
- •Preface
- •Part IV. Basic Single Equation Analysis
- •Chapter 18. Basic Regression Analysis
- •Equation Objects
- •Specifying an Equation in EViews
- •Estimating an Equation in EViews
- •Equation Output
- •Working with Equations
- •Estimation Problems
- •References
- •Chapter 19. Additional Regression Tools
- •Special Equation Expressions
- •Robust Standard Errors
- •Weighted Least Squares
- •Nonlinear Least Squares
- •Stepwise Least Squares Regression
- •References
- •Chapter 20. Instrumental Variables and GMM
- •Background
- •Two-stage Least Squares
- •Nonlinear Two-stage Least Squares
- •Limited Information Maximum Likelihood and K-Class Estimation
- •Generalized Method of Moments
- •IV Diagnostics and Tests
- •References
- •Chapter 21. Time Series Regression
- •Serial Correlation Theory
- •Testing for Serial Correlation
- •Estimating AR Models
- •ARIMA Theory
- •Estimating ARIMA Models
- •ARMA Equation Diagnostics
- •References
- •Chapter 22. Forecasting from an Equation
- •Forecasting from Equations in EViews
- •An Illustration
- •Forecast Basics
- •Forecasts with Lagged Dependent Variables
- •Forecasting with ARMA Errors
- •Forecasting from Equations with Expressions
- •Forecasting with Nonlinear and PDL Specifications
- •References
- •Chapter 23. Specification and Diagnostic Tests
- •Background
- •Coefficient Diagnostics
- •Residual Diagnostics
- •Stability Diagnostics
- •Applications
- •References
- •Part V. Advanced Single Equation Analysis
- •Chapter 24. ARCH and GARCH Estimation
- •Basic ARCH Specifications
- •Estimating ARCH Models in EViews
- •Working with ARCH Models
- •Additional ARCH Models
- •Examples
- •References
- •Chapter 25. Cointegrating Regression
- •Background
- •Estimating a Cointegrating Regression
- •Testing for Cointegration
- •Working with an Equation
- •References
- •Binary Dependent Variable Models
- •Ordered Dependent Variable Models
- •Censored Regression Models
- •Truncated Regression Models
- •Count Models
- •Technical Notes
- •References
- •Chapter 27. Generalized Linear Models
- •Overview
- •How to Estimate a GLM in EViews
- •Examples
- •Working with a GLM Equation
- •Technical Details
- •References
- •Chapter 28. Quantile Regression
- •Estimating Quantile Regression in EViews
- •Views and Procedures
- •Background
- •References
- •Chapter 29. The Log Likelihood (LogL) Object
- •Overview
- •Specification
- •Estimation
- •LogL Views
- •LogL Procs
- •Troubleshooting
- •Limitations
- •Examples
- •References
- •Part VI. Advanced Univariate Analysis
- •Chapter 30. Univariate Time Series Analysis
- •Unit Root Testing
- •Panel Unit Root Test
- •Variance Ratio Test
- •BDS Independence Test
- •References
- •Part VII. Multiple Equation Analysis
- •Chapter 31. System Estimation
- •Background
- •System Estimation Methods
- •How to Create and Specify a System
- •Working With Systems
- •Technical Discussion
- •References
- •Vector Autoregressions (VARs)
- •Estimating a VAR in EViews
- •VAR Estimation Output
- •Views and Procs of a VAR
- •Structural (Identified) VARs
- •Vector Error Correction (VEC) Models
- •A Note on Version Compatibility
- •References
- •Chapter 33. State Space Models and the Kalman Filter
- •Background
- •Specifying a State Space Model in EViews
- •Working with the State Space
- •Converting from Version 3 Sspace
- •Technical Discussion
- •References
- •Chapter 34. Models
- •Overview
- •An Example Model
- •Building a Model
- •Working with the Model Structure
- •Specifying Scenarios
- •Using Add Factors
- •Solving the Model
- •Working with the Model Data
- •References
