
- •Preface
- •Part IV. Basic Single Equation Analysis
- •Chapter 18. Basic Regression Analysis
- •Equation Objects
- •Specifying an Equation in EViews
- •Estimating an Equation in EViews
- •Equation Output
- •Working with Equations
- •Estimation Problems
- •References
- •Chapter 19. Additional Regression Tools
- •Special Equation Expressions
- •Robust Standard Errors
- •Weighted Least Squares
- •Nonlinear Least Squares
- •Stepwise Least Squares Regression
- •References
- •Chapter 20. Instrumental Variables and GMM
- •Background
- •Two-stage Least Squares
- •Nonlinear Two-stage Least Squares
- •Limited Information Maximum Likelihood and K-Class Estimation
- •Generalized Method of Moments
- •IV Diagnostics and Tests
- •References
- •Chapter 21. Time Series Regression
- •Serial Correlation Theory
- •Testing for Serial Correlation
- •Estimating AR Models
- •ARIMA Theory
- •Estimating ARIMA Models
- •ARMA Equation Diagnostics
- •References
- •Chapter 22. Forecasting from an Equation
- •Forecasting from Equations in EViews
- •An Illustration
- •Forecast Basics
- •Forecasts with Lagged Dependent Variables
- •Forecasting with ARMA Errors
- •Forecasting from Equations with Expressions
- •Forecasting with Nonlinear and PDL Specifications
- •References
- •Chapter 23. Specification and Diagnostic Tests
- •Background
- •Coefficient Diagnostics
- •Residual Diagnostics
- •Stability Diagnostics
- •Applications
- •References
- •Part V. Advanced Single Equation Analysis
- •Chapter 24. ARCH and GARCH Estimation
- •Basic ARCH Specifications
- •Estimating ARCH Models in EViews
- •Working with ARCH Models
- •Additional ARCH Models
- •Examples
- •References
- •Chapter 25. Cointegrating Regression
- •Background
- •Estimating a Cointegrating Regression
- •Testing for Cointegration
- •Working with an Equation
- •References
- •Binary Dependent Variable Models
- •Ordered Dependent Variable Models
- •Censored Regression Models
- •Truncated Regression Models
- •Count Models
- •Technical Notes
- •References
- •Chapter 27. Generalized Linear Models
- •Overview
- •How to Estimate a GLM in EViews
- •Examples
- •Working with a GLM Equation
- •Technical Details
- •References
- •Chapter 28. Quantile Regression
- •Estimating Quantile Regression in EViews
- •Views and Procedures
- •Background
- •References
- •Chapter 29. The Log Likelihood (LogL) Object
- •Overview
- •Specification
- •Estimation
- •LogL Views
- •LogL Procs
- •Troubleshooting
- •Limitations
- •Examples
- •References
- •Part VI. Advanced Univariate Analysis
- •Chapter 30. Univariate Time Series Analysis
- •Unit Root Testing
- •Panel Unit Root Test
- •Variance Ratio Test
- •BDS Independence Test
- •References
- •Part VII. Multiple Equation Analysis
- •Chapter 31. System Estimation
- •Background
- •System Estimation Methods
- •How to Create and Specify a System
- •Working With Systems
- •Technical Discussion
- •References
- •Vector Autoregressions (VARs)
- •Estimating a VAR in EViews
- •VAR Estimation Output
- •Views and Procs of a VAR
- •Structural (Identified) VARs
- •Vector Error Correction (VEC) Models
- •A Note on Version Compatibility
- •References
- •Chapter 33. State Space Models and the Kalman Filter
- •Background
- •Specifying a State Space Model in EViews
- •Working with the State Space
- •Converting from Version 3 Sspace
- •Technical Discussion
- •References
- •Chapter 34. Models
- •Overview
- •An Example Model
- •Building a Model
- •Working with the Model Structure
- •Specifying Scenarios
