
- •Preface
- •Part IV. Basic Single Equation Analysis
- •Chapter 18. Basic Regression Analysis
- •Equation Objects
- •Specifying an Equation in EViews
- •Estimating an Equation in EViews
- •Equation Output
- •Working with Equations
- •Estimation Problems
- •References
- •Chapter 19. Additional Regression Tools
- •Special Equation Expressions
- •Robust Standard Errors
- •Weighted Least Squares
- •Nonlinear Least Squares
- •Stepwise Least Squares Regression
- •References
- •Chapter 20. Instrumental Variables and GMM
- •Background
- •Two-stage Least Squares
- •Nonlinear Two-stage Least Squares
- •Limited Information Maximum Likelihood and K-Class Estimation
- •Generalized Method of Moments
- •IV Diagnostics and Tests
- •References
- •Chapter 21. Time Series Regression
- •Serial Correlation Theory
- •Testing for Serial Correlation
- •Estimating AR Models
- •ARIMA Theory
- •Estimating ARIMA Models
- •ARMA Equation Diagnostics
- •References
- •Chapter 22. Forecasting from an Equation
- •Forecasting from Equations in EViews
- •An Illustration
- •Forecast Basics
- •Forecasts with Lagged Dependent Variables
- •Forecasting with ARMA Errors
- •Forecasting from Equations with Expressions
- •Forecasting with Nonlinear and PDL Specifications
- •References
- •Chapter 23. Specification and Diagnostic Tests
- •Background
- •Coefficient Diagnostics
- •Residual Diagnostics
- •Stability Diagnostics
- •Applications
- •References
- •Part V. Advanced Single Equation Analysis
- •Chapter 24. ARCH and GARCH Estimation
- •Basic ARCH Specifications
- •Estimating ARCH Models in EViews
- •Working with ARCH Models
- •Additional ARCH Models
- •Examples
- •References
- •Chapter 25. Cointegrating Regression
- •Background
- •Estimating a Cointegrating Regression
- •Testing for Cointegration
- •Working with an Equation
- •References
- •Binary Dependent Variable Models
- •Ordered Dependent Variable Models
- •Censored Regression Models
- •Truncated Regression Models
- •Count Models
- •Technical Notes
- •References
- •Chapter 27. Generalized Linear Models
- •Overview
- •How to Estimate a GLM in EViews
- •Examples
- •Working with a GLM Equation
- •Technical Details
- •References
- •Chapter 28. Quantile Regression
- •Estimating Quantile Regression in EViews
- •Views and Procedures
- •Background
- •References
- •Chapter 29. The Log Likelihood (LogL) Object
- •Overview
- •Specification
- •Estimation
- •LogL Views
- •LogL Procs
- •Troubleshooting
- •Limitations
- •Examples
- •References
- •Part VI. Advanced Univariate Analysis
- •Chapter 30. Univariate Time Series Analysis
- •Unit Root Testing
- •Panel Unit Root Test
- •Variance Ratio Test
- •BDS Independence Test
- •References
- •Part VII. Multiple Equation Analysis
- •Chapter 31. System Estimation
- •Background
- •System Estimation Methods
- •How to Create and Specify a System
- •Working With Systems
- •Technical Discussion
- •References
- •Vector Autoregressions (VARs)
- •Estimating a VAR in EViews
- •VAR Estimation Output
- •Views and Procs of a VAR
- •Structural (Identified) VARs
- •Vector Error Correction (VEC) Models
- •A Note on Version Compatibility
- •References
- •Chapter 33. State Space Models and the Kalman Filter
- •Background
- •Specifying a State Space Model in EViews
- •Working with the State Space
- •Converting from Version 3 Sspace
- •Technical Discussion
- •References
- •Chapter 34. Models
- •Overview
- •An Example Model
- •Building a Model