- •Part VIII. Panel and Pooled Data
- •Chapter 35. Pooled Time Series, Cross-Section Data
- •The Pool Workfile
- •The Pool Object
- •Pooled Data
- •Setting up a Pool Workfile
- •Working with Pooled Data
- •Pooled Estimation
- •References
- •Chapter 36. Working with Panel Data
- •Structuring a Panel Workfile
- •Panel Workfile Display
- •Panel Workfile Information
- •Working with Panel Data
- •Basic Panel Analysis
- •References
- •Chapter 37. Panel Estimation
- •Estimating a Panel Equation
- •Panel Estimation Examples
- •Panel Equation Testing
- •Estimation Background
- •References
- •Part IX. Advanced Multivariate Analysis
- •Chapter 38. Cointegration Testing
- •Johansen Cointegration Test
- •Single-Equation Cointegration Tests
- •Panel Cointegration Testing
- •References
- •Chapter 39. Factor Analysis
- •Creating a Factor Object
- •Rotating Factors
- •Estimating Scores
- •Factor Views
- •Factor Procedures
- •Factor Data Members
- •An Example
- •Background
- •References
- •Appendix B. Estimation and Solution Options
- •Setting Estimation Options
- •Optimization Algorithms
- •Nonlinear Equation Solution Methods
- •References
- •Appendix C. Gradients and Derivatives
- •Gradients
- •Derivatives
- •References
- •Appendix D. Information Criteria
- •Definitions
- •Using Information Criteria as a Guide to Model Selection
- •References
- •Appendix E. Long-run Covariance Estimation
- •Technical Discussion
- •Kernel Function Properties
- •References
- •Index
- •Symbols
- •Numerics
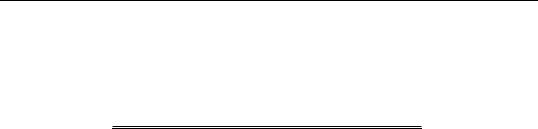
36—Chapter 19. Additional Regression Tools
Dependent Variable: 100*D(LOG(POJ)) Method: Least Squares
Date: 04/14/09 Time: 14:27 Sample: 1950:01 2000:12 Included observations: 612
HAC standard errors & covariance (Bartlett kernel, User bandwidth = 8.0000)
Variable |
Coefficient |
Std. Error |
t-Statistic |
Prob. |
|
|
|
|
|
|
|
|
|
|
FDD |
0.503798 |
0.139563 |
3.609818 |
0.0003 |
FDD(-1) |
0.169918 |
0.088943 |
1.910407 |
0.0566 |
FDD(-2) |
0.067014 |
0.060693 |
1.104158 |
0.2700 |
FDD(-3) |
0.071087 |
0.044894 |
1.583444 |
0.1139 |
FDD(-4) |
0.024776 |
0.031656 |
0.782679 |
0.4341 |
FDD(-5) |
0.031935 |
0.030763 |
1.038086 |
0.2997 |
FDD(-6) |
0.032560 |
0.047602 |
0.684014 |
0.4942 |
FDD(-7) |
0.014913 |
0.015743 |
0.947323 |
0.3439 |
FDD(-8) |
-0.042196 |
0.034885 |
-1.209594 |
0.2269 |
FDD(-9) |
-0.010300 |
0.051452 |
-0.200181 |
0.8414 |
FDD(-10) |
-0.116300 |
0.070656 |
-1.646013 |
0.1003 |
FDD(-11) |
-0.066283 |
0.053014 |
-1.250288 |
0.2117 |
FDD(-12) |
-0.142268 |
0.077424 |
-1.837518 |
0.0666 |
FDD(-13) |
-0.081575 |
0.042992 |
-1.897435 |
0.0583 |
FDD(-14) |
-0.056372 |
0.035300 |
-1.596959 |
0.1108 |
FDD(-15) |
-0.031875 |
0.028018 |
-1.137658 |
0.2557 |
FDD(-16) |
-0.006777 |
0.055701 |
-0.121670 |
0.9032 |
FDD(-17) |
0.001394 |
0.018445 |
0.075584 |
0.9398 |
FDD(-18) |
0.001824 |
0.016973 |
0.107450 |
0.9145 |
C |
-0.340237 |
0.273659 |
-1.243289 |
0.2143 |
|
|
|
|
|
|
|
|
|
|
R-squared |
0.128503 |
Mean dependent var |
-0.115821 |
|
Adjusted R-squared |
0.100532 |
S.D. dependent var |
5.065300 |
|
S.E. of regression |
4.803944 |
Akaike info criterion |
6.008886 |
|
Sum squared resid |
13662.11 |
Schwarz criterion |
6.153223 |
|
Log likelihood |
-1818.719 |
Hannan-Quinn criter. |
6.065023 |
|
F-statistic |
4.594247 |
Durbin-Watson stat |
1.821196 |
|
Prob(F-statistic) |
0.000000 |
|
|
|
|
|
|
|
|
|
|
|
|
|
Note in particular that the top of the equation output shows the use of HAC covariance estimates along with relevant information about the settings used to compute the long-run covariance matrix.
