
- •Preface
- •Introduction
- •1.1 Spatial coordinate systems
- •1.2 Sound fields and their physical characteristics
- •1.2.1 Free-field and sound waves generated by simple sound sources
- •1.2.2 Reflections from boundaries
- •1.2.3 Directivity of sound source radiation
- •1.2.4 Statistical analysis of acoustics in an enclosed space
- •1.2.5 Principle of sound receivers
- •1.3 Auditory system and perception
- •1.3.1 Auditory system and its functions
- •1.3.2 Hearing threshold and loudness
- •1.3.3 Masking
- •1.3.4 Critical band and auditory filter
- •1.4 Artificial head models and binaural signals
- •1.4.1 Artificial head models
- •1.4.2 Binaural signals and head-related transfer functions
- •1.5 Outline of spatial hearing
- •1.6 Localization cues for a single sound source
- •1.6.1 Interaural time difference
- •1.6.2 Interaural level difference
- •1.6.3 Cone of confusion and head movement
- •1.6.4 Spectral cues
- •1.6.5 Discussion on directional localization cues
- •1.6.6 Auditory distance perception
- •1.7 Summing localization and spatial hearing with multiple sources
- •1.7.1 Summing localization with two sound sources
- •1.7.2 The precedence effect
- •1.7.3 Spatial auditory perceptions with partially correlated and uncorrelated source signals
- •1.7.4 Auditory scene analysis and spatial hearing
- •1.7.5 Cocktail party effect
- •1.8 Room reflections and auditory spatial impression
- •1.8.1 Auditory spatial impression
- •1.8.2 Sound field-related measures and auditory spatial impression
- •1.8.3 Binaural-related measures and auditory spatial impression
- •1.9.1 Basic principle of spatial sound
- •1.9.2 Classification of spatial sound
- •1.9.3 Developments and applications of spatial sound
- •1.10 Summary
- •2.1 Basic principle of a two-channel stereophonic sound
- •2.1.1 Interchannel level difference and summing localization equation
- •2.1.2 Effect of frequency
- •2.1.3 Effect of interchannel phase difference
- •2.1.4 Virtual source created by interchannel time difference
- •2.1.5 Limitation of two-channel stereophonic sound
- •2.2.1 XY microphone pair
- •2.2.2 MS transformation and the MS microphone pair
- •2.2.3 Spaced microphone technique
- •2.2.4 Near-coincident microphone technique
- •2.2.5 Spot microphone and pan-pot technique
- •2.2.6 Discussion on microphone and signal simulation techniques for two-channel stereophonic sound
- •2.3 Upmixing and downmixing between two-channel stereophonic and mono signals
- •2.4 Two-channel stereophonic reproduction
- •2.4.1 Standard loudspeaker configuration of two-channel stereophonic sound
- •2.4.2 Influence of front-back deviation of the head
- •2.5 Summary
- •3.1 Physical and psychoacoustic principles of multichannel surround sound
- •3.2 Summing localization in multichannel horizontal surround sound
- •3.2.1 Summing localization equations for multiple horizontal loudspeakers
- •3.2.2 Analysis of the velocity and energy localization vectors of the superposed sound field
- •3.2.3 Discussion on horizontal summing localization equations
- •3.3 Multiple loudspeakers with partly correlated and low-correlated signals
- •3.4 Summary
- •4.1 Discrete quadraphone
- •4.1.1 Outline of the quadraphone
- •4.1.2 Discrete quadraphone with pair-wise amplitude panning
- •4.1.3 Discrete quadraphone with the first-order sound field signal mixing
- •4.1.4 Some discussions on discrete quadraphones
- •4.2 Other horizontal surround sounds with regular loudspeaker configurations
- •4.2.1 Six-channel reproduction with pair-wise amplitude panning
- •4.2.2 The first-order sound field signal mixing and reproduction with M ≥ 3 loudspeakers
- •4.3 Transformation of horizontal sound field signals and Ambisonics
- •4.3.1 Transformation of the first-order horizontal sound field signals
- •4.3.2 The first-order horizontal Ambisonics
- •4.3.3 The higher-order horizontal Ambisonics
