
- •Preface
- •Part IV. Basic Single Equation Analysis
- •Chapter 18. Basic Regression Analysis
- •Equation Objects
- •Specifying an Equation in EViews
- •Estimating an Equation in EViews
- •Equation Output
- •Working with Equations
- •Estimation Problems
- •References
- •Chapter 19. Additional Regression Tools
- •Special Equation Expressions
- •Robust Standard Errors
- •Weighted Least Squares
- •Nonlinear Least Squares
- •Stepwise Least Squares Regression
- •References
- •Chapter 20. Instrumental Variables and GMM
- •Background
- •Two-stage Least Squares
- •Nonlinear Two-stage Least Squares
- •Limited Information Maximum Likelihood and K-Class Estimation
- •Generalized Method of Moments
- •IV Diagnostics and Tests
- •References
- •Chapter 21. Time Series Regression
- •Serial Correlation Theory
- •Testing for Serial Correlation
- •Estimating AR Models
- •ARIMA Theory
- •Estimating ARIMA Models
- •ARMA Equation Diagnostics
- •References
- •Chapter 22. Forecasting from an Equation
- •Forecasting from Equations in EViews
- •An Illustration
- •Forecast Basics
- •Forecasts with Lagged Dependent Variables
- •Forecasting with ARMA Errors
- •Forecasting from Equations with Expressions
- •Forecasting with Nonlinear and PDL Specifications
- •References
- •Chapter 23. Specification and Diagnostic Tests
- •Background
- •Coefficient Diagnostics
- •Residual Diagnostics
- •Stability Diagnostics
- •Applications
- •References
- •Part V. Advanced Single Equation Analysis
- •Chapter 24. ARCH and GARCH Estimation
- •Basic ARCH Specifications
- •Estimating ARCH Models in EViews
- •Working with ARCH Models
- •Additional ARCH Models
- •Examples
- •References
- •Chapter 25. Cointegrating Regression
- •Background
- •Estimating a Cointegrating Regression
- •Testing for Cointegration
- •Working with an Equation
- •References
- •Binary Dependent Variable Models
- •Ordered Dependent Variable Models
- •Censored Regression Models
- •Truncated Regression Models
- •Count Models
- •Technical Notes
- •References
- •Chapter 27. Generalized Linear Models
- •Overview
- •How to Estimate a GLM in EViews
- •Examples
- •Working with a GLM Equation
- •Technical Details
- •References
- •Chapter 28. Quantile Regression
- •Estimating Quantile Regression in EViews
- •Views and Procedures
- •Background
- •References
- •Chapter 29. The Log Likelihood (LogL) Object
- •Overview
- •Specification
- •Estimation
- •LogL Views
- •LogL Procs
- •Troubleshooting
- •Limitations
- •Examples
- •References
- •Part VI. Advanced Univariate Analysis
- •Chapter 30. Univariate Time Series Analysis
- •Unit Root Testing
- •Panel Unit Root Test
- •Variance Ratio Test
- •BDS Independence Test
- •References
- •Part VII. Multiple Equation Analysis
- •Chapter 31. System Estimation
- •Background
- •System Estimation Methods
- •How to Create and Specify a System
- •Working With Systems
- •Technical Discussion
- •References
- •Vector Autoregressions (VARs)
- •Estimating a VAR in EViews
- •VAR Estimation Output
- •Views and Procs of a VAR
- •Structural (Identified) VARs
- •Vector Error Correction (VEC) Models
- •A Note on Version Compatibility
- •References
- •Chapter 33. State Space Models and the Kalman Filter
- •Background
- •Specifying a State Space Model in EViews
- •Working with the State Space
- •Converting from Version 3 Sspace
- •Technical Discussion
- •References
- •Chapter 34. Models
- •Overview
- •An Example Model
- •Building a Model
- •Working with the Model Structure
- •Specifying Scenarios
- •Using Add Factors
- •Solving the Model
- •Working with the Model Data
- •References
- •Part VIII. Panel and Pooled Data