Weighted Least Squares
Suppose that you have heteroskedasticity of known form, where the conditional error variances are given by j2t . The presence of heteroskedasticity does not alter the bias or consistency properties of ordinary least squares estimates, but OLS is no longer efficient and conventional estimates of the coefficient standard errors are not valid.
If the variances j2t are known up to a positive scale factor, you may use weighted least squares (WLS) to obtain efficient estimates that support valid inference. Specifically, if
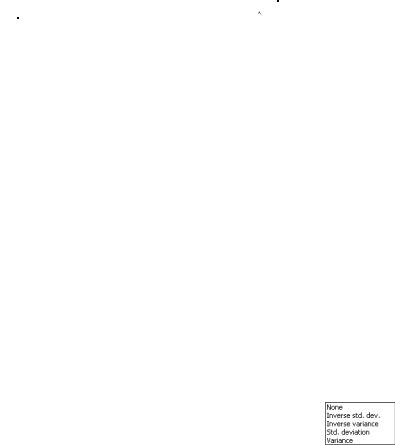
|
|
|
|
|
|
|
Weighted Least Squares—37 |
|
|
|
|
|
|
||
yt = |
|
|
|
xt¢b + et |
|
||
E(et |
|
Xt) = 0 |
(19.12) |
||||
|
|||||||
Var(et |
|
Xt) = jt2 |
|
||||
|
|
||||||
and we observe ht = ajt2 , the WLS estimator for b minimizes the weighted sum-of- |
|||||||
squared residuals: |
|
|
|
|
|
|
|
|
|
1 |
2 |
||||
S(b) = |
Âh |
|
|
||||
|
|
|
|
----(yt – xt¢b) |
|
||
|
t |
|
|
|
|
t |
|
(19.13)
= Âwt(yt – xt¢b)2
t
with respect to the k -dimensional vector of parameters b , where the weights wt = 1 § ht are proportional to the inverse conditional variances. Equivalently, you may estimate the regression of the square-root weighted transformed data yt = wt yt on the transformed xt =
wt xt .
In matrix notation, let W be a diagonal matrix containing the scaled w along the diagonal
and zeroes elsewhere, and let y and X be the matrices associated with yt |
and xt . The |
|||
WLS estimator may be written, |
|
|
|
|
ˆ |
= (X¢WX) |
–1 |
X¢Wy |
(19.14) |
bWLS |
|
and the default estimated coefficient covariance matrix is:
|
|
|
ˆ |
|
= |
s |
2 |
(X¢WX) |
–1 |
(19.15) |
|
|
|
SWLS |
|
|
|||||
where |
|
|
|
|
|
|
|
|
|
|
s |
2 |
= |
1 |
(y |
|
ˆ |
|
|
ˆ |
(19.16) |
|
------------- |
– XbWLS)¢W(y – XbWLS) |
||||||||
|
|
|
T – k |
|
|
|
|
|
|
|
is a d.f. corrected estimator of the weighted residual variance.