- •4.3.4 Discussion and implementation of the horizontal Ambisonics
- •4.4 Summary
- •5.1 Outline of surround sounds with accompanying picture and general uses
- •5.2 5.1-Channel surround sound and its signal mixing analysis
- •5.2.1 Outline of 5.1-channel surround sound
- •5.2.2 Pair-wise amplitude panning for 5.1-channel surround sound
- •5.2.3 Global Ambisonic-like signal mixing for 5.1-channel sound
- •5.2.4 Optimization of three frontal loudspeaker signals and local Ambisonic-like signal mixing
- •5.2.5 Time panning for 5.1-channel surround sound
- •5.3 Other multichannel horizontal surround sounds
- •5.4 Low-frequency effect channel
- •5.5 Summary
- •6.1 Summing localization in multichannel spatial surround sound
- •6.1.1 Summing localization equations for spatial multiple loudspeaker configurations
- •6.1.2 Velocity and energy localization vector analysis for multichannel spatial surround sound
- •6.1.3 Discussion on spatial summing localization equations
- •6.1.4 Relationship with the horizontal summing localization equations
- •6.2 Signal mixing methods for a pair of vertical loudspeakers in the median and sagittal plane
- •6.3 Vector base amplitude panning
- •6.4 Spatial Ambisonic signal mixing and reproduction
- •6.4.1 Principle of spatial Ambisonics
- •6.4.2 Some examples of the first-order spatial Ambisonics
- •6.4.4 Recreating a top virtual source with a horizontal loudspeaker arrangement and Ambisonic signal mixing
- •6.5 Advanced multichannel spatial surround sounds and problems
- •6.5.1 Some advanced multichannel spatial surround sound techniques and systems
- •6.5.2 Object-based spatial sound
- •6.5.3 Some problems related to multichannel spatial surround sound
- •6.6 Summary
- •7.1 Basic considerations on the microphone and signal simulation techniques for multichannel sounds
- •7.2 Microphone techniques for 5.1-channel sound recording
- •7.2.1 Outline of microphone techniques for 5.1-channel sound recording
- •7.2.2 Main microphone techniques for 5.1-channel sound recording
- •7.2.3 Microphone techniques for the recording of three frontal channels
- •7.2.4 Microphone techniques for ambience recording and combination with frontal localization information recording
- •7.2.5 Stereophonic plus center channel recording
- •7.3 Microphone techniques for other multichannel sounds
- •7.3.1 Microphone techniques for other discrete multichannel sounds
- •7.3.2 Microphone techniques for Ambisonic recording
- •7.4 Simulation of localization signals for multichannel sounds
- •7.4.1 Methods of the simulation of directional localization signals
- •7.4.2 Simulation of virtual source distance and extension
- •7.4.3 Simulation of a moving virtual source
- •7.5 Simulation of reflections for stereophonic and multichannel sounds
- •7.5.1 Delay algorithms and discrete reflection simulation
- •7.5.2 IIR filter algorithm of late reverberation
- •7.5.3 FIR, hybrid FIR, and recursive filter algorithms of late reverberation
- •7.5.4 Algorithms of audio signal decorrelation
- •7.5.5 Simulation of room reflections based on physical measurement and calculation
- •7.6 Directional audio coding and multichannel sound signal synthesis
- •7.7 Summary
- •8.1 Matrix surround sound
- •8.1.1 Matrix quadraphone
- •8.1.2 Dolby Surround system
- •8.1.3 Dolby Pro-Logic decoding technique
- •8.1.4 Some developments on matrix surround sound and logic decoding techniques
- •8.2 Downmixing of multichannel sound signals
- •8.3 Upmixing of multichannel sound signals
- •8.3.1 Some considerations in upmixing
- •8.3.2 Simple upmixing methods for front-channel signals
- •8.3.3 Simple methods for Ambient component separation
- •8.3.4 Model and statistical characteristics of two-channel stereophonic signals
- •8.3.5 A scale-signal-based algorithm for upmixing
- •8.3.6 Upmixing algorithm based on principal component analysis
- •8.3.7 Algorithm based on the least mean square error for upmixing
