
- •Table of Contents
- •Foreword
- •Chapter 1. A Quick Walk Through
- •Workfile: The Basic EViews Document
- •Viewing an individual series
- •Looking at different samples
- •Generating a new series
- •Looking at a pair of series together
- •Estimating your first regression in EViews
- •Saving your work
- •Forecasting
- •What’s Ahead
- •Chapter 2. EViews—Meet Data
- •The Structure of Data and the Structure of a Workfile
- •Creating a New Workfile
- •Deconstructing the Workfile
- •Time to Type
- •Identity Noncrisis
- •Dated Series
- •The Import Business
- •Adding Data To An Existing Workfile—Or, Being Rectangular Doesn’t Mean Being Inflexible
- •Among the Missing
- •Quick Review
- •Appendix: Having A Good Time With Your Date
- •Chapter 3. Getting the Most from Least Squares
- •A First Regression
- •The Really Important Regression Results
- •The Pretty Important (But Not So Important As the Last Section’s) Regression Results
- •A Multiple Regression Is Simple Too
- •Hypothesis Testing
- •Representing
- •What’s Left After You’ve Gotten the Most Out of Least Squares
- •Quick Review
- •Chapter 4. Data—The Transformational Experience
- •Your Basic Elementary Algebra
- •Simple Sample Says
- •Data Types Plain and Fancy
- •Numbers and Letters
- •Can We Have A Date?
- •What Are Your Values?
- •Relative Exotica
- •Quick Review
- •Chapter 5. Picture This!
- •A Simple Soup-To-Nuts Graphing Example
- •A Graphic Description of the Creative Process
- •Picture One Series
- •Group Graphics
- •Let’s Look At This From Another Angle
- •To Summarize
- •Categorical Graphs
- •Togetherness of the Second Sort
- •Quick Review and Look Ahead
- •Chapter 6. Intimacy With Graphic Objects
- •To Freeze Or Not To Freeze Redux
- •A Touch of Text
- •Shady Areas and No-Worry Lines
- •Templates for Success
- •Point Me The Way
- •Your Data Another Sorta Way
- •Give A Graph A Fair Break
- •Options, Options, Options
- •Quick Review?
- •Chapter 7. Look At Your Data
- •Sorting Things Out
- •Describing Series—Just The Facts Please
- •Describing Series—Picturing the Distribution
- •Tests On Series
- •Describing Groups—Just the Facts—Putting It Together
- •Chapter 8. Forecasting
- •Just Push the Forecast Button
- •Theory of Forecasting
- •Dynamic Versus Static Forecasting
- •Sample Forecast Samples
- •Facing the Unknown
- •Forecast Evaluation
- •Forecasting Beneath the Surface
- •Quick Review—Forecasting
- •Chapter 9. Page After Page After Page
- •Pages Are Easy To Reach
- •Creating New Pages
- •Renaming, Deleting, and Saving Pages
- •Multi-Page Workfiles—The Most Basic Motivation
- •Multiple Frequencies—Multiple Pages
- •Links—The Live Connection
- •Unlinking
- •Have A Match?
- •Matching When The Identifiers Are Really Different
- •Contracted Data
- •Expanded Data
- •Having Contractions
- •Two Hints and A GotchYa
- •Quick Review
- •Chapter 10. Prelude to Panel and Pool
- •Pooled or Paneled Population
- •Nuances
- •So What Are the Benefits of Using Pools and Panels?
- •Quick (P)review
- •Chapter 11. Panel—What’s My Line?
- •What’s So Nifty About Panel Data?
- •Setting Up Panel Data
- •Panel Estimation
- •Pretty Panel Pictures
- •More Panel Estimation Techniques
- •One Dimensional Two-Dimensional Panels
- •Fixed Effects With and Without the Social Contrivance of Panel Structure
- •Quick Review—Panel
- •Chapter 12. Everyone Into the Pool
- •Getting Your Feet Wet
- •Playing in the Pool—Data
- •Getting Out of the Pool
- •More Pool Estimation
- •Getting Data In and Out of the Pool
- •Quick Review—Pools
- •Chapter 13. Serial Correlation—Friend or Foe?
- •Visual Checks
- •Testing for Serial Correlation
- •More General Patterns of Serial Correlation
- •Correcting for Serial Correlation
- •Forecasting
- •ARMA and ARIMA Models
- •Quick Review
- •Chapter 14. A Taste of Advanced Estimation
- •Weighted Least Squares
- •Heteroskedasticity
- •Nonlinear Least Squares
- •Generalized Method of Moments
- •Limited Dependent Variables
- •ARCH, etc.
- •Maximum Likelihood—Rolling Your Own
- •System Estimation
- •Vector Autoregressions—VAR
- •Quick Review?
