
- •Preface
- •Part IV. Basic Single Equation Analysis
- •Chapter 18. Basic Regression Analysis
- •Equation Objects
- •Specifying an Equation in EViews
- •Estimating an Equation in EViews
- •Equation Output
- •Working with Equations
- •Estimation Problems
- •References
- •Chapter 19. Additional Regression Tools
- •Special Equation Expressions
- •Robust Standard Errors
- •Weighted Least Squares
- •Nonlinear Least Squares
- •Stepwise Least Squares Regression
- •References
- •Chapter 20. Instrumental Variables and GMM
- •Background
- •Two-stage Least Squares
- •Nonlinear Two-stage Least Squares
- •Limited Information Maximum Likelihood and K-Class Estimation
- •Generalized Method of Moments
- •IV Diagnostics and Tests
- •References
- •Chapter 21. Time Series Regression
- •Serial Correlation Theory
- •Testing for Serial Correlation
- •Estimating AR Models
- •ARIMA Theory
- •Estimating ARIMA Models
- •ARMA Equation Diagnostics
- •References
- •Chapter 22. Forecasting from an Equation
- •Forecasting from Equations in EViews
- •An Illustration
- •Forecast Basics
- •Forecasts with Lagged Dependent Variables
- •Forecasting with ARMA Errors
- •Forecasting from Equations with Expressions
- •Forecasting with Nonlinear and PDL Specifications
- •References
- •Chapter 23. Specification and Diagnostic Tests
- •Background
- •Coefficient Diagnostics
- •Residual Diagnostics
- •Stability Diagnostics
- •Applications
- •References
- •Part V. Advanced Single Equation Analysis
- •Chapter 24. ARCH and GARCH Estimation
- •Basic ARCH Specifications
- •Estimating ARCH Models in EViews
- •Working with ARCH Models
- •Additional ARCH Models
- •Examples
- •References
- •Chapter 25. Cointegrating Regression
- •Background
- •Estimating a Cointegrating Regression
- •Testing for Cointegration
- •Working with an Equation
- •References
- •Binary Dependent Variable Models
- •Ordered Dependent Variable Models
- •Censored Regression Models
- •Truncated Regression Models
- •Count Models
- •Technical Notes
- •References
- •Chapter 27. Generalized Linear Models
- •Overview
- •How to Estimate a GLM in EViews
- •Examples
- •Working with a GLM Equation
- •Technical Details
- •References
- •Chapter 28. Quantile Regression
- •Estimating Quantile Regression in EViews
- •Views and Procedures
- •Background
- •References
- •Chapter 29. The Log Likelihood (LogL) Object
- •Overview
- •Specification
- •Estimation
- •LogL Views
- •LogL Procs
- •Troubleshooting
- •Limitations
- •Examples
- •References
- •Part VI. Advanced Univariate Analysis
- •Chapter 30. Univariate Time Series Analysis
- •Unit Root Testing
- •Panel Unit Root Test
- •Variance Ratio Test
- •BDS Independence Test
- •References
- •Part VII. Multiple Equation Analysis
- •Chapter 31. System Estimation
- •Background
- •System Estimation Methods
- •How to Create and Specify a System
- •Working With Systems
- •Technical Discussion
- •References
- •Vector Autoregressions (VARs)
- •Estimating a VAR in EViews
- •VAR Estimation Output
- •Views and Procs of a VAR
- •Structural (Identified) VARs
- •Vector Error Correction (VEC) Models
- •A Note on Version Compatibility
- •References
- •Chapter 33. State Space Models and the Kalman Filter
- •Background
- •Specifying a State Space Model in EViews
- •Working with the State Space
- •Converting from Version 3 Sspace
- •Technical Discussion
- •References
- •Chapter 34. Models
- •Overview
- •An Example Model
- •Building a Model
- •Working with the Model Structure
- •Specifying Scenarios
- •Using Add Factors
- •Solving the Model
- •Working with the Model Data
- •References
- •Part VIII. Panel and Pooled Data
- •Chapter 35. Pooled Time Series, Cross-Section Data
- •The Pool Workfile
- •The Pool Object
- •Pooled Data
- •Setting up a Pool Workfile
- •Working with Pooled Data
- •Pooled Estimation
- •References
- •Chapter 36. Working with Panel Data
- •Structuring a Panel Workfile
- •Panel Workfile Display
- •Panel Workfile Information
- •Working with Panel Data
- •Basic Panel Analysis
- •References
- •Chapter 37. Panel Estimation
- •Estimating a Panel Equation
- •Panel Estimation Examples
- •Panel Equation Testing
- •Estimation Background
- •References
- •Part IX. Advanced Multivariate Analysis
- •Chapter 38. Cointegration Testing
- •Johansen Cointegration Test
- •Single-Equation Cointegration Tests