- •8.3.8 Adaptive normalized algorithm based on the least mean square for upmixing
- •8.3.9 Some advanced upmixing algorithms
- •8.4 Summary
- •9.1 Each order approximation of ideal reproduction and Ambisonics
- •9.1.1 Each order approximation of ideal horizontal reproduction
- •9.1.2 Each order approximation of ideal three-dimensional reproduction
- •9.2 General formulation of multichannel sound field reconstruction
- •9.2.1 General formulation of multichannel sound field reconstruction in the spatial domain
- •9.2.2 Formulation of spatial-spectral domain analysis of circular secondary source array
- •9.2.3 Formulation of spatial-spectral domain analysis for a secondary source array on spherical surface
- •9.3 Spatial-spectral domain analysis and driving signals of Ambisonics
- •9.3.1 Reconstructed sound field of horizontal Ambisonics
- •9.3.2 Reconstructed sound field of spatial Ambisonics
- •9.3.3 Mixed-order Ambisonics
- •9.3.4 Near-field compensated higher-order Ambisonics
- •9.3.5 Ambisonic encoding of complex source information
- •9.3.6 Some special applications of spatial-spectral domain analysis of Ambisonics
- •9.4 Some problems related to Ambisonics
- •9.4.1 Secondary source array and stability of Ambisonics
- •9.4.2 Spatial transformation of Ambisonic sound field
- •9.5 Error analysis of Ambisonic-reconstructed sound field
- •9.5.1 Integral error of Ambisonic-reconstructed wavefront
- •9.5.2 Discrete secondary source array and spatial-spectral aliasing error in Ambisonics
- •9.6 Multichannel reconstructed sound field analysis in the spatial domain
- •9.6.1 Basic method for analysis in the spatial domain
- •9.6.2 Minimizing error in reconstructed sound field and summing localization equation
- •9.6.3 Multiple receiver position matching method and its relation to the mode-matching method
- •9.7 Listening room reflection compensation in multichannel sound reproduction
- •9.8 Microphone array for multichannel sound field signal recording
- •9.8.1 Circular microphone array for horizontal Ambisonic recording
- •9.8.2 Spherical microphone array for spatial Ambisonic recording
- •9.8.3 Discussion on microphone array recording
- •9.9 Summary
- •10.1 Basic principle and implementation of wave field synthesis
- •10.1.1 Kirchhoff–Helmholtz boundary integral and WFS
- •10.1.2 Simplification of the types of secondary sources
- •10.1.3 WFS in a horizontal plane with a linear array of secondary sources
- •10.1.4 Finite secondary source array and effect of spatial truncation
- •10.1.5 Discrete secondary source array and spatial aliasing
- •10.1.6 Some issues and related problems on WFS implementation
- •10.2 General theory of WFS
- •10.2.1 Green’s function of Helmholtz equation
- •10.2.2 General theory of three-dimensional WFS
- •10.2.3 General theory of two-dimensional WFS
- •10.2.4 Focused source in WFS
- •10.3 Analysis of WFS in the spatial-spectral domain
- •10.3.1 General formulation and analysis of WFS in the spatial-spectral domain
- •10.3.2 Analysis of the spatial aliasing in WFS
- •10.3.3 Spatial-spectral division method of WFS
- •10.4 Further discussion on sound field reconstruction
- •10.4.1 Comparison among various methods of sound field reconstruction
- •10.4.2 Further analysis of the relationship between acoustical holography and sound field reconstruction
- •10.4.3 Further analysis of the relationship between acoustical holography and Ambisonics
- •10.4.4 Comparison between WFS and Ambisonics
- •10.5 Equalization of WFS under nonideal conditions
- •10.6 Summary
- •11.1 Basic principles of binaural reproduction and virtual auditory display
- •11.1.1 Binaural recording and reproduction
- •11.1.2 Virtual auditory display
- •11.2 Acquisition of HRTFs
- •11.2.1 HRTF measurement
- •11.2.2 HRTF calculation
- •11.2.3 HRTF customization
- •11.3 Basic physical features of HRTFs