- •Chapter 35. Pooled Time Series, Cross-Section Data
- •The Pool Workfile
- •The Pool Object
- •Pooled Data
- •Setting up a Pool Workfile
- •Working with Pooled Data
- •Pooled Estimation
- •References
- •Chapter 36. Working with Panel Data
- •Structuring a Panel Workfile
- •Panel Workfile Display
- •Panel Workfile Information
- •Working with Panel Data
- •Basic Panel Analysis
- •References
- •Chapter 37. Panel Estimation
- •Estimating a Panel Equation
- •Panel Estimation Examples
- •Panel Equation Testing
- •Estimation Background
- •References
- •Part IX. Advanced Multivariate Analysis
- •Chapter 38. Cointegration Testing
- •Johansen Cointegration Test
- •Single-Equation Cointegration Tests
- •Panel Cointegration Testing
- •References
- •Chapter 39. Factor Analysis
- •Creating a Factor Object
- •Rotating Factors
- •Estimating Scores
- •Factor Views
- •Factor Procedures
- •Factor Data Members
- •An Example
- •Background
- •References
- •Appendix B. Estimation and Solution Options
- •Setting Estimation Options
- •Optimization Algorithms
- •Nonlinear Equation Solution Methods
- •References
- •Appendix C. Gradients and Derivatives
- •Gradients
- •Derivatives
- •References
- •Appendix D. Information Criteria
- •Definitions
- •Using Information Criteria as a Guide to Model Selection
- •References
- •Appendix E. Long-run Covariance Estimation
- •Technical Discussion
- •Kernel Function Properties
- •References
- •Index
- •Symbols
- •Numerics

Examples—307
ting or Hessian - expected, Hessian - observed, and OPG - BHHH. If you are computing Huber/White covariances, only the two Hessian based selections will be displayed.
By default, EViews will match the estimator to the one used in estimation as specified in the Estimation Options section. Thus, equations estimated by Quadratic Hill Climbing and Newton-Raphson will use the observed information, while those using IRLS or BHHH will use the expected information matrix or outer-product of the gradients, respectively.
The one exception to the default matching of estimation and covariance information matrices occurs when you estimate the equation using BHHH and request Huber/White covariances. For this combination, there is no obvious choice for estimating the outer matrix in the sandwich, so the observed information is arbitrarily used as the default.
Lastly you may use the d.f. Adjustment checkbox choose whether to apply a degree-of-free- dom correction to the coefficient covariance. By default, EViews will perform this adjustment.
Examples
In this section, we offer three examples illustrating GLM estimation in EViews.
Exponential Regression
Our first example uses the Kennen (1983) dataset (“Strike.WF1”) on number of strikes (NUMB), industrial production (IP), and dummy variable representing the month of February (FEB). To account for the non-negative response variable NUMB, we may estimate a nonlinear specification of the form:
NUMBi = exp(b1 + b2IPi + b3FEBi) + ei |
(27.3) |
where ei ~ N(0, j2). This model falls into the GLM framework with a log link and normal family. To estimate this specification, bring up the GLM dialog and fill out the equation specification page as follows:
numb c ip feb
then change the Link function to Log. For the moment, we leave the remaining settings and those on the Options page at their default values. Click on OK to accept the specification and estimate the model. EViews displays the following results:
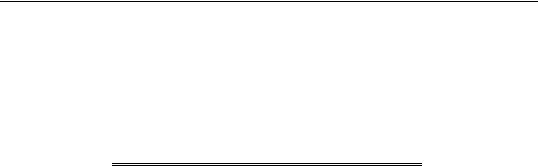
308—Chapter 27. Generalized Linear Models
Dependent Variable: NUMB
Method: Generalized Linear Model (Quadratic Hill Climbing)
Date: 06/15/09 Time: 09:31
Sample: 1 103
