
Rivero L.Encyclopedia of database technologies and applications.2006
.pdfdata have been generated. Scientists often have to use exploratory methods instead of confirming already suspected hypotheses. Data mining is a research area that aims to provide the analysts with novel and efficient computational tools to overcome the obstacles and constraints posed by the traditional statistical methods. Feature selection, normalization, and standardization of the data, visualization of the results, and evaluation of the produced knowledge are equally important steps in the knowledge discovery process. The mission of bioinformatics as a new and critical research domain is to provide the tools and use them to extract accurate and reliable information in order to gain new biological insights.
REFERENCES
2can Bioinformatics. (2004). Bioinformatics introduction. Retrieved February 2, 2005, from http://www.ebi.ac.uk/ 2can/bioinformatics/index.html
Aas, K. (2001). Microarray data mining: A survey. NR Note, SAMBA, Norwegian Computing Center.
Brazma, A., Parkinson, H., Schlitt, T., & Shojatalab, M. (2004). A quick introduction to elements of biology: Cells, molecules, genes, functional genomics, microarrays. Retrieved February 2, 2005, from http://www.ebi.ac.uk/ microarray/biology_intro.html
Dudoit, S., Fridlyand, J., & Speed, T.P. (2002). Comparison of discrimination methods for the classifcation of tumors using gene expression data. Journal of the American Statistical Association, 97(457), 77-87.
Dunham, M.H. (2002). Data mining: Introductory and advanced topics. Upper Saddle River, NJ: Prentice Hall.
Fayyad, U.M., Piatetsky-Shapiro, G., Smyth, P., & Uthurusamy, R. (1996). Advances in knowledge discovery and data mining. Menlo Park, CA: AAAI Press/MIT Press.
Ghosh, D., & Chinnaiyan, A.M. (2002). Mixture modeling of gene expression data from microarray experiments.
Bioinformatics, 18, 275-286.
Golub, T.R., Slonim, D.K., Tamayo, P., Huard, C., Gaasenbeek, M., Mesirov, J.P., et al. (1999). Molecular classification of cancer: Class discovery and class prediction by gene expression monitoring. Science, 286(5439), 531-537.
Han, J. (2002). How can data mining help bio-data analysis? Proceedings of the 2nd ACM SIGKDD Workshop on Data Mining in Bioinformatics (pp. 1-2).
Biological Data Mining
Hastie, T., Tibshirani, R., Eisen, M.B., Alizadeh, A., Levy, R., Staudt, L., et al. (2000). ‘Gene shaving’ as a method for identifying distinct sets of genes with similar expression patterns. Genome Biology, 1(2), research0003.
Hirsh, H., & Noordewier, M. (1994). Using background knowledge to improve inductive learning of DNA sequences. Proceedings of the 10th IEEE Conference on Artificial Intelligence for Applications (pp. 351-357).
Houle, J.L., Cadigan, W., Henry, S., Pinnamaneni, A., & Lundahl, S. (2004). Database mining in the human genome initiative. Whitepaper, Bio-databases.com, Amita Corporation. Retrieved February 2, 2005, from http:// www.biodatabases.com/ whitepaper.html
Hunter, L. (2004). Life and its molecules: A brief introduction. AI Magazine, 25(1), 9-22.
Kerr, M.K., & Churchill, G.A. (2001). Bootstrapping cluster analysis: Assessing the reliability of conclusions from microarray experiments. Proceedings of the National Academy of Sciences, 98 (pp. 8961-8965).
Lazzeroni, L., & Owen, A. (2002). Plaid models for gene expression data. Statistica Sinica, 12(2002), 61-86.
Li, J., Ng, K.-S., & Wong, L. (2003). Bioinformatics adventures in database research. Proceedings of the 9th International Conference on Database Theory (pp. 31-46).
Luscombe, N.M., Greenbaum, D., & Gerstein, M. (2001). What is bioinformatics? A proposed definition and overview of the field. Methods of Information in Medicine, 40(4), 346-358.
Ma, Q., & Wang, J.T.L. (1999). Biological data mining using Bayesian neural networks: A case study. International Journal on Artificial Intelligence Tools, Special Issue on Biocomputing, 8(4), 433-451.
Molla, M., Waddell, M., Page, D., & Shavlik, J. (2004). Using machine learning to design and interpret geneexpression microarrays. AI Magazine, 25(1), 23-44.
National Center for Biotechnology Information. (2004). Genbank statistics. Retrieved February 2, 2005, from http:/ /www.ncbi.nlm.nih.gov/Genbank/genbankstats.html
Piatetsky-Shapiro, G., & Tamayo, P. (2003). Microarray data mining: Facing the challenges. SIGKDD Explorations, 5(2), 1-5.
Sander, J., Ester, M., Kriegel, P.-H., & Xu, X. (1998). Density-based clustering in spatial databases: The algorithm GDBSCAN and its applications. Data Mining and Knowledge Discovery, 2, (pp. 169-194).
40
TEAM LinG

Biological Data Mining
Smolkin, M., & Ghosh, D. (2003). Cluster stability scores for microarraydataincancerstudies.BMCBioinformatics,4,36.
Tibshirani, R., Hastie, T., Eisen, M., Ross, D., Botstein, D., & Brown, P. (1999). Clustering methods for the analysis of DNA microarray data (Tech. Rep.). Stanford, CA: Stanford University.
Two Crows Corporation. (1999). Introduction to data mining and knowledge discovery (3rd ed.).
U.S. Department of Energy Office of Science. (2004). Human Genome Project information. Retrieved February 2, 2005, from http://www.ornl.gov/sci/techresources/ Human_Genome/home.shtml
Velculescu, V.E., Zhang, L., Vogelstein, B., & Kinzler, K.W. (1995). Serial analysis of gene expression. Science, 270(5235), 484-487.
Whishart, D.S. (2002). Tools for protein technologies. In C.W. Sensen (Ed.), Biotechnology, (Vol 5b) genomics and bioinformatics (pp. 325-344). Wiley-VCH.