- •11.3.1 Time-domain features of far-field HRIRs
- •11.3.2 Frequency domain features of far-field HRTFs
- •11.3.3 Features of near-field HRTFs
- •11.4 HRTF-based filters for binaural synthesis
- •11.5 Spatial interpolation and decomposition of HRTFs
- •11.5.1 Directional interpolation of HRTFs
- •11.5.2 Spatial basis function decomposition and spatial sampling theorem of HRTFs
- •11.5.3 HRTF spatial interpolation and signal mixing for multichannel sound
- •11.5.4 Spectral shape basis function decomposition of HRTFs
- •11.6 Simplification of signal processing for binaural synthesis
- •11.6.1 Virtual loudspeaker-based algorithms
- •11.6.2 Basis function decomposition-based algorithms
- •11.7.1 Principle of headphone equalization
- •11.7.2 Some problems with binaural reproduction and VAD
- •11.8 Binaural reproduction through loudspeakers
- •11.8.1 Basic principle of binaural reproduction through loudspeakers
- •11.8.2 Virtual source distribution in two-front loudspeaker reproduction
- •11.8.3 Head movement and stability of virtual sources in Transaural reproduction
- •11.8.4 Timbre coloration and equalization in transaural reproduction
- •11.9 Virtual reproduction of stereophonic and multichannel surround sound
- •11.9.1 Binaural reproduction of stereophonic and multichannel sound through headphones
- •11.9.2 Stereophonic expansion and enhancement
- •11.9.3 Virtual reproduction of multichannel sound through loudspeakers
- •11.10.1 Binaural room modeling
- •11.10.2 Dynamic virtual auditory environments system
- •11.11 Summary
- •12.1 Physical analysis of binaural pressures in summing virtual source and auditory events
- •12.1.1 Evaluation of binaural pressures and localization cues
- •12.1.2 Method for summing localization analysis
- •12.1.3 Binaural pressure analysis of stereophonic and multichannel sound with amplitude panning
- •12.1.4 Analysis of summing localization with interchannel time difference
- •12.1.5 Analysis of summing localization at the off-central listening position
- •12.1.6 Analysis of interchannel correlation and spatial auditory sensations
- •12.2 Binaural auditory models and analysis of spatial sound reproduction
- •12.2.1 Analysis of lateral localization by using auditory models
- •12.2.2 Analysis of front-back and vertical localization by using a binaural auditory model
- •12.2.3 Binaural loudness models and analysis of the timbre of spatial sound reproduction
- •12.3 Binaural measurement system for assessing spatial sound reproduction
- •12.4 Summary
- •13.1 Analog audio storage and transmission
- •13.1.1 45°/45° Disk recording system
- •13.1.2 Analog magnetic tape audio recorder
- •13.1.3 Analog stereo broadcasting
- •13.2 Basic concepts of digital audio storage and transmission
- •13.3 Quantization noise and shaping
- •13.3.1 Signal-to-quantization noise ratio
- •13.3.2 Quantization noise shaping and 1-Bit DSD coding
- •13.4 Basic principle of digital audio compression and coding
- •13.4.1 Outline of digital audio compression and coding
- •13.4.2 Adaptive differential pulse-code modulation
- •13.4.3 Perceptual audio coding in the time-frequency domain
- •13.4.4 Vector quantization
- •13.4.5 Spatial audio coding
- •13.4.6 Spectral band replication
- •13.4.7 Entropy coding
- •13.4.8 Object-based audio coding
- •13.5 MPEG series of audio coding techniques and standards
- •13.5.1 MPEG-1 audio coding technique
- •13.5.2 MPEG-2 BC audio coding
- •13.5.3 MPEG-2 advanced audio coding
- •13.5.4 MPEG-4 audio coding
- •13.5.5 MPEG parametric coding of multichannel sound and unified speech and audio coding
- •13.5.6 MPEG-H 3D audio
- •13.6 Dolby series of coding techniques
- •13.6.1 Dolby digital coding technique
- •13.6.2 Some advanced Dolby coding techniques
- •13.7 DTS series of coding technique
- •13.8 MLP lossless coding technique
- •13.9 ATRAC technique
- •13.10 Audio video coding standard