- •Panel Cointegration Testing
- •References
- •Chapter 39. Factor Analysis
- •Creating a Factor Object
- •Rotating Factors
- •Estimating Scores
- •Factor Views
- •Factor Procedures
- •Factor Data Members
- •An Example
- •Background
- •References
- •Appendix B. Estimation and Solution Options
- •Setting Estimation Options
- •Optimization Algorithms
- •Nonlinear Equation Solution Methods
- •References
- •Appendix C. Gradients and Derivatives
- •Gradients
- •Derivatives
- •References
- •Appendix D. Information Criteria
- •Definitions
- •Using Information Criteria as a Guide to Model Selection
- •References
- •Appendix E. Long-run Covariance Estimation
- •Technical Discussion
- •Kernel Function Properties
- •References
- •Index
- •Symbols
- •Numerics

Pooled Data—569
Object/Copy Selected… in the main workfile toolbar, or right mouse-click and select Object/Copy... and enter the new name
Pooled Data
As noted previously, all of your pooled data will be held in ordinary EViews series. These series can be used in all of the usual ways: they may, among other things, be tabulated, graphed, used to generate new series, or used in estimation. You may also use a pool object to work with sets of the individual series.
There are two classes of series in a pooled workfile: ordinary series and cross-section specific series.
Ordinary Series
An ordinary series is one that has common values across all cross-sections. A single series may be used to hold the data for each variable, and these data may be applied to every cross-section. For example, in a pooled workfile with firm cross-section identifiers, data on overall economic conditions such as GDP or money supply do not vary across firms. You need only create a single series to hold the GDP data, and a single series to hold the money supply variable.
Since ordinary series do not interact with cross-sections, they may be defined without reference to a pool object. Most importantly, there are no naming conventions associated with ordinary series beyond those for ordinary EViews objects.
Cross-section Specific Series
Cross-section specific series are those that have values that differ between cross-sections. A set of these series are required to hold the data for a given variable, with each series corresponding to data for a specific cross-section.
Since cross-section specific series interact with cross-sections, they should be defined in conjunction with the identifiers in pool objects. Suppose, for example, that you have a pool object that contains the identifiers “_USA,” “_KOR,” “_JPN,” and “_UK”, and that you have time series data on GDP for each of the cross-section units. In this setting, you should have a four cross-section specific GDP series in your workfile.
The key to naming your cross-section specific series is to use names that are a combination of a base name and a cross-section identifier. The cross-section identifiers may be embedded at an arbitrary location in the series name, so long as this is done consistently across identifiers.
You may elect to place the identifier at the end of the base name, in which case, you should name your series “GDP_USA,” “GDP_KOR,” “GDP_JPN,” and “GDP_UK”. Alternatively, you may choose to put the section identifiers in front of the name, so that you have the

570—Chapter 35. Pooled Time Series, Cross-Section Data
names “_USAGDP,” “_KORGDP,” “_JPNGDP,” and “_UKGDP”. The identifiers may also be placed in the middle of series names—for example, using the names “GDP_USAINF,” “GDP_KORIN,” “GDP_JPNIN,” “GDP_UKIN”.
It really doesn’t matter whether the identifiers are used at the beginning, middle, or end of your cross-section specific names; you should adopt a naming style that you find easiest to manage. Consistency in the naming of the set of cross-section series is, however, absolutely essential. You should not, for example, name your four GDP series “GDP_USA”, “GDP_KOR”, “_JPNGDPIN”, “_UKGDP”, as this will make it impossible for EViews to refer to the set of series using a pool object.
Pool Series
Once your series names have been chosen to correspond with the identifiers in your pool, the pool object can be used to work with a set of series as though it were a single item. The key to this processing is the concept of a pool series.
A pool series is actually a set of series defined by a base name and the entire list of crosssection identifiers in a specified pool. Pool series are specified using the base name, and a “?” character placeholder for the cross-section identifier. If your series are named “GDP_USA”, “GDP_KOR”, “GDP_JPN”, and “GDP_UK”, the corresponding pool series may be referred to as “GDP?”. If the names of your series are “_USAGDP”, “_KORGDP”, “_JPNGDP”, and “_UKGDP”, the pool series is “?GDP”.
When you use a pool series name, EViews understands that you wish to work with all of the series in the workfile that match the pool series specification. EViews loops through the list of cross-section identifiers in the specified pool, and substitutes each identifier in place of the “?”. EViews then uses the complete set of cross-section specific series formed in this fashion.
In addition to pool series defined with “?”, EViews provides a special function, @INGRP, that you may use to generate a group identity pool series that takes the value 1 if an observation is in the specified group, and 0 otherwise.
Consider, for example, the @GROUP for “ASIA” defined using the identifiers “_KOR” and “_JPN”, and suppose that we wish to create a dummy variable series for whether an observation is in the group. One approach to representing these data is to create the following four cross-section specific series:
series asia_usa = 0 series asia_kor = 1 series asia_jpn = 1 series asia_uk = 0

Setting up a Pool Workfile—571
and to refer to them collectively as the pool series “ASIA_?”. While not particularly difficult to do, this direct approach becomes more cumbersome the greater the number of cross-sec- tion identifiers.
More easily, we may use the special pool series expression:
@ingrp(asia)
to define a special virtual pool series in which each observation takes a 0 or 1 indicator for whether an observation is in the specified group. This expression is equivalent to creating the four cross-section specific series, and referring to them as “ASIA_?”.
We must emphasize that pool series specifiers using the “?” and the @INGRP function may only be used through a pool object, since they have no meaning without a list of cross-sec- tion identifiers. If you attempt to use a pool series outside the context of a pool object, EViews will attempt to interpret the “?” as a wildcard character (see Appendix A. “Wildcards,” on page 559 in the Command and Programming Reference). The result, most often, will be an error message saying that your variable is not defined.
Setting up a Pool Workfile
Your goal in setting up a pool workfile is to obtain a workfile containing individual series for ordinary variables, sets of appropriately named series for the cross-section specific data, and pool objects containing the related sets of identifiers. The workfile should have frequency and range matching the time series dimension of your pooled data.
There are two basic approaches to setting up such a workfile. The direct approach involves first creating an empty workfile with the desired structure, and then importing data into individual series using either standard or pool specific import methods. The indirect approach involves first creating a stacked representation of the data in EViews, and then using EViews built-in reshaping tools to set up a pooled workfile.
Direct Setup
The direct approach to setting up your pool workfile involves three distinct steps: first creating a workfile with the desired time series structure; next, creating one or more pool objects containing the desired cross-section identifiers; and lastly, using pool object tools to import data into individual series in the workfile.
Creating the Workfile and Pool Object
The first step in the direct setup is to create an ordinary EViews workfile structured to match the time series dimension of your data. The range of your workfile should represent the earliest and latest dates or observations you wish to consider for any of the cross-section units.
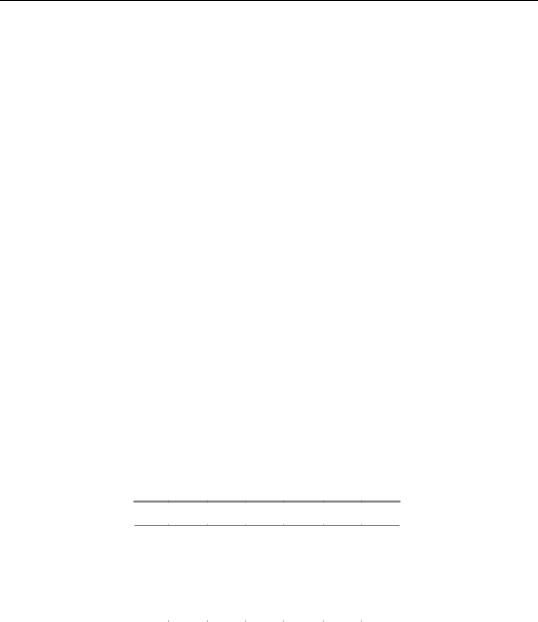
572—Chapter 35. Pooled Time Series, Cross-Section Data
Simply select File/New workfile... to bring up the Workfile Create dialog which you will use to describe the structure of your workfile. For additional detail, see “Creating a Workfile by Describing its Structure” on page 35 of User’s Guide I.
