
EViews Guides BITCH / EViews_tutorial
.pdf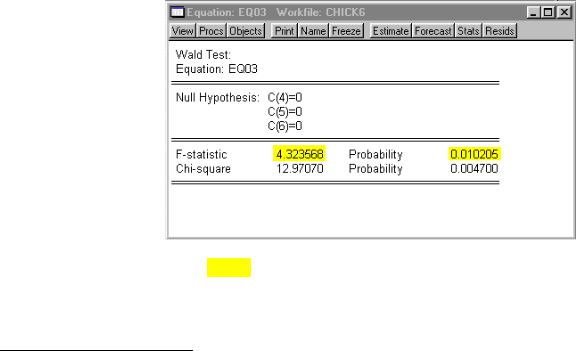
Ramsey's Regression Specification Error Test (RESET) (UE 6.8.1):
Complete Steps 1 - 5 of the section entitled Adding or deleting variables to/from an OLS model in EViews before attempting this section (i.e., EQ02 should be present in the workfile). Follow these steps to carry out the Ramsey's Regression Specification Error Test (RESET) using the step-by-step approach followed in UE, pp. 193-195:
Step 1. Open the EViews workfile named Chick6.wf1.
Step 2. Open EQ02 by double clicking its icon in the workfile window (see UE, Equation 6.9, p. 194).
Step 3. Select Forecast on the equation menu bar, enter YF in the Forecast name: window, and click OK.3
Step 4. Select Objects/New Object/Equation on the workfile menu bar, enter Y C PC YD YF^2 YF^3 YF^4 in the Equation Specification: window, and click OK (see UE, Equation 6.10, p. 194).
Step 5.Select Name on the equation menu bar, enter EQ03 in the Name to identify object: window, and click OK.
Step 6. Select View/Coefficient Tests/Wald-Coefficient Restrictions on the equation menu bar, enter C(4)=0, C(5)=0, C(6)=0 in the Coefficient restrictions separated by commas: window,4 and click OK
to get the graphic shown on the right.
Step 7. Determine the critical F-statistic from UE, Table B-2 or by entering the formula:
=@qfdist(0.95,3,eq03. @regobseq03.@ncoef) in the command window, pressing Enter, and reading the value on the status line in the lower left of the screen.
Step 8. The F-statistic, highlighted in yellow, is the same as reported in UE, p. 195.5 Since the calculated F-statistic of 4.32 exceeds the critical F-statistic of 2.85, the null hypothesis that the coefficients on the added variables are jointly zero can be rejected at the 5% level. This is in spite of the fact that none of their coefficients are individually significant.
3This creates a new series with forecast values of Y based on the estimated coefficients for EQ02.
4Note that the coefficient restrictions are written as C(i), where i represents the coefficient order number of the variable as it was entered in the Equation Specification: window, following the dependent variable. Thus, C(4),C(5) and C(6) represent the coefficients for YF^2 YF^3 YF^4 in the Equation Specification: Y C PC YD YF^2 YF^3 YF^4.
5The Chi-square statistic is equal to the F-statistic times the number of restrictions under test. In this example, there are three restrictions, so the Chi-square test statistic is three times the size of the F-statistic, but the p-values of both statistics indicate that we can decisively reject the null hypothesis that the three coefficients are zero.