To perform WLS in EViews, open the equation estimation dialog and select a method that supports WLS such as LS—Least Squares (NLS and ARMA), then click on the Options tab. (You should note that weighted estimation is not offered in equations containing ARMA specifications, nor is it available for some equation methods, such as those estimated with ARCH, binary, count, censored and truncated, or ordered discrete choice techniques.)
You will use the three parts of the Weights section of the Options tab to specify your weights.
The Type combo is used to specify the form in which the weight data are provided. If, for example, your weight series VARWGT contains values proportional to the conditional variance, you should select Variance.
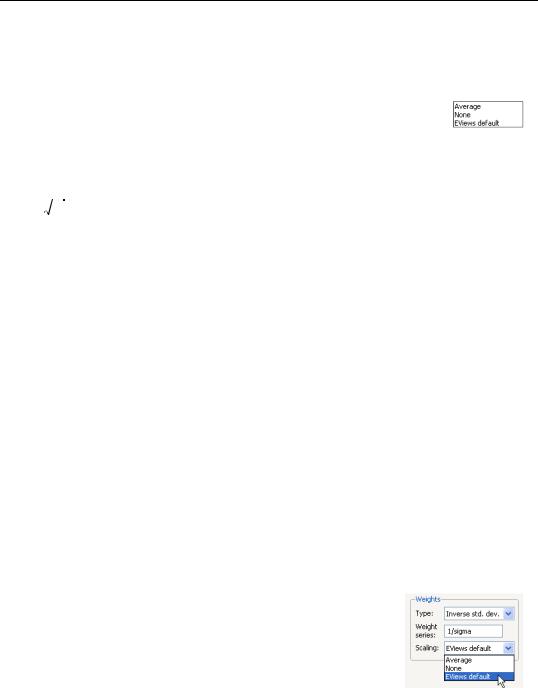
38—Chapter 19. Additional Regression Tools
Alternately, if your series INVARWGT contains the values proportional to the inverse of the standard deviation of the residuals you should choose Inverse std. dev.
Next, you should enter an expression for your weight series in the Weight series edit field.
Lastly, you should choose a scaling method for the weights. There are three choices: Average, None, and (in some cases) EViews default. If you select Average, EViews will, prior to use, scale the weights prior so that
the wi sum to T . The EViews default specification scales the weights so the square roots of the wi sum to T . (The latter square root scaling, which offers backward compatibility to EViews 6 and earlier, was originally introduced in an effort to make the weighted residuals
wt (yt xt¢ˆ ) comparable to the unweighted residuals.) Note that the EViews default b
–
method is only available if you select Inverse std. dev. as weighting Type.
Unless there is good reason to do so, we recommend that you employ Inverse std. dev. weights with EViews default scaling, even if it means you must transform your weight series. The other weight types and scaling methods were introduced in EViews 7, so equations estimated using the alternate settings may not be read by prior versions of EViews.
We emphasize the fact that bWLS and ˆ WLS are almost always invariant to the scaling of
S
weights. One important exception to this invariance occurs in the special case where some of the weight series values are non-positive since observations with non-positive weights will be excluded from the analysis unless you have selected EViews default scaling, in which case only observations with zero weights are excluded.
As an illustration, we consider a simple example taken from Gujarati (2003, Example 11.7, p. 416) which examines the relationship between compensation (Y) and index for employment size (X) for nine nondurable manufacturing industries. The data, which are in the workfile “Gujarati_wls.WF1”, also contain a series SIGMA believed to be proportional to the standard deviation of each error.
To estimate WLS for this specification, open an equation dialog and enter
y c x
as the equation specification.
Click on the Options tab, and fill out the Weights section as depicted here. We select Inverse std. dev. as our Type, and specify “1/SIGMA” for our Weight series. Lastly, we select
EViews default as our Scaling method.