Wikipedia Online Encyclopedia. (2004). Retrieved February 2, 2005, from http://en2.wikipedia.org
Yeung, Y.K., Medvedovic, M., & Bumgarner, R.E. (2003). Clustering gene-expression data with repeated measurements. Genome Biology, 4(5), R34.
Zeng, F., Yap C.H.R., & Wong, L. (2002). Using feature generation and feature selection for accurate prediction of translation initiation sites. Genome Informatics, 13, 192-200.
Zien, A., Ratsch, G., Mika, S., Scholkopf, B., Lengauer, T.,
& Muller, R.-K. (2000). Engineering support vector ma- B chine kernels that recognize translation initiation sites.
Bioinformatics, 16(9), 799-807.
KEY TERMS
Data Cleaning (Cleansing): The act of detecting and removing errors and inconsistencies in data to improve its quality.
Genotype: The exact genetic makeup of an organism.
Machine Learning: An area of artificial intelligence, the goal of which is to build computer systems that can adapt and learn from their experience.
Mapping: The process of locating genes on a chromosome.
Phenotype: The physical appearance characteristics of an organism.
Sequence Alignment: The process to test for similarities between a sequence of an unknown target protein and a single (or a family of) known protein(s).
Sequencing: The process of determining the order of nucleotides in a DNA or RNA molecule or the order of amino acids in a protein.
Visualization: Graphical display of data and models facilitating the understanding and interpretation of the information contained in them.
41
TEAM LinG
42
Biometric Databases
MayankVatsa
Indian Institute of Technology, India
Richa Singh
Indian Institute of Technology, India
P.Gupta
Indian Institute of Technology, India
A.K.Kaushik
Ministry of Communication and Information Technology, India
INTRODUCTION
Biometric databases are the set of biometric features collected from a large public domain for the evaluation of biometric systems. For the evaluation of any algorithm for a particular biometric trait, the database should be a large collection of that particular biometric trait. The creation and maintenance of biometric databases involve various steps like enrollment and training.
Enrollment is storing the identity of an individual in the biometric system with his/her biometric templates. Every system has some enrollment policy, like the procedure for capturing the biometric samples, quality of the templates. These policies should be made such that they are acceptable to the public. For example, the procedure for capturing iris images involves the use of infrared light, which might even cause damage to human eyes. The signature of any individual is considered private for him, and he might have some objection to giving his signature for enrollment in any such system because of the unpredicted use of their templates (where and how this information may be used).
There are two types of enrollment, positive enrollment and negative enrollment. Positive enrollment is for candidates with correct identity civilians while negative enrollment is for unlawful or prohibited people.
For enrollment, the biometric sample captured should be such that the algorithms can perform well on that. The features which are required for any algorithm to verify or match the template should be present. The poor biometric sample quality increases the failure to enroll rate. For verification also, if the biometric sample is not captured properly this may lead to an increase in false acceptance and false rejection rates. This increase in failure to enroll and false acceptance and false rejection rates increases the manual intervention for every task, which leads to a further increase in the operational cost along with the risk in security.
Training is tuning the system for noise reduction, preprocessing, and extracting the region of interest so as to increase the verification accuracy (decreasing the false acceptance and false rejection rates).
MAIN THRUST
There are various biometric databases available in the public domain for researchers to evaluate their algorithms. Given below are the various databases for different biometric traits along with their specifications and Web sites.
Face Database
The face database is the necessary requirement for a face authentication system. The face database considers differing scaling factors, rotational angles, lighting conditions, background environment, and facial expressions.
Most of the algorithms have been developed using photo databases and still images that were created under a constant predefined environment. Since a controlled environment removes the need for normalization, reliable techniques have been developed to recognize faces with reasonable accuracy with the constraint that the database contains perfectly aligned and normalized face images. Thus the challenge for face recognition systems lies not in the recognition of the faces, but in normalizing all input face images to a standardized format that is compliant with the strict requirements of the face recognition module (Vatsa, Singh, & Gupta, 2003; Zhao, Chellappa, Rosenfeld, & Philips, 2000). Although there are models developed to describe faces, such as texture mapping with the Candide model, there are no clear definitions or mathematical models to specify what the important and necessary components are in describing a face.
Copyright © 2006, Idea Group Inc., distributing in print or electronic forms without written permission of IGI is prohibited.
TEAM LinG

Biometric Databases
Using still images taken under restraint conditions as input, it is reasonable to omit the face normalization stage. However, when locating and segmenting a face in complex scenes under unconstraint environments, such as in a video scene, it is necessary to define and design a standardized face database.
There are several face databases are available which can be used for face detection and recognition (see Table 1). The FERET database contains monochrome images taken in different frontal views and in left and right profiles. Only the upper torso of an individual (mostly head and neck) appears in an image on a uniform and uncluttered background. Turk and Pentland created a face database of 16 people. The face database from AT&T Cambridge Laboratories, formerly known as the Olivetti database and also known as the ORL-AT&T database, consists of 10 different images for 40 persons. The images were taken at different times, varying the lighting, facial expressions, and facial details. The Harvard database consists of cropped, masked frontal face images taken from a wide variety of light sources. There are images of 16 individuals in the Yale face database, which contains 10 frontal images per person, each with different facial expressions, with and without glasses, and under different lighting conditions. The XM2VTS multimodal database contains sequences of face images of 37 people. The five sequences for each person were taken over one week. Each image sequence contains images from right profile (- 90 degree) to left profile (90 degree) while the subjects count from 0 to 9 in their native languages. The UMIST database consists of 564 images of 20 people with varying poses. The images of each subject cover a range of poses from right profile to frontal views. The Purdue AR database contains over 3,276 color images of 126 people (70 males and 56 females) in frontal view. This database is designed for face recognition experiments under several mixing factors such as facial expressions, illumination conditions, and occlusions. All the faces appear with different facial expressions (neutral, smile, anger, and scream), illumination (left light source, right light source, and sources from both sides), and occlusion (wearing sunglasses or scarf). The images were taken during two sessions separated by two weeks. All the images were taken by the same camera setup under tightly controlled conditions of illumination and pose. This face database has been applied to image and video indexing as well as retrieval. Vatsa et al. (2003) and Zhao et al. (2000) can be referred to for the details of preparation of face databases. These works have described how to prepare the face image database and the challenges in database preparation.
Table 1. Brief description of popular face databases
B
Face |
Specifications |
WWW Address |
|
Database |
|||
|
|
||
AR Face |
4,000 color images of 126 people, |
http://rvl1.ecn.purdue.edu/~aleix |
|
with different facial expressions, |
|||
Database |
/aleix_face_DB.html |
||
illumination, and occlusions. |
|||
|
|
||
|
It consists of ten 92-by-112-pixel |
|
|
AT&T Face |
gray scale images of 40 subjects, |
http://www.uk.research.att.com/f |
|
Database |
with a variety of lightning and |
acedatabase.html |
|
|
facial expressions. |
|
|
|
114 persons, 7 images for each |
|
|
CVL Face |
person, under uniform |
|
|
illumination, no flash, and with |
http://lrv.fri.uni-lj.si/facedb.html |
||
Database |
|||
projection screen in the |
|
||
|
|
||
|
background. |
|
|
CMU/VASC |
2,000 image sequences from over |
http://vasc.ri.cmu.edu/idb/html/f |
|
200 subjects. |
ace/facial_expression/ |
||
|
|||
|
Largest collection of faces, over |
|
|
FERET |
14,000 images, with different |
http://www.itl.nist.gov/iad/huma |
|
Database |
facial expressions, illumination, |
nid/feret/ |
|
|
and positions. |
|
|
Harvard |
Cropped, masked frontal face |
http://cvc.yale.edu/people/facult |
|
images under a wide range of |
|||
Database |
y/belhumeur.html |
||
illumination conditions. |
|||
|
|
||
|
1,000 color images of 50 |
|
|
IITK Face |
individuals in lab environment, |
http://www.cse.iitk.ac.in/users/m |
|
Database |
having different poses, occlusions, |
ayankv |
|
|
and head orientations. |
|
|
Extended |
XM2VTSDB contains four |
|
|
recordings of 295 subjects taken |
|
||
M2VTS |
http://www.ee.surrey.ac.uk/Rese |
||
over a period of four months. Each |
|||
Database |
arch/VSSP/xm2vtsdb |
||
recording contains a speaking head |
|||
XM2VTSDB |
|
||
shot and a rotating head shot. |
|
||
|
|
||
|
37 different faces and 5 shots for |
|
|
|
each person, taken at one-week |
|
|
M2VTS |
intervals or when drastic face |
|
|
Multimodal |
changes occurred, from 0 to 9 |
http://www.tele.ucl.ac.be/PROJE |
|
Face |
speaking head rotation and from 0 |
CTS/M2VTS/ |
|
Database |
to 90 degrees and then back to 0 |
|
|
|
degrees, and once again without |
|
|
|
glasses if they wore. |
|
|
Max Planck |
|
|
|
Institute for |
7 views of 200 laser-scanned heads |
http://faces.kyb.tuebingen.mpg.d |
|
Biological |
without hair. |
e/ |
|
Cybernetics |
|
|
|
|
Faces of 16 people, 27 of each |
|
|
MIT Database |
person, under various conditions of |
ftp://whitechapel.media.mit.edu/ |
|
illumination, scale, and head |
pub/images/ |
||
|
|||
|
orientation. |
|
|
NIST 18 Mug |
Consists of 3,248 mug shot |
|
|
images, 131 cases with both profile |
|
||
Shot |
http://www.nist.gov/szrd/nistd18 |
||
and frontal views, 1,418 with only |
|||
Identification |
.htm |
||
frontal view, scanning resolution is |
|||
Database |
|
||
500 dpi. |
|
||
|
|
||
|
|
|
|
|
Consists of 564 images of 20 |
|
|
UMIST |
people, covering a range of |
http://images.ee.umist.ac.uk/dan |
|
Database |
different poses from profile to |
ny/database.html |
|
|
frontal views. |
|
|
|
125 different faces, each in 16 |
|
|
University of |
different camera calibrations and |
|
|
illuminations, an additional 16 if |
|
||
Oulu Physics- |
http://www.ee.oulu.fi/research/i |
||
the person has glasses, faces in |
|||
Based Face |
mag/color/pbfd.html |
||
frontal position captured under |
|||
Database |
|
||
horizon, incandescent, fluorescent, |
|
||
|
|
||
|
and daylight illuminance. |
|
|
University of |
300 frontal-view face images of 30 |
ftp://iamftp.unibe.ch/pub/Images |
|
Bern |
people and 150 profile face |
||
/FaceImages/ |
|||
Database |
images. |
||
|
|||
Usenix |
5,592 faces from 2,547 sites. |
ftp://ftp.uu.net/published/usenix/ |
|
faces/ |
|||
|
|
||
Weizmann |
28 faces, includes variations due to |
ftp.eris.weizmann.ac.il |
|
Institute of |
changes in viewpoint, illumination, |
||
ftp.wisdom.weizmann.ac.il |
|||
science |
and three different expressions. |
||
|
|||
Yale Face |
165 images (15 individuals), with |
|
|
different lighting, expression, and |
http://cvc.yale.edu |
||
Database |
|||
occlusion configurations. |
|
||
|
|
||
|
5,760 single light source images of |
|
|
Yale Face |
10 subjects, each seen under 576 |
http://cvc.yale.edu/ |
|
Database B |
viewing conditions (9 poses x 64 |
||
|
|||
|
illumination conditions). |
|
43
TEAM LinG
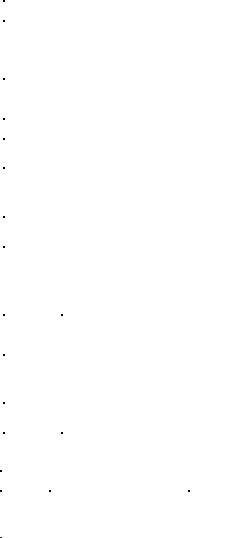
Fingerprint Database
Fingerprints are the ridge and furrow patterns on the tip of the finger and have been used extensively for personal identification of people. Fingerprint-based biometric systems offer positive identification with a very high degree of confidence. Thus, fingerprint-based authentication is becoming popular in a number of civilian and commercial applications. Fingerprints even have a number of disadvantages as compared to other biometrics. Some of the people do not have clear fingerprints; for example, people working in mines and construction sites get regular scratches on their fingers. Finger skin peels off due to weather, sometimes develops natural permanent creases, and even forms temporary creases when the hands are immersed in water for a long time. Such fingerprints can not be properly imaged with existing fingerprint sensors. Further, since fingerprints can not be captured without the user’s knowledge, they are not suited for certain applications such as surveillance.
For the database, the user has the option of giving the fingerprint of any finger at the time of enrollment; of course, one should give the image of the same finger for correct matching at the time of verification also. The advantage with the generalization in considering the finger for authentication is that if anyone has a damaged thumb, then we can authenticate using the other finger. Also the performance and efficiency of any algorithm are absolutely independent of the choice of the finger. For the database, right-hand thumb images have been taken, but in case of any problem some other finger can also be considered. Some of the important issues of preparation of fingerprint image databases are discussed in the literature (Maltoni, Maio, Jain, & Prabhakar, 2003; Vatsa et al., 2003). The important issues are as follows.
1.Resolution of image: The resolution should be good enough to detect the basic features, i.e., loops, whorls, and arches in the fingerprint.
2.The part of thumb in fingerprint image: The fingerprint image must contain the basic features like whorls, arches, etc.
3.The orientation of fingerprint: The orientation is taken with the help of ridge flow direction. Because of the nature of fingerprint features, orientation also matters and thus is considered as a feature for recognition.
Iris Database
Iris recognition has been an active research topic in recent years due to its high accuracy. The only database available in the public domain is CASIA Iris Database. To prepare
|
|
|
|
Biometric Databases |
||||||
Table 2. |
|
|
|
|
|
|
|
|
||
|
|
|
|
|
|
|
|
|
|
|
|
Fingerprint |
|
Specifications |
|
|
|
WWW Address |
|
||
|
Database |
|
|
|
|
|
||||
|
|
|
|
|
|
|
|
|
||
|
|
|
|
4 databases, each database consists of |
|
|
|
|
||
|
|
|
|
fingerprints from 110 individuals and 8 |
|
|
http://bias.csr.unib |
|
||
|
|
|
|
images each. Images were taken at different |
|
|
|
|||
|
FVC 2000 |
|
|
|
o.it/fvc2000/databa |
|
||||
|
|
resolutions by different scanners at 500 dpi. |
|
|
|
|||||
|
|
|
|
|
|
ses.asp |
|
|||
|
|
|
|
One database (DB4) contains synthetically |
|
|
|
|||
|
|
|
|
|
|
|
|
|||
|
|
|
|
generated fingerprints also. |
|
|
|
|
|
|
|
|
|
|
4 databases, each consisting of 110 |
|
|
http://bias.csr.unib |
|
||
|
|
|
|
individuals with 8 images per individual at |
|
|
|
|||
|
FVC 2002 |
|
|
|
o.it/fvc2002/databa |
|
||||
|
|
500 dpi. One database (DB4) contains |
|
|
|
|||||
|
|
|
|
|
|
ses.asp |
|
|||
|
|
|
|
synthetically generated fingerprints also. |
|
|
|
|||
|
|
|
|
|
|
|
|
|||
|
NIST 4 |
|
Consists of 2,000 fingerprint pairs (400 image |
|
http://www.nist.go |
|
||||
|
|
pairs from 5 classes) at 500 dpi. |
|
|
v/srd/nistsd4.htm |
|
||||
|
|
|
|
|
|
|
||||
|
|
|
|
Consists of 5 volumes, each has 270 mated |
|
|
http://www.nist.go |
|
||
|
NIST 9 |
|
card pairs of fingerprints (total of 5,400 |
|
|
|
||||
|
|
|
|
v/srd/nistsd9.htm |
|
|||||
|
|
|
|
images). Each image is 832 by 768 at 500 dpi. |
|
|
||||
|
|
|
|
|
|
|
||||
|
|
|
|
Consists of fingerprint patterns that have a |
|
|
|
|
||
|
|
|
|
low natural frequency of occurrence and |
|
|
http://www.nist.go |
|
||
|
NIST 10 |
|
transitional fingerprint classes. There are |
|
|
|
||||
|
|
|
|
v/srd/nistsd10.htm |
|
|||||
|
|
|
|
5,520 images of size 832 by 768 pixels at 500 |
|
|
||||
|
|
|
|
|
|
|
||||
|
|
|
|
dpi. |
|
|
|
|
|
|
|
|
|
|
27,000 fingerprint pairs with 832 by 768 |
|
|
http://www.nist.go |
|
||
|
NIST 14 |
|
pixels at 500 dpi. Images show a natural |
|
|
|
||||
|
|
|
|
v/srd/nistsd14.htm |
|
|||||
|
|
|
|
distribution of fingerprint classes. |
|
|
|
|||
|
|
|
|
|
|
|
|
|||
|
|
|
|
There are two components. First component |
|
|
|
|
||
|
|
|
|
consists of MPEG-2 compressed video of |
|
|
|
|
||
|
|
|
|
fingerprint images acquired with intentionally |
|
http://www.nist.go |
|
|||
|
NIST 24 |
|
distorted impressions; each frame is 720 by |
|
|
|
||||
|
|
|
|
v/srd/nistsd24.htm |
|
|||||
|
|
|
|
480 pixels. Second component deals with |
|
|
|
|||
|
|
|
|
|
|
|
|
|||
|
|
|
|
rotation; it consists of sequences of all 10 |
|
|
|
|
||
|
|
|
|
fingers of 10 subjects. |
|
|
|
|
|
|
|
|
|
|
Consists of 258 latent fingerprints from crime |
|
|
|
|||
|
NIST 27 |
|
scenes and their matching rolled and latent |
|
|
http://www.nist.go |
|
|||
|
|
fingerprint mates with the minutiae marked on |
|
v/srd/nistsd27.htm |
|
|||||
|
|
|
|
|
|
|||||
|
|
|
|
both the latent and rolled fingerprints. |
|
|
|
|
||
|
|
|
|
Consists of 4,320 rolled fingers from 216 |
|
|
|
|
||
|
|
|
|
individuals (all 10 fingers) scanned at 500 dpi |
|
http://www.nist.go |
|
|||
|
NIST 29 |
|
and scanned using WSQ. Two impressions of |
|
|
|||||
|
|
|
v/srd/nistsd29.htm |
|
||||||
|
|
|
|
each finger are available in addition to the |
|
|
|
|||
|
|
|
|
|
|
|
|
|||
|
|
|
|
plain finger images. |
|
|
|
|
|
|
|
|
|
|
Consists of 36 ten-print paired cards with both |
|
http://www.nist.go |
|
|||
|
NIST 30 |
|
the rolled and plain images scanned at 500 dpi |
|
|
|||||
|
|
|
v/srd/nistsd30.htm |
|
||||||
|
|
|
|
and 1,500 dpi. |
|
|
|
|
||
|
|
|
|
|
|
|
|
|
||
Table 3. |
|
|
|
|
|
|
|
|
||
|
|
|
|
|
|
|
|
|
|
|
|
Iris |
|
|
Specification |
|
|
WWW Address |
|||
|
Database |
|
|
|
|
|||||
|
|
|
|
|
|
|
|
|
|
|
|
|
|
It includes 756 iris images from 108 |
|
|
|
|
|
|
|
|
CASIA |
|
|
classes. For each eye, 7 images are |
|
http://www.sinobiometrics.com/r |
||||
|
|
|
captured in two sessions, where 3 |
|
||||||
|
Database |
|
|
|
|
|
esources.htm |
|||
|
|
|
samples are collected in the first |
|
|
|
||||
|
|
|
|
|
|
|
|
|
|
|
|
|
|
|
session and 4in the second session. |
|
|
|
|
|
|
a database of iris images, it is required to have a setup consisting of a camera and lighting system. The details of the setup can be found in Daugman (1998) and Wildes (1999).
Speaker Recognition Databases
Speaker recognition is finding the peculiar characters that the speaker possesses and reveals in his speech. The issues involved in designing a database for speaker recognition are the type of speech (text dependent or text independent), duration of the acquired speech, and acoustic environment (Campbell & Reynolds, 1999).
44
TEAM LinG
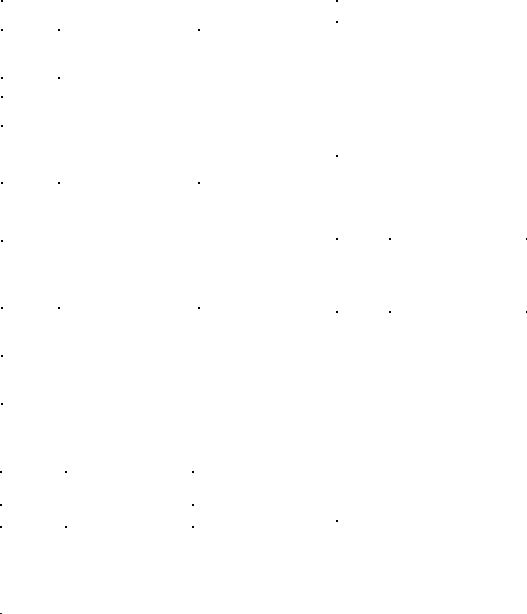
Biometric Databases
Table 4.
Speaker |
|
|
|
Recognition |
Specifications |
WWW Address |
|
Database |
|
|
|
|
Consists of 2,728 five-minute |
|
|
|
conversations with 640 speakers. For |
http://www.nist.gov/speech/tes |
|
NIST |
every call by the same person, different |
||
ts/spk/index.htm |
|||
|
handsets were used; whereas, the |
||
|
|
||
|
receiver always had the same handset. |
|
|
TIMIT |
It consists of 630 speakers, where the |
http://www.ldc.upenn.edu/Cat |
|
subjects read 10 sentences each. |
alog/LDC93S1.html |
||
|
|||
|
The original TIMIT recordings were |
http://www.ldc.upenn.edu/Cat |
|
NTIMIT |
transmitted over long-distance phone |
||
alog/LDC93S2.html |
|||
|
lines and redigitized. |
||
|
|
||
|
It consists of 128 subjects speaking the |
|
|
|
prompted digit phrases into high |
|
|
YOHO |
quality handsets. Four enrollment |
http://www.ldc.upenn.edu/Cat |
|
Database |
sessions were held, followed by 10 |
alog/LDC94S16.html |
|
|
verification sessions at an interval of |
|
|
|
several days. |
|
|
|
It consists of telephone speech from |
|
|
|
about 1,084 participants. Twelve |
|
|
OGI Database |
sessions were conducted over a 2-year |
http://cslu.cse.ogi.edu/corpora/ |
|
period, with different noise conditions |
spkrec/ |
||
|
|||
|
and using different handsets and |
|
|
|
telephone environments. |
|
|
|
It is an Italian speaker database |
|
|
|
consisting of 2,000 speakers. The |
|
|
ELRA— |
recordings were made in a house or |
http://www.elda.fr/catalogue/e |
|
SIVA |
office environment over several |
||
n/speech/S0028.html |
|||
Database |
sessions. It contains the data of |
||
|
|||
|
imposters also. |
|
|
|
|
|
|
|
The speakers are comprised of native |
|
|
ELRA— |
and non-native speakers of French |
http://www.elda.fr/catalogue/e |
|
PolyVar |
(mainly from Switzerland). It consists |
||
n/speech/S0046.html |
|||
Database |
of 143 Swiss-German and French |
||
|
|||
|
speakers with 160 hours of speech. |
|
|
|
The database contains 1,285 calls |
|
|
ELRA— |
(around 10 sessions per speaker) |
http://www.elda.fr/catalogue/e |
|
PolyCost |
recorded by 133 speakers. Data was |
||
n/speech/S0042.html |
|||
Database |
collected over ISDN phone lines with |
||
|
|||
|
foreigners speaking English. |
|
Table 5.