For example, to create a pool workfile that has annual data ranging from 1950 to 1992, simply select Annual in the Frequency combo box, and enter “1950” as the Start date and “1992” as the End date.
Next, you should create one or more pool objects containing cross-section identifiers and group definitions as described in “The Pool Object” on page 566.
Importing Pooled Data
Lastly, you should use one of the various methods for importing data into series in the workfile. Before considering the various approaches, we require an understanding the various representations of pooled time series, cross-section data that you may encounter.
Bear in mind that in a pooled setting, a given observation on a variable may be indexed along three dimensions: the variable, the cross-section, and the time period. For example, you may be interested in the value of GDP, for the U.K., in 1989.
Despite the fact that there are three dimensions of interest, you will eventually find yourself working with a two-dimensional representation of your pooled data. There is obviously no unique way to organize three-dimensional data in two-dimensions, but several formats are commonly employed.
Unstacked Data
In this form, observations on a given variable for a given cross-section are grouped together, but are separated from observations for other variables and other cross sections. For example, suppose the top of our Excel data file contains the following:
year c_usa c_kor c_jpn g_usa g_jpn g_kor
1954 |
61.6 |
77.4 |
66 |
17.8 |
18.7 |
17.6 |
1955 |
61.1 |
79.2 |
65.7 |
15.8 |
17.1 |
16.9 |
1956 |
61.7 |
80.2 |
66.1 |
15.7 |
15.9 |
17.5 |
1957 |
62.4 |
78.6 |
65.5 |
16.3 |
14.8 |
16.3 |
… |
… |
… |
… |
… |
… |
… |
Here, the base name “C” represents consumption, while “G” represents government expenditure. Each country has its own separately identified column for consumption, and its own column for government expenditure.
EViews pooled workfiles are structured to work naturally with data that are unstacked, since the sets of cross-section specific series in the pool workfile correspond directly to the
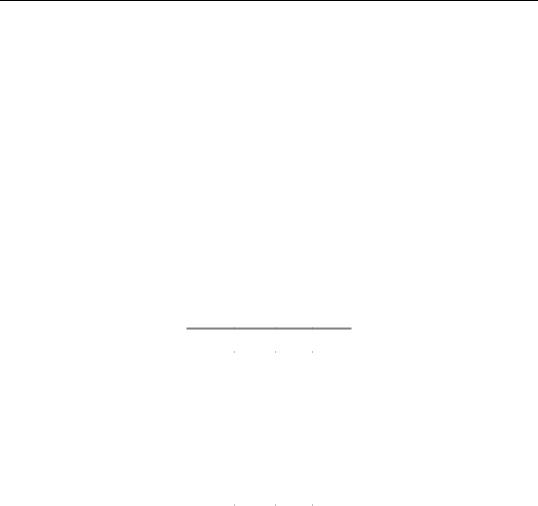
Setting up a Pool Workfile—573
multiple columns of unstacked source data. You may read unstacked data directly into EViews using the standard workfile creation procedures described in “Creating a Workfile by Reading from a Foreign Data Source” on page 39 of User’s Guide I. Each cross-section specific variable should be read as an individual series, with the names of the resulting series follow the pool naming conventions given in your pool object. Ordinary series may be imported in the usual fashion with no additional complications.
In this example, we should use the standard EViews tools to read separate series for each column. We create the individual series “YEAR”, “C_USA”, “C_KOR”, “C_JPN”, “G_USA”, “G_JPN”, and “G_KOR”.
Stacked Data
Pooled data can also be arranged in stacked form, where all of the data for a variable are grouped together in a single column.
In the most common form, the data for different cross-sections are stacked on top of one another, with all of the sequentially dated observations for a given cross-section grouped together. We may say that these data are stacked by cross-section:
id |
year |
c |
g |
_usa |
1954 |
61.6 |
17.8 |
_usa |
… |
… |
… |
_usa |
… |
… |
… |
_usa |
1992 |
68.1 |
13.2 |
…… … …
_kor |
1954 |
77.4 |
17.6 |
_kor |
… |
… |
… |
_kor |
1992 |
na |
na |
Alternatively, we may have data that are stacked by date, with all of the observations of a given period grouped together:
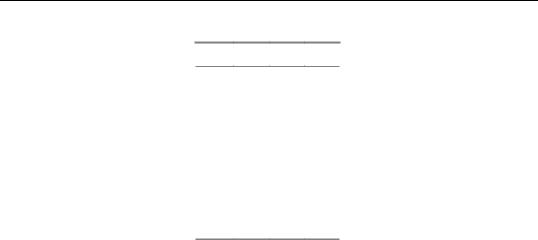
574—Chapter 35. Pooled Time Series, Cross-Section Data
per id c g
1954 _usa 61.6 17.8
1954 _uk 62.4 23.8
1954 _jpn 66 18.7
1954 _kor 77.4 17.6
…… … …
1992 _usa 68.1 13.2
1992 _uk 67.9 17.3
1992 _jpn 54.2 7.6
1992 _kor na na
Each column again represents a single variable, but within each column, all of the cross-sec- tions for a given year are grouped together. If data are stacked by year, you should make certain that the ordering of the cross-sectional identifiers within a year is consistent across years.
There are to primary approaches to importing data into your pool series: you may read the data in stacked form then use EViews tools to restructure the data in pool form, or you may directly read or copy the data into a stacked representation of the pooled series.
Indirect Setup (Restructuring) of Stacked Data
The easiest approach to reading stacked pool data is to create an EViews workfile containing the data in stacked form, and then use the built-in workfile reshaping tools to create a pool workfile with the desired structure and data. (Alternately, you can perform the first step and simply work with the data in stacked form: see Chapter 36. “Working with Panel Data,” on page 615 for details.)
The first step in the indirect setup of a pool workfile is to create a workfile containing the contents of your stacked data file. You may manually create the workfile and import the stacked series data, or you may use EViews tools for opening foreign source data directly into a new workfile (“Creating a Workfile by Reading from a Foreign Data Source” on page 39 of User’s Guide I).
Once you have your stacked data in an EViews workfile, you may use the workfile reshaping tools to unstack the data into a pool workfile page. In addition to unstacking the data into multiple series, EViews will create a pool object containing identifiers obtained from patterns in the series names. See “Reshaping a Workfile,” beginning on page 248 of User’s Guide I for a general discussion of reshaping, and “Unstacking a Workfile” on page 251 of User’s Guide I for a more specific discussion of the unstack procedure.
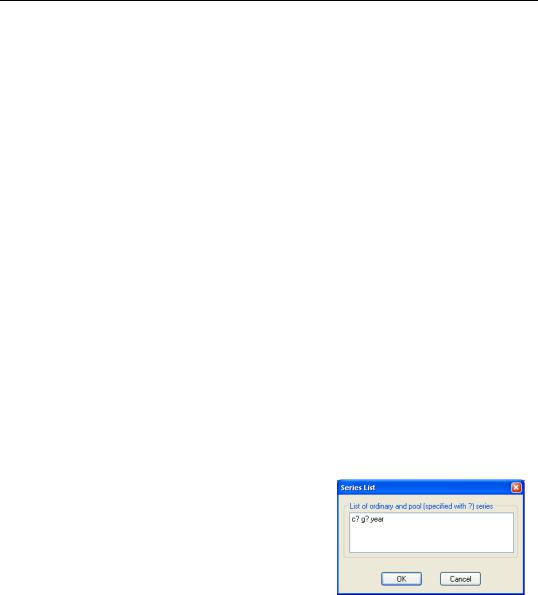
Setting up a Pool Workfile—575
The indirect method is generally easier to use than the direct approach and has the advantage of not requiring that the stacked data be balanced. It has the disadvantage of using more computer memory since EViews must have two copies of the source data in memory at the same time.
Direct Import of Stacked Data
An alternative approach is to enter or read the data directly into the workfile using a pool object. You may enter or copy-and-paste data from the source into and a stacked representation of your data, or you may use the pool object to describe how to read the stacked data into the unstacked workfile.
To enter data or copy-and-paste, you use the pool object to create a stacked representation of the data in EViews:
•First, specify which time series observations will be included in your stacked spreadsheet by setting the workfile sample.
•Next, open the pool, then select View/Spreadsheet View… EViews will prompt you for a list of series. You can enter ordinary series names or pool series names. If the series exist, then EViews will display the data in the series. If the series do not exist, then EViews will create the series or group of series, using the cross-section identifiers if you specify a pool series.
•EViews will open the stacked spreadsheet view of the pool series. If desired, click on the Order +/– button to toggle between stacking by cross-section and stacking by date.
•Click Edit +/– to turn on edit mode in the spreadsheet window, and enter your data, or cut-and-paste from another application.
For example, if we have a pool object that contains the identifiers “_USA”, “_UK”, “_JPN”, and “_KOR”, we can instruct EViews to create the series C_USA, C_UK, C_JPN, C_KOR, and G_USA, G_UK, G_JPN, G_KOR, and YEAR simply by entering the pool series names “C?”, “G?” and the ordinary series name “YEAR”, and pressing OK.
EViews will open a stacked spreadsheet view of the
series in your list. Here we see the series stacked by cross-section, with the pool or ordinary series names in the column header, and the cross-section/date identifiers labeling each row. Note that since YEAR is an ordinary series, its values are repeated for each cross-section in the stacked spreadsheet.
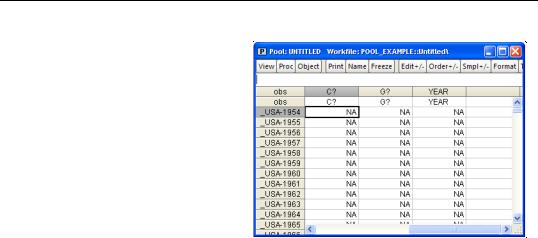
576—Chapter 35. Pooled Time Series, Cross-Section Data
If desired, click on Order +/– to toggle between stacking methods to match the organization of the data to be imported. Click on Edit +/– to turn on edit mode, and enter or cut- and-paste into the window.
Alternatively, you can import stacked data from a file using import tools built into the pool object. While the data in the file may be stacked either by cross-section or by period, EViews does require that the
stacked data are “balanced,” and that the cross-sections ordering in the file matches the cross-sectional identifiers in the pool. By “balanced,” we mean that if the data are stacked by cross-section, each cross-section should contain exactly the same number of periods—if the data are stacked by date, each date should have exactly the same number of cross-sec- tional observations arranged in the same order.
We emphasize that only the representation of the data in the import file needs to be balanced; the underlying data need not be balanced. Notably, if you have missing values for some observations, you should make certain that there are lines in the file representing the missing values. In the two examples above, the underlying data are not balanced, since information is not available for Korea in 1992. The data in the file have been balanced by including an observation for the missing data.
To import stacked pool data from a file, first open the pool object, then select Proc/Import Pool data (ASCII, .XLS, .WK?)…It is important that you use the import procedure associated with the pool object, and not the standard file import procedure.
Select your input file in the usual fashion. If you select a spreadsheet file, EViews will open a spreadsheet import dialog prompting you for additional input.
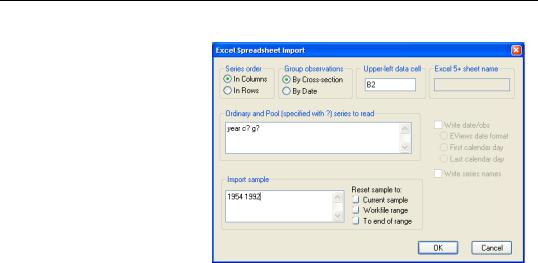
Setting up a Pool Workfile—577
Much of this dialog should be familiar from the discussion in “Importing Data from a Spreadsheet or Text File” on page 105 of User’s Guide I.
First, indicate whether the pool series are in rows or in columns, and whether the data are stacked by crosssection, or stacked by date.
Next, in the pool series edit box, enter the names of the
series you wish to import. This list may contain any combination of ordinary series names and pool series names.
Lastly, fill in the sample information, starting cell location, and optionally, the sheet name.
When you specify your series using pool series names, EViews will, if necessary, create and name the corresponding set of pool series using the list of cross-section identifiers in the pool object. If you list an ordinary series name, EViews will, if needed, create a single series to hold the data.
EViews will read the contents of your file into the specified pool variables using the sample information. When reading into pool series, the first set of observations in the file will be placed in the individual series corresponding to the first cross-section (if reading data that is grouped by cross-section), or the first sample observation of each series in the set of crosssectional series (if reading data that is grouped by date), and so forth.
If you read data into an ordinary series, EViews will continually assign values into the corresponding observation of the single series, so that upon completion of the import procedure, the series will contain the last set of values read from the file.
The basic technique for importing stacked data from ASCII text files is analogous, but the corresponding dialog contains many additional options to handle the complexity of text files.