- •13.11 Optical disks for audio storage
- •13.11.1 Structure, principle, and classification of optical disks
- •13.11.2 CD family and its audio formats
- •13.11.3 DVD family and its audio formats
- •13.11.4 SACD and its audio formats
- •13.11.5 BD and its audio formats
- •13.12 Digital radio and television broadcasting
- •13.12.1 Outline of digital radio and television broadcasting
- •13.12.2 Eureka-147 digital audio broadcasting
- •13.12.3 Digital radio mondiale
- •13.12.4 In-band on-channel digital audio broadcasting
- •13.12.5 Audio for digital television
- •13.13 Audio storage and transmission by personal computer
- •13.14 Summary
- •14.1 Outline of acoustic conditions and requirements for spatial sound intended for domestic reproduction
- •14.2 Acoustic consideration and design of listening rooms
- •14.3 Arrangement and characteristics of loudspeakers
- •14.3.1 Arrangement of the main loudspeakers in listening rooms
- •14.3.2 Characteristics of the main loudspeakers
- •14.3.3 Bass management and arrangement of subwoofers
- •14.4 Signal and listening level alignment
- •14.5 Standards and guidance for conditions of spatial sound reproduction
- •14.6 Headphones and binaural monitors of spatial sound reproduction
- •14.7 Acoustic conditions for cinema sound reproduction and monitoring
- •14.8 Summary
- •15.1 Outline of psychoacoustic and subjective assessment experiments
- •15.2 Contents and attributes for spatial sound assessment
- •15.3 Auditory comparison and discrimination experiment
- •15.3.1 Paradigms of auditory comparison and discrimination experiment
- •15.3.2 Examples of auditory comparison and discrimination experiment
- •15.4 Subjective assessment of small impairments in spatial sound systems
- •15.5 Subjective assessment of a spatial sound system with intermediate quality
- •15.6 Virtual source localization experiment
- •15.6.1 Basic methods for virtual source localization experiments
- •15.6.2 Preliminary analysis of the results of virtual source localization experiments
- •15.6.3 Some results of virtual source localization experiments
- •15.7 Summary
- •16.1.1 Application to commercial cinema and related problems
- •16.1.2 Applications to domestic reproduction and related problems
- •16.1.3 Applications to automobile audio
- •16.2.1 Applications to virtual reality
- •16.2.2 Applications to communication and information systems
- •16.2.3 Applications to multimedia
- •16.2.4 Applications to mobile and handheld devices
- •16.3 Applications to the scientific experiments of spatial hearing and psychoacoustics
- •16.4 Applications to sound field auralization
- •16.4.1 Auralization in room acoustics
- •16.4.2 Other applications of auralization technique
- •16.5 Applications to clinical medicine
- •16.6 Summary
- •References
- •Index

Storage and transmission of spatial sound signals 615
13.4.3 Perceptual audio coding in the time-frequency domain
Perceptual audio coding utilizes the redundancy in the perceptual domain to compress audio signals and is a lossy coding method (Brandenburg and Bosi, 1997). The masking effect in Section 1.3.3 is the psychoacoustic basis for perceptual audio coding. Section 13.3.1 indicates that mapping the continuous amplitude of a signal to finite discreate values leads to quantization noise. In the absence of noise shaping, signal-to-quantization-noise ratio increases as the quantization bit increases. A larger quantization bit is needed to obtain high signal-to-quantization-noise ratio. However, quantization noise is not always audible because of the masking effect. If the level of quantization noise is below the threshold of a masking curve (pattern), the quantization noise is inaudible. If the level of a signal is below the hearing threshold or below the threshold of the masking curve, the signal is also inaudible. The above psychoacoustic principles are applicable to perceptual audio coding.
