
- •Preface
- •Part IV. Basic Single Equation Analysis
- •Chapter 18. Basic Regression Analysis
- •Equation Objects
- •Specifying an Equation in EViews
- •Estimating an Equation in EViews
- •Equation Output
- •Working with Equations
- •Estimation Problems
- •References
- •Chapter 19. Additional Regression Tools
- •Special Equation Expressions
- •Robust Standard Errors
- •Weighted Least Squares
- •Nonlinear Least Squares
- •Stepwise Least Squares Regression
- •References
- •Chapter 20. Instrumental Variables and GMM
- •Background
- •Two-stage Least Squares
- •Nonlinear Two-stage Least Squares
- •Limited Information Maximum Likelihood and K-Class Estimation
- •Generalized Method of Moments
- •IV Diagnostics and Tests
- •References
- •Chapter 21. Time Series Regression
- •Serial Correlation Theory
- •Testing for Serial Correlation
- •Estimating AR Models
- •ARIMA Theory
- •Estimating ARIMA Models
- •ARMA Equation Diagnostics
- •References
- •Chapter 22. Forecasting from an Equation
- •Forecasting from Equations in EViews
- •An Illustration
- •Forecast Basics
- •Forecasts with Lagged Dependent Variables
- •Forecasting with ARMA Errors
- •Forecasting from Equations with Expressions
- •Forecasting with Nonlinear and PDL Specifications
- •References
- •Chapter 23. Specification and Diagnostic Tests
- •Background
- •Coefficient Diagnostics
- •Residual Diagnostics
- •Stability Diagnostics
- •Applications
- •References
- •Part V. Advanced Single Equation Analysis
- •Chapter 24. ARCH and GARCH Estimation
- •Basic ARCH Specifications
- •Estimating ARCH Models in EViews
- •Working with ARCH Models
- •Additional ARCH Models
- •Examples
- •References
- •Chapter 25. Cointegrating Regression
- •Background
- •Estimating a Cointegrating Regression
- •Testing for Cointegration
- •Working with an Equation
- •References
- •Binary Dependent Variable Models
- •Ordered Dependent Variable Models
- •Censored Regression Models
- •Truncated Regression Models
- •Count Models
- •Technical Notes
- •References
- •Chapter 27. Generalized Linear Models
- •Overview
- •How to Estimate a GLM in EViews
- •Examples
- •Working with a GLM Equation
- •Technical Details
- •References
- •Chapter 28. Quantile Regression
- •Estimating Quantile Regression in EViews
- •Views and Procedures
- •Background
- •References
- •Chapter 29. The Log Likelihood (LogL) Object
- •Overview
- •Specification
- •Estimation
- •LogL Views
- •LogL Procs
- •Troubleshooting
- •Limitations
- •Examples
- •References
- •Part VI. Advanced Univariate Analysis
- •Chapter 30. Univariate Time Series Analysis
- •Unit Root Testing
- •Panel Unit Root Test
- •Variance Ratio Test
- •BDS Independence Test
- •References
- •Part VII. Multiple Equation Analysis
- •Chapter 31. System Estimation
- •Background
- •System Estimation Methods
- •How to Create and Specify a System
- •Working With Systems
- •Technical Discussion
- •References
- •Vector Autoregressions (VARs)
- •Estimating a VAR in EViews
- •VAR Estimation Output
- •Views and Procs of a VAR
- •Structural (Identified) VARs
- •Vector Error Correction (VEC) Models
- •A Note on Version Compatibility
- •References
- •Chapter 33. State Space Models and the Kalman Filter
- •Background
- •Specifying a State Space Model in EViews
- •Working with the State Space
- •Converting from Version 3 Sspace
- •Technical Discussion
- •References
- •Chapter 34. Models
- •Overview
- •An Example Model
- •Building a Model
- •Working with the Model Structure
- •Specifying Scenarios
- •Using Add Factors
- •Solving the Model
- •Working with the Model Data
- •References
- •Part VIII. Panel and Pooled Data
- •Chapter 35. Pooled Time Series, Cross-Section Data
- •The Pool Workfile
- •The Pool Object
- •Pooled Data
- •Setting up a Pool Workfile
- •Working with Pooled Data
- •Pooled Estimation
- •References
- •Chapter 36. Working with Panel Data
- •Structuring a Panel Workfile
- •Panel Workfile Display
- •Panel Workfile Information
- •Working with Panel Data
- •Basic Panel Analysis
- •References
- •Chapter 37. Panel Estimation
- •Estimating a Panel Equation
- •Panel Estimation Examples
- •Panel Equation Testing
- •Estimation Background
- •References
- •Part IX. Advanced Multivariate Analysis
- •Chapter 38. Cointegration Testing
- •Johansen Cointegration Test
- •Single-Equation Cointegration Tests
- •Panel Cointegration Testing
- •References
- •Chapter 39. Factor Analysis
- •Creating a Factor Object
- •Rotating Factors
- •Estimating Scores
- •Factor Views
- •Factor Procedures
- •Factor Data Members
- •An Example
- •Background
- •References
- •Appendix B. Estimation and Solution Options
- •Setting Estimation Options
- •Optimization Algorithms
- •Nonlinear Equation Solution Methods
- •References
- •Appendix C. Gradients and Derivatives
- •Gradients
- •Derivatives
- •References
- •Appendix D. Information Criteria
- •Definitions
- •Using Information Criteria as a Guide to Model Selection
- •References
- •Appendix E. Long-run Covariance Estimation
- •Technical Discussion
- •Kernel Function Properties
- •References
- •Index
- •Symbols
- •Numerics
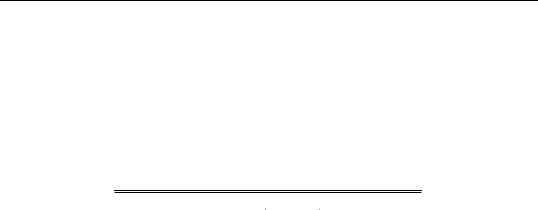
Working with an Equation—243
sidered previously. The results are presented in two parts: the test specification and test results are displayed at the top of the output, and the results for the test equation (not depicted) are displayed at the bottom:
Cointegration Test - Park Added Variables
Date: 08/11/09 Time: 13:49
Equation: EQ_19_3_31
Series: LC LY
Null hypothesis: Series are cointegrated
Original trend specification: Linear trend
Added trends: Powers of trend up to 3
Added deterministics to test: @TREND^2 (@TREND/170)^3
|
Value |
df |
Probability |
|
Chi-square |
12.72578 |
2 |
0.0017 |
|
|
|
|
|
|
The null hypothesis is that the series are cointegrated. The original specification includes a constant and linear trend and the test equation will include up to a cubic trend. The Park test evaluates the statistical significance of the @TREND^2 and the (@TREND/170)^3 terms using a conventional Wald test. (You may notice that the latter cubic trend term—and any higher order trends that you may include—uses the trend scaled by the number of observations in the sample.)
The test results reject the null hypothesis of cointegration, in direct contrast to the results for the Engle-Granger, Phillips-Ouliarias, and Hansen tests (though the latter, which also tests the null of cointegration, is borderline). Note however, adding a quadratic trend to the original equation and then testing for cointegration yields results that, for all four tests, point to cointegration between LC and LY.
Working with an Equation
Once you estimate your equation, EViews offers a variety of views and procedures for examining the properties of the equation, testing, forecasting, and generating new data. For the most part, these views and procedures are a subset of those available in other estimation settings such as least squares estimation. (The one new view, for cointegration testing, is described in depth in “Testing for Cointegration,” beginning on page 234.) In some cases there have been modifications to account for the nature of cointegrating regression.
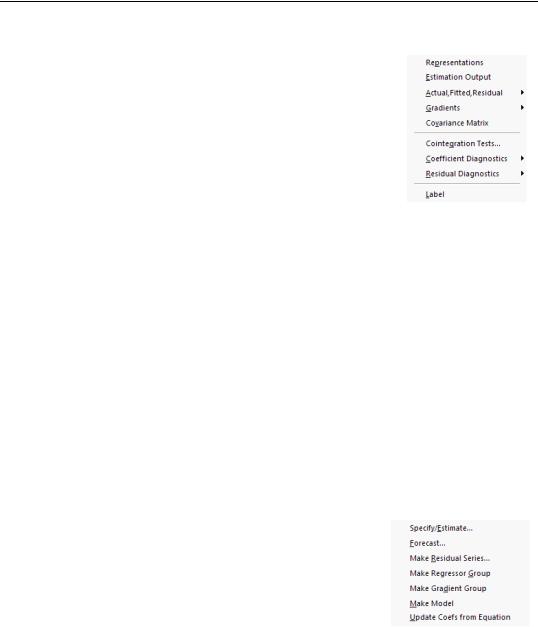
244—Chapter 25. Cointegrating Regression
Views
For the most part, the views of a cointegrating equation require little discussion. For example, the Representations view offers text descriptions of the estimated cointegrating equation, the Covariance Matrix displays the coefficient covariance, and the Residual Diagnostics (Correlogram - Q-statistics, Correlogram Squared Residuals, Histogram - Normality Test) offer statistics based on residuals. That said, a few comments about the construction of these views are in order.
First, the Representations and Covariance Matrix views of an
equation only show results for the cointegrating equation and the long-run coefficients. In particular, the short-run dynamics included in a DOLS equation are not incorporated into the equation. Similarly, Coefficient Diagnostics and Gradients views do not include any of the short-run coefficients.
Second, the computation of the residuals used in the Actual, Fitted, Residual views and the Residual Diagnostics views differs depending on the estimation method. For FMOLS and CCR, the residuals are derived simply by substituting the estimated coefficients into the cointegrating equation and computing the residuals. The values are not based on the transformed data. For DOLS, the residuals from the cointegrating equation are adjusted for the estimated short-run dynamics. In all cases, the test statistics results in the Residual Diagnostics should only be viewed is illustrative as they are not supported by asymptotic theory.
The Gradient (score) views are based on the moment conditions implied by the particular estimation method. For FMOLS and CCR, these moment conditions are based on the transformed data (see Equation (25.25) for the expression for FMOLS scores). For DOLS, these val-
ues are simply proportional (-2 times) to the residuals times the regressors.
Procedures
The procs for an equation estimated using cointegrating regression are virtually identical to those found in least squares estimation.
Most of the relevant issues were discussed previously (e.g., construction of residuals and gradients), however you should also note that forecasts constructed using the Forecast... procedure and models created using Make Model procedure follow
the Representations view in omitting DOLS short-run dynamics. Furthermore, the forecast standard errors generated by the Forecast... proc and from solving models created using the Make Model... proc both employ the S.E. of the regression reported in the estimation output. This may not be appropriate.

References—245
Data Members
The summary statistics results in the bottom of the equation output may be accessed using data member functions (see “Equation Data Members” on page 34 for a list of common data members). For equations estimated using DOLS (with default standard errors), FMOLS, or CCR, EViews computes an estimate of the long-run variance of the residuals. This statistic may be accessed using the @lrvar member function, so that if you have an equation named FMOLS,
scalar mylrvar = fmols.@lrvar
will store the desired value in the scalar MYLRVAR.
References
Engle, R. F., and C. W. J. Granger (1987). “Co-integration and Error Correction: Representation, Estimation, and Testing,” Econometrica, 55, 251-276.
Hamilton, James D. (1994). Time Series Analysis, Princeton: Princeton University Press.
Hansen, Bruce E. (1992a). “Efficient Estimation and Testing of Cointegrating Vectors in the Presence of Deterministic Trends,” Journal of Econometrics, 53, 87-121.
Hansen, Bruce E. (1992b). “Tests for Parameter Instability in Regressions with I(1) Processes,” Journal of Business and Economic Statistics, 10, 321-335.
Hayashi, Fumio (2000). Econometrics, Princeton: Princeton University Press.
MacKinnon, James G. (1996). “Numerical Distribution Functions for Unit Root and Cointegration Tests,”
Journal of Applied Econometrics, 11, 601-618.
Ogaki, Masao (1993). “Unit Roots in Macroeconometrics: A Survey,” Monetary and Economic Studies, 11, 131-154.
Park, Joon Y. (1992). “Canonical Cointegrating Regressions,” Econometrica, 60, 119-143.
Park, Joon Y. and Masao Ogaki (1991). “Inferences in Cointegrated Models Using VAR Prewhitening to Estimate Short-run Dynamics,” Rochester Center for Economic Research Working Paper No. 281.
Phillips, Peter C. B. and Bruce E. Hansen (1990). “Statistical Inference in Instrumental Variables Regression with I(1) Processes,” Review of Economics Studies, 57, 99-125.
Phillips, Peter C. B. and Hyungsik R. Moon (1999). “Linear Regression Limit Theory for Nonstationary Panel Data,” Econometrica, 67, 1057-1111.
Phillips, Peter C. B. and Mico Loretan (1991). “Estimating Long-run Economic Equilibria,” Review of Economic Studies, 59, 407-436.
Saikkonen, Pentti (1992). “Estimation and Testing of Cointegrated Systems by an Autoregressive Approximation,” Econometric Theory, 8, 1-27.
Stock, James H. (1994). “Unit Roots, Structural Breaks and Trends,” Chapter 46 in Handbook of Econometrics, Volume 4, R. F. Engle & D. McFadden (eds.), 2739-2841, Amsterdam: Elsevier Science Publishers B.V.
Stock, James H. and Mark Watson (1993). “A Simple Estimator Of Cointegrating Vectors In Higher Order Integrated Systems,” Econometrica, 61, 783-820.

246—Chapter 25. Cointegrating Regression