- •Using Add Factors
- •Solving the Model
- •Working with the Model Data
- •References
- •Part VIII. Panel and Pooled Data
- •Chapter 35. Pooled Time Series, Cross-Section Data
- •The Pool Workfile
- •The Pool Object
- •Pooled Data
- •Setting up a Pool Workfile
- •Working with Pooled Data
- •Pooled Estimation
- •References
- •Chapter 36. Working with Panel Data
- •Structuring a Panel Workfile
- •Panel Workfile Display
- •Panel Workfile Information
- •Working with Panel Data
- •Basic Panel Analysis
- •References
- •Chapter 37. Panel Estimation
- •Estimating a Panel Equation
- •Panel Estimation Examples
- •Panel Equation Testing
- •Estimation Background
- •References
- •Part IX. Advanced Multivariate Analysis
- •Chapter 38. Cointegration Testing
- •Johansen Cointegration Test
- •Single-Equation Cointegration Tests
- •Panel Cointegration Testing
- •References
- •Chapter 39. Factor Analysis
- •Creating a Factor Object
- •Rotating Factors
- •Estimating Scores
- •Factor Views
- •Factor Procedures
- •Factor Data Members
- •An Example
- •Background
- •References
- •Appendix B. Estimation and Solution Options
- •Setting Estimation Options
- •Optimization Algorithms
- •Nonlinear Equation Solution Methods
- •References
- •Appendix C. Gradients and Derivatives
- •Gradients
- •Derivatives
- •References
- •Appendix D. Information Criteria
- •Definitions
- •Using Information Criteria as a Guide to Model Selection
- •References
- •Appendix E. Long-run Covariance Estimation
- •Technical Discussion
- •Kernel Function Properties
- •References
- •Index
- •Symbols
- •Numerics
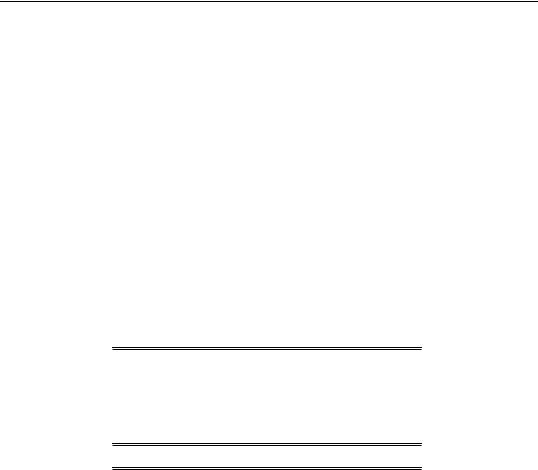
676—Chapter 37. Panel Estimation
The next portion of output provides additional test detail, showing the coefficient estimates from both the random and fixed effects estimators, along with the variance of the difference and associated p-values for the hypothesis that there is no difference. Note that in some cases, the estimated variances can be negative so that the probabilities cannot be computed.
Cross-section random effects test comparisons:
Variable |
Fixed |
Random |
Var(Diff.) |
Prob. |
|
|
|
|
|
|
|
|
|
|
F |
0.110124 |
0.109781 |
0.000031 |
0.9506 |
C01 |
0.310065 |
0.308113 |
0.000006 |
0.4332 |
|
|
|
|
|
|
|
|
|
|
The bottom portion of the output contains the results from the corresponding fixed effects estimation:
Cross-section random effects test equation:
Dependent Variable: I
Method: Panel Least Squares
Date: 08/18/09 Time: 12:51
Sample: 1935 1954
Periods included: 20
Cross-sections included: 10
Total panel (balanced) observations: 200
Variable |
Coefficient |
Std. Error |
t-Statistic |
Prob. |
|
|
|
|
|
|
|
|
|
|
C |
-58.74394 |
12.45369 |
-4.716990 |
0.0000 |
F |
0.110124 |
0.011857 |
9.287901 |
0.0000 |
C01 |
0.310065 |
0.017355 |
17.86656 |
0.0000 |
|
|
|
|
|
|
|
|
|
|
Effects Specification
Cross-section fixed (dummy variables)
R-squared |
0.944073 |
Mean dependent var |
145.9583 |
Adjusted R-squared |
0.940800 |
S.D. dependent var |
216.8753 |
S.E. of regression |
52.76797 |
Akaike info criterion |
10.82781 |
Sum squared resid |
523478.1 |
Schwarz criterion |
11.02571 |
Log likelihood |
-1070.781 |
Hannan-Quinn criter. |
10.90790 |
F-statistic |
288.4996 |
Durbin-Watson stat |
0.716733 |
Prob(F-statistic) |
0.000000 |
|
|
|
|
|
|
In some cases, EViews will automatically drop non-varying variables in order to construct the test statistic. These dropped variables will be indicated in this latter estimation output.
Estimation Background
The basic class of models that can be estimated using panel techniques may be written as:
Yit = f(Xit, b) + di + gt + eit |
(37.1) |

Estimation Background—677
The leading case involves a linear conditional mean specification, so that we have:
|
|
Yit = a + Xit¢b + di + gt + eit |
(37.2) |
where Yit |
is the dependent variable, and Xit is a k -vector of regressors, and eit |
are the |
|
error terms for i = 1, 2, º, M cross-sectional units observed for dated periods |
|
||
t |
= 1, 2, º, T . The a parameter represents the overall constant in the model, while the |
||
di |
and gt |
represent cross-section or period specific effects (random or fixed). |
|
Note that in contrast to the pool specifications described in Equation (35.2) on page 601, EViews panel equations allow you to specify equations in general form, allowing for nonlinear coefficients mean equations with additive effects. Panel equations do not automatically allow for b coefficients that vary across cross-sections or periods, but you may, of course, create interaction variables that permit such variation.
Other than these differences, the pool equation discussion of “Estimation Background” on page 601 applies to the estimation of panel equations. In particular, the calculation of fixed and random effects, GLS weighting, AR estimation, and coefficient covariances for least squares and instrumental variables is equally applicable in the present setting.
Accordingly, the remainder of this discussion will focus on a brief review of the relevant econometric concepts surrounding GMM estimation of panel equations.
GMM Details
The following is a brief review of GMM estimation and dynamic panel estimators. As always, the discussion is merely an overview. For detailed surveys of the literature, see Wooldridge (2002) and Baltagi (2005).
Background
The basic GMM panel estimators are based on moments of the form,
|
M |
M |
|
g(b) = Â gi(b) |
= Â Zi¢ei(b) |
(37.3) |
|
i |
= 1 |
i = 1 |
|
where Zi is a Ti ¥ p matrix of instruments for cross-section i , and, |
|
||
ei(b) |
= (Yi |
– f(Xit, b)) |
(37.4) |
In some cases we will work symmetrically with moments where the summation is taken over periods t instead of i .
GMM estimation minimizes the quadratic form:

678—Chapter 37. Panel Estimation
|
|
M |
¢ |
|
M |
|
|
S(b) = |
|
 Zi¢ei(b) |
H |
 Zi¢ei(b) |
(37.5) |
||
|
i = 1 |
|
i = 1 |
|
|
||
= |
g(b)¢Hg(b) |
|
|
|
|
|
with respect to b for a suitably chosen p ¥ p weighting matrix H .
|
|
|
|
ˆ |
|
|
|
|
|
Given estimates of the coefficient vector, b , an estimate of the coefficient covariance matrix |
|||||||||
is computed as, |
|
|
|
|
|
|
|
|
|
ˆ |
(G¢HG) |
–1 |
(G¢HLHG)(G¢HG) |
–1 |
(37.6) |
||||
V(b) = |
|
|
|
||||||
where L is an estimator of E(gi(b)gi(b)¢) |
= |
E(Zi¢ei(b)ei(b)¢Zi), and G is a Ti ¥ k |
|||||||
derivative matrix given by: |
|
|
|
|
|
|
|
|
|
|
|
|
|
M |
Z |
¢ f |
|
|
(37.7) |
|
G(b)= – |
 |
(b) |
|
|||||
|
|
|
i |
i |
|
|
|
||
|
|
|
i = 1 |
|
|
|
|
|
In the simple linear case where f(Xit, b) = Xit¢b , we may write the coefficient estimator in closed form as,
ˆ |
M |
Z |
¢ |
|
M |
|
Z |
|
|
–1 M |
Z |
¢ |
M |
Z |
|
|
||||||
b = |
 |
¢X H |
|
 |
|
|
¢X |
|
 |
¢X |
H |
 |
¢Y |
|||||||||
|
|
i |
i |
|
|
|
i |
i |
|
i |
i |
|
i |
|
i |
|||||||
|
|
i = 1 |
|
|
|
i = 1 |
|
|
|
|
|
|
i = 1 |
|
|
|
i = 1 |
|
|
|
||
|
= (MZX¢HMZX)–1(MZX¢HMZY) |
|
|
|
|
|
|
|
|
|
|
|||||||||||
with variance estimator, |
|
|
|
|
|
|
|
|
|
|
|
|
|
|
|
|
|
|
||||
|
ˆ |
|
(MZX¢HMZX) |
–1 |
(MZX |
¢HLHMZX)(MZX¢HMZX) |
–1 |
|||||||||||||||
|
V(b) = |
|
|
|||||||||||||||||||
for MAB of the general form: |
|
|
|
|
|
|
|
|
|
|
|
|
|
|
|
|
|
|
||||
|
|
|
|
M |
|
|
= |
|
|
|
M |
A |
|
|
|
|
|
|
|
|
||
|
|
|
|
AB |
M–1 |
|
 |
¢B |
|
|
|
|
|
|
||||||||
|
|
|
|
|
|
|
|
|
|
|
i |
|
i |
|
|
|
|
|
|
|||
|
|
|
|
|
|
|
|
|
|
|
i = 1 |
|
|
|
|
|
|
|
|
|
|
(37.8)
(37.9)
(37.10)
The basics of GMM estimation involve: (1) specifying the instruments Z , (2) choosing the weighting matrix H , and (3) determining an estimator for L .
It is worth pointing out that the summations here are taken over individuals; we may equivalently write the expressions in terms of summations taken over periods. This symmetry will prove useful in describing some of the GMM specifications that EViews supports.
A wide range of specifications may be viewed as specific cases in the GMM framework. For example, the simple 2SLS estimator, using ordinary estimates of the coefficient covariance, specifies:

Estimation Background—679
|
|
|
|
|
|
ˆ |
2 |
MZZ) |
–1 |
|
|
|
|
|
||
|
|
|
H = (j |
|
|
|
|
|
|
|||||||
|
|
|
|
|
|
ˆ 2 |
MZZ |
|
|
|
|
|
|
|||
|
|
|
L = j |
|
|
|
|
|
|
|||||||
Substituting, we have the familiar expressions, |
|
|
|
|
|
|
||||||||||
ˆ |
ˆ 2 |
MZZ) |
–1 |
MZX) |
–1 |
|
|
ˆ 2 |
MZZ) |
–1 |
MZY) |
|||||
b = (MZX¢(j |
|
|
|
(MZX¢(j |
|
|||||||||||
|
= (MZX¢MZZ–1MZX )–1(MZX¢MZZ–1MZY ) |
|
|
|||||||||||||
and, |
|
|
|
|
|
|
|
|
|
|
|
|
|
|
|
|
|
ˆ |
|
ˆ 2 |
(MZX¢MZZ |
–1 |
MZX) |
–1 |
|
|
|||||||
|
V(b) |
= j |
|
|
|
|
(37.11)
(37.12)
(37.13)
Standard errors that are robust to conditional or unconditional heteroskedasticity and contemporaneous correlation may be computed by substituting a new expression for L ,
|
–1 |
|
T |
|
ˆ |
ˆ |
|
|
|
L = T |
|
 |
Z |
|
¢Z |
(37.14) |
|||
|
¢e |
e |
|||||||
|
|
|
t |
t |
|
t |
t |
|
|
|
|
|
t = 1 |
|
|
|
|
|
|
so that we have a White cross-section robust coefficient covariance estimator. Additional robust covariance methods are described in detail in “Robust Coefficient Covariances” on page 611.