Because masking is related to the time-frequency resolution of human hearing, time domain signals should be transformed to time-frequency domain signals prior to perceptual coding. Transformation has two types. They are similar in nature but different in time-frequency resolution. In practical coding, appropriate transformations and related parameters should be chosen so that time-frequency resolution meets the requirement of auditory perception.
The first type of transformation uses an analysis filter bands to decompose time domain signals into subband components. The bandwidth of subband filters can be uniform or nonuniform. Filters with a uniform bandwidth are relatively simple, but filters with a nonuniform bandwidth can be adapted to the frequency resolution of human auditory. Generally, subband filters exhibit higher time resolution and lower frequency resolution. According to Shannon–Nyquist temporal sampling theorem (Oppenheim et al., 1999), each subband component can be downconverted into a baseband and then subsampled at a sampling frequency not less than twice the bandwidth of the subband.The original signal can be restored by first upsampling and upconverting the baseband representation of each subband component and combining the components of all subbands by using a synthesis filter bank. Ideally, analysis filters should have abrupt transition characteristics between the passband and the stopband to avoid the frequency domain overlap in the restored signal. In addition, the analysis filters should have linear-phase characteristics. Filters with such kind of characteristics are difficult to be implemented. In practice, the bandwidth of signals is often divided into K uniform subbands, where K is the power of 2. Then, analysis filtering is implemented by K quadrature mirror filters (QMF). Although the outputs of quadrature mirror filters overlap at the boundaries between the subbands, overlapping components are cancelled in synthesis filtering, and the original signal is restored. A polyphase quadrature mirror filter (PQMF) band is often used in practical subband coding. For MPEG-1 Layer I and Layer II coding in Section 13.5.1, a PQMF bands is used to divide the time domain input into 32 uniform subband components. An analysis filter bands with a critical bandwidth is used for MPEG-1 Layer III coding.
In the second type of transformation, the discrete time samples of the input signal are divided into block or frames with an appropriate length, and short-term discrete orthogonal transform is used to convert each block of time samples into spectral coefficients in the transform domain (such as in the frequency domain or more strictly in the time-frequency domain). Generally, various short-term discrete orthogonal transforms exhibit higher-frequency resolution and lower time resolution. A well-known short-term discrete orthogonal transform is the short-term Fourier transform (STFT) in Equation (8.3.15). However, modified discrete cosine transform (MDCT) is often used in spatial sound signal coding, such as Dolby Digital coding described in Section 13.6.1. The advantage of MDCT is that the power of a signal is dominated by the preceding spectral components, which are beneficial to signal compression. In addition to STFT and MDCT, other short-term discrete orthogonaltransforms, which yield the coefficients in the transform domain, are applicable to audio coding.
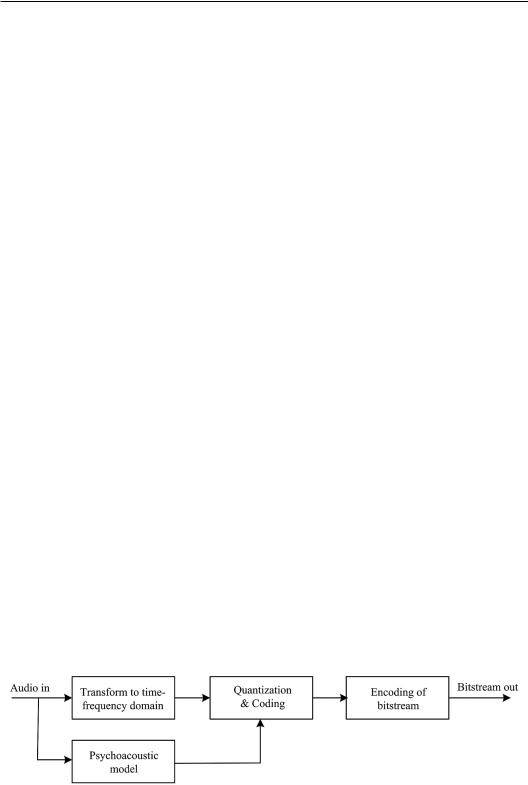
616 Spatial Sound
Similar to the case in Section 8.3, the discrete signal in the time domain is denoted by ex(n), and n is the discrete time. After subband filtering or short-term discrete orthogonal transform, the subband components or coefficients of the transform are denoted by Ex(n′, k). For subband filtering, n′ is the variable of discrete time, and k is the index of the subband, e.g., Ex(n′, k) is the sample of the signal at the kth subband and time n′. For short-term discrete orthogonal transform, n′ is the variable of time (block or frame), k is the variable in the transformed domain (such as frequency domain), and Ex(n′, k) is the coefficient of transform at time (block or frame) n′.