Included observations: 103
Family: Normal
Link: Log
Dispersion computed using Pearson Chi-Square
Coefficient covariance computed using observed Hessian
Convergence achieved after 5 iterations
Variable |
Coefficient |
Std. Error |
z-Statistic |
Prob. |
|
|
|
|
|
|
|
|
|
|
C |
1.727368 |
0.066206 |
26.09097 |
0.0000 |
IP |
2.664874 |
1.237904 |
2.152732 |
0.0313 |
FEB |
-0.391015 |
0.313445 |
-1.247476 |
0.2122 |
|
|
|
|
|
|
|
|
|
|
Mean dependent var |
5.495146 |
S.D. dependent var |
3.653829 |
|
Sum squared resid |
1273.783 |
Log likelihood |
|
-275.6964 |
Akaike info criterion |
5.411580 |
Schwarz criterion |
5.488319 |
|
Hannan-Quinn criter. |
5.442662 |
Deviance |
|
1273.783 |
Deviance statistic |
12.73783 |
Restr. deviance |
1361.748 |
|
LR statistic |
6.905754 |
Prob(LR statistic) |
0.031654 |
|
Pearson SSR |
1273.783 |
Pearson statistic |
12.73783 |
|
Dispersion |
12.73783 |
|
|
|
|
|
|
|
|
|
|
|
|
|
The top portion of the output displays the estimation settings and basic results, in particular the choice of algorithm (Quadratic Hill Climbing), distribution family (Normal), and link function (Log), as well as the dispersion estimator, coefficient covariance estimator, and estimation status. We see that the dispersion estimator is based on the Pearson x2 statistic and the coefficient covariance is computed using the inverse of the observed Hessian.
The coefficient estimates indicate that IP is positively related to the number of strikes, and that the relationship is statistically significant at conventional levels. The FEB dummy variable is negatively related to NUMB, but the relationship is not statistically significant.
The bottom portion of the output displays various descriptive statistics. Note that in place of some of the more familiar statistics, EViews reports the deviance, deviance statistic (deviance divided by the degrees-of-freedom) restricted deviance (deviance for the model with only a constant), and the corresponding LR test statistic and probability. The test indicates that the IP and FEB variables are jointly significant at roughly the 3% level. Also displayed are the sum-of-squared Pearson residuals and the estimate of the dispersion, which in this example is the Pearson statistic.
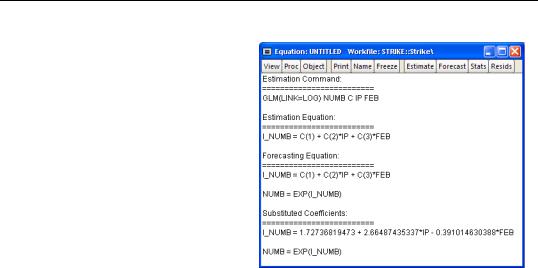
Examples—309
It may be instructive to examine the representations view of this equation. Simply go to the equation toolbar or the main menu and click on
View/Representations to display the view.
Notably, the representations view displays both the specification of the linear predictor (I_NUMB) as well as the mean specification (EXP(I_NUMB)) in terms of the EViews coefficient names, and in terms of the estimated values. These are the expressions used when fore-
casting the index or the dependent variable using the Forecast procedure (see “Forecasting” on page 316).
Binomial
We illustrate the estimation of GLM binomial logistic regression using a simple example from Agresti (2007, Table 3.1, p. 69) examining the relationship between snoring and heart disease. The data in the first page of the workfile “Snoring.WF1” consist of grouped binomial response data for 2,484 subjects divided into four risk factor groups for snoring level (SNORE), coded as 0, 2, 4, 5. Associated with each of the four groups is the number of individuals in the group exhibiting heart disease (DISEASE) as well as a total group size (TOTAL).
SNORE |
DISEASE |
TOTAL |
|
|
|
0 |
24 |
1379 |
|
|
|
2 |
35 |
638 |
|
|
|
4 |
21 |
213 |
|
|
|
5 |
21 |
213 |
|
|
|
We may estimate a logistic regression model for these data in either raw frequency or proportions form.