In addition, EViews supports a variety of weighting matrix choices. All of the choices available for covariance calculation are also available for weight calculations in the standard panel GMM setting: 2SLS, White cross-section, White period, White diagonal, Cross-section SUR (3SLS), Cross-section weights, Period SUR, Period weights. An additional differenced error weighting matrix may be employed when estimating a dynamic panel data specification using GMM.
The formulae for these weights are follow immediately from the choices given in “Robust Coefficient Covariances” on page 611. For example, the Cross-section SUR (3SLS) weighting matrix is computed as:
|
|
|
–1 |
T |
|
ˆ |
|
|
–1 |
H |
= |
 |
Z |
|
(37.15) |
||||
T |
|
¢Q |
M |
Z |
|||||
|
|
|
|
t |
|
t |
|
||
|
|
|
|
t = 1 |
|
|
|
|
|
ˆ
where QM is an estimator of the contemporaneous covariance matrix. Similarly, the White period weights are given by:
|
|
–1 |
M |
|
|
|
|
|
–1 |
H = |
 |
Z |
ˆ |
ˆ |
|
(37.16) |
|||
M |
|
¢e |
e |
¢Z |
|||||
|
|
|
i |
i |
|
i |
i |
|
|
|
|
|
i = 1 |
|
|
|
|
|
|
These latter GMM weights are associated with specifications that have arbitrary serial correlation and time-varying variances in the disturbances.

680—Chapter 37. Panel Estimation
GLS Specifications
EViews allows you to estimate a GMM specification on GLS transformed data. Note that the moment conditions are modified to reflect the GLS weighting:
|
|
M |
M |
–1 |
|
|
g(b) |
= |
 gi(b) |
ˆ |
ei(b) |
(37.17) |
|
= Â Zi¢Q |
|
|||||
|
|
i = 1 |
i = 1 |
|
|
|
Dynamic Panel Data |
|
|
|
|
|
|
Consider the linear dynamic panel data specification given by: |
|
|||||
|
|
p |
|
|
|
|
Yit |
= |
 rjYit – j |
+ Xit¢b + di + eit |
(37.18) |
j = 1
First-differencing this specification eliminates the individual effect and produces an equation of the form:
p |
|
DYit = Â rjDYit – j + DXit¢b + Deit |
(37.19) |
j = 1
which may be estimated using GMM techniques.
Efficient GMM estimation of this equation will typically employ a different number of instruments for each period, with the period-specific instruments corresponding to the different numbers of lagged dependent and predetermined variables available at a given period. Thus, along with any strictly exogenous variables, one may use period-specific sets of instruments corresponding to lagged values of the dependent and other predetermined variables.
Consider, for example, the motivation behind the use of the lagged values of the dependent variable as instruments in Equation (37.19). If the innovations in the original equation are i.i.d., then in t = 3 , the first period available for analysis of the specification, it is obvious
that Yi1 |
is a valid instrument since it is correlated with DYi2 , but uncorrelated with Dei3 . |
||||||||||
Similarly, in t = 4 , both Yi2 |
and Yi1 |
are potential instruments. Continuing in this vein, |
|||||||||
we may form a set of predetermined instruments for individual i |
using lags of the depen- |
||||||||||
dent variable: |
|
|
|
|
|
|
|
|
|
||
|
|
|
Yi1 |
0 |
0 |
º |
º |
º |
º |
0 |
|
|
|
|
|
||||||||
Wi |
= |
|
0 |
Yi1 |
Yi2 |
º º º º 0 |
(37.20) |
||||
|
|
|
º |
º |
º |
º |
º |
º |
º |
º |
|
|
|
|
0 |
0 |
0 |
º Yi1 |
Yi2 |
º YiTi – 2 |
|
||
|
|
|
|
|
|
|
|
|
|
|
|
Similar sets of instruments may be formed for each predetermined variables.