- •Working with the Model Structure
- •Specifying Scenarios
- •Using Add Factors
- •Solving the Model
- •Working with the Model Data
- •References
- •Part VIII. Panel and Pooled Data
- •Chapter 35. Pooled Time Series, Cross-Section Data
- •The Pool Workfile
- •The Pool Object
- •Pooled Data
- •Setting up a Pool Workfile
- •Working with Pooled Data
- •Pooled Estimation
- •References
- •Chapter 36. Working with Panel Data
- •Structuring a Panel Workfile
- •Panel Workfile Display
- •Panel Workfile Information
- •Working with Panel Data
- •Basic Panel Analysis
- •References
- •Chapter 37. Panel Estimation
- •Estimating a Panel Equation
- •Panel Estimation Examples
- •Panel Equation Testing
- •Estimation Background
- •References
- •Part IX. Advanced Multivariate Analysis
- •Chapter 38. Cointegration Testing
- •Johansen Cointegration Test
- •Single-Equation Cointegration Tests
- •Panel Cointegration Testing
- •References
- •Chapter 39. Factor Analysis
- •Creating a Factor Object
- •Rotating Factors
- •Estimating Scores
- •Factor Views
- •Factor Procedures
- •Factor Data Members
- •An Example
- •Background
- •References
- •Appendix B. Estimation and Solution Options
- •Setting Estimation Options
- •Optimization Algorithms
- •Nonlinear Equation Solution Methods
- •References
- •Appendix C. Gradients and Derivatives
- •Gradients
- •Derivatives
- •References
- •Appendix D. Information Criteria
- •Definitions
- •Using Information Criteria as a Guide to Model Selection
- •References
- •Appendix E. Long-run Covariance Estimation
- •Technical Discussion
- •Kernel Function Properties
- •References
- •Index
- •Symbols
- •Numerics
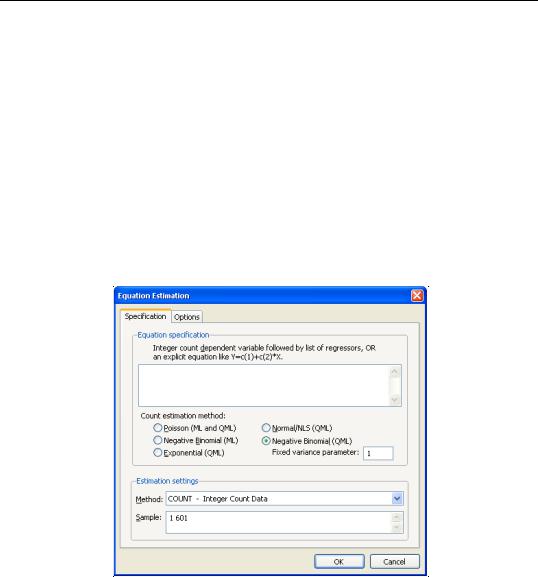
Count Models—287
Count Models
Count models are employed when y takes integer values that represent the number of events that occur—examples of count data include the number of patents filed by a company, and the number of spells of unemployment experienced over a fixed time interval.
EViews provides support for the estimation of several models of count data. In addition to the standard poisson and negative binomial maximum likelihood (ML) specifications, EViews provides a number of quasi-maximum likelihood (QML) estimators for count data.
Estimating Count Models in EViews
To estimate a count data model, select Quick/Estimate Equation… from the main menu, and select COUNT - Integer Count Data as the estimation method. EViews displays the count estimation dialog into which you will enter the dependent and explanatory variable regressors, select a type of count model, and if desired, set estimation options.
There are three parts to the specification of the count model:
•In the upper edit field, you should list the dependent variable and the independent variables or you should provide an explicit expression for the index. The list of explanatory variables specifies a model for the conditional mean of the dependent variable:
m(xi, b) = E(yi |
|
xi, b) = exp(xi¢b). |
(26.38) |
|

288—Chapter 26. Discrete and Limited Dependent Variable Models
•Next, click on Options and, if desired, change the default estimation algorithm, convergence criterion, starting values, and method of computing the coefficient covariance.
•Lastly, select one of the entries listed under count estimation method, and if appropriate, specify a value for the variance parameter. Details for each method are provided in the following discussion.
