
- •Preface
- •Part IV. Basic Single Equation Analysis
- •Chapter 18. Basic Regression Analysis
- •Equation Objects
- •Specifying an Equation in EViews
- •Estimating an Equation in EViews
- •Equation Output
- •Working with Equations
- •Estimation Problems
- •References
- •Chapter 19. Additional Regression Tools
- •Special Equation Expressions
- •Robust Standard Errors
- •Weighted Least Squares
- •Nonlinear Least Squares
- •Stepwise Least Squares Regression
- •References
- •Chapter 20. Instrumental Variables and GMM
- •Background
- •Two-stage Least Squares
- •Nonlinear Two-stage Least Squares
- •Limited Information Maximum Likelihood and K-Class Estimation
- •Generalized Method of Moments
- •IV Diagnostics and Tests
- •References
- •Chapter 21. Time Series Regression
- •Serial Correlation Theory
- •Testing for Serial Correlation
- •Estimating AR Models
- •ARIMA Theory
- •Estimating ARIMA Models
- •ARMA Equation Diagnostics
- •References
- •Chapter 22. Forecasting from an Equation
- •Forecasting from Equations in EViews
- •An Illustration
- •Forecast Basics
- •Forecasts with Lagged Dependent Variables
- •Forecasting with ARMA Errors
- •Forecasting from Equations with Expressions
- •Forecasting with Nonlinear and PDL Specifications
- •References
- •Chapter 23. Specification and Diagnostic Tests
- •Background
- •Coefficient Diagnostics
- •Residual Diagnostics
- •Stability Diagnostics
- •Applications
- •References
- •Part V. Advanced Single Equation Analysis
- •Chapter 24. ARCH and GARCH Estimation
- •Basic ARCH Specifications
- •Estimating ARCH Models in EViews
- •Working with ARCH Models
- •Additional ARCH Models
- •Examples
- •References
- •Chapter 25. Cointegrating Regression
- •Background
- •Estimating a Cointegrating Regression
- •Testing for Cointegration
- •Working with an Equation
- •References
- •Binary Dependent Variable Models
- •Ordered Dependent Variable Models
- •Censored Regression Models
- •Truncated Regression Models
- •Count Models
- •Technical Notes
- •References
- •Chapter 27. Generalized Linear Models
- •Overview
- •How to Estimate a GLM in EViews
- •Examples
- •Working with a GLM Equation
- •Technical Details
- •References
- •Chapter 28. Quantile Regression
- •Estimating Quantile Regression in EViews
- •Views and Procedures
- •Background
- •References
- •Chapter 29. The Log Likelihood (LogL) Object
- •Overview
- •Specification
- •Estimation
- •LogL Views
- •LogL Procs
- •Troubleshooting
- •Limitations
- •Examples
- •References
- •Part VI. Advanced Univariate Analysis
- •Chapter 30. Univariate Time Series Analysis
- •Unit Root Testing
- •Panel Unit Root Test
- •Variance Ratio Test
- •BDS Independence Test
- •References
- •Part VII. Multiple Equation Analysis
- •Chapter 31. System Estimation
- •Background
- •System Estimation Methods
- •How to Create and Specify a System
- •Working With Systems
- •Technical Discussion
- •References
- •Vector Autoregressions (VARs)
- •Estimating a VAR in EViews
- •VAR Estimation Output
- •Views and Procs of a VAR
- •Structural (Identified) VARs
- •Vector Error Correction (VEC) Models
- •A Note on Version Compatibility
- •References
- •Chapter 33. State Space Models and the Kalman Filter
- •Background
- •Specifying a State Space Model in EViews
- •Working with the State Space
- •Converting from Version 3 Sspace
- •Technical Discussion
- •References
- •Chapter 34. Models
- •Overview
- •An Example Model
- •Building a Model
- •Working with the Model Structure
- •Specifying Scenarios
- •Using Add Factors
- •Solving the Model
- •Working with the Model Data
- •References
- •Part VIII. Panel and Pooled Data
- •Chapter 35. Pooled Time Series, Cross-Section Data
- •The Pool Workfile
- •The Pool Object
- •Pooled Data
- •Setting up a Pool Workfile
- •Working with Pooled Data
- •Pooled Estimation
- •References
- •Chapter 36. Working with Panel Data
- •Structuring a Panel Workfile
- •Panel Workfile Display
- •Panel Workfile Information
- •Working with Panel Data
- •Basic Panel Analysis
- •References
- •Chapter 37. Panel Estimation
- •Estimating a Panel Equation
- •Panel Estimation Examples
- •Panel Equation Testing
- •Estimation Background
- •References
- •Part IX. Advanced Multivariate Analysis
- •Chapter 38. Cointegration Testing
- •Johansen Cointegration Test
- •Single-Equation Cointegration Tests
- •Panel Cointegration Testing
- •References
- •Chapter 39. Factor Analysis
- •Creating a Factor Object
- •Rotating Factors
- •Estimating Scores
- •Factor Views
- •Factor Procedures
- •Factor Data Members
- •An Example
- •Background
- •References
- •Appendix B. Estimation and Solution Options
- •Setting Estimation Options
- •Optimization Algorithms
- •Nonlinear Equation Solution Methods
- •References
- •Appendix C. Gradients and Derivatives
- •Gradients
- •Derivatives
- •References
- •Appendix D. Information Criteria
- •Definitions
- •Using Information Criteria as a Guide to Model Selection
- •References
- •Appendix E. Long-run Covariance Estimation
- •Technical Discussion
- •Kernel Function Properties
- •References
- •Index
- •Symbols
- •Numerics
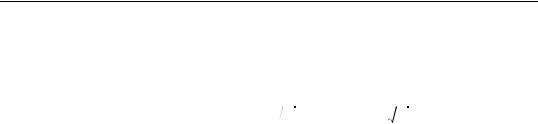
40—Chapter 19. Additional Regression Tools
The weighted mean is the value of the estimated intercept in the restricted model, and is used in forming the reported F-test.
The remaining statistics such as the “Mean dependent var.”, “Sum squared resid”, and the “Log likelihood” all depend on the choice of scale. They may be thought of as the statistics
computed using the weighted data, yt = |
wt yt and xt |
= wt xt . For example, the |
||
mean of the dependent variable is computed as (Âyt ) § T , and the sum-of-squared resid- |
||||
ˆ |
2 |
|
|
|
uals is given by Âwt(yt – xt ¢b) |
. These values should not be compared across equa- |
|||
tions estimated using different weight scaling. |
|
|
||
Lastly, EViews reports a set of Unweighted Statistics. As the name suggests, these are sta- |
||||
tistics computed using the unweighted data and the WLS coefficients. |
|
|||
Nonlinear Least Squares |
|
|
|
|
Suppose that we have the regression specification: |
|
|
||
yt |
= f(xt, b) + et , |
|
(19.18) |
|
where f is a general function of the explanatory variables xt |
and the parameters b . Least |
|||
squares estimation chooses the parameter values that minimize the sum of squared residu- |
||||
als: |
|
|
|
|
S(b) = Â(yt – f(xt, b))2 = |
(y – f(X, b))¢(y – f(X, b)) |
(19.19) |
||
t |
|
|
|
|
We say that a model is linear in parameters if the derivatives of f with respect to the parameters do not depend upon b ; if the derivatives are functions of b , we say that the model is nonlinear in parameters.
For example, consider the model given by: |
|
yt = b1 + b2logLt + b3logKt + et . |
(19.20) |
It is easy to see that this model is linear in its parameters, implying that it can be estimated using ordinary least squares.
In contrast, the equation specification: |
|
yt = b1Ltb2 Ktb3 + et |
(19.21) |
has derivatives that depend upon the elements of b . There is no way to rearrange the terms in this model so that ordinary least squares can be used to minimize the sum-of-squared residuals. We must use nonlinear least squares techniques to estimate the parameters of the model.

Nonlinear Least Squares—41
Nonlinear least squares minimizes the sum-of-squared residuals with respect to the choice of parameters b . While there is no closed form solution for the parameter estimates, the estimates satisfy the first-order conditions:
(G(b))¢(y – f(X, b)) = |
0 , |
(19.22) |
where G(b) is the matrix of first derivatives of f(X, |
b) |
with respect to b (to simplify |
notation we suppress the dependence of G upon X ). The estimated covariance matrix is given by:
ˆ |
= |
s |
2 |
(G(bNLLS)¢G(bNLLS )) |
–1 |
. |
(19.23) |
SNLLS |
|
|
where bNLLS are the estimated parameters. For additional discussion of nonlinear estimation, see Pindyck and Rubinfeld (1998, p. 265-273) or Davidson and MacKinnon (1993).
Estimating NLS Models in EViews
It is easy to tell EViews that you wish to estimate the parameters of a model using nonlinear least squares. EViews automatically applies nonlinear least squares to any regression equation that is nonlinear in its coefficients. Simply select Object/New Object.../Equation, enter the equation in the equation specification dialog box, and click OK. EViews will do all of the work of estimating your model using an iterative algorithm.
A full technical discussion of iterative estimation procedures is provided in Appendix B. “Estimation and Solution Options,” beginning on page 751.
Specifying Nonlinear Least Squares
For nonlinear regression models, you will have to enter your specification in equation form using EViews expressions that contain direct references to coefficients. You may use elements of the default coefficient vector C (e.g. C(1), C(2), C(34), C(87)), or you can define and use other coefficient vectors. For example:
y = c(1) + c(2)*(k^c(3)+l^c(4))
is a nonlinear specification that uses the first through the fourth elements of the default coefficient vector, C.
To create a new coefficient vector, select Object/New Object.../Matrix-Vector-Coef in the main menu and provide a name. You may now use this coefficient vector in your specification. For example, if you create a coefficient vector named CF, you can rewrite the specification above as:
y = cf(11) + cf(12)*(k^cf(13)+l^cf(14))
which uses the eleventh through the fourteenth elements of CF.
You can also use multiple coefficient vectors in your specification:
y = c(11) + c(12)*(k^cf(1)+l^cf(2))

42—Chapter 19. Additional Regression Tools
which uses both C and CF in the specification.
It is worth noting that EViews implicitly adds an additive disturbance to your specification. For example, the input
y = (c(1)*x + c(2)*z + 4)^2
is interpreted as yt = (c(1)xt + c(2)zt + 4)2 + et , and EViews will minimize: |
|
S(c(1), c(2)) = Â(yt – (c(1)xt + c(2)zt + 4)2)2 |
(19.24) |
t |
|
If you wish, the equation specification may be given by a simple expression that does not include a dependent variable. For example, the input,
(c(1)*x + c(2)*z + 4)^2
is interpreted by EViews as –(c(1)xt + c(2)zt + 4)2 = et , and EViews will minimize:
S(c(1), c(2)) = Â(–(c(1)xt + c(2)zt + 4)2 )2 |
(19.25) |
t |
|
While EViews will estimate the parameters of this last specification, the equation cannot be used for forecasting and cannot be included in a model. This restriction also holds for any equation that includes coefficients to the left of the equal sign. For example, if you specify,
x + c(1)*y = z^c(2)
EViews will find the values of C(1) and C(2) that minimize the sum of squares of the implicit equation:
xt + c(1)yt – ztc(2) = et |
(19.26) |
The estimated equation cannot be used in forecasting or included in a model, since there is no dependent variable.
Estimation Options
Starting Values. Iterative estimation procedures require starting values for the coefficients of the model. There are no general rules for selecting starting values for parameters. The closer to the true values the better, so if you have reasonable guesses for parameter values, these can be useful. In some cases, you can obtain good starting values by estimating a restricted version of the model using least squares. In general, however, you will have to experiment in order to find starting values.
EViews uses the values in the coefficient vector at the time you begin the estimation procedure as starting values for the iterative procedure. It is easy to examine and change these coefficient starting values.

Nonlinear Least Squares—43
To see the starting values, double click on the coefficient vector in the workfile directory. If the values appear to be reasonable, you can close the window and proceed with estimating your model.
If you wish to change the starting values, first make certain that the spreadsheet view of your coefficients is in edit mode, then enter the coefficient values. When you are finished setting the initial values, close the coefficient vector window and estimate your model.
You may also set starting coefficient values from the command window using the PARAM command. Simply enter the PARAM keyword, following by each coefficient and desired value:
param c(1) 153 c(2) .68 c(3) .15
sets C(1)=153, C(2)=.68, and C(3)=.15.
See Appendix B, “Estimation and Solution Options” on page 751, for further details.
Derivative Methods. Estimation in EViews requires computation of the derivatives of the regression function with respect to the parameters. EViews provides you with the option of computing analytic expressions for these derivatives (if possible), or computing finite difference numeric derivatives in cases where the derivative is not constant. Furthermore, if numeric derivatives are computed, you can choose whether to favor speed of computation (fewer function evaluations) or whether to favor accuracy (more function evaluations). Additional issues associated with ARIMA models are discussed in “Estimation Options” on page 100.
Iteration and Convergence Options. You can control the iterative process by specifying convergence criterion and the maximum number of iterations. Press the Options button in the equation dialog box and enter the desired values.
EViews will report that the estimation procedure has converged if the convergence test value is below your convergence tolerance. See “Iteration and Convergence Options” on page 753 for details.
In most cases, you will not need to change the maximum number of iterations. However, for some difficult to estimate models, the iterative procedure will not converge within the maximum number of iterations. If your model does not converge within the allotted number of iterations, simply click on the Estimate button, and, if desired, increase the maximum number of iterations. Click on OK to accept the options, and click on OK to begin estimation. EViews will start estimation using the last set of parameter values as starting values.
These options may also be set from the global options dialog. See Appendix A, “Estimation Defaults” on page 630.
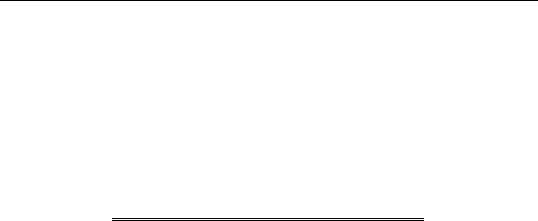
44—Chapter 19. Additional Regression Tools
Output from NLS
Once your model has been estimated, EViews displays an equation output screen showing the results of the nonlinear least squares procedure. Below is the output from a regression of LOG(CS) on C, and the Box-Cox transform of GDP using the data in the workfile “Chow_var.WF1”:
Dependent Variable: LOG(CS)
Method: Least Squares
Date: 08/08/09 Time: 22:28
Sample (adjusted): 1947Q1 1995Q1
Included observations: 193 after adjustments
Convergence achieved after 14 iterations
LOG(CS)=C(1)+C(2)*(GDP^C(3)-1)/C(3)
|
Coefficient |
Std. Error |
t-Statistic |
Prob. |
|
|
|
|
|
|
|
|
|
|
C(1) |
2.769341 |
0.286679 |
9.660067 |
0.0000 |
C(2) |
0.269884 |
0.043126 |
6.258029 |
0.0000 |
C(3) |
0.177070 |
0.020194 |
8.768404 |
0.0000 |
|
|
|
|
|
|
|
|
|
|
R-squared |
0.997253 |
Mean dependent var |
7.476058 |
|
Adjusted R-squared |
0.997224 |
S.D. dependent var |
0.465503 |
|
S.E. of regression |
0.024527 |
Akaike info criterion |
-4.562688 |
|
Sum squared resid |
0.114296 |
Schwarz criterion |
-4.511973 |
|
Log likelihood |
443.2994 |
Hannan-Quinn criter. |
-4.542150 |
|
F-statistic |
34486.03 |
Durbin-W atson stat |
0.134844 |
|
Prob(F-statistic) |
0.000000 |
|
|
|
|
|
|
|
|
|
|
|
|
|
If the estimation procedure has converged, EViews will report this fact, along with the number of iterations that were required. If the iterative procedure did not converge, EViews will report “Convergence not achieved after” followed by the number of iterations attempted.
Below the line describing convergence, EViews will repeat the nonlinear specification so that you can easily interpret the estimated coefficients of your model.
EViews provides you with all of the usual summary statistics for regression models. Provided that your model has converged, the standard statistical results and tests are asymptotically valid.
NLS with ARMA errors
EViews will estimate nonlinear regression models with autoregressive error terms. Simply select Object/New Object.../Equation… or Quick/Estimate Equation… and specify your model using EViews expressions, followed by an additive term describing the AR correction enclosed in square brackets. The AR term should consist of a coefficient assignment for each AR term, separated by commas. For example, if you wish to estimate,
CSt |
= c1 |
+ GDPtc2 + ut |
(19.27) |
|
|
|
ut = c3ut – 1 + c4ut – 2 + et

Nonlinear Least Squares—45
you should enter the specification:
cs = c(1) + gdp^c(2) + [ar(1)=c(3), ar(2)=c(4)]
See “How EViews Estimates AR Models,” on page 92 for additional details. EViews does not currently estimate nonlinear models with MA errors, nor does it estimate weighted models with AR terms—if you add AR terms to a weighted nonlinear model, the weighting series will be ignored.
Weighted NLS
Weights can be used in nonlinear estimation in a manner analogous to weighted linear least squares in equations without ARMA terms. To estimate an equation using weighted nonlinear least squares, enter your specification, press the Options button and fill in the weight specification.
EViews minimizes the sum of the weighted squared residuals:
S(b) = Âwt(yt – f(xt, b))2 = (y – f(X, b))¢W(y – f(X, b)) |
(19.28) |
t |
|
with respect to the parameters b , where wt are the values of the weight series and W is the diagonal matrix of weights. The first-order conditions are given by,
(G(b))¢W(y – f(X, b)) = 0 |
(19.29) |
and the default covariance estimate is computed as:
ˆ |
= |
s |
2 |
(G(bWNLLS)¢WG(bWNLLS)) |
–1 |
. |
(19.30) |
SWNLLS |
|
|
Solving Estimation Problems
EViews may not be able to estimate your nonlinear equation on the first attempt. Sometimes, the nonlinear least squares procedure will stop immediately. Other times, EViews may stop estimation after several iterations without achieving convergence. EViews might even report that it cannot improve the sums-of-squares. While there are no specific rules on how to proceed if you encounter these estimation problems, there are a few general areas you might want to examine.
Starting Values
If you experience problems with the very first iteration of a nonlinear procedure, the problem is almost certainly related to starting values. See the discussion above for how to examine and change your starting values.
Model Identification
If EViews goes through a number of iterations and then reports that it encounters a “Near Singular Matrix”, you should check to make certain that your model is identified. Models