
- •Table of Contents
- •Foreword
- •Chapter 1. A Quick Walk Through
- •Workfile: The Basic EViews Document
- •Viewing an individual series
- •Looking at different samples
- •Generating a new series
- •Looking at a pair of series together
- •Estimating your first regression in EViews
- •Saving your work
- •Forecasting
- •What’s Ahead
- •Chapter 2. EViews—Meet Data
- •The Structure of Data and the Structure of a Workfile
- •Creating a New Workfile
- •Deconstructing the Workfile
- •Time to Type
- •Identity Noncrisis
- •Dated Series
- •The Import Business
- •Adding Data To An Existing Workfile—Or, Being Rectangular Doesn’t Mean Being Inflexible
- •Among the Missing
- •Quick Review
- •Appendix: Having A Good Time With Your Date
- •Chapter 3. Getting the Most from Least Squares
- •A First Regression
- •The Really Important Regression Results
- •The Pretty Important (But Not So Important As the Last Section’s) Regression Results
- •A Multiple Regression Is Simple Too
- •Hypothesis Testing
- •Representing
- •What’s Left After You’ve Gotten the Most Out of Least Squares
- •Quick Review
- •Chapter 4. Data—The Transformational Experience
- •Your Basic Elementary Algebra
- •Simple Sample Says
- •Data Types Plain and Fancy
- •Numbers and Letters
- •Can We Have A Date?
- •What Are Your Values?
- •Relative Exotica
- •Quick Review
- •Chapter 5. Picture This!
- •A Simple Soup-To-Nuts Graphing Example
- •A Graphic Description of the Creative Process
- •Picture One Series
- •Group Graphics
- •Let’s Look At This From Another Angle
- •To Summarize
- •Categorical Graphs
- •Togetherness of the Second Sort
- •Quick Review and Look Ahead
- •Chapter 6. Intimacy With Graphic Objects
- •To Freeze Or Not To Freeze Redux
- •A Touch of Text
- •Shady Areas and No-Worry Lines
- •Templates for Success
- •Point Me The Way
- •Your Data Another Sorta Way
- •Give A Graph A Fair Break
- •Options, Options, Options
- •Quick Review?
- •Chapter 7. Look At Your Data
- •Sorting Things Out
- •Describing Series—Just The Facts Please
- •Describing Series—Picturing the Distribution
- •Tests On Series
- •Describing Groups—Just the Facts—Putting It Together
- •Chapter 8. Forecasting
- •Just Push the Forecast Button
- •Theory of Forecasting
- •Dynamic Versus Static Forecasting
- •Sample Forecast Samples
- •Facing the Unknown
- •Forecast Evaluation
- •Forecasting Beneath the Surface
- •Quick Review—Forecasting
- •Chapter 9. Page After Page After Page
- •Pages Are Easy To Reach
- •Creating New Pages
- •Renaming, Deleting, and Saving Pages
- •Multi-Page Workfiles—The Most Basic Motivation
- •Multiple Frequencies—Multiple Pages
- •Links—The Live Connection
- •Unlinking
- •Have A Match?
- •Matching When The Identifiers Are Really Different
- •Contracted Data
- •Expanded Data
- •Having Contractions
- •Two Hints and A GotchYa
- •Quick Review
- •Chapter 10. Prelude to Panel and Pool
- •Pooled or Paneled Population
- •Nuances
- •So What Are the Benefits of Using Pools and Panels?
- •Quick (P)review
- •Chapter 11. Panel—What’s My Line?
- •What’s So Nifty About Panel Data?
- •Setting Up Panel Data
- •Panel Estimation
- •Pretty Panel Pictures
- •More Panel Estimation Techniques
- •One Dimensional Two-Dimensional Panels
- •Fixed Effects With and Without the Social Contrivance of Panel Structure
- •Quick Review—Panel
- •Chapter 12. Everyone Into the Pool
- •Getting Your Feet Wet
- •Playing in the Pool—Data
- •Getting Out of the Pool
- •More Pool Estimation
- •Getting Data In and Out of the Pool
- •Quick Review—Pools
- •Chapter 13. Serial Correlation—Friend or Foe?
- •Visual Checks
- •Testing for Serial Correlation
- •More General Patterns of Serial Correlation
- •Correcting for Serial Correlation
- •Forecasting
- •ARMA and ARIMA Models
- •Quick Review
- •Chapter 14. A Taste of Advanced Estimation
- •Weighted Least Squares
- •Heteroskedasticity
- •Nonlinear Least Squares
- •Generalized Method of Moments
- •Limited Dependent Variables
- •ARCH, etc.
- •Maximum Likelihood—Rolling Your Own
- •System Estimation
- •Vector Autoregressions—VAR
- •Quick Review?
- •Chapter 15. Super Models
- •Your First Homework—Bam, Taken Up A Notch!
- •Looking At Model Solutions
- •More Model Information
- •Your Second Homework
- •Simulating VARs
- •Rich Super Models
- •Quick Review
- •Chapter 16. Get With the Program
- •I Want To Do It Over and Over Again
- •You Want To Have An Argument
- •Program Variables
- •Loopy
- •Other Program Controls
- •A Rolling Example
- •Quick Review
- •Appendix: Sample Programs
- •Chapter 17. Odds and Ends
- •How Much Data Can EViews Handle?
- •How Long Does It Take To Compute An Estimate?
- •Freeze!
- •A Comment On Tables
- •Saving Tables and Almost Tables
- •Saving Graphs and Almost Graphs
- •Unsubtle Redirection
- •Objects and Commands
- •Workfile Backups
- •Updates—A Small Thing
- •Updates—A Big Thing
- •Ready To Take A Break?
- •Help!
- •Odd Ending
- •Chapter 18. Optional Ending
- •Required Options
- •Option-al Recommendations
- •More Detailed Options
- •Window Behavior
- •Font Options
- •Frequency Conversion
- •Alpha Truncation
- •Spreadsheet Defaults
- •Workfile Storage Defaults
- •Estimation Defaults
- •File Locations
- •Graphics Defaults
- •Quick Review
- •Index
- •Symbols
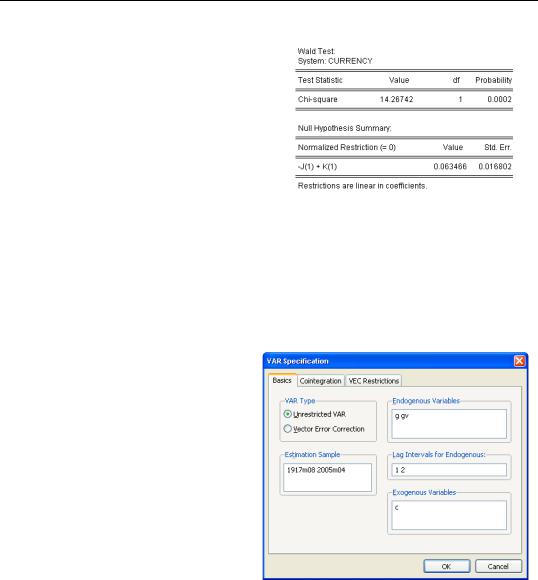
Vector Autoregressions—VAR—361
The answer, which is unsurprising given the reported coefficients and standard errors, is “No, the coefficients are not equal.”
Vector Autoregressions—VAR
In Chapter 8, “Forecasting,” we discussed predictions based on ARMA and ARIMA models. This kind of forecasting generalizes, at least in the case of autoregressive models, to multiple dependent variables through the use of vector autoregressions or VARs. While VARs can be quite sophisticated (see the User’s Guide), at its heart a VAR simply takes a list of series and regresses each on its own past values as well as lags of all the other series in the list.
Create a VAR object either through the Object menu or the var command. The button opens the VAR Specification dialog. Enter the variables to be explained in the Endogenous Variables field.
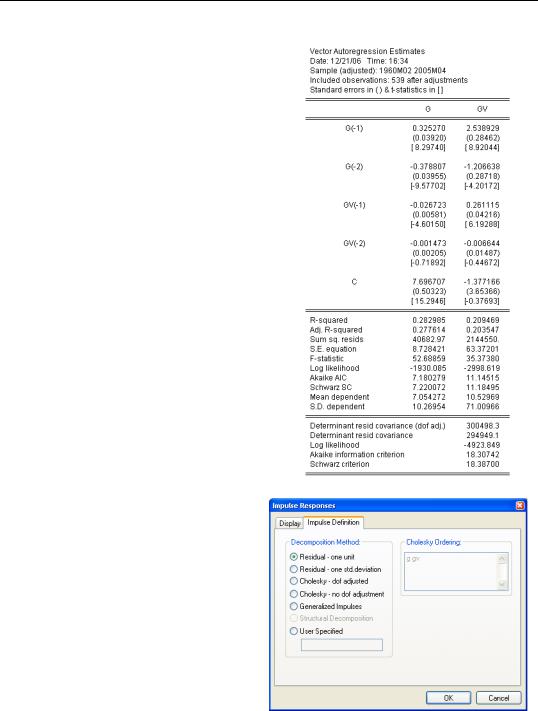
362—Chapter 14. A Taste of Advanced Estimation
EViews estimates least squares equations for both series.
Impulse response
To answer the question “How do the series evolve following a shock to the error term?” click the button. The phrase “following a shock” is less straightforward than it sounds. In general, the error terms will be correlated across equations, so one wants to be careful about shocking one equation but not the other. And how big a shock? One unit? One standard deviation?
You control how you deal with these questions on the Impulse Definition tab. For illustration purposes, let’s consider a unit shock to each error term.
Once we choose a specification, we get a set of impulse response functions. We get a plot of the response over time of each endogenous variable to a shock in each equation. In other words, we see how G responds to shocks to both the G equation and the GV equation, and similarly, how GV responds to shocks to the GV equation and the G equation.
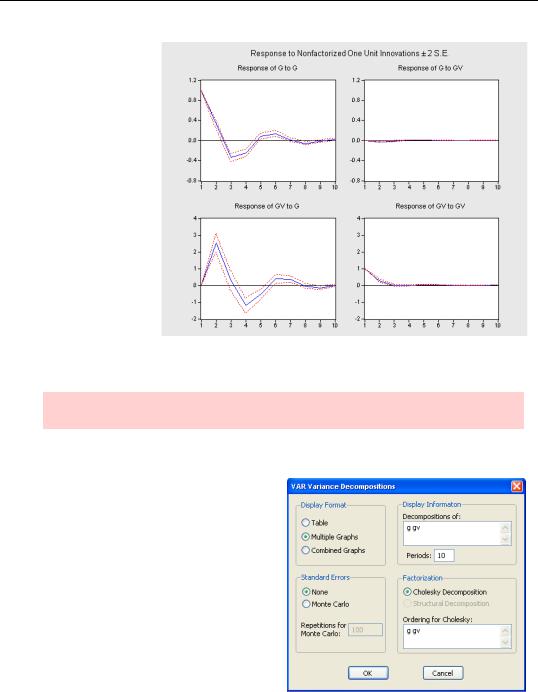
Vector Autoregressions—VAR—363
By default (there are other options) we get one figure containing all four impulse response graphs. The line graph in the upper left-hand corner shows that following a shock to the G equation, G wiggles around for a quarter or so, but by the fifth quarter the response has effectively dissipated. In contrast, the upper righthand corner graph shows that G is
effectively unresponsive to shocks in the GV equation.
Hint: The dashed lines enclose intervals of plus or minus two standard errors.
Variance decomposition
How much of the variance in G is explained by shocks in the G equation and how much is explained by shocks in the GV equation? The answer depends on, among other things, the estimated coefficients, the estimated standard error of each equation, and the order in which you evaluate the shocks. View/Variance Decomposition… leads to the VAR Variance Decompositions dialog where you can set various options.
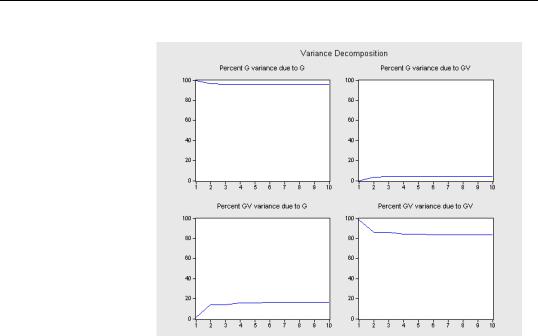
364—Chapter 14. A Taste of Advanced Estimation
The variance decomposition shows one graph for the variance of each equation from each source. The horizontal axis tells the number of periods following a shock to which the decomposition applies and the vertical axis gives the fraction of variance explained by the shock source. In this example, most of the variance comes from
the “own-shock” (i.e., G-shocks effect on G), rather than from the shock to the other equation.
Forecasting from VARs
In order to forecast from a VAR, you need to use the model object. An example is given in Simulating VARs in Chapter 15, “Super Models.”
Vector error correction, cointegration tests, structural VARs
VARs have become an important tool of modern econometrics, especially in macroeconomics. Since the User’s Guide devotes an entire chapter to the subject, we’ll just say that the VAR object provides tools that handle everything listed in the topic heading above this paragraph.
Quick Review?
A quick review of EViews’ advanced estimation features suggests that a year or two of Ph.D.-level econometrics would help in learning to use all the available tools. This chapter has tried to touch the surface of many of EViews advanced techniques. Even this extended introduction hasn’t covered everything that’s available. For example, EViews offers a sophisticated state-space (Kalman filter) module. As usual, we’ll refer you to the User’s Guide for more advanced discussion.