- •Chapter 15. Super Models
- •Your First Homework—Bam, Taken Up A Notch!
- •Looking At Model Solutions
- •More Model Information
- •Your Second Homework
- •Simulating VARs
- •Rich Super Models
- •Quick Review
- •Chapter 16. Get With the Program
- •I Want To Do It Over and Over Again
- •You Want To Have An Argument
- •Program Variables
- •Loopy
- •Other Program Controls
- •A Rolling Example
- •Quick Review
- •Appendix: Sample Programs
- •Chapter 17. Odds and Ends
- •How Much Data Can EViews Handle?
- •How Long Does It Take To Compute An Estimate?
- •Freeze!
- •A Comment On Tables
- •Saving Tables and Almost Tables
- •Saving Graphs and Almost Graphs
- •Unsubtle Redirection
- •Objects and Commands
- •Workfile Backups
- •Updates—A Small Thing
- •Updates—A Big Thing
- •Ready To Take A Break?
- •Help!
- •Odd Ending
- •Chapter 18. Optional Ending
- •Required Options
- •Option-al Recommendations
- •More Detailed Options
- •Window Behavior
- •Font Options
- •Frequency Conversion
- •Alpha Truncation
- •Spreadsheet Defaults
- •Workfile Storage Defaults
- •Estimation Defaults
- •File Locations
- •Graphics Defaults
- •Quick Review
- •Index
- •Symbols
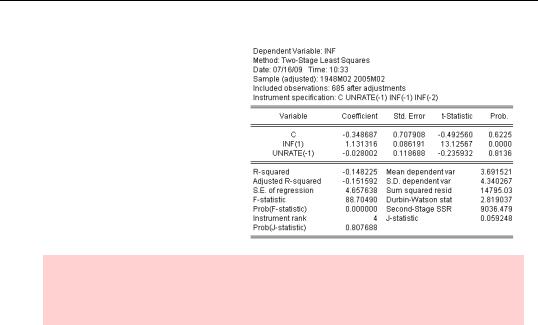
Generalized Method of Moments—347
Thus, to get 2SLS results we can give the command:
tsls inf c inf(1) unrate(-1) @ c unrate(-1) inf(-1) inf(-2)
The coefficient on future inflation is now close to 1.0, as theory predicts. The coefficient on unemployment is negative, albeit small and not significant.
Hint: By default, if you don’t include the constant, C, in the instrument list, EViews puts one in for you. You can tell EViews not to add the constant by unchecking the Include a constant box in the estimation dialog.
Did you notice the R2 in the 2SLS output? It’s negative. This means that the equation fits the data really poorly. That’s okay. Our interest here is in accurate parameter estimation.
Generalized Method of Moments
What happens if you put together nonlinear estimation and two-stage least squares? While EViews will happily estimate a nonlinear equation using the tsls command, nowadays econometricians are more likely to use the Generalized Method of Moments, or GMM.
Two-stage least squares can be thought of as a special case of GMM. GMM extends 2SLS in two dimensions:
•GMM estimation typically accounts for heteroskedasticity and/or serial correlation.
•GMM specification is based on an orthogonality condition between a (possibly nonlinear) function and instruments.
As an example, suppose instead of the tsls command above we gave the gmm command:
gmm inf c inf(1) unrate(-1) @ c unrate(-1) inf(-1) inf(-2)
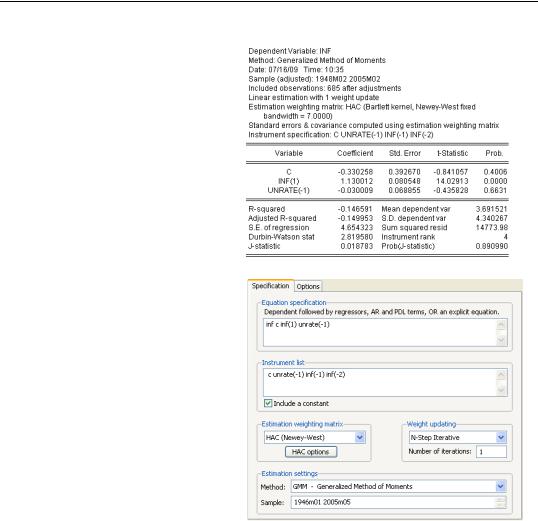
348—Chapter 14. A Taste of Advanced Estimation
The resulting estimate is close to the 2SLS estimate, but it’s not identical. By default, EViews applies one of the many available options for estimation that is robust to heteroskedasticity and serial correlation.
Clicking the button reveals the GMM Specification tab. The entire right side of this tab is devoted to the choice of robust estimation methods. See the User’s Guide for more information.