Online |
|
|
|
Handwriting |
Specifications |
WWW Address |
|
Database |
|
|
|
UPINEN |
It consists of over 5 million |
http://unipen.nici.kun.nl |
|
Database |
characters from 2,200 writers. |
||
|
|||
|
It consists of lines of text by |
|
|
|
around 200 writers. Total |
|
|
CEDAR |
number of words in the |
|
|
database is 105,573. The |
http://www.cedar.buffalo.ed |
||
Handwriting |
|||
database contains both cursive |
u/handwriting |
||
Database |
|||
and printed writing, as well as |
|
||
|
|
||
|
some writing which is a |
|
|
|
mixture of cursive and printed. |
|
Table 6. |
|
|
|
|
|
|
|
B |
|
|
|
|
|
|
Gait |
Specifications |
WWW Address |
|
|
|
|
|||
Database |
|
|
||
|
|
|
|
|
|
It consists of image sequences |
|
|
|
|
captured in an outdoor |
|
|
|
|
environment with natural light by a |
|
|
|
|
surveillance camera (Panasonic |
|
|
|
|
NV-DX100EN digital video |
|
|
|
NLPR Gait |
camera). The database contains 20 |
http://www.sinobiometrics.co |
|
|
different subjects and three views, |
|
|||
Database |
m/gait.htm |
|
|
|
namely, lateral view, frontal view, |
|
|||
|
|
|
|
|
|
and oblique view with respect to |
|
|
|
|
the image plane. The captured 24- |
|
|
|
|
bit full color images of 240 |
|
|
|
|
sequences have a resolution of 352 |
|
|
|
|
by 240. |
|
|
|
|
It contains a data set over 4 days, |
|
|
|
|
the persons walking in an elliptical |
|
|
|
USF Gait |
path at an axis of (minor ~= 5m |
|
|
|
and major ~= 15m). The variations |
http://figment.csee.usf.edu/Gai |
|
|
|
Baseline |
|
|||
considered are different |
tBaseline/ |
|
|
|
Database |
|
|||
viewpoints, shoe types, carrying |
|
|
|
|
|
|
|
|
|
|
conditions, surface types, and even |
|
|
|
|
some at 2 differing time instants. |
|
|
|
|
It consists of 25 subjects walking |
|
|
|
|
with 4 different styles of walking |
|
|
|
CMU |
(slow walk, fast walk, inclined |
http://hid.ri.cmu.edu/HidEval/i |
|
|
Mobo |
walk, and slow walk holding a |
|
||
ndex.html |
|
|
||
Database |
ball). The sequences are captured |
|
||
|
|
|
||
|
from 6 synchronized cameras at 30 |
|
|
|
|
frames per second for 11 seconds. |
|
|
|
|
Large Database—The variations |
|
|
|
|
captured are: indoor track, normal |
|
|
|
|
camera, subject moving towards |
|
|
|
|
the left; indoor track, normal |
|
|
|
|
camera, subject moving towards |
|
|
|
|
the right; treadmill, normal |
|
|
|
|
camera; indoor track, oblique |
|
|
|
Southampto |
camera; and outdoor track, normal |
|
|
|
camera. |
|
|
|
|
n Human |
|
|
|
|
|
http://www.gait.ecs.soton.ac.u |
|
|
|
ID at a |
|
|
||
Small Database—The variations |
k/database/ |
|
|
|
Distance |
|
|||
captured are: normal camera, |
|
|
|
|
Database |
|
|
|
|
subject wearing flip-flops and |
|
|
|
|
|
|
|
|
|
|
moving towards the left; normal |
|
|
|
|
camera, subject wearing flip-flops |
|
|
|
|
and moving towards the right; |
|
|
|
|
normal camera, subject wearing a |
|
|
|
|
trench coat; and normal camera, |
|
|
|
|
subject carrying a barrel bag on |
|
|
|
|
shoulder. |
|
|
|
walking sequences. Details of preparation of gait databases can be found in Nixon, Carter, Cunado, Huang, and Stevenage (1999). The various gait databases available in the public domain are listed in Table 6.
Online Handwriting Recognition
Online handwriting is recognizing the handwriting obtained from a digitized pad which provides the trajectory of the pen even when the pen is not in contact with the pad. There are only a few databases only in the public domain.
Gait Recognition
Gait recognition is identifying the person from the way of walking. The walking style is analyzed from captured
CONCLUSION
Biometric databases should thus be prepared with a proper sample for enrollment and for verification as well so that the system accuracy can be increased with the decrease in cost of the system. For the other biometric traits, public databases still need to be created on some well-defined user-accepted policies so that there should be a fair evaluation of the algorithms developed by various researchers. The traits which need to be explored for
45
TEAM LinG
database creation are ear, hand geometry, palm print, keystroke, DNA, thermal imagery, etc.
REFERENCES
Burge. M.. & Burger, W. (2000). Ear biometrics for machine vision. In Proceedings of the International Conference on Pattern Recognition (pp. 826-830).
Campbell, J. P., & Reynolds, D. A. (1999). Corpora for the evaluation of speaker recognition systems. In Proceedings of the IEEE International Conference on Acoustics, Speech and Signal Processing (Vol. 2, pp. 829-832).
Daugman, J. (1998). Recognizing persons by their iris patterns. In A. Jain, R. Bolle, & S. Pankati (Eds.), Biometric: Personal identification in networked society (pp. 103-121). Amsterdam: Kluwer.
Federal Bureau of Investigation. (1984). The science of fingerprints: Classification and uses. Washington, D.C.: U.S. Government Printing Office.
Hong, L., Wan, Y., & Jain, A. K. (1998). Fingerprint image enhancement: Algorithms and performance evaluation. In
Proceedings of the IEEE Computer Society Workshop on Empirical Evaluation Techniques in Computer Vision
(pp. 117-134).
Maltoni, D., Maio, D., Jain, A. K., & Prabhakar, S. (2003). Handbook of fingerprint recognition. Springer.
Moghaddam, B., & Pentland, A. (1999). Bayesian image retrieval in biometric databases. In Proceedings of the IEEE International Conference on Multimedia Computing and Systems (Vol. 2, p. 610).
Monrose, F., & Rubin, A. D. (2000). Keystroke dynamics as a biometric for authentication. Future Generation Computer Systems.
Nixon, M. S., Carter, J. N., Cunado, D., Huang, P. S., & Stevenage, S. V. (1999). Automatic gait recognition. In
Biometrics: Personal identification in networked society (pp. 231-249). Kluwer.
Ratha, N., Chen, S., Karu, K., & Jain, A. K. (1996). A realtime matching system for large fingerprint databases. IEEE Transactions on Pattern Analysis and Machine Intelligence, 18(8), 799-813.
Vatsa, M., Singh, R., & Gupta, P. (2003). Image database for biometrics personal authentication system. In Proceedings of CVPRIP.
Biometric Databases
Wildes, P. R. (1999). Iris recognition: An emerging biometric technology. Proceedings of the IEEE, 85(9), 13481363.
Zhao, W., Chellappa, R., Rosenfeld, A., & Philips, P. J. (2000). Face recognition: A literature survey (UMD Tech. Rep.).
KEY TERMS
Biometric: A physiological or behavioral characteristic used to recognize the claimed identity of any user. The technique used for measuring the characteristic and comparing it is known as biometrics.
Biometric Sample: The unprocessed image or physical or behavioral characteristic captured to generate the biometric template.
Biometric Template: This is the mathematical representation of the biometric sample which is finally used for matching. The size of a template varies from 9 bytes for hand geometry to 256 bytes for iris recognition to thousands of bytes for face.
Enrollment: The initial process of collecting biometric data from a user and then storing it in a template for later comparison. There are two types: positive enrollment and negative enrollment.
Failure to Enroll Rate: Failure to enroll rate is the condition which arises if the biometric sample captured is not of proper quality that a template can be generated from it.
False Acceptance Rate: The probability of incorrectly identifying any impostor against a valid user’s biometric template.
False Rejection Rate: The probability of incorrectly rejecting the valid users or failing to verify the legitimate claimed identity of any user.
Verification: Comparing the two biometric templates (enrollment template and verification template) and giving the validity of the claimed identity as to whether both are from the same person or not. This is a one-to-one comparison.
46
TEAM LinG
|
47 |
|
Business Rules in Databases |
|
|
|
B |
|
|
|
|
|
|
|
Antonio Badia
University of Louisville, USA
INTRODUCTION
Though informal, the concept of business rule is very important to the modeling and definition of information systems. Business rules are used to express many different aspects of the representation, manipulation and processing of data (Paton, 1999). However, perhaps due to its informal nature, business rules have been the subject of a limited body of research in academia. There is little agreement on the exact definition of business rule, on how to capture business rules in requirements specification (the most common conceptual models, entityrelationship and UML, have no proviso for capturing business rules), and, if captured at all, on how to express rules in database systems. Usually, business rules are implemented as triggers in relational databases. However, the concept of business rule is more versatile and may require the use of other tools.
In this article, I give an intuitive definition of business rule and discuss several classifications proposed in the literature. I then argue that there is no adequate framework to capture and implement business rules and show some of the issues involved, the options available, and propose a methodology to help clarify the process. This article assumes basic knowledge of the relational data model (Date, 2000).
BACKGROUND
The term business rule is an informal, intuitive one. It is used with different (but overlapping) meanings in different contexts. GUIDE (2000) defined business rule as follows: “A business rule is a statement that defines or constrains some aspect of the business. It is intended to assert business structure or to control or influence the behavior of the business.” Thus, the rule expresses a policy (explicitly stated or implicitly followed) about some aspect of how the organization carries out its tasks. This definition is very open and includes things outside the realm of information systems (e.g., a policy on smoking). I will not deal with this kind of rule here1; instead, I will focus only on business rules that deal with information. Intuitively, a business rule in this sense usually specifies issues concerning some particular data item (e.g., what fact it is supposed
to express, the way it is handled, its relationship to other data items). In any case, the business rule represents information about the real world, and that information makes sense from a business point of view. That is, people are interested in “the set of rules that determine how a business operates” (GUIDE, 2000). Note that while data models give the structure of data, business rules are sometimes used to tell how the data can and should be used. That is, the data model tends to be static, and rules tend to be dynamic.
Business rules are very versatile; they allow expression of many different types of actions to be taken. Because some types of actions are very frequent and make sense in many environments, they are routinely expressed in rules. Among them are:
•enforcement of policies,
•checks of constraints (on data values, on effect of actions), and
•data manipulation.
Note: When used to manipulate data, rules can be used for several purposes: (a) standardizing (transforming the implementation of a domain to a standard, predefined layout); (b) specifying how to identify or delete duplicates (especially for complex domains); (c) specifying how to make complex objects behave when parts are created–deleted–updated; (d) specifying the lifespan of data (rules can help discard old data automatically when certain conditions are met); and (e) consolidating data (putting together data from different sources to create a unified, global, and consistent view of the data).
•time-dependent rules (i.e., rules that capture the lifespan, rate of change, freshness requirements and expiration of data).
Clearly, business rules are a powerful concept that should be used when designing an information system.
Types of Business Rules
Von Halle and Fleming (1989) analyzed business rules in the framework of relational database design. They distinguished three types of rules:
Copyright © 2006, Idea Group Inc., distributing in print or electronic forms without written permission of IGI is prohibited.
TEAM LinG
1.Domain rules: Rules that constrain the values that an attribute may take. Note that domain rules, although seemingly trivial, are extremely important. In particular, domain rules allow
•verification that values for an attribute make “business sense”;
•decision about whether two occurrences of the same value in different attributes denote the same real-world value;
•decision about whether comparison of two values makes sense for joins and other operations. For instance, EMPLOYEE.AGE and PAYROLL.CLERK-NUMBER may both be integer but they are unrelated. However, EMPLOYEE.HIRE-DATEandPAYROLL.DATE- PAID are both dates and can be compared to make sure employees do not receive checks prior to their hire date.
2.Integrity rules: Rules that bind together the existence or nonexistence of values in attributes. This comes down to the normal referential integrity of the relational model and specification of primary keys and foreign keys.