An N-point (even number) MDCT-modified discrete cosine transform can be written as follows by using the above notation (Bosi et al., 1997):
|
NH |
|
2π |
|
1 |
|
|
|
|
|
|||||
Ex (n′, k) = |
2 ∑ex (n′ + n)cos |
|
(n + n′ + n0 ) k + |
2 |
|
|
|
N |
|
||||||
|
n=NL |
|
|
|
(13.4.6) |
||
|
|
|
|
|
|
||
n0 = |
N / 2 + 1 |
k = 0, 1 … (N/2 − 1), |
|
|
|
||
|
2 |
|
|
|
|
|
|
where NL ≤ 0 and NH > 0 are the initial and end times for MDCT calculation, and N = NH − NL + 1 is the length of the block or frame for MDCT calculation. An N-point MDCT satisfies the following odd symmetry:
Ex (n′, k) = −Ex (n′, N − 1 − k). |
(13.4.7) |
Therefore, a signal can be completely described by the preceding N/2 MDCT coefficients with k = 0, 1… (N/2 − 1). An N-point inverse MDCT is given as
|
2 |
N /2−1 |
|
2π |
|
1 |
|
|
ex (n′ + n) = |
|
∑Ex (n′, k)cos |
|
(n + n′ + n0 ) k + |
2 |
|
|
|
N |
N |
|
||||||
|
k=0 |
|
|
|
(13.4.8) |
|||
n = NL, NL + 1,.…NH. |
|
|
|
|
|
|||
|
|
|
|
|
|
Figure 13.14 presents the block diagram of the principle of perceptual audio coding. It involves the following stages.
1.The discrete time input signal is converted into the time-frequency domain by an analysis filter band or short-term discrete orthogonal transform, yielding subband components or spectral coefficients Ex(n′, k).
2.The short-term power spectra of input signals within a certain time window (sampling block) are evaluated by converting the input signal to the time-frequency domain (such
Figure 13.14 Block diagram of the principle of perceptual audio coding.

Storage and transmission of spatial sound signals 617
as using STFT) or directly evaluated from the result of stage (1). The resultant power spectra are analyzed by a psychoacoustic model inputted to the time-frequency domain.
3.The subband or spectral components of input signals are quantized and coded. Different
subband components or spectral coefficients Ex(n′, k) are quantized with different bits. Given the available bit rate, the algorithms of dynamic bit allocation are often used. According to the short-term power spectra evaluated in stage (2) and certain psychoacoustic models, available bits are allocated to each subband component or spectral coefficients (set) to optimize the final perceived performance.
4.The quantized data are organized into frames and then assembled into the bit stream.
In addition to the quantized and coded samples E′x(n′, k), bit stream involves some side information, such as bit allocation information for reconstructing a signal in decoding.
A decoder reconstructs the signal from the bit stream of coded signals. Decoding is an inverse course of coding (Figure 13.14). The subband components or spectral coefficients of each frame are extracted from the bit stream from which PCM audio signals are reconstructed.
Quantization, dynamic bit allocation, and coding have various algorithms. The performance of these algorithms, such as computational cost, compression ratio, and perceived effect, varies. Dynamic bit allocation strategies in audio coding have two kinds. In forwardadaptive allocation, allocation is performed in the coder, and information is included in the bit stream. An advantage of forward-adaptive allocation is that the psychoacoustic model is only included in the coder. A revision of the psychoacoustic model does not influence the design of the decoder. A disadvantage is that some bit resources are needed to convey the allocation information to the decoder. In backward-adaptive allocation, bit allocation information in the decoder is derived from the coded audio data, and the transmission of bit allocation information is not needed. Backward-adaptive allocation has a higher transmission efficiency, but it consumes the computational resource of the decoder. In addition, the psychoacoustic model in the decoder cannot be easily improved when it is applied.
Psychoacoustic models simulate the perception of human hearing to sound. They are essential for perceptual audio coding, especially dynamic bit allocation. Various psychoacoustic models with different accuracies and complicities exist. The quantitative analysis and simulation of the masking effects are the cores of various psychoacoustic models related to audio coding. In many cases, SNRQ in each subband (critical band) is calculated from the shortterm power spectral level and given quantization bits for each frame (sampling block) of input signals. The larger the quantization bits are, the larger SNRQ will be. According to the psychoacoustic pattern of masking, the signal-to-masking-ratio SMR in each subband, which is the difference between power spectral level of signal and minimum masking threshold, is calculated. The noise-masking ratio in each subband is determined using the following formula:
NMR = SMR − SNRQ (dB). |
(13.4.9) |
NMR> 0 means that quantization noise is audible. Figure 13.15 illustrates the relationship among NMR, SMR, and SNRQ. For a given the overall bit rate, dynamic bit allocation, which is usually implemented by iterative algorithm, minimizes the overall NMR across all subbands. In addition, signal components with a level lower than the hearing threshold is inaudible. They are not coded or coded with lower bits.
Masking pattern models are needed to calculate SMR. These patterns depend on the components of stimuli (tonal or nontonal components). Many models in audio coding detect tonality in signals (such as local maximum in the spectrum or spectral flatness measure) to
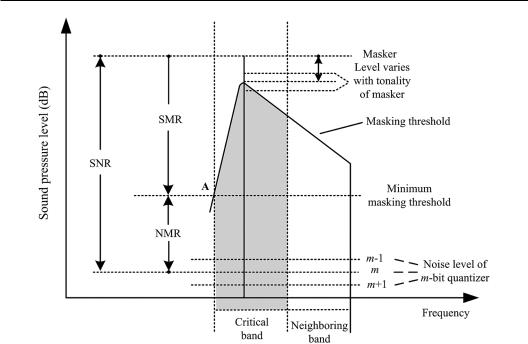
618 Spatial Sound
Figure 13.15 Relationship of NMR, SMR, and SNRQ (Noll, 1997, with the permission of IEEE).
determine the range and amount of masking. In addition, the effect of the critical bandwidth component of a masker is not limited to a single critical band, but it is also distributed to other bands. The spreading function describes masking across several critical bands to simulate the masking response of the entire basilar membrane. The effects of maskers in several critical bands should be considered in the calculation of the overall masking threshold.
13.4.4 Vector quantization
Scalar quantization is discussed in the preceding sections in which each sample (or each differential sample) of the input signal is quantized and coded. Vector quantization (VQ) assembles some scalar data into a set according to a certain rule. Each set can be regarded as a vector and can be quantized jointly in a vector space (Gersho and Gray, 1992; Furui, 2000). VQ maximizes the statistical correlation between the components of the vector and compresses the data with less loss of information. As a lossy coding technique, VQ is widely used in speech coding and sometimes used in audio coding.
If the data of K scalar inputs ex0, ex1…exK constitute a K-dimensional vector ex = [ex0, ex1… exK], and the set {ex} of all K-dimensional vectors constitutes a K-dimensional Euclid space. The Euclid space is divided into L subspaces that do not intersect. Each subspace is approximately represented by a vector eyl. The set {eyl} of L representative vectors eyl (l = 0, 1… L − 1) is termed a code book. The number L of representative vectors in a code book is called the length of a code book. Various divisions of subspaces or choices of the code book constitute different vector quantizers.
For an arbitrary input vector ex, a vector quantizer first determines the subspace in which ex belongs to and then represents ex by a corresponding vector eyl. Therefore, the nature of VQ is to map the arbitrary vector ex in a K-dimensional Euclid space into a finite set {eyl} of L representative vectors.