Poisson Model
For the Poisson model, the conditional density of yi given xi |
is: |
||
f(yi |
|
xi, b) = e–m(xi, b)m(xi, b)yi § yi! |
(26.39) |
|
where yi is a non-negative integer valued random variable. The maximum likelihood estimator (MLE) of the parameter b is obtained by maximizing the log likelihood function:
N |
|
l(b) = Â yilogm(xi, b) – m(xi, b) – log(yi!). |
(26.40) |
i = 1
Provided the conditional mean function is correctly specified and the conditional distribu-
tion of y |
ˆ |
is Poisson, the MLE b is consistent, efficient, and asymptotically normally distrib- |
uted, with coefficient variance matrix consistently estimated by the inverse of the Hessian:
|
|
|
|
ˆ |
|
|
N |
|
|
|
|
|
–1 |
|
|
|
|
|
|
= |
|
 |
m x x ¢ |
|
(26.41) |
||||||
|
|
V = var(b ) |
|
||||||||||||
|
|
|
|
|
|
|
ˆ |
i |
i |
i |
|
|
|||
|
|
|
|
|
|
|
i = 1 |
|
|
|
|
|
|
|
|
ˆ |
= |
ˆ |
|
|
|
|
|
|
|
|
|
|
|
|
|
where mi |
m(xi, b). Alternately, one could estimate the coefficient covariance using the |
||||||||||||||
inverse of the outer-product of the scores: |
|
|
|
|
|
|
|
|
|
|
|||||
|
|
|
ˆ |
|
|
N |
|
|
|
|
|
2 |
|
–1 |
|
|
|
V = |
= |
 |
(y |
|
– m ) |
x x |
¢ |
(26.42) |
|||||
|
|
var(b ) |
|
|
|||||||||||
|
|
|
|
|
|
i |
|
ˆ |
i |
|
i i |
|
|
||
|
|
|
|
|
i |
= 1 |
|
|
|
|
|
|
|
|
|
The Poisson assumption imposes restrictions that are often violated in empirical applications. The most important restriction is the equality of the (conditional) mean and variance:
v(xi, b) = var(yi |
|
xi, b) = E(yi |
|
xi, b) = m(xi, b). |
(26.43) |
|
|
If the mean-variance equality does not hold, the model is misspecified. EViews provides a number of other estimators for count data which relax this restriction.
We note here that the Poisson estimator may also be interpreted as a quasi-maximum likelihood estimator. The implications of this result are discussed below.
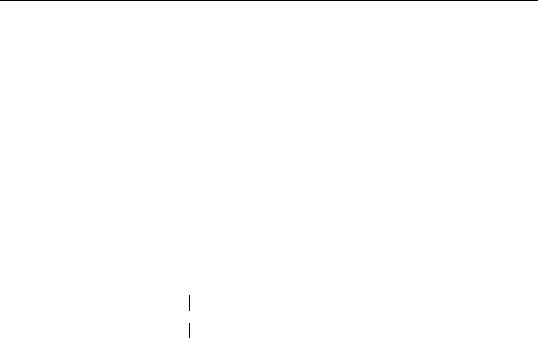
Count Models—289
Negative Binomial (ML)
One common alternative to the Poisson model is to estimate the parameters of the model using maximum likelihood of a negative binomial specification. The log likelihood for the negative binomial distribution is given by:
N |
|
l(b, h) = Â yilog(h2m(xi, b)) – (yi + 1 § h2)log(1 + h2m(xi, b)) |
(26.44) |
i = 1
+ logG(yi + 1 § h2 )–log(yi!)–logG(1 § h2)
where h2 is a variance parameter to be jointly estimated with the conditional mean parameters b . EViews estimates the log of h2 , and labels this parameter as the “SHAPE” parameter in the output. Standard errors are computed using the inverse of the information matrix.
The negative binomial distribution is often used when there is overdispersion in the data, so that v(xi, b) > m(xi, b), since the following moment conditions hold:
E(yi xi, b) = m(xi, b)
(26.45)
var(yi xi, b) = m(xi, b)(1 + h2m(xi, b))
h2 is therefore a measure of the extent to which the conditional variance exceeds the conditional mean.
Consistency and efficiency of the negative binomial ML requires that the conditional distribution of y be negative binomial.
Quasi-maximum Likelihood (QML)
We can perform maximum likelihood estimation under a number of alternative distributional assumptions. These quasi-maximum likelihood (QML) estimators are robust in the sense that they produce consistent estimates of the parameters of a correctly specified conditional mean, even if the distribution is incorrectly specified.
This robustness result is exactly analogous to the situation in ordinary regression, where the normal ML estimator (least squares) is consistent, even if the underlying error distribution is not normally distributed. In ordinary least squares, all that is required for consistency is a correct specification of the conditional mean m(xi, b) = xi¢b . For QML count models, all that is required for consistency is a correct specification of the conditional mean m(xi, b).
The estimated standard errors computed using the inverse of the information matrix will not be consistent unless the conditional distribution of y is correctly specified. However, it is possible to estimate the standard errors in a robust fashion so that we can conduct valid inference, even if the distribution is incorrectly specified.

290—Chapter 26. Discrete and Limited Dependent Variable Models
EViews provides options to compute two types of robust standard errors. Click Options in the Equation Specification dialog box and mark the Robust Covariance option. The Huber/ White option computes QML standard errors, while the GLM option computes standard errors corrected for overdispersion. See “Technical Notes” on page 296 for details on these options.
Further details on QML estimation are provided by Gourioux, Monfort, and Trognon (1994a, 1994b). Wooldridge (1997) provides an excellent summary of the use of QML techniques in estimating parameters of count models. See also the extensive related literature on Generalized Linear Models (McCullagh and Nelder, 1989).
Poisson
The Poisson MLE is also a QMLE for data from alternative distributions. Provided that the conditional mean is correctly specified, it will yield consistent estimates of the parameters b of the mean function. By default, EViews reports the ML standard errors. If you wish to compute the QML standard errors, you should click on Options, select Robust Covariances, and select the desired covariance matrix estimator.
Exponential
The log likelihood for the exponential distribution is given by:
N |
|
l(b) = Â – logm(xi, b) – yi § m(xi, b). |
(26.46) |
i = 1
As with the other QML estimators, the exponential QMLE is consistent even if the conditional distribution of yi is not exponential, provided that mi is correctly specified. By default, EViews reports the robust QML standard errors.
Normal
The log likelihood for the normal distribution is:
N |
|
1 yi – m(xi, b) 2 |
|
1 |
2 |
|
1 |
|
|||
l(b) = Â |
– |
– |
) – |
(26.47) |
|||||||
2 |
j |
|
2log(j |
|
2log(2p). |
||||||
|
|
-- |
------------------------------- |
|
-- |
|
|
-- |
|
i = 1
For fixed j2 and correctly specified mi , maximizing the normal log likelihood function provides consistent estimates even if the distribution is not normal. Note that maximizing the normal log likelihood for a fixed j2 is equivalent to minimizing the sum of squares for the nonlinear regression model:
yi = m(xi, b) + ei . |
(26.48) |
EViews sets j2 = 1 by default. You may specify any other (positive) value for j2 |
by |
changing the number in the Fixed variance parameter field box. By default, EViews reports the robust QML standard errors when estimating this specification.

Count Models—291
Negative Binomial
If we maximize the negative binomial log likelihood, given above, for fixed h2 , we obtain the QMLE of the conditional mean parameters b . This QML estimator is consistent even if the conditional distribution of y is not negative binomial, provided that mi is correctly specified.
EViews sets h2 = 1 by default, which is a special case known as the geometric distribution. You may specify any other (positive) value by changing the number in the Fixed variance parameter field box. For the negative binomial QMLE, EViews by default reports the robust QMLE standard errors.
Views of Count Models
EViews provides a full complement of views of count models. You can examine the estimation output, compute frequencies for the dependent variable, view the covariance matrix, or perform coefficient tests. Additionally, you can select View/Actual, Fitted, Residual… and
pick from a number of views describing the ordinary residuals eoi = |
ˆ |
yi – m(xi, b), or you |
can examine the correlogram and histogram of these residuals. For the most part, all of these views are self-explanatory.
Note, however, that the LR test statistics presented in the summary statistics at the bottom of the equation output, or as computed under the View/Coefficient Diagnostics/Redundant Variables - Likelihood Ratio… have a known asymptotic distribution only if the conditional distribution is correctly specified. Under the weaker GLM assumption that the true variance is proportional to the nominal variance, we can form a quasi-likelihood ratio, QLR = LR § jˆ 2 , where jˆ 2 is the estimated proportional variance factor. This QLR statistic has an asymptotic x2 distribution under the assumption that the mean is correctly specified and that the variances follow the GLM structure. EViews does not compute the QLR statistic, but it can be estimated by computing an estimate of jˆ 2 based upon the standardized residuals. We provide an example of the use of the QLR test statistic below.
If the GLM assumption does not hold, then there is no usable QLR test statistic with a known distribution; see Wooldridge (1997).
Procedures for Count Models
Most of the procedures are self-explanatory. Some details are required for the forecasting and residual creation procedures.
• Forecast… provides you the option to forecast the dependent variable yi |
or the pre- |
|||
|
ˆ |
|
|
yi are |
dicted linear index xi¢b . Note that for all of these models the forecasts of |
||||
given by yi |
= m(xi, b) |
where m(xi, b) = |
exp(xi¢b). |
|
ˆ |
ˆ |
ˆ |
ˆ |
|
|
|
|
|
•Make Residual Series… provides the following three types of residuals for count models:
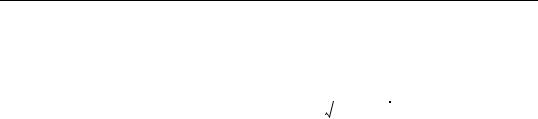
292—Chapter 26. Discrete and Limited Dependent Variable Models
Ordinary |
eoi |
= |
|
ˆ |
|
yi–m(xi, b) |
|||
Standardized (Pearson) |
|
|
|
ˆ |
|
esi |
= |
yi–m(xi, b) |
|
|
----------------------------- |
|||
|
|
|
ˆ |
ˆ |
|
|
|
v(xi, b, g) |
|
Generalized |
|
eg =(varies) |
|
|
|
|
|
||
|
|
|
|
|
where the g represents any additional parameters in the variance specification. Note that the specification of the variances may vary significantly between specifications.
|
|
|
ˆ |
= |
ˆ |
For example, the Poisson model has v(xi, b) |
m(xi, b), while the exponential has |
||||
ˆ |
= |
ˆ |
2 |
|
|
v(xi, b) |
m(xi, b) |
. |
|
|
The generalized residuals can be used to obtain the score vector by multiplying the generalized residuals by each variable in x . These scores can be used in a variety of LM or conditional moment tests for specification testing; see Wooldridge (1997).
Demonstrations
A Specification Test for Overdispersion
Consider the model:
NUMBi = b1 + b2IPi + b3FEBi + ei , |
(26.49) |
where the dependent variable NUMB is the number of strikes, IP is a measure of industrial production, and FEB is a February dummy variable, as reported in Kennan (1985, Table 1) and provided in the workfile “Strike.WF1”.
The results from Poisson estimation of this model are presented below:
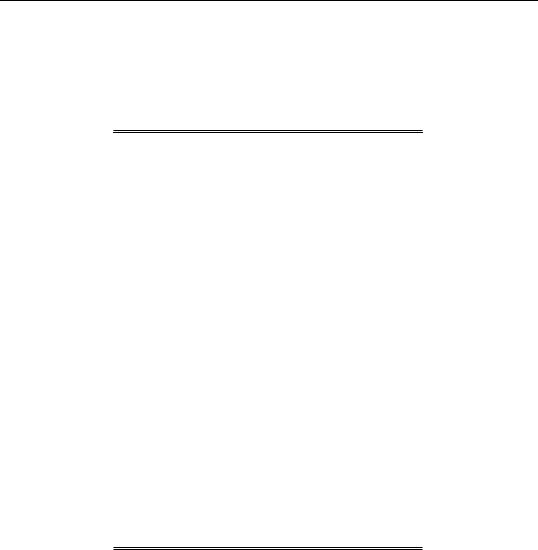
Count Models—293
Dependent Variable: NUMB
Method: ML/QML - Poisson Count (Quadratic hill climbing)
Date: 08/12/09 Time: 09:55
Sample: 1 103
Included observations: 103
Convergence achieved after 4 iterations
Covariance matrix computed using second derivatives
Variable |
Coefficient |
Std. Error |
z-Statistic |
Prob. |
|
|
|
|
|
|
|
|
|
|
C |
1.725630 |
0.043656 |
39.52764 |
0.0000 |
IP |
2.775334 |
0.819104 |
3.388254 |
0.0007 |
FEB |
-0.377407 |
0.174520 |
-2.162540 |
0.0306 |
|
|
|
|
|
|
|
|
|
|
R-squared |
0.064502 |
Mean dependent var |
5.495146 |
|
Adjusted R-squared |
0.045792 |
S.D. dependent var |
3.653829 |
|
S.E. of regression |
3.569190 |
Akaike info criterion |
5.583421 |
|
Sum squared resid |
1273.912 |
Schwarz criterion |
5.660160 |
|
Log likelihood |
-284.5462 |
Hannan-Quinn criter. |
5.614503 |
|
Restr. log likelihood |
-292.9694 |
LR statistic |
|
16.84645 |
Avg. log likelihood |
-2.762584 |
Prob(LR statistic) |
0.000220 |
|
|
|
|
|
|
|
|
|
|
|
Cameron and Trivedi (1990) propose a regression based test of the Poisson restriction
v(xi, b) = m(xi, b). To carry out the test, first estimate the Poisson model and obtain the fitted values of the dependent variable. Click Forecast and provide a name for the forecasted dependent variable, say NUMB_F. The test is based on an auxiliary regression of e2oi – yi on yˆ 2i and testing the significance of the regression coefficient. For this example, the test regression can be estimated by the command:
equation testeq.ls (numb-numb_f)^2-numb numb_f^2
yielding the following results:
Dependent Variable: (NUMB-NUMB_F)^2-NUMB
Method: Least Squares
Date: 08/12/09 Time: 09:57
Sample: 1 103
Included observations: 103
Variable |
Coefficient |
Std. Error |
t-Statistic |
Prob. |
|
|
|
|
|
|
|
|
|
|
NUMB_F^2 |
0.238874 |
0.052115 |
4.583571 |
0.0000 |
|
|
|
|
|
|
|
|
|
|
R-squared |
0.043930 |
Mean dependent var |
6.872929 |
|
Adjusted R-squared |
0.043930 |
S.D. dependent var |
17.65726 |
|
S.E. of regression |
17.26506 |
Akaike info criterion |
8.544908 |
|
Sum squared resid |
30404.41 |
Schwarz criterion |
8.570488 |
|
Log likelihood |
-439.0628 |
Hannan-Quinn criter. |
8.555269 |
|
Durbin-Watson stat |
1.711805 |
|
|
|
|
|
|
|
|
|
|
|
|
|
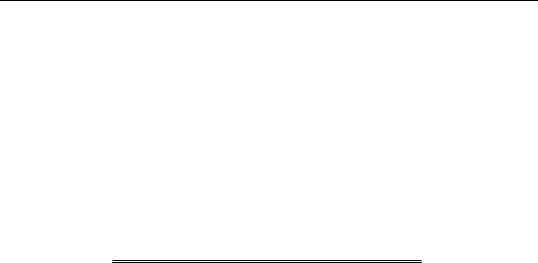
294—Chapter 26. Discrete and Limited Dependent Variable Models
The t-statistic of the coefficient is highly significant, leading us to reject the Poisson restriction. Moreover, the estimated coefficient is significantly positive, indicating overdispersion in the residuals.
An alternative approach, suggested by Wooldridge (1997), is to regress esi – 1 , on yˆ i . To perform this test, select Proc/Make Residual Series… and select Standardized. Save the results in a series, say SRESID. Then estimating the regression specification:
sresid^2-1 numbf
yields the results:
Dependent Variable: SRESID^2-1
Method: Least Squares
Date: 08/12/09 Time: 10:55
Sample: 1 103
Included observations: 103
Variable |
Coefficient |
Std. Error |
t-Statistic |
Prob. |
|
|
|
|
|
|
|
|
|
|
NUMB_F |
0.221238 |
0.055002 |
4.022326 |
0.0001 |
|
|
|
|
|
|
|
|
|
|
R-squared |
0.017556 |
Mean dependent var |
1.161573 |
|
Adjusted R-squared |
0.017556 |
S.D. dependent var |
3.138974 |
|
S.E. of regression |
3.111299 |
Akaike info criterion |
5.117619 |
|
Sum squared resid |
987.3785 |
Schwarz criterion |
5.143199 |
|
Log likelihood |
-262.5574 |
Hannan-Quinn criter. |
5.127980 |
|
Durbin-Watson stat |
1.764537 |
|
|
|
|
|
|
|
|
|
|
|
|
|
Both tests suggest the presence of overdispersion, with the variance approximated by roughly v = m(1 + 0.23m).
Given the evidence of overdispersion and the rejection of the Poisson restriction, we will reestimate the model, allowing for mean-variance inequality. Our approach will be to estimate the two-step negative binomial QMLE specification (termed the quasi-generalized pseudomaximum likelihood estimator by Gourieroux, Monfort, and Trognon (1984a, b)) using the estimate of hˆ 2 from the Wooldridge test derived above. To compute this estimator, simply select Negative Binomial (QML) and enter “0.22124” in the edit field for Fixed variance parameter.
We will use the GLM variance calculations, so you should click on Option in the Equation Specification dialog and mark the Robust Covariance and GLM options. The estimation results are shown below:
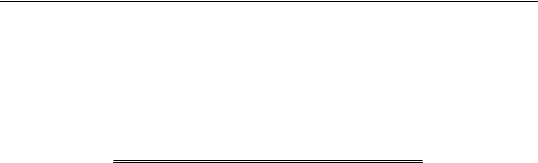
Count Models—295
Dependent Variable: NUMB
Method: QML - Negative Binomial Count (Quadratic hill climbing)
Date: 08/12/09 Time: 10:55
Sample: 1 103
Included observations: 103
QML parameter used in estimation: 0.22124
Convergence achieved after 4 iterations
GLM Robust Standard Errors & Covariance
Variance factor estimate = 0.989996509662
Covariance matrix computed using second derivatives
Variable |
Coefficient |
Std. Error |
z-Statistic |
Prob. |
|
|
|
|
|
|
|
|
|
|
C |
1.724906 |
0.064976 |
26.54671 |
0.0000 |
IP |
2.833103 |
1.216260 |
2.329356 |
0.0198 |
FEB |
-0.369558 |
0.239125 |
-1.545463 |
0.1222 |
|
|
|
|
|
|
|
|
|
|
R-squared |
0.064374 |
Mean dependent var |
5.495146 |
|
Adjusted R-squared |
0.045661 |
S.D. dependent var |
3.653829 |
|
S.E. of regression |
3.569435 |
Akaike info criterion |
5.174385 |
|
Sum squared resid |
1274.087 |
Schwarz criterion |
5.251125 |
|
Log likelihood |
-263.4808 |
Hannan-Quinn criter. |
5.205468 |
|
Restr. log likelihood |
-522.9973 |
LR statistic |
|
519.0330 |
Avg. log likelihood |
-2.558066 |
Prob(LR statistic) |
0.000000 |
|
|
|
|
|
|
|
|
|
|
|
The negative binomial QML should be consistent, and under the GLM assumption, the standard errors should be consistently estimated. It is worth noting that the coefficient on FEB, which was strongly statistically significant in the Poisson specification, is no longer significantly different from zero at conventional significance levels.
Quasi-likelihood Ratio Statistic
As described by Wooldridge (1997), specification testing using likelihood ratio statistics requires some care when based upon QML models. We illustrate here the differences between a standard LR test for significant coefficients and the corresponding QLR statistic.
From the results above, we know that the overall likelihood ratio statistic for the Poisson model is 16.85, with a corresponding p-value of 0.0002. This statistic is valid under the assumption that m(xi, b) is specified correctly and that the mean-variance equality holds.
We can decisively reject the latter hypothesis, suggesting that we should derive the QML estimator with consistently estimated covariance matrix under the GLM variance assumption. While EViews currently does not automatically adjust the LR statistic to reflect the QML assumption, it is easy enough to compute the adjustment by hand. Following Wooldridge, we construct the QLR statistic by dividing the original LR statistic by the estimated GLM variance factor. (Alternately, you may use the GLM estimators for count models described in Chapter 27. “Generalized Linear Models,” on page 301, which do compute the QLR statistics automatically.)