To estimate the model in raw frequency form, bring up the GLM equation dialog, enter the linear predictor specification:
disease c snore

310—Chapter 27. Generalized Linear Models
select Binomial Count in the Family combo, and enter “TOTAL” in the Number of trials edit field. Next switch over to the Options page and turn off the d.f. Adjustment for the coefficient covariance. Click on OK to estimate the equation.
Dependent Variable: DISEASE
Method: Generalized Linear Model (Quadratic Hill Climbing) Date: 06/15/09 Time: 16:20
Sample: 1 4
Included observations: 4
Family: Binomial Count (n = TOTAL) Link: Logit
Dispersion fixed at 1
Coefficient covariance computed using observed Hessian Summary statistics are for the binomial proportions and implicit
variance weights used in estimation Convergence achieved after 4 iterations
No d.f. adjustment for standard errors & covariance
The output header shows relevant information for the estimation procedure. Note in particular the EViews message that summary statistics are computed for the binomial proportions data. This message is a hint at the fact that EViews estimates the binomial count model by scaling the dependent variable by the number of trials, and estimating the corresponding proportions specification.
Equivalently, you could have specified the model in proportions form. Simply enter the linear predictor specification:
disease/total c snore
with Binomial Proportions specified in the Family combo and “TOTAL” entered in the
Number of trials edit field.
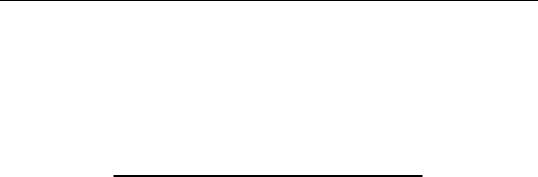
Examples—311
Dependent Variable: DISEASE/TOTAL
Method: Generalized Linear Model (Quadratic Hill Climbing)
Date: 06/15/09 Time: 16:31
Sample: 1 4
Included observations: 4
Family: Binomial Proportion (trials = TOTAL)
Link: Logit
Dispersion fixed at 1
Coefficient covariance computed using observed Hessian
Convergence achieved after 4 iterations
No d.f. adjustment for standard errors & covariance
Variable |
Coefficient |
Std. Error |
z-Statistic |
Prob. |
|
|
|
|
|
|
|
|
|
|
C |
-3.866248 |
0.166214 |
-23.26061 |
0.0000 |
SNORING |
0.397337 |
0.050011 |
7.945039 |
0.0000 |
|
|
|
|
|
|
|
|
|
|
Mean dependent var |
0.023490 |
S.D. dependent var |
0.001736 |
|
Sum squared resid |
0.000357 |
Log likelihood |
|
-11.53073 |
Akaike info criterion |
6.765367 |
Schwarz criterion |
6.458514 |
|
Hannan-Quinn criter. |
6.092001 |
Deviance |
|
2.808912 |
Deviance statistic |
1.404456 |
Restr. deviance |
65.90448 |
|
LR statistic |
63.09557 |
Prob(LR statistic) |
0.000000 |
|
Pearson SSR |
2.874323 |
Pearson statistic |
1.437162 |
|
Dispersion |
1.000000 |
|
|
|
|
|
|
|
|
|
|
|
|
|
The top portion of the output changes to show the different settings, but the remaining output is identical. In particular, there is strong evidence that SNORING is related to heart disease in these data, with the estimated probability of heart disease increasing with the level of snoring.
