
- •Preface
- •Part IV. Basic Single Equation Analysis
- •Chapter 18. Basic Regression Analysis
- •Equation Objects
- •Specifying an Equation in EViews
- •Estimating an Equation in EViews
- •Equation Output
- •Working with Equations
- •Estimation Problems
- •References
- •Chapter 19. Additional Regression Tools
- •Special Equation Expressions
- •Robust Standard Errors
- •Weighted Least Squares
- •Nonlinear Least Squares
- •Stepwise Least Squares Regression
- •References
- •Chapter 20. Instrumental Variables and GMM
- •Background
- •Two-stage Least Squares
- •Nonlinear Two-stage Least Squares
- •Limited Information Maximum Likelihood and K-Class Estimation
- •Generalized Method of Moments
- •IV Diagnostics and Tests
- •References
- •Chapter 21. Time Series Regression
- •Serial Correlation Theory
- •Testing for Serial Correlation
- •Estimating AR Models
- •ARIMA Theory
- •Estimating ARIMA Models
- •ARMA Equation Diagnostics
- •References
- •Chapter 22. Forecasting from an Equation
- •Forecasting from Equations in EViews
- •An Illustration
- •Forecast Basics
- •Forecasts with Lagged Dependent Variables
- •Forecasting with ARMA Errors
- •Forecasting from Equations with Expressions
- •Forecasting with Nonlinear and PDL Specifications
- •References
- •Chapter 23. Specification and Diagnostic Tests
- •Background
- •Coefficient Diagnostics
- •Residual Diagnostics
- •Stability Diagnostics
- •Applications
- •References
- •Part V. Advanced Single Equation Analysis
- •Chapter 24. ARCH and GARCH Estimation
- •Basic ARCH Specifications
- •Estimating ARCH Models in EViews
- •Working with ARCH Models
- •Additional ARCH Models
- •Examples
- •References
- •Chapter 25. Cointegrating Regression
- •Background
- •Estimating a Cointegrating Regression
- •Testing for Cointegration
- •Working with an Equation
- •References
- •Binary Dependent Variable Models
- •Ordered Dependent Variable Models
- •Censored Regression Models
- •Truncated Regression Models
- •Count Models
- •Technical Notes
- •References
- •Chapter 27. Generalized Linear Models
- •Overview
- •How to Estimate a GLM in EViews
- •Examples
- •Working with a GLM Equation
- •Technical Details
- •References
- •Chapter 28. Quantile Regression
- •Estimating Quantile Regression in EViews
- •Views and Procedures
- •Background
- •References
- •Chapter 29. The Log Likelihood (LogL) Object
- •Overview
- •Specification
- •Estimation
- •LogL Views
- •LogL Procs
- •Troubleshooting
- •Limitations
- •Examples
- •References
- •Part VI. Advanced Univariate Analysis
- •Chapter 30. Univariate Time Series Analysis
- •Unit Root Testing
- •Panel Unit Root Test
- •Variance Ratio Test
- •BDS Independence Test
- •References
- •Part VII. Multiple Equation Analysis
- •Chapter 31. System Estimation
- •Background
- •System Estimation Methods
- •How to Create and Specify a System
- •Working With Systems
- •Technical Discussion
- •References
- •Vector Autoregressions (VARs)
- •Estimating a VAR in EViews
- •VAR Estimation Output
- •Views and Procs of a VAR
- •Structural (Identified) VARs
- •Vector Error Correction (VEC) Models
- •A Note on Version Compatibility
- •References
- •Chapter 33. State Space Models and the Kalman Filter
- •Background
- •Specifying a State Space Model in EViews
- •Working with the State Space
- •Converting from Version 3 Sspace
- •Technical Discussion
- •References
- •Chapter 34. Models
- •Overview
- •An Example Model
- •Building a Model
- •Working with the Model Structure
- •Specifying Scenarios
- •Using Add Factors