Business Rules in Databases
a.Definition of business terms: The terms appearing on a rule are common terms and business terms. The most important difference between the two is that a business term can be defined using facts and other terms, and the common term can be considered understood. Hence, a business rule captures the business term’s specific meaning of a context.
b.Facts relating terms to each other: Facts are relationships among terms; some of the relationships among terms expressed are (a) being an attribute of, (b) being a subterm of, (c) being an aggregation of, (d) playing the role of, or (e) a having a semantic relationship. Terms may appear in more than one fact; they play a role in each fact.
2.Constraints (also called action assertions):
Constraints are used to express dynamic aspects. Because they impose constraints, they usually involve directives such as “must (not)” or “should (not).” An action assertion has an anchor object (which may be any business rule), of which it is a property. They may express conditions, integrity constraints, or authorizations.
3.Triggering operations: Rules that govern the ef- 3. Derivations: Derivations express procedures to
fects of insert, delete, update operations in different attributes or different entities.
This division is tightly tied to the relational data model, which limits its utility in a general setting. Another, more general way to classify business rules is the following, from GUIDE (2000):
1.Structural assertions: Something relevant to the business exists or is in relation to something else. Usually expressed by terms in a language, they refer to facts (things that are known, or how known things relate to each other). A term is a phrase that references a specific business concept. Business terms are independent of representation; however, the same term may have different meanings in different contexts (e.g., different industries, organizations, line of business). Facts, on the other hand, state relationships between concepts or attributes (properties) of a concept (attribute facts), specify that an object falls within the scope of another concept (generalization facts), or describe an interaction between concepts (participation facts). Facts should be built from the available terms. There are two main types of structural assertions:
compute or infer new facts from known facts. They can express either a mathematical calculation or a logical derivation.
There are other classifications of business rules (Ross, 1994), but the ones shown give a good idea of the general flavor of such classifications.
Business Rules and Data Models
There are two main problems in capturing and expressing business rules in database systems. First, most conceptual models do not have means to capture many of them. Second, even if they are somehow captured and given to a database designer, most data models are simply not able to handle business rules. Both problems are discussed in the following sections. As a background, I discuss how data models could represent the information in business rules. Focusing on the relational model, it is clear that domain constraints and referential integrity constraints can be expressed in the model. However, other rules (expressing actions, etc.) are highly problematic. The tools that the relational model offers for the task are checks, assertions, and triggers.
48
TEAM LinG

Business Rules in Databases
Checks are constraints over a single attribute on a table. Checks are written by giving a condition, which is similar to a condition in a WHERE clause of an SQL query. As such, the mechanism is very powerful, as the condition can contain subqueries and therefore mention other attributes in the same or different tables. However, not all systems fully implement the CHECK mechanism; many limit the condition to refer only to the table in which the attribute resides. Checks are normally used in the context of domains (Melton and Simon, 2002); in this context, a check is used to constrain the values of an attribute. For instance,
CREATE DOMAIN age int CHECK (Value >= 18 and Value <= 65)
creates a domain named age, expressed as an integer; only values between 18 and 65 are allowed. Checks can be used inside a CREATE TABLE statement, too. Checks are tested by the system when a tuple is inserted into a table (if the check was given within a CREATE TABLE statement, or if the check was given within a CREATE DOMAIN and the table schema uses the domain), or when a tuple is updated in the table. If the condition in the check is not satisfied, the insertion or update is rejected. However, it is very important to realize that when check conditions involve attributes in other tables, changes to those attributes do not make the system test the check; therefore, such checks can be violated. For instance, the following declaration
CREATE TABLE dept ( Dno int,
Mgrname VARCHAR(50),
Check (Mgrname NOT IN (Select name from employee where salary < 50,000))
would block insertions into table dept (or updates of existing tuples) if someone introduced in the tuple a manager that made less than 50,000. However, if someone updated the table emp to change a manager’s salary to less than 50,000, the update would not be rejected.
Assertions are constraints associated with a table as a whole or with two or more tables. Like checks, assertions use conditions similar to conditions in WHERE clauses; but the condition may now refer to several existing tables in the database. Note that, unlike checks, which are associated with domains or attributes in tables, assertions exist at the database level and are independent of any table. Assertions are checked by the system whenever there is an insertion or update in any of the tables mentioned in the condition. Any transaction must keep the assertion true; otherwise, it is rejected. This makes assertions quite powerful; unfortunately, assertions are
not fully or partially supported by many commercial systems. B
Triggers are expressions of the form E-C-A, where E is called an event, C is called a condition, and A is called an action. An event is an occurrence of a situation. An event is instantaneous and atomic (i.e., it cannot be further subdivided, and it happens at once or does not happen at all). The typical events considered by active rules are primitive for database state changes (e.g., an insert, delete, or update). A condition is a database predicate; the result of such a condition is a Boolean (TRUE/FALSE). Action is a data manipulation program, including insertions, deletions, updates, transactional commands, or external procedures. In order to allow the action to process data according to the event, most systems use the variables OLD and NEW to refer to data in the database before and after the event takes place. If a row is updated, both OLD and NEW variables have values. If a row is inserted, then only the NEW variable has a value; if a row is deleted, then only the OLD variable has a value.
As a final note, it is necessary to point out that many database developers avoid the use of triggers, if at all possible, for two main reasons: (a) most trigger systems add considerable overhead, and (b) triggers bring in issues of complexity usually associated with programming (i.e., it is hard to ensure that the right semantics are being implemented). However, triggers are very powerful tools and sometimes have no substitute to express business logic (Widom & Ceri, 1996).
CAPTURING BUSINESS RULES
Eliciting and Representing Business
Rules in Conceptual Models
Because business rules sometimes represent an implicit type of knowledge (Krogh, 2000), elicitation of business rules share problems and challenges with knowledge elicitation in general. In particular, complex managerial and organizational issues must be addressed; at the minimum, a managerial action must be taken that encourages the sharing of knowledge by human agents. I will not address elicitation issues here, as they are outside the scope of this article.
Clearly, business rules should be captured in the requirement specification phase of a software project. It is during this phase that real-world information is analyzed and modeled and the scope of the system decided (Davis, 1993). However, there is no single technique that will guarantee capturing all business rules that an organization may need. Most conceptual
49
TEAM LinG