Click on OK to estimate the specified equation. The results are given by:
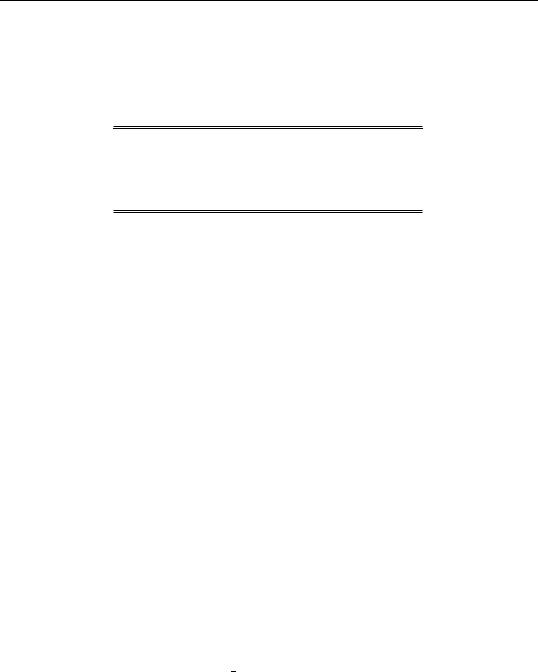
Weighted Least Squares—39
Dependent Variable: Y
Method: Least Squares
Date: 06/17/09 Time: 10:01
Sample: 1 9
Included observations: 9
Weighting series: 1/SIGMA
Weight type: Inverse standard deviation (EViews default scaling)
Variable |
Coefficient |
Std. Error |
t-Statistic |
Prob. |
|
|
|
|
|
|
|
|
|
|
C |
3406.640 |
80.98322 |
42.06600 |
0.0000 |
X |
154.1526 |
16.95929 |
9.089565 |
0.0000 |
|
|
|
|
|
|
|
|
|
|
Weighted Statistics
R-squared |
0.921893 |
Mean dependent var |
4098.417 |
Adjusted R-squared |
0.910734 |
S.D. dependent var |
629.1767 |
S.E. of regression |
126.6652 |
Akaike info criterion |
12.71410 |
Sum squared resid |
112308.5 |
Schwarz criterion |
12.75793 |
Log likelihood |
-55.21346 |
Hannan-Quinn criter. |
12.61952 |
F-statistic |
82.62018 |
Durbin-Watson stat |
1.183941 |
Prob(F-statistic) |
0.000040 |
Weighted mean dep. |
4039.404 |
|
|
|
|
|
|
|
|
|
Unweighted Statistics |
|
|
|
|
|
|
|
|
|
|
R-squared |
0.935499 |
Mean dependent var |
4161.667 |
Adjusted R-squared |
0.926285 |
S.D. dependent var |
420.5954 |
S.E. of regression |
114.1939 |
Sum squared resid |
91281.79 |
Durbin-Watson stat |
1.141034 |
|
|
|
|
|
|
|
|
|
|
The top portion of the output displays the estimation settings which show both the specified weighting series and the type of weighting employed in estimation. The middle section shows the estimated coefficient values and corresponding standard errors, t-statistics and probabilities.
The bottom portion of the output displays two sets of statistics. The Weighted Statistics show statistics corresponding to the actual estimated equation. For purposes of discussion, there are two types of summary statistics: those that are (generally) invariant to the scaling of the weights, and those that vary with the weight scale.
The “R-squared”, “Adjusted R-squared”, “F-statistic” and “Prob(F-stat)”, and the “DurbinWatson stat”, are all invariant to your choice of scale. Notice that these are all fit measures or test statistics which involve ratios of terms that remove the scaling.
One additional invariant statistic of note is the “Weighted mean dep.” which is the weighted mean of the dependent variable, computed as:
y |
|
= |
Âwtyt |
(19.17) |
w |
----------------- |
|||
|
|
Âwt |
|