- •Solving the Model
- •Working with the Model Data
- •References
- •Part VIII. Panel and Pooled Data
- •Chapter 35. Pooled Time Series, Cross-Section Data
- •The Pool Workfile
- •The Pool Object
- •Pooled Data
- •Setting up a Pool Workfile
- •Working with Pooled Data
- •Pooled Estimation
- •References
- •Chapter 36. Working with Panel Data
- •Structuring a Panel Workfile
- •Panel Workfile Display
- •Panel Workfile Information
- •Working with Panel Data
- •Basic Panel Analysis
- •References
- •Chapter 37. Panel Estimation
- •Estimating a Panel Equation
- •Panel Estimation Examples
- •Panel Equation Testing
- •Estimation Background
- •References
- •Part IX. Advanced Multivariate Analysis
- •Chapter 38. Cointegration Testing
- •Johansen Cointegration Test
- •Single-Equation Cointegration Tests
- •Panel Cointegration Testing
- •References
- •Chapter 39. Factor Analysis
- •Creating a Factor Object
- •Rotating Factors
- •Estimating Scores
- •Factor Views
- •Factor Procedures
- •Factor Data Members
- •An Example
- •Background
- •References
- •Appendix B. Estimation and Solution Options
- •Setting Estimation Options
- •Optimization Algorithms
- •Nonlinear Equation Solution Methods
- •References
- •Appendix C. Gradients and Derivatives
- •Gradients
- •Derivatives
- •References
- •Appendix D. Information Criteria
- •Definitions
- •Using Information Criteria as a Guide to Model Selection
- •References
- •Appendix E. Long-run Covariance Estimation
- •Technical Discussion
- •Kernel Function Properties
- •References
- •Index
- •Symbols
- •Numerics
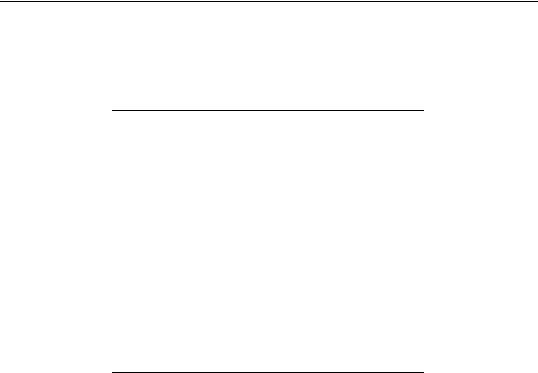
32—Chapter 19. Additional Regression Tools
Dependent Variable: LPRICE
Method: Least Squares
Date: 08/08/09 Time: 22:15
Sample: 1 506
Included observations: 506
Variable |
Coefficient |
Std. Error |
t-Statistic |
Prob. |
|
|
|
|
|
|
|
|
|
|
C |
8.811812 |
0.217787 |
40.46069 |
0.0000 |
LNOX |
-0.487579 |
0.084998 |
-5.736396 |
0.0000 |
ROOMS |
0.284844 |
0.018790 |
15.15945 |
0.0000 |
RADIAL=1 |
0.118444 |
0.072129 |
1.642117 |
0.1012 |
RADIAL=2 |
0.219063 |
0.066055 |
3.316398 |
0.0010 |
RADIAL=3 |
0.274176 |
0.059458 |
4.611253 |
0.0000 |
RADIAL=4 |
0.149156 |
0.042649 |
3.497285 |
0.0005 |
RADIAL=5 |
0.298730 |
0.037827 |
7.897337 |
0.0000 |
RADIAL=6 |
0.189901 |
0.062190 |
3.053568 |
0.0024 |
RADIAL=7 |
0.201679 |
0.077635 |
2.597794 |
0.0097 |
RADIAL=8 |
0.258814 |
0.066166 |
3.911591 |
0.0001 |
|
|
|
|
|
|
|
|
|
|
R-squared |
0.573871 |
Adjusted R-squared |
0.565262 |
S.E. of regression |
0.269841 |
Sum squared resid |
36.04295 |
Log likelihood |
-49.60111 |
F-statistic |
66.66195 |
Prob(F-statistic) |
0.000000 |
Mean dependent var |
9.941057 |
S.D. dependent var |
0.409255 |
Akaike info criterion |
0.239530 |
Schwarz criterion |
0.331411 |
Hannan-Quinn criter. |
0.275566 |
Durbin-W atson stat |
0.671010 |
Robust Standard Errors
In the standard least squares model, the coefficient variance-covariance matrix may be derived as:
S = |
E(b – b)(b – b)¢ |
|
||
|
ˆ |
ˆ |
|
|
= |
(X¢X)–1E(X¢ee¢X)(X¢X)–1 |
(19.8) |
||
= |
(X¢X)–1T Q (X¢X)–1 |
|||
|
||||
= |
j2(X¢X)–1 |
|
A key part of this derivation is the assumption that the error terms, e , are conditionally homoskedastic, which implies that Q = E(X¢ee¢X § T) = j2(X¢X § T) . A sufficient, but not necessary, condition for this restriction is that the errors are i.i.d. In cases where this assumption is relaxed to allow for heteroskedasticity or autocorrelation, the expression for the covariance matrix will be different.
EViews provides built-in tools for estimating the coefficient covariance under the assumption that the residuals are conditionally heteroskedastic, and under the assumption of heteroskedasticity and autocorrelation. The coefficient covariance estimator under the first assumption is termed a Heteroskedasticity Consistent Covariance (White) estimator, and the
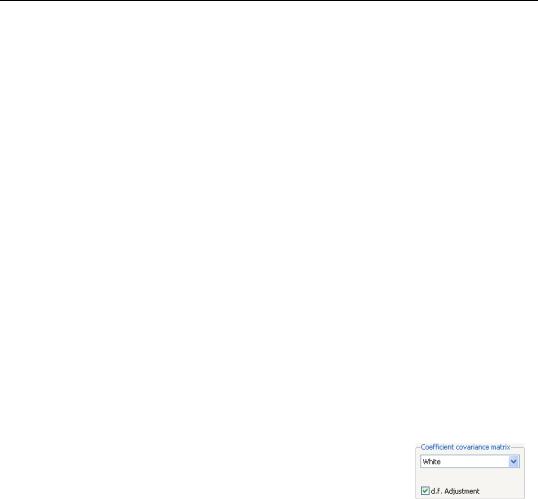
Robust Standard Errors—33
estimator under the latter is a Heteroskedasticity and Autocorrelation Consistent Covariance (HAC) or Newey-West estimator. Note that both of these approaches will change the coefficient standard errors of an equation, but not their point estimates.
Heteroskedasticity Consistent Covariances (White)
White (1980) derived a heteroskedasticity consistent covariance matrix estimator which provides consistent estimates of the coefficient covariances in the presence of conditional
heteroskedasticity of unknown form. Under the White specification we estimate |
Q using: |
||||||
ˆ |
|
T |
T |
|
2 |
|
|
= |
 |
ˆ |
XtXt¢ § T |
|
|||
Q |
------------ |
|
(19.9) |
||||
T – k |
e |
t |
|||||
|
|
|
t = 1 |
|
|
|
|
ˆ |
are the estimated residuals, T is the number of observations, k is the number of |
||||||||||
where et |
|||||||||||
regressors, and T § (T – k) is an optional degree-of-freedom correction. The degree-of-free- |
|||||||||||
dom White heteroskedasticity consistent covariance matrix estimator is given by |
|
||||||||||
|
ˆ |
= |
T |
(X¢X) |
–1 |
T |
e |
2 |
|
–1 |
(19.10) |
|
SW |
------------ |
|
|
|
X X ¢ (X¢X) |
|
||||
|
|
|
|
|
tÂ= 1 |
ˆ |
t |
t t |
|
|
|
|
|
|
T – k |
|
|
|
|
|
To illustrate the use of White covariance estimates, we use an example from Wooldridge (2000, p. 251) of an estimate of a wage equation for college professors. The equation uses dummy variables to examine wage differences between four groups of individuals: married men (MARRMALE), married women (MARRFEM), single women (SINGLEFEM), and the base group of single men. The explanatory variables include levels of education (EDUC), experience (EXPER) and tenure (TENURE). The data are in the workfile “Wooldridge.WF1”.
To select the White covariance estimator, specify the equation as before, then select the Options tab and select White in the Coefficient covariance matrix drop-down. You may, if desired, use the checkbox to remove the default d.f. Adjustment, but in this example, we will use the default setting.
The output for the robust covariances for this regression are shown below:
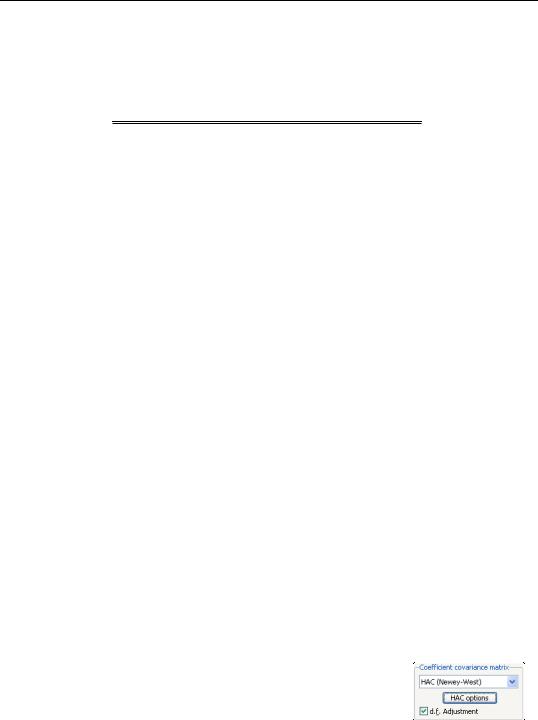
34—Chapter 19. Additional Regression Tools
Dependent Variable: LOG(WAGE)
Method: Least Squares
Date: 04/13/09 Time: 16:56
Sample: 1 526
Included observations: 526
White heteroskedasticity-consistent standard errors & covariance
Variable |
Coefficient |
Std. Error |
t-Statistic |
Prob. |
|
|
|
|
|
|
|
|
|
|
C |
0.321378 |
0.109469 |
2.935791 |
0.0035 |
MARRMALE |
0.212676 |
0.057142 |
3.721886 |
0.0002 |
MARRFEM |
-0.198268 |
0.058770 |
-3.373619 |
0.0008 |
SINGFEM |
-0.110350 |
0.057116 |
-1.932028 |
0.0539 |
EDUC |
0.078910 |
0.007415 |
10.64246 |
0.0000 |
EXPER |
0.026801 |
0.005139 |
5.215010 |
0.0000 |
EXPER^2 |
-0.000535 |
0.000106 |
-5.033361 |
0.0000 |
TENURE |
0.029088 |
0.006941 |
4.190731 |
0.0000 |
TENURE^2 |
-0.000533 |
0.000244 |
-2.187835 |
0.0291 |
|
|
|
|
|
|
|
|
|
|
R-squared |
0.460877 |
Mean dependent var |
1.623268 |
|
Adjusted R-squared |
0.452535 |
S.D. dependent var |
0.531538 |
|
S.E. of regression |
0.393290 |
Akaike info criterion |
0.988423 |
|
Sum squared resid |
79.96799 |
Schwarz criterion |
1.061403 |
|
Log likelihood |
-250.9552 |
Hannan-Quinn criter. |
1.016998 |
|
F-statistic |
55.24559 |
Durbin-Watson stat |
1.784785 |
|
Prob(F-statistic) |
0.000000 |
|
|
|
|
|
|
|
|
As Wooldridge notes, the heteroskedasticity robust standard errors for this specification are not very different from the non-robust forms, and the test statistics for statistical significance of coefficients are generally unchanged. While robust standard errors are often larger than their usual counterparts, this is not necessarily the case, and indeed this equation has some robust standard errors that are smaller than the conventional estimates.
HAC Consistent Covariances (Newey-West)
The White covariance matrix described above assumes that the residuals of the estimated equation are serially uncorrelated. Newey and West (1987b) have proposed a more general covariance estimator that is consistent in the presence of both heteroskedasticity and autocorrelation of unknown form. They propose using HAC methods to form an estimate of
E(X¢ee¢X § T). Then the HAC coefficient covariance estimator is given by:
ˆ |
= (X'X) |
–1 |
ˆ |
–1 |
(19.11) |
SNW |
|
T Q (X'X) |
|
where ˆ is any of the LRCOV estimators described in Appendix E. “Long-run Covariance
Q
Estimation,” on page 775.
To use the Newey-West HAC method, select the Options tab and select HAC (Newey-West) in the Coefficient covariance matrix drop-down. As before, you may use the checkbox to remove the default d.f. Adjustment.
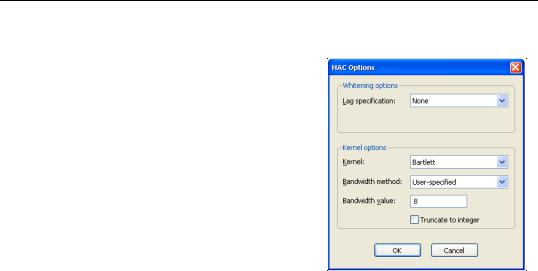
Robust Standard Errors—35
Press the HAC options button to change the options for the LRCOV estimate.
We illustrate the computation of HAC covariances using an example from Stock and Watson (2007, p. 620). In this example, the percentage change of the price of orange juice is regressed upon a constant and the number of days the temperature in Florida reached zero for the current and previous 18 months, using monthly data from 1950 to 2000 The data are in the workfile “Stock_wat.WF1”.
Stock and Watson report Newey-West standard errors computed using a non pre-whitened Bartlett Kernel with a user-specified bandwidth of 8 (note that the bandwidth is equal to one plus what Stock and Watson term the “truncation parameter” m ).
The results of this estimation are shown below: