
- •Preface
- •Part IV. Basic Single Equation Analysis
- •Chapter 18. Basic Regression Analysis
- •Equation Objects
- •Specifying an Equation in EViews
- •Estimating an Equation in EViews
- •Equation Output
- •Working with Equations
- •Estimation Problems
- •References
- •Chapter 19. Additional Regression Tools
- •Special Equation Expressions
- •Robust Standard Errors
- •Weighted Least Squares
- •Nonlinear Least Squares
- •Stepwise Least Squares Regression
- •References
- •Chapter 20. Instrumental Variables and GMM
- •Background
- •Two-stage Least Squares
- •Nonlinear Two-stage Least Squares
- •Limited Information Maximum Likelihood and K-Class Estimation
- •Generalized Method of Moments
- •IV Diagnostics and Tests
- •References
- •Chapter 21. Time Series Regression
- •Serial Correlation Theory
- •Testing for Serial Correlation
- •Estimating AR Models
- •ARIMA Theory
- •Estimating ARIMA Models
- •ARMA Equation Diagnostics
- •References
- •Chapter 22. Forecasting from an Equation
- •Forecasting from Equations in EViews
- •An Illustration
- •Forecast Basics
- •Forecasts with Lagged Dependent Variables
- •Forecasting with ARMA Errors
- •Forecasting from Equations with Expressions
- •Forecasting with Nonlinear and PDL Specifications
- •References
- •Chapter 23. Specification and Diagnostic Tests
- •Background
- •Coefficient Diagnostics
- •Residual Diagnostics
- •Stability Diagnostics
- •Applications
- •References
- •Part V. Advanced Single Equation Analysis
- •Chapter 24. ARCH and GARCH Estimation
- •Basic ARCH Specifications
- •Estimating ARCH Models in EViews
- •Working with ARCH Models
- •Additional ARCH Models
- •Examples
- •References
- •Chapter 25. Cointegrating Regression
- •Background
- •Estimating a Cointegrating Regression
- •Testing for Cointegration
- •Working with an Equation
- •References
- •Binary Dependent Variable Models
- •Ordered Dependent Variable Models
- •Censored Regression Models
- •Truncated Regression Models
- •Count Models
- •Technical Notes
- •References
- •Chapter 27. Generalized Linear Models
- •Overview
- •How to Estimate a GLM in EViews
- •Examples
- •Working with a GLM Equation
- •Technical Details
- •References
- •Chapter 28. Quantile Regression
- •Estimating Quantile Regression in EViews
- •Views and Procedures
- •Background
- •References
- •Chapter 29. The Log Likelihood (LogL) Object
- •Overview
- •Specification
- •Estimation
- •LogL Views
- •LogL Procs
- •Troubleshooting
- •Limitations
- •Examples
- •References
- •Part VI. Advanced Univariate Analysis
- •Chapter 30. Univariate Time Series Analysis
- •Unit Root Testing
- •Panel Unit Root Test
- •Variance Ratio Test
- •BDS Independence Test
- •References
- •Part VII. Multiple Equation Analysis
- •Chapter 31. System Estimation
- •Background
- •System Estimation Methods
- •How to Create and Specify a System
- •Working With Systems
- •Technical Discussion
- •References
- •Vector Autoregressions (VARs)
- •Estimating a VAR in EViews
- •VAR Estimation Output
- •Views and Procs of a VAR
- •Structural (Identified) VARs
- •Vector Error Correction (VEC) Models
- •A Note on Version Compatibility
- •References
- •Chapter 33. State Space Models and the Kalman Filter
- •Background
- •Specifying a State Space Model in EViews
- •Working with the State Space
- •Converting from Version 3 Sspace
- •Technical Discussion
- •References
- •Chapter 34. Models
- •Overview
- •An Example Model
- •Building a Model
- •Working with the Model Structure
- •Specifying Scenarios
- •Using Add Factors
- •Solving the Model
- •Working with the Model Data
- •References
- •Part VIII. Panel and Pooled Data
- •Chapter 35. Pooled Time Series, Cross-Section Data
- •The Pool Workfile
- •The Pool Object
- •Pooled Data
- •Setting up a Pool Workfile
- •Working with Pooled Data
- •Pooled Estimation
- •References
- •Chapter 36. Working with Panel Data
- •Structuring a Panel Workfile
- •Panel Workfile Display
- •Panel Workfile Information
- •Working with Panel Data
- •Basic Panel Analysis
- •References
- •Chapter 37. Panel Estimation
- •Estimating a Panel Equation
- •Panel Estimation Examples
- •Panel Equation Testing
- •Estimation Background
- •References
- •Part IX. Advanced Multivariate Analysis
- •Chapter 38. Cointegration Testing
- •Johansen Cointegration Test
- •Single-Equation Cointegration Tests
- •Panel Cointegration Testing
- •References
- •Chapter 39. Factor Analysis
- •Creating a Factor Object
- •Rotating Factors
- •Estimating Scores
- •Factor Views
- •Factor Procedures
- •Factor Data Members
- •An Example
- •Background
- •References
- •Appendix B. Estimation and Solution Options
- •Setting Estimation Options
- •Optimization Algorithms
- •Nonlinear Equation Solution Methods
- •References
- •Appendix C. Gradients and Derivatives
- •Gradients
- •Derivatives
- •References
- •Appendix D. Information Criteria
- •Definitions
- •Using Information Criteria as a Guide to Model Selection
- •References
- •Appendix E. Long-run Covariance Estimation
- •Technical Discussion
- •Kernel Function Properties
- •References
- •Index
- •Symbols
- •Numerics
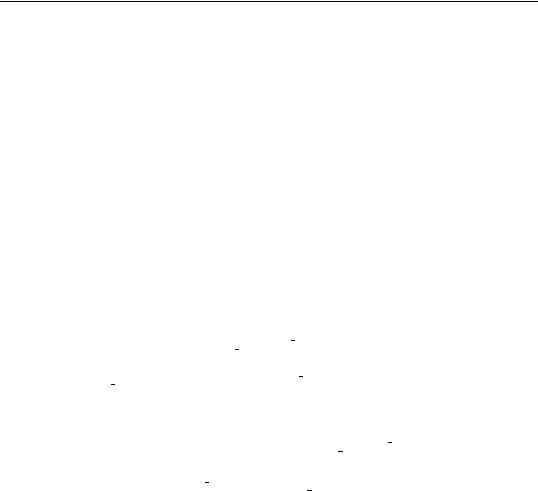
Truncated Regression Models—283
Double click on the scalar name to display the value in the status line at the bottom of the EViews window. For the example data set, the p-value is 0.066, which rejects the tobit model at the 10% level, but not at the 5% level.
For other specification tests for the tobit, see Greene (2008, 23.3.4) or Pagan and Vella (1989).
Truncated Regression Models
A close relative of the censored regression model is the truncated regression model. Suppose that an observation is not observed whenever the dependent variable falls below one threshold, or exceeds a second threshold. This sampling rule occurs, for example, in earnings function studies for low-income families that exclude observations with incomes above a threshold, and in studies of durables demand among individuals who purchase durables.
The general two-limit truncated regression model may be written as: |
|
yi = xi¢b + jei |
(26.32) |
where yi = yi is only observed if: |
|
ci < yi < ci . |
(26.33) |
If there is no lower truncation, then we can set ci = –•. If there is no upper truncation, then we set ci = •.
The log likelihood function associated with these data is given by:
N |
|
l(b, j) = Â logf((yi – xi¢b) § j) 1(ci < yi < ci) |
(26.34) |
i = 1
N
–Â log(F((ci – xi¢b) § j)–F((ci – xi¢b) § j)).
i= 1
The likelihood function is maximized with respect to b and j , using standard iterative methods.
Estimating a Truncated Model in EViews
Estimation of a truncated regression model follows the same steps as estimating a censored regression:
•Select Quick/Estimate Equation… from the main menu, and in the Equation Specification dialog, select the CENSORED estimation method. The censored and truncated regression dialog will appear.
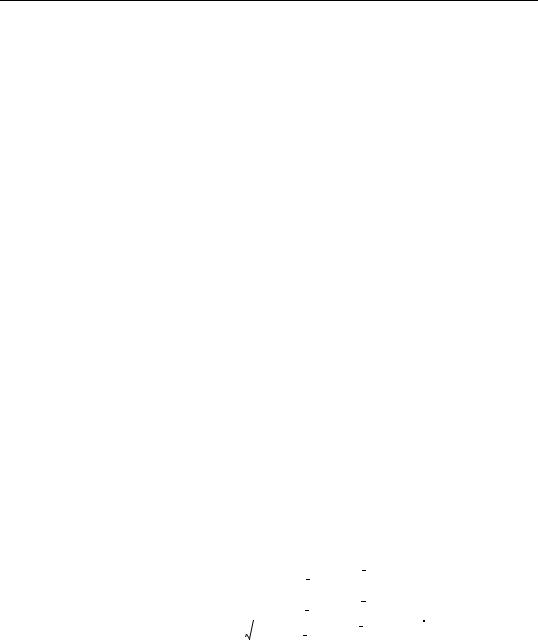
284—Chapter 26. Discrete and Limited Dependent Variable Models
•Enter the name of the truncated dependent variable and the list of the regressors or provide explicit expression for the equation in the Equation Specification field, and select one of the three distributions for the error term.
•Indicate that you wish to estimate the truncated model by checking the Truncated sample option.
•Specify the truncation points of the dependent variable by entering the appropriate expressions in the two edit fields. If you leave an edit field blank, EViews will assume that there is no truncation along that dimension.
You should keep a few points in mind. First, truncated estimation is only available for models where the truncation points are known, since the likelihood function is not otherwise defined. If you attempt to specify your truncation points by index, EViews will issue an error message indicating that this selection is not available.
Second, EViews will issue an error message if any values of the dependent variable are outside the truncation points. Furthermore, EViews will automatically exclude any observations that are exactly equal to a truncation point. Thus, if you specify zero as the lower truncation limit, EViews will issue an error message if any observations are less than zero, and will exclude any observations where the dependent variable exactly equals zero.
The cumulative distribution function and density of the assumed distribution will be used to form the likelihood function, as described above.
Procedures for Truncated Equations
EViews provides the same procedures for truncated equations as for censored equations. The residual and forecast calculations differ to reflect the truncated dependent variable and the different likelihood function.
Make Residual Series
Select Proc/Make Residual Series, and select from among the three types of residuals. The three types of residuals for censored models are defined as:
Ordinary |
eoi |
= |
yi – E(yi |
|
|
|
|
ˆ ˆ |
|
|
|
||||||||
|
|
|
ci < yi |
< ci ; xi, b, j) |
|||||
|
|
|
|
|
|
|
|
|
|
Standardized |
|
|
yi – E(yi |
|
|
ci < yi |
|
ˆ ˆ |
|
|
|
|
|||||||
|
esi |
= |
|
|
|
< ci ; xi, b, j) |
|||
|
----------------------------------------------------------------------------------- |
|
ci < yi |
|
ˆ ˆ - |
||||
|
|
|
|
||||||
|
|
|
var(yi |
|
|
|
|
< ci ; xi, b, j) |
|
|
|
|
|
|
|
|
|
|
|
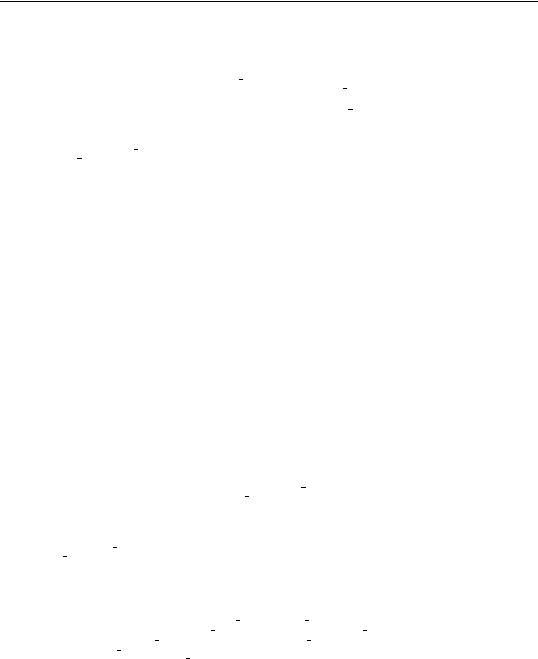
Truncated Regression Models—285
Generalized |
|
|
f¢((yi – xi¢b) § j) |
|
|||
egi |
= – |
|
ˆ |
ˆ |
|
||
|
|
ˆ |
ˆ |
|
|||
|
|
f |
jf((yi – xi¢b) § j) |
|
|||
|
|
((ci – xi¢b) § j) – f((ci – xi¢b) § j) |
|||||
|
– |
|
ˆ |
ˆ |
|
ˆ |
ˆ |
|
-------------- |
----------------------------------------------------------------------------------- |
ˆ |
ˆ |
ˆ |
ˆ |
|
|
|
|
|
||||
|
|
j(F((ci – xi¢b) § |
j)–F((ci – xi¢b) § j)) |
where f , F , are the density and distribution functions. Details on the computation of
E(yi |
|
|
ˆ ˆ |
|
|||
|
ci < yi |
< ci; xi, b, j) are provided below. |
The generalized residuals may be used as the basis of a number of LM tests, including LM tests of normality (see Chesher and Irish (1984, 1987), and Gourieroux, Monfort and Trognon (1987); Greene (2008) provides a brief discussion and additional references).
Forecasting
EViews provides you with the option of forecasting the expected observed dependent vari-
able, E(yi |
|
ˆ |
ˆ |
E(yi |
|
|
ˆ |
ˆ |
|
|
|||||||
|
||||||||
|
xi, b, j), or the expected latent variable, |
|
|
xi, b, j). |
To forecast the expected latent variable, select Forecast from the equation toolbar to open the forecast dialog, click on Index - Expected latent variable, and enter a name for the
series to hold the output. The forecasts of the expected latent variable E(yi |
|
|
ˆ |
ˆ |
|||||||
|
|||||||||||
|
|
xi, b, j) are |
|||||||||
computed using: |
|
|
ˆ |
|
= |
ˆ |
|
|
|
|
|
ˆ |
|
ˆ |
ˆ |
|
|
|
(26.35) |
||||
|
|
|
|
||||||||
yi = |
E(yi |
|
xi, b, j) |
xi¢b – jg . |
|
|
|
where g is the Euler-Mascheroni constant (g ª 0.5772156649 ).
To forecast the expected observed dependent variable for the truncated model, you should select Expected dependent variable, and enter a series name. These forecasts are computed using:
ˆ |
= E(yi |
|
|
ci < yi |
|
ˆ ˆ |
(26.36) |
|
|||||||
yi |
|
|
|
< ci; xi, b, j) |
so that the expectations for the latent variable are taken with respect to the conditional (on being observed) distribution of the yi . Note that these forecasts always satisfy the inequality ci < yˆ i < ci .
It is instructive to compare this latter expected value with the expected value derived for the censored model in Equation (26.30) above (repeated here for convenience):
yi = |
E(yi |
|
ˆ |
|
||
|
|
|||||
|
xi, b, |
|
||||
ˆ |
|
|
|
|
|
|
+ E(yi |
|
ci < yi |
|
|||
|
|
|||||
+ ci Pr |
|
(yi = ci |
|
|||
|
|
ˆ |
ci Pr(yi = ci |
|
ˆ |
ˆ |
|
|
(26.37) |
|
|
|
|||||
j) = |
xi, b, j) |
|
|
||||
< ci; |
ˆ ˆ |
|
|
< ci |
|
ˆ |
ˆ |
|
|
||||||
xi, b, j) Pr(ci < yi |
|
|
xi, b, j) |
||||
ˆ |
ˆ |
|
|
|
|
|
|
xi, b, j). |
|
|
|
|
|
|
The expected value of the dependent variable for the truncated model is the first part of the middle term of the censored expected value. The differences between the two expected values (the probability weight and the first and third terms) reflect the different treatment of
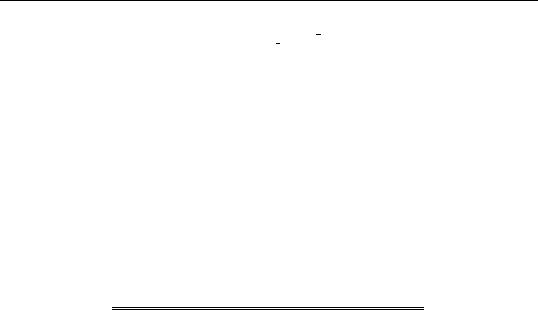
286—Chapter 26. Discrete and Limited Dependent Variable Models
latent observations that do not lie between ci and ci . In the censored case, those observations are included in the sample and are accounted for in the expected value. In the truncated case, data outside the interval are not observed and are not used in the expected value computation.
An Illustration
As an example, we reestimate the Fair tobit model from above, truncating the data so that observations at or below zero are removed from the sample. The output from truncated estimation of the Fair model is presented below:
Dependent Variable: Y_PT
Method: ML - Censored Normal (TOBIT) (Quadratic hill climbing)
Date: 08/12/09 Time: 00:43
Sample (adjusted): 452 601
Included observations: 150 after adjustments
Truncated sample
Left censoring (value) at zero
Convergence achieved after 8 iterations
Covariance matrix computed using second derivatives
Variable |
Coefficient |
Std. Error |
z-Statistic |
Prob. |
|
|
|
|
|
|
|
|
|
|
C |
12.37287 |
5.178533 |
2.389261 |
0.0169 |
Z1 |
-1.336854 |
1.451426 |
-0.921063 |
0.3570 |
Z2 |
-0.044791 |
0.116125 |
-0.385719 |
0.6997 |
Z3 |
0.544174 |
0.217885 |
2.497527 |
0.0125 |
Z4 |
-2.142868 |
1.784389 |
-1.200897 |
0.2298 |
Z5 |
-1.423107 |
0.594582 |
-2.393459 |
0.0167 |
Z6 |
-0.316717 |
0.321882 |
-0.983953 |
0.3251 |
Z7 |
0.621418 |
0.477420 |
1.301618 |
0.1930 |
Z8 |
-1.210020 |
0.547810 |
-2.208833 |
0.0272 |
|
|
|
|
|
|
|
|
|
|
|
Error Distribution |
|
|
|
|
|
|
|
|
|
|
|
|
|
SCALE:C(10) |
5.379485 |
0.623787 |
8.623910 |
0.0000 |
|
|
|
|
|
|
|
|
|
|
Mean dependent var |
5.833333 |
S.D. dependent var |
4.255934 |
|
S.E. of regression |
3.998870 |
Akaike info criterion |
5.344456 |
|
Sum squared resid |
2254.725 |
Schwarz criterion |
5.545165 |
|
Log likelihood |
-390.8342 |
Hannan-Quinn criter. |
5.425998 |
|
Avg. log likelihood |
-2.605561 |
|
|
|
|
|
|
|
|
Left censored obs |
0 |
Right censored obs |
0 |
|
Uncensored obs |
150 |
Total obs |
|
150 |
|
|
|
|
|
|
|
|
|
|
Note that the header information indicates that the model is a truncated specification with a sample that is adjusted accordingly, and that the frequency information at the bottom of the screen shows that there are no left and right censored observations.