It is worth mentioning that data of this form are sometimes represented in a frequency weighted form in which the data each group is divided into two records, one for the binomial successes, and one for the failures. Each each record contains the number of repeats in the group and a binary indicator for success (the total number of records is G , where G is the number of groups) The FREQ page of the “Snoring.WF1” workfile contains the data represented in this fashion:
SNORE |
DISEASE |
N |
|
|
|
0 |
1 |
24 |
|
|
|
2 |
1 |
35 |
|
|
|
4 |
1 |
21 |
|
|
|
5 |
1 |
30 |
|
|
|
0 |
0 |
1379 |
|
|
|
2 |
0 |
638 |
|
|
|
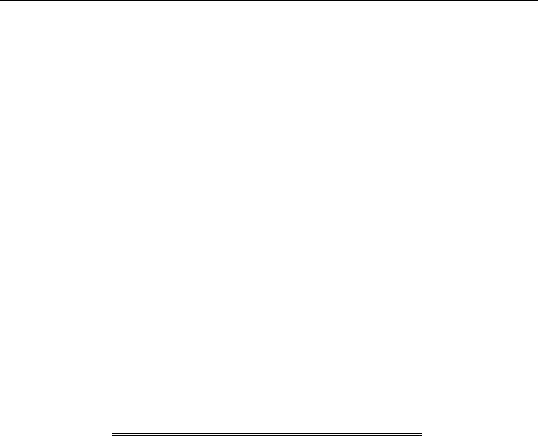
312—Chapter 27. Generalized Linear Models
4 |
0 |
213 |
|
|
|
5 |
0 |
213 |
|
|
|
In this representation, DISEASE is an indicator for whether the record corresponds to individuals with heart disease or not, and N is the number of individuals in the category.
Estimation of the equivalent GLM model specified using the frequency weighted data is straightforward. Simply enter the linear predictor specification:
disease c snore
with either Binomial Proportions or Binomial Count specified in the Family combo. Since each observation corresponds to a binary indicator, you should enter “1” enter as the Number of trials edit field. The multiple individuals in the category are handled by entering “N” in the Frequency weights field in the Options page.
Dependent Variable: DISEASE
Method: Generalized Linear Model (Quadratic Hill Climbing)
Date: 06/16/09 Time: 14:45
Sample: 1 8
Included cases: 8
Total observations: 2484
Family: Binomial Count (n = 1)
Link: Logit
Frequency weight series: N
Dispersion fixed at 1
Coefficient covariance computed using observed Hessian
Convergence achieved after 6 iterations
No d.f. adjustment for standard errors & covariance
Variable |
Coefficient |
Std. Error |
z-Statistic |
Prob. |
|
|
|
|
|
C |
-3.866248 |
0.166214 |
-23.26061 |
0.0000 |
SNORING |
0.397337 |
0.050011 |
7.945039 |
0.0000 |
|
|
|
|
|
|
|
|
|
|
Mean dependent var |
0.044283 |
S.D. dependent var |
0.205765 |
|
Sum squared resid |
102.1917 |
Log likelihood |
|
-418.8658 |
Akaike info criterion |
0.338861 |
Schwarz criterion |
0.343545 |
|
Hannan-Quinn criter. |
0.340562 |
Deviance |
|
837.7316 |
Deviance statistic |
0.337523 |
Restr. deviance |
900.8272 |
|
LR statistic |
63.09557 |
Prob(LR statistic) |
0.000000 |
|
Pearson SSR |
2412.870 |
Pearson statistic |
0.972147 |
|
Dispersion |
1.000000 |
|
|
|
|
|
|
|
|
|
|
|
|
|
Note that while a number of the summary statistics differ due to the different representation of the data (notably the Deviance and Pearson SSRs), the coefficient estimates and LR test statistics in this case are identical to those outlined above. There will, however, be substantive differences between the two results in settings when the dispersion is estimated since the effective number of observations differs in the two settings.

Examples—313
Lastly the data may be represented in individual trial form, which expands observations for each trial in the group into a separate record. The total number of records in the data is Âni , where ni is the number of trials in the i-th (of G ) group. This representation is the traditional ungrouped binary response form for the data. Results for data in this representation should match those for the frequency weighted data.
Binomial Proportions
Papke and Wooldridge (1996) apply GLM techniques to the analysis of fractional response data for 401K tax advantaged savings plan participation rates (“401kjae.WF1”). Their analysis focuses on the relationship between plan participation rates (PRATE) and the employer matching contribution rates (MRATE), accounting for the log of total employment (LOG(TOTEMP), LOG(TOTEMP)^2), plan age (AGE, AGE^2), and a binary indicator for whether the plan is the only pension plan offered by the plan sponsor (SOLE).
We focus on two of the equations estimated in the paper. In both, the authors employ a GLM specification using a binomial proportion family and logit link. Information on the binomial group size ni is ignored, but variance misspecification is accounted for in two ways: first using a binomial QMLE with GLM standard errors, and second using the robust Huber-White covariance approach.
To estimate the GLM standard error specification, we first call up the GLM dialog and enter the linear predictor specification:
prate mprate log(totemp) log(totemp)^2 age age^2 sole
Next, select the Binomial Proportion family, and enter the sample description
@all if mrate<=1
Lastly, we leave the Number of trials edit field at the default value of 1, but correct for heterogeneity by going to the Options page and specifying Pearson Chi-Sq. dispersion estimates. Click on OK to continue.
The resulting estimates correspond the coefficient estimates and first set of standard errors in Papke and Wooldridge (Table II, column 2):
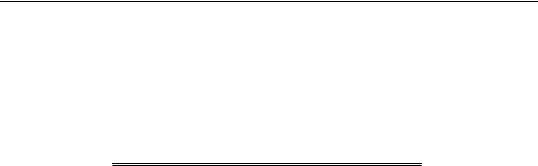
314—Chapter 27. Generalized Linear Models
Dependent Variable: PRATE
Method: Generalized Linear Model (Quadratic Hill Climbing)
Date: 08/12/09 Time: 11:28
Sample: 1 4735 IF MRATE <=1
Included observations: 3784
Family: Binomial Proportion (trials = 1) (quasi-likelihood)
Link: Logit
Dispersion computed using Pearson Chi-Square
Coefficient covariance computed using observed Hessian
Convergence achieved after 8 iterations
Variable |
Coefficient |
Std. Error |
z-Statistic |
Prob. |
|
|
|
|
|
|
|
|
|
|
MRATE |
1.390080 |
0.100368 |
13.84981 |
0.0000 |
LOG(TOTEMP) |
-1.001875 |
0.111222 |
-9.007920 |
0.0000 |
LOG(TOTEMP)^2 |
0.052187 |
0.007105 |
7.345551 |
0.0000 |
AGE |
0.050113 |
0.008710 |
5.753136 |
0.0000 |
AGE^2 |
-0.000515 |
0.000211 |
-2.444532 |
0.0145 |
SOLE |
0.007947 |
0.046785 |
0.169859 |
0.8651 |
C |
5.058001 |
0.426942 |
11.84704 |
0.0000 |
|
|
|
|
|
|
|
|
|
|
Mean dependent var |
0.847769 |
S.D. dependent var |
0.169961 |
|
Sum squared resid |
92.69516 |
Quasi-log likelihood |
-8075.396 |
|
Deviance |
765.0353 |
Deviance statistic |
0.202551 |
|
Restr. deviance |
895.5505 |
Quasi-LR statistic |
680.4838 |
|
Prob(Quasi-LR stat) |
0.000000 |
Pearson SSR |
|
724.4200 |
Pearson statistic |
0.191798 |
Dispersion |
|
0.191798 |
|
|
|
|
|
|
|
|
|
|
Papke and Wooldridge offer a detailed analysis of the results (p. 628-629), which we will not duplicate here. We will point out that the estimate of the dispersion (0.191798) taken from the Pearson statistic is far from the restricted value of 1.0.
The results using the QML with GLM standard errors rely on validity of the GLM assumption for the variance given in Equation (27.2), an assumption that may be too restrictive. We may instead estimate the equation without imposing a particular conditional variance specification by computing our estimates using a robust Huber-White sandwich method. Click on Estimate to bring up the equation dialog, select the Options tab, then change the Covariance method from Default to Huber/White. Click on OK to estimate the revised specification: