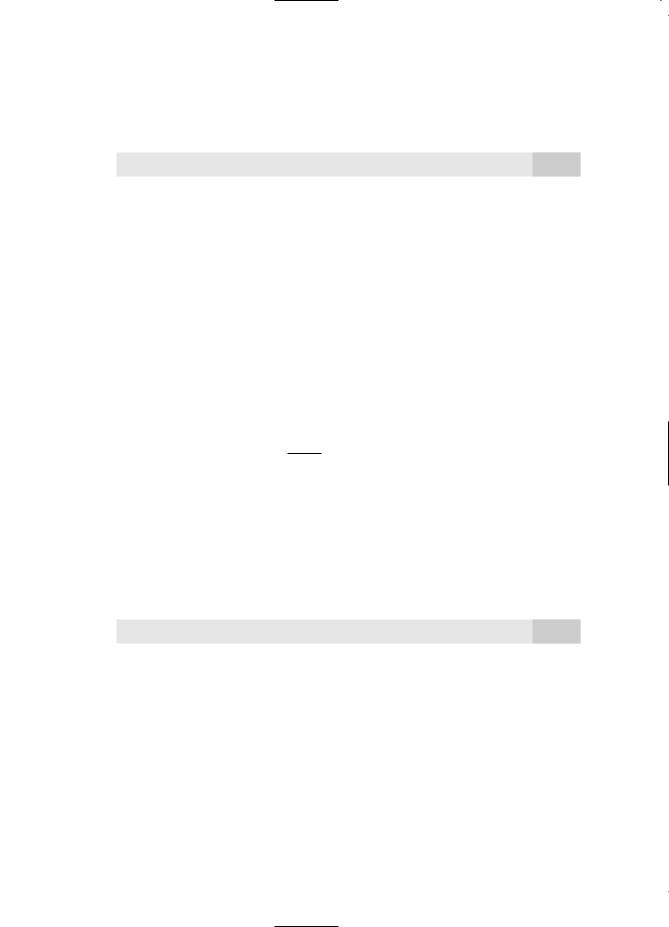
Part 1 |
Regression Analysis with Cross-Sectional Data |
Multiple regression analysis is also useful for generalizing functional relationships between variables. As an example, suppose family consumption (cons) is a quadratic function of family income (inc):
cons |
|
inc inc2 |
u, |
(3.4) |
0 |
1 |
2 |
|
|
where u contains other factors affecting consumption. In this model, consumption depends on only one observed factor, income; so it might seem that it can be handled in a simple regression framework. But the model falls outside simple regression because it contains two functions of income, inc and inc2 (and therefore three parameters, 0, 1, and 2). Nevertheless, the consumption function is easily written as a regression model with two independent variables by letting x1 inc and x2 inc2.
Mechanically, there will be no difference in using the method of ordinary least squares (introduced in Section 3.2) to estimate equations as different as (3.1) and (3.4). Each equation can be written as (3.3), which is all that matters for computation. There is, however, an important difference in how one interprets the parameters. In equation (3.1), 1 is the ceteris paribus effect of educ on wage. The parameter 1 has no such interpretation in (3.4). In other words, it makes no sense to measure the effect of inc on cons while holding inc2 fixed, because if inc changes, then so must inc2! Instead, the change in consumption with respect to the change in income—the marginal propensity to consume—is approximated by
cons
inc 1 2 2inc.
See Appendix A for the calculus needed to derive this equation. In other words, the marginal effect of income on consumption depends on 2 as well as on 1 and the level of income. This example shows that, in any particular application, the definition of the independent variables are crucial. But for the theoretical development of multiple regression, we can be vague about such details. We will study examples like this more completely in Chapter 6.
In the model with two independent variables, the key assumption about how u is related to x1 and x2 is
E(u x1,x2) 0. |
(3.5) |
The interpretation of condition (3.5) is similar to the interpretation of Assumption SLR.3 for simple regression analysis. It means that, for any values of x1 and x2 in the population, the average unobservable is equal to zero. As with simple regression, the important part of the assumption is that the expected value of u is the same for all combinations of x1 and x2; that this common value is zero is no assumption at all as long as the intercept 0 is included in the model (see Section 2.1).
How can we interpret the zero conditional mean assumption in the previous examples? In equation (3.1), the assumption is E(u educ,exper) 0. This implies that other factors affecting wage are not related on average to educ and exper. Therefore, if we think innate ability is part of u, then we will need average ability levels to be the same across all combinations of education and experience in the working population. This
68
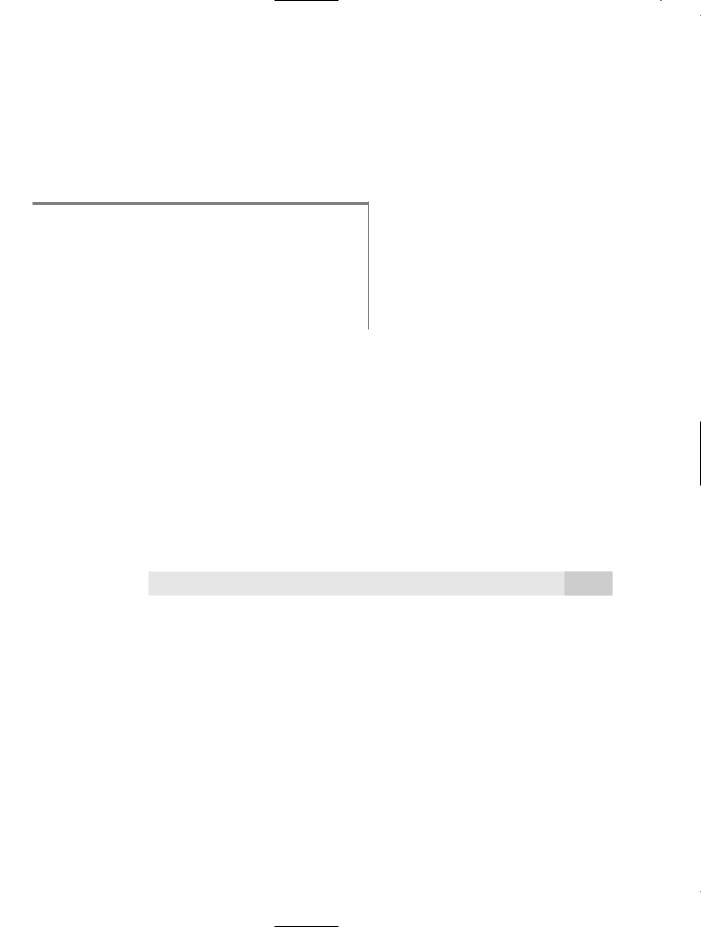
Chapter 3 Multiple Regression Analysis: Estimation
may or may not be true, but, as we will see in Section 3.3, this is the question we need to ask in order to determine whether the method of ordinary least squares produces unbiased estimators.
The example measuring student performance [equation (3.2)] is similar to the wage equation. The zero conditional mean assumption is E(u expend,avginc) 0, which means that other factors affecting test scores—school or student characteristics—are, on average, unrelated to per student fund-
ing and average family income.
When applied to the quadratic consumption function in (3.4), the zero conditional mean assumption has a slightly different interpretation. Written literally, equation (3.5) becomes E(u inc,inc2) 0. Since inc2 is known when inc is known, including inc2 in the expectation is redundant: E(u inc,inc2) 0 is the same as
E(u inc) 0. Nothing is wrong with putting inc2 along with inc in the expectation when stating the assumption, but E(u inc) 0 is more concise.
The Model with k Independent Variables
Once we are in the context of multiple regression, there is no need to stop with two independent variables. Multiple regression analysis allows many observed factors to affect y. In the wage example, we might also include amount of job training, years of tenure with the current employer, measures of ability, and even demographic variables like number of siblings or mother’s education. In the school funding example, additional variables might include measures of teacher quality and school size.
The general multiple linear regression model (also called the multiple regression model) can be written in the population as
y 0 1x1 2x2 3x3 … kxk u, |
(3.6) |
where 0 is the intercept, 1 is the parameter associated with x1, 2 is the parameter associated with x2, and so on. Since there are k independent variables and an intercept, equation (3.6) contains k 1 (unknown) population parameters. For shorthand purposes, we will sometimes refer to the parameters other than the intercept as slope parameters, even though this is not always literally what they are. [See equation (3.4), where neither 1 nor 2 is itself a slope, but together they determine the slope of the relationship between consumption and income.]
The terminology for multiple regression is similar to that for simple regression and is given in Table 3.1. Just as in simple regression, the variable u is the error term or disturbance. It contains factors other than x1, x2, …, xk that affect y. No matter how many explanatory variables we include in our model, there will always be factors we cannot include, and these are collectively contained in u.