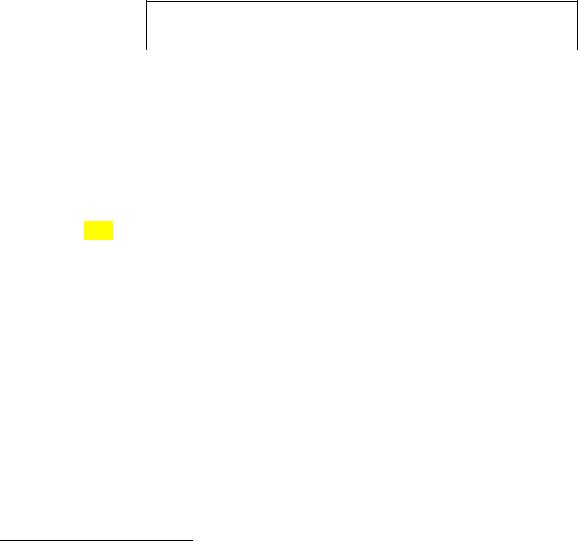
Ramsey's Regression Specification Error Test (RESET) (EViews):
Complete Steps 1 - 5 of the section entitled Adding or deleting variables to/from an OLS model in EViews before attempting this section (i.e., EQ02 should be present in the workfile). Follow these steps to carry out the Ramsey's Regression Specification Error Test (RESET) using EViews, built in Ramsey's RESET Test:
Step 1. Open the EViews workfile named Chick6.wf1.
Step 2. Open EQ02 by double clicking its icon in the workfile window (see UE, Equation 6.9, p. 194).
Step 3. Select View/Stability Tests/Ramsey RESET Test…, enter 3 in the Number of fitted terms: window6, and click OK to get the table below.
Note that the output is very similar to the output achieved with the step-by- step approach in the previous section. In this case, the test results are printed above the regression output table.
Since the calculated F-statistic of 4.32
exceeds the critical F-statistic of 2.85, the null hypothesis that the coefficients on the added variables are jointly zero can be rejected at the 5% level. This
Ramsey RESET Test:
F-statistic |
|
|
4.323568 |
|
Probability |
|
|
0.010205 |
|
Log likelihood ratio |
|
|
12.92125 |
|
Probability |
|
|
0.004810 |
|
|
|
|
|
|
|
|
|
|
|
Test Equation: |
|
|
|
|
|
|
|
|
|
Dependent Variable: Y |
|
|
|
|
|
|
|
|
|
Method: Least Squares |
|
|
|
|
|
|
|
|
|
Date: 07/27/00 Time: 07:26 |
|
|
|
|
|
||||
Sample: 1951 1994 |
|
|
|
|
|
|
|
|
|
Included observations: 44 |
|
|
|
|
|
||||
Variable |
|
Coefficient |
Std. Error |
t-Statistic |
|
Prob. |
|||
C |
|
23.80305 |
|
55.36771 |
0.429908 |
0.6697 |
|||
PC |
|
-0.591937 |
1.718030 |
-0.344544 |
0.7323 |
|
|||
YD |
|
0.360179 |
0.714812 |
0.503880 |
0.6173 |
|
|||
FITTED^2 |
|
0.023868 |
0.082475 |
0.289394 |
0.7739 |
||||
FITTED^3 |
|
-0.000748 |
0.001106 |
-0.676301 |
0.5029 |
||||
FITTED^4 |
|
|
5.48E-06 |
5.36E-06 |
1.022646 |
0.3129 |
|||
R-squared |
|
0.988647 |
|
Mean dependent var |
43.37500 |
|
|||
Adjusted R-squared |
|
0.987154 |
|
S.D. dependent var |
16.83854 |
|
|||
S.E. of regression |
|
1.908510 |
Akaike info criterion |
4.256646 |
|||||
Sum squared resid |
|
138.4116 |
|
Schwarz criterion |
4.499945 |
||||
Log likelihood |
|
-87.64622 |
F-statistic |
|
661.8504 |
||||
Durbin-Watson stat |
|
0.861509 |
|
Prob(F-statistic) |
0.000000 |
is in spite of the fact that all of their individual t-statistics are insignificant.
6 The fitted terms are the powers of the fitted values from the original regression, starting with the square or second power. For example, if you specify 3, then the test will add ŷ2, ŷ3, and ŷ4 in the regression. If you specify a large number of fitted terms, EViews may report a near singular matrix error message since the powers of the fitted values are likely to be highly collinear. The Ramsey RESET test is applicable only to an equation estimated by least squares.