When applying the general multiple regression model, we must know how to interpret the parameters. We will get plenty of practice now and in subsequent chapters, but
69
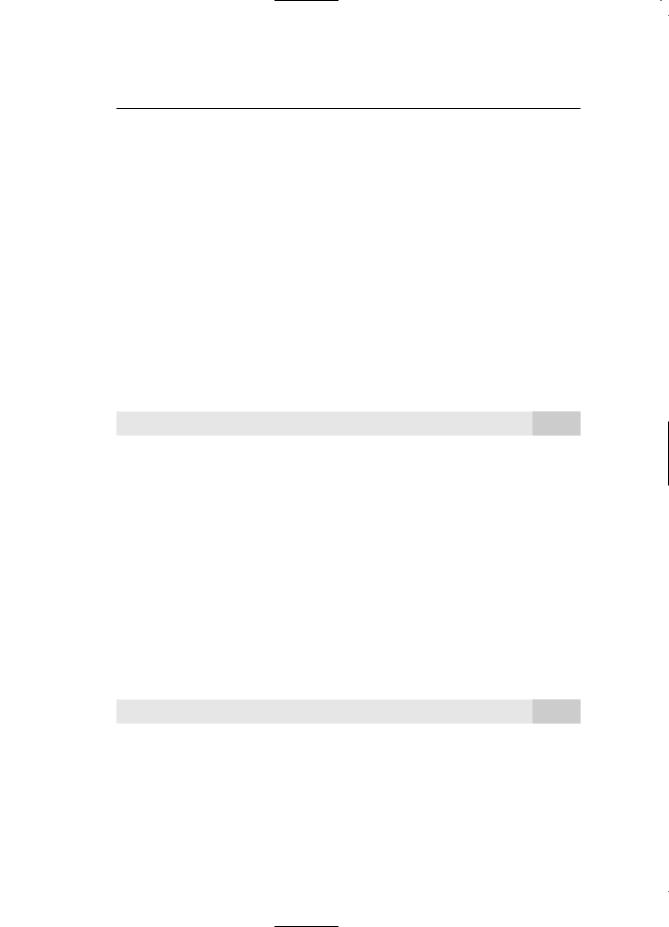
Part 1 Regression Analysis with Cross-Sectional Data
Table 3.1
Terminology for Multiple Regression
y |
x1, x2, …, xk |
|
|
Dependent Variable |
Independent Variables |
|
|
Explained Variable |
Explanatory Variables |
|
|
Response Variable |
Control Variables |
|
|
Predicted Variable |
Predictor Variables |
|
|
Regressand |
Regressors |
|
|
it is useful at this point to be reminded of some things we already know. Suppose that CEO salary (salary) is related to firm sales and CEO tenure with the firm by
log(salary) 0 1log(sales) 2ceoten 3ceoten2 u. |
(3.7) |
This fits into the multiple regression model (with k 3) by defining y log(salary), x1 log(sales), x2 ceoten, and x3 ceoten2. As we know from Chapter 2, the parameter 1 is the (ceteris paribus) elasticity of salary with respect to sales. If 3 0, then 100 2 is approximately the ceteris paribus percentage increase in salary when ceoten increases by one year. When 3 0, the effect of ceoten on salary is more complicated. We will postpone a detailed treatment of general models with quadratics until Chapter 6.
Equation (3.7) provides an important reminder about multiple regression analysis. The term “linear” in multiple linear regression model means that equation (3.6) is linear in the parameters, j. Equation (3.7) is an example of a multiple regression model that, while linear in the j, is a nonlinear relationship between salary and the variables sales and ceoten. Many applications of multiple linear regression involve nonlinear relationships among the underlying variables.
The key assumption for the general multiple regression model is easy to state in terms of a conditional expectation:
E(u x1,x2, …, xk) 0. |
(3.8) |
At a minimum, equation (3.8) requires that all factors in the unobserved error term be uncorrelated with the explanatory variables. It also means that we have correctly accounted for the functional relationships between the explained and explanatory variables. Any problem that allows u to be correlated with any of the independent variables causes (3.8) to fail. In Section 3.3, we will show that assumption (3.8) implies that OLS is unbiased and will derive the bias that arises when a key variable has been omitted
70