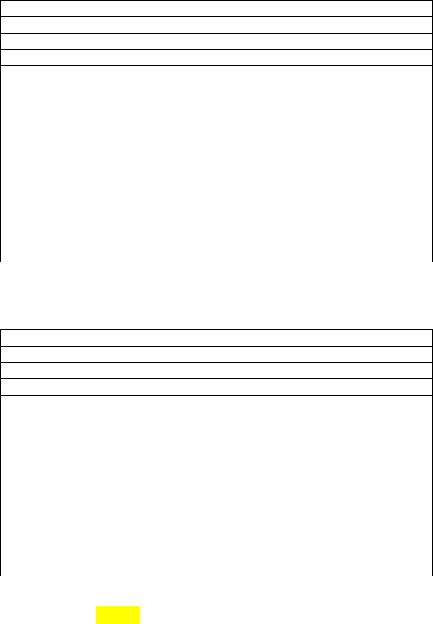
Akaike's Information Criterion (AIC) and the Schwartz Criterion (SC):
Complete Steps 1 - 5 of the section entitled Adding or deleting variables to/from an OLS model in EViews before attempting this section (i.e., EQ01 and EQ02 should be present in the workfile). The Akaike's Information Criterion (AIC) and Schwartz Criterion (SC) are both printed in the Estimation Output of EViews' OLS regressions.
Step 1. Open the EViews workfile named Chick6.wf1.
Step 2. Open EQ01 by double clicking its icon in the workfile window (see UE, Equation 6.8, p. 160 or 196) to get the Estimation Output below.
Dependent Variable: Y
Method: Least Squares
Date: 07/26/00 Time: 09:11
Sample: 1951 1994
Included observations: 44
Variable |
Coefficient |
Std. Error |
t-Statistic |
|
Prob. |
|
C |
31.49604 |
1.312586 |
23.99541 |
0.0000 |
||
PC |
-0.729695 |
0.080020 |
-9.118941 |
0.0000 |
|
|
PB |
0.114148 |
0.045686 |
2.498536 |
0.0167 |
|
|
YD |
0.233830 |
0.016447 |
14.21738 |
0.0000 |
|
|
R-squared |
0.986828 |
Mean dependent var |
43.37500 |
|
||
Adjusted R-squared |
0.985840 |
S.D. dependent var |
16.83854 |
|
||
S.E. of regression |
2.003702 |
Akaike info criterion |
|
4.314378 |
|
|
Sum squared resid |
160.5929 |
Schwarz criterion |
|
4.476577 |
|
|
Log likelihood |
-90.91632 |
F-statistic |
|
|
998.9207 |
|
Durbin-Watson stat |
0.978759 |
Prob(F-statistic) |
0.000000 |
Step 3. Open EQ02 by double clicking its icon in the workfile window (see UE, Equation 6.9, p. 161) to get the Estimation Output below.
Dependent Variable: Y
Method: Least Squares
Date: 07/26/00 Time: 08:01
Sample: 1951 1994
Included observations: 44
Variable |
Coefficient |
Std. Error |
t-Statistic |
|
Prob. |
|
C |
32.94193 |
1.251191 |
26.32845 |
0.0000 |
||
PC |
-0.700954 |
0.084099 |
-8.334841 |
0.0000 |
|
|
YD |
0.272477 |
0.005936 |
45.90552 |
0.0000 |
|
|
R-squared |
0.984772 |
Mean dependent var |
43.37500 |
|
||
Adjusted R-squared |
0.984030 |
S.D. dependent var |
16.83854 |
|
||
S.E. of regression |
2.127957 |
Akaike info criterion |
|
4.413948 |
|
|
Sum squared resid |
185.6562 |
Schwarz criterion |
|
4.535597 |
|
|
Log likelihood |
-94.10685 |
F-statistic |
|
|
1325.737 |
|
Durbin-Watson stat |
0.946570 |
Prob(F-statistic) |
0.000000 |
Note that the AIC & SC information criterion reported in EViews (see numbers highlighted in yellow) are larger when PB is omitted from the OLS regression (i.e., EQ02). Both Akaike's and the Schwartz Criterion provide evidence that UE, Equation 6.8 (i.e., EViews EQ01) is preferable to UE, Equation 6.9 (i.e., EViews EQ02).
Exercise:
15.Open the EViews workfile named Drugs.wf1. a.
i)Select Objects/New Object/Equation on the workfile menu bar, enter P C GDPN CVN PP DPC IPC CV in the Equation Specification: window, and click OK. Select Name on the equation window menu bar, enter EQ01 in the Name to identify object: window, and click OK.
ii)Select Objects/New Object/Equation on the workfile menu bar, enter P C GDPN CVN PP DPC IPC N in the Equation Specification: window, and click OK. Select Name on the equation window menu bar, enter EQ02 in the Name to identify object: window, and click OK.
b. Open EQ01 and EQ02 at the same time. Use information in these tables, UE 6.8 (Appendix) and the procedures outlined in this guide to determine whether CV and/or N are irrelevant or omitted variables.
c.
d.
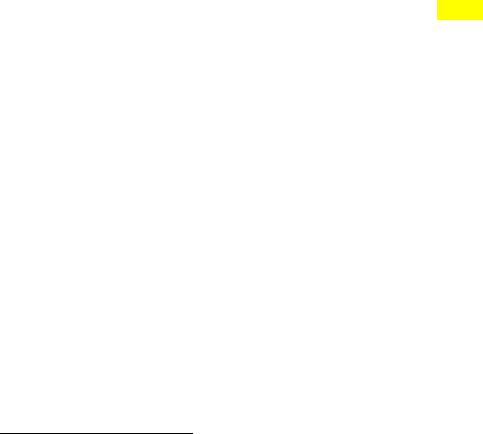
Chapter 7: Specification: Choosing A Functional Form
In this chapter:
1.Table with EViews specification for functional forms
2.Calculating "Quasi - R2" in EViews (UE 7.3.1, footnote 5, p. 215)
3.Calculating "Quasi - R2" for a linear versus log-lin model using EViews
4.Coefficient restrictions tests using EViews (UE, Appendix 7.7)
5.The Chow test, alternately termed Chow's Breakpoint Test (UE, Appendix 7.7)
UE section 7.2 presents alternative functional forms that are useful when specifying econometric models. Linear models are frequently too restrictive to properly fit the functional form suggested by the underlying theory.
The last column of Table 7.1 below shows the correct EViews specification for the alternative functional forms printed in UE, Table 7.1, p. 214. You can use the table as a guide, but you must realize that Y represents the dependent variable while X1 & X2 represent the only independent variables in all of the equations/specifications. Note that a constant (C) should be included in all models even if theory suggests otherwise (see UE, p. 201). You must have a workfile open in order to specify and estimate a regression model. Then, to specify a regression model in EViews, select Objects/New Object/Equation from the workfile menu and enter the appropriate EViews specification (see the last column of the table below), in the Equation Specification: window.1
Table 7.1: EViews Specification of Functional Forms
Section |
Equation # |
Fcn. Form |
Equation specification |
|
|
EViews |
specification |
||||||||
7.2.1 |
---- |
Linear |
Y = β 0 + β 1X1 + β 2X2 |
|
|
|
|
|
|
|
|
Y C X1 |
X2 |
|
|
|
|
|
|
|
|
|
|
|
|
|
|
||||
7.2.2 |
7.3 |
Double-Log |
lnY = β 0 + β 1lnX1 + β 2lnX2 |
|
log(Y) C log(X1) log(X2) |
|
|||||||||
|
|
|
|
|
|
|
|
|
|
|
|
|
|
||
7.2.3 |
7.7 |
Lin-Log |
Y = β 0 + β 1lnX1 |
+ β 2X2 |
|
|
|
|
|
Y C log(X1) X2 |
|
||||
|
|
|
|
|
|
|
|
|
|
|
|
|
|
|
|
7.2.3 |
7.9 |
Log-Lin |
lnY = β 0 + β 1X1 |
+ β 2X2 |
|
|
|
|
|
log(Y) C X1 |
X2 |
|
|||
|
|
|
|
|
|
|
|
|
|
|
|
||||
7.2.4 |
7.10 |
Polynomial |
Y = β 0 + β 1X1 + β 2(X12) + β 3X2 |
|
|
|
|
Y C X1 X1^2 X2 |
|
||||||
|
|
|
|
|
|
|
|
|
|
|
|
|
|
|
|
7.2.5 |
7.13 |
Inverse |
Y = β 0 + β 1(1/X1) + β 2X2 |
|
|
|
|
|
|
|
Y C 1/X1 |
X2 |
|
||
|
|
|
|
|
|
|
|
|
|
|
|
|
|
|
|
7.5 |
7.20 |
Dummy* |
Y = β 0 + β 1X1 |
+ β 2D1 |
|
|
|
|
|
|
|
|
Y C X1 D1 |
|
|
|
|
|
|
|
|
|
|
|
|||||||
7.5 |
7.22 |
Dummy** |
Y = β 0 + β 1X1 + β 2D1 + β 3D1X1 |
|
|
|
Y C X1 D1 D1*X1 |
|
|||||||
|
|
|
|
|
|
|
|
|
|
|
|
|
|
|
|
* Intercept dummy variable. ** Intercept and slope dummy variables.
Calculating "Quasi - R2" in EViews (UE 7.3.1, footnote 5, p. 215):
The dependent variable must be in the same form when using R2 and adjusted R2 to compare the overall goodness of fit between two equations. For example, it would not be appropriate to compare the R2 for a linear model with a double-log or a log-lin model. However, it would be appropriate to compare R2 for a linear model with a lin-log, a
1 Alternately, select Quick/Estimate Equation from the main menu. If this method is used you must name the equation to save it. Select Name on the equation menu bar and enter the desired name in the Name to identify object: window, and click OK.

polynomial, or an inverse functional form model. Likewise, it would be appropriate to compare R2 for double-log and log-lin functional form models. In order to demonstrate the process, the car acceleration data introduced in UE, Exercise 16, p. 234, will be used to demonstrate the process of calculating the quasi-R2. The steps below show how to compare the goodness of fit for models using S (the number of seconds it takes a car to accelerate from 0 to 60 miles per hour) as the dependent variable versus using the natural log of S as the dependent variable. In both models, the independent variables are the same as the original model printed at the top of UE, p. 236.
Calculating "Quasi - R2" for a linear versus a log-lin model using EViews:
Step 1. Open the EViews workfile named Cars7.wk1.
Step 2. Select Objects/New Object/Equation on the workfile menu bar, enter S C T E P H in the Equation Specification: window, and click OK.
Step 3. Select Name on the equation menu bar, write linear in the Name to identify object: window, and click OK. Minimize the equation object named linear.
Step 4. Select Objects/New Object/Equation on the workfile menu bar, enter log(S) C T E P H in the Equation Specification: window (i.e., the log-lin functional form), and click
OK.
Step 5. Select Name on the equation menu bar, write loglin in the Name to identify object: window, and click OK.
Step 6. Select Forecast on the equation menu bar, select S in the Forecast of:2 window, enter SF in the Forecast name: window, uncheck the two boxes in the Output: window (the only objective here is to create a forecast series, not a forecast evaluation), and click OK. A new series named SF appears in the workfile window.
Steps 7, 8 & 9 calculate the quasi-R2 for this regression (UE 7.3.1, footnote 5, p. 215).
Step 7. Minimize the equation window, select Genr on the workfile menu bar, type numerator=(S-SF)^2 in the Enter equation: window, and click OK (this step generates the un-summed variable in the numerator of the quasi-R2 equation).
Step 8. Select Genr on the workfile menu bar, type denominator=(S-@mean(S))^2 in the Enter equation: window, and click OK (this step generates the un-summed variable in the denominator of the quasi-R2 equation).
Step 9. To calculate the quasi-R2, type the following equation in the command window and press Enter: scalar quasir2=1-(@sum(numerator)/@sum(denominator)). A new variable named quasir2 will appear in the workfile window. Double click on it and the value for the quasi-R2 will be displayed in the lower left of the screen (0.783958974). The quasi-R2 calculated in Step 9 (i.e., 0.78) is in-between the R2 from the linear model estimated in Step 2 (i.e., 0.71) and the R2 from the log-lin model estimated in Step 5 (i.e., 0.81).
2 The Forecast procedure in EViews gives you the option of forecasting the transformed dependent variable (i.e., LOG(S) in this case) or the original variable (i.e., S in this case). Select S, since the computation of quasi-R2 requires converting of LOG(S) to S by taking the anti-log of the dependent variable (this can also be done by using the EViews command @exp(LOG(S)).
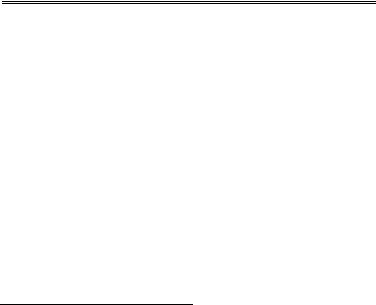
Coefficient restrictions tests using EViews (UE, Appendix 7.7):
The F-test can be used to test a wide range of hypothesis concerning regression coefficients. For example, suppose that the claim was made that when a car has a manual transmission it increases its acceleration speed (i.e., decreases the number of seconds it takes to accelerate from 0 to 60 miles per hour) just as much as adding 100 horsepower to the car. Translating this into the language of UE, Equation 7.28, p. 235, this means that the absolute value of the coefficient on Ti is 100 times larger than the absolute value of the coefficient on Hi. Just looking at the size of the estimated coefficients, it appears that you can easily reject the hypothesis because the absolute value of the coefficient on Ti is only about 41.5 times larger than the absolute value of the coefficient on Hi (divide the coefficient on Ti by the coefficient on Hi). However, these coefficients are just estimates. Follow these steps to carry out an F-test for the null hypothesis that the absolute value of the coefficient on Ti is 100 times larger than the absolute value of the coefficient on Hi. :
Step 1. Open the EViews workfile named Cars7.wk1.
Step 2. Select Objects/New Object/Equation on the workfile menu bar, enter S C T E P H in the Equation Specification: window, and click OK.
Step 3.Select Name on the equation menu bar, write EQ01 in the Name to identify object: window, and click OK.
Step 4. Select View/Coefficients Tests/Wald-Coefficient Restrictions … on the equation menu bar, enter -C(2)=-100*C(5) in the Coefficients separated by commas: window, and click OK to reveal the following output:3
Wald Test:
Equation: EQ01
Null Hypothesis: |
-C(2)=-100*C(5) |
|
|
|||||
|
|
|
|
|
|
|
|
|
F-statistic |
2.485049 |
|
|
Probability |
0.124472 |
|||
Chi-square |
2.485049 |
|
|
Probability |
0.114933 |
|||
|
|
|
|
|
|
|
|
|
|
|
|
|
|
|
|
|
|
The null hypothesis is -C(2)=-100*C(5), since variable T is the second coefficient and variable H is the fifth coefficient in the EViews Estimation Output from Step 2. The F- statistic compares the residual sum of squares computed with and without the restrictions imposed. If the restrictions are valid, there should be little difference in the two residual sum-of-squares and the F-value should be small. Based on the Wald Test: results table, the null hypothesis cannot be rejected at the 5% level of significance. The calculated F- statistic of 2.49 is less than the critical F-value of 4.14. The critical F-value can be found in UE, Table B-2, p. 609 for 1 degree of freedom in the numerator and 33 (interpolate between the 30 and 40) degrees of freedom in the denominator or EViews can calculate
3 The coefficients should be referred to as C(1), C(2), and so on (do not use series names). Multiple coefficient restrictions must be separated by commas and the restrictions should be expressed as equations involving estimated coefficients and constants. The coefficients should be referred to as C(1), C(2), and so on (do not use series names).
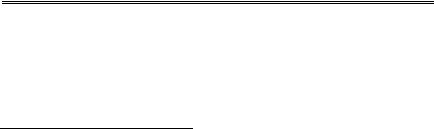
its value.4 The reported probability is the marginal significance level of the F-test. It supports this result in that rejecting the null hypothesis would be wrong less than 12.44% of the time.
The Chi-square statistic is equal to the F-statistic times the number of restrictions under test. In this example, there is only one restriction and so the two test statistics are identical with the p-values of both statistics indicating that we cannot reject the null hypothesis, that the absolute value of the coefficient on Ti is 100 times larger than the absolute value of the coefficient on Hi, at the 10% significance level. The 10% significance critical value for the χ 2 test can be found in UE, Table B-8, p. 619 to be 2.71.
The Chow test, alternately termed Chow's Breakpoint Test (UE, Appendix 7.7):
Chow's Breakpoint Test divides the data into two sub-samples.5 It then estimates the same equation for each sub-sample separately, to see whether there are significant differences in the estimated equations. A significant difference indicates a structural change in the relationship.
Follow these steps to apply the Chow breakpoint test, as described in UE, pp. 241-242, to determine whether there was a structural change in the demand for chicken in 1976:
Step 1. Open the EViews workfile named Chick6.wf1.
Step 2. Select Objects/New Object/Equation on the workfile menu bar, enter Y C PC PB YD in the Equation Specification: window, and click OK.
Step 3. Select Name on the equation menu bar, write EQ01 in the Name to identify object: window, and click OK.
Step 4. Select View/Stability Tests/Chow Breakpoint Test… on the equation menu bar, enter
1976 in the Enter one date (observation) for the Forecast Test or one or more dates for the Breakpoint Test: window, and click OK to reveal the following output:
Chow Breakpoint Test: 1976
F-statistic |
4.542962 |
|
Probability |
0.004498 |
||
Log likelihood ratio |
17.98027 |
|
Probability |
0.001245 |
||
|
|
|
|
|
|
|
|
|
|
|
|
|
|
EViews reports two test statistics for the Chow breakpoint test. The F-statistic is based on the comparison of the restricted and unrestricted sum of squared residuals. EViews calculates the F-statistic using the formula printed in UE, Equation 7.36, p. 242. In this
4 To have EViews calculate the 5% critical F-value for this problem, type the following equation in the command window =@qfdist(0.95,1,eq01.@regobs-eq01.@ncoefs), press Enter and view the following
value on the status bar in the lower left of the screen . For the 10% critical F- value type =@qfdist(0.90,1,eq01.@regobs-eq01.@ncoefs) in the command window, and press Enter and
view the following value on the status bar in the lower left of the screen .
5 One major drawback of the breakpoint test is that each sub-sample requires at least as many observations as the number of estimated parameters. This may be a problem if, for example, you want to test for structural change between wartime and peacetime where there are only a few observations in the wartime sample.

case, the calculated F-statistic of 4.54 exceeds the critical F-value of 2.63 for the 5% level of significance so the null hypothesis of no structural change can be rejected. The critical F-value can be found in UE, Table B-2, p. 609 for 4 degrees of freedom in the numerator and 36 (interpolate between the 30 and 40) degrees of freedom in the denominator or EViews can calculate its value.6 The reported probability is the marginal significance level of the F-test. It supports this result in that rejecting the null hypothesis would be wrong less than 0.4498% of the time.
The log likelihood ratio statistic is based on the comparison of the restricted and unrestricted maximum of the log likelihood function. The LR test statistic has an asymptotic χ 2 distribution with degrees of freedom equal to (m-1)*(k+1) under the null hypothesis of no structural change, where m is the number of sub-samples and k is the number of independent variables in the model (i.e., m = 2 in this case because one breakpoint is selected and k = 3). The calculated value for LR test statistic of 17.98 exceeds of 9.49 for the 5% level of significance and 13.28 for the 1% level of significance so the null hypothesis of no structural change can be rejected.7 The reported probability is the marginal significance level of the χ 2 test. It supports this result in that rejecting the null hypothesis would be wrong less than 0.1245% of the time.
6 To have EViews calculate the 5% critical F-value for this problem, type the following equation in the
command window =@qfdist(0.95,eq01.@ncoef,eq01.@regobs-2*eq01.@ncoef), press Enter
and view the following value on the status bar in the lower left of the screen . 7 The critical value for the χ 2 test can be found in UE, Table B-8, p. 619.

Chapter 8: Multicollinearity
In this chapter:
1.Perfect multicollinearity (UE 8.1.1)
2.Detecting multicollinearity with simple correlation coefficients (UE 8.3.1)
3.Calculating Variance Inflation Factors (UE 8.3.2)
4.Transforming multicollinear variables (UE 8.4.3)
5.Exercises
Perfect multicollinearity (UE 8.1.1):
EViews is incapable of generating estimates of regression coefficients when the model specification contains two or more variables that are perfectly collinear. When the equation specification contains two or more perfectly collinear (or even some highly collinear) variables, EViews will put out the error message “Near singular matrix.”
The next two sections explain how EViews can be used to detect severe multicollinearity (UE 8.3). The data for the fish/Pope example found in UE, Table 8.1, p. 267, will be used to demonstrate the two methods discussed in UE.
Detecting multicollinearity with simple correlation coefficients (UE 8.3.1):
High simple correlation coefficients between variables is a sign of multicollinearity. Follow these steps to compute the simple correlation coefficient between variables:
Step 1. Open the EViews workfile named Fish8.wk1.
Step 2. Create a group object for the variables found in UE, Equation 8.24, p. 268 (i.e., F PF PB log(YD) N P). An easy way to create a group object for a set of variables from a regression model is to select Procs/Make Regressor Group on the equation window menu bar (refer to Chapter 3 to review the usual way of creating a group object).
Step 3. Select View/Correlations on the group object menu bar to reveal the simple correlation coefficients between all of the variables in the group.
Step 4. Select Freeze on the group object menu bar to create a table of the simple correlation coefficients. Select Name on the table object menu bar to name the table.
|
F |
PF |
PB |
LOG(YD) |
N |
P |
F |
1.000000 |
0.847590 |
0.818532 |
0.780012 |
0.736549 |
0.585630 |
PF |
0.847590 |
1.000000 |
0.958096 |
0.915320 |
0.883207 |
0.734643 |
PB |
0.818532 |
0.958096 |
1.000000 |
0.814890 |
0.781400 |
0.663162 |
LOG(YD) |
0.780012 |
0.915320 |
0.814890 |
1.000000 |
0.945766 |
0.744500 |
N |
0.736549 |
0.883207 |
0.781400 |
0.945766 |
1.000000 |
0.571129 |
P |
0.585630 |
0.734643 |
0.663162 |
0.744500 |
0.571129 |
1.000000 |
To test whether a particular simple correlation coefficient between variables is significant, refer to Performing the t-test of the simple correlation coefficient (UE 5.3.3).