Orthogonality Conditions
The basic notion behind GMM is that each of the instruments is orthogonal to a specified function. You can specify the function in any of three ways:
•If you give the usual—dependent variable followed by independent variables—series list, the function is the residual.
•If you give an explicit equation, linear or nonlinear, the function is the value to the left of the equal sign minus the value to the right of the equal sign.
•If you give a formula with no equal sign, the formula is the function.
See System Estimation, below, for a brief discussion of GMM estimation for systems of equations.
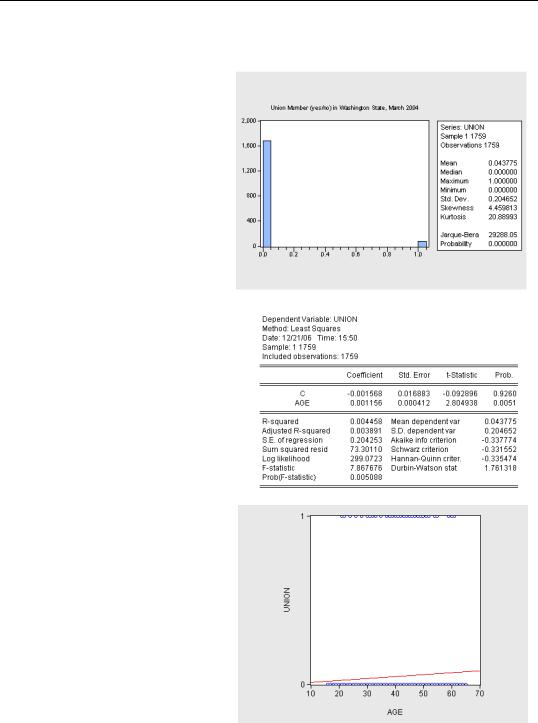
Limited Dependent Variables—349
Limited Dependent Variables
Suppose we’re interested in studying the determinants of union membership and that, coincidentally, we have data on a cross-section of workers in Washington State in the workfile “CPSMAR2004WA.wf1”. The series UNION is coded as one for union members and zero for non-members. Between four and five percent of workers in our sample are members of a union.
Is age an important determinant
of union membership? We might run a regression to see. According to the least squares results, age is highly significant statistically (the t-statistic is 2.8), but doesn’t explain much of the variation in the dependent variable (the R2 is low).
We can also look at the regression on a scatter plot. The dependent variable is all zeros and ones. The predicted values from the regression lie on a continuous line.
While the regression results aren’t necessarily “wrong,” what does it mean to say that predicted union membership is 0.045? Either you are a member of a union, or you are not a member of a union!
This example is a member of a class called limited dependent
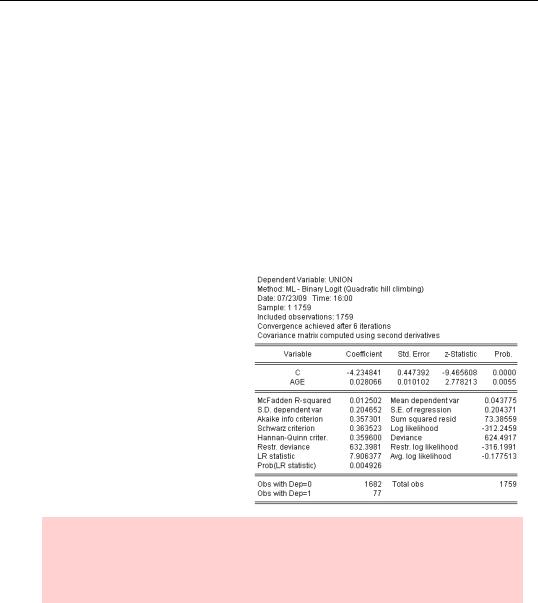
350—Chapter 14. A Taste of Advanced Estimation
variable problems. EViews provides estimation methods for binary dependent variables, as in our union membership example, ordered choice models, censored and truncated models (tobit being an example), and count models. The User’s Guide provides its usual clear explanation of how to use these models in EViews, as well as a guide to the underlying theory.
We’ll illustrate with the simplest model: logit.
Logit
Instead of fitting zeros and ones, the logit model uses the right-hand side variables to predict the probability of being a union member, i.e., of observing a 1.0. One can think of the model as having two parts. First, an index s is created, which is a weighted combination of the explanatory variables. Then the probability of observing the outcome depends on the cumulative distribution function (cdf) of the index. Logit uses the cdf of the logistic distribution; probit uses the normal distribution instead.
The logit command is straightforward:
logit union c age
The coefficients shown in the output are the coefficients for constructing the index. In this case, our estimated model says:
s = – 4.23 + 0.028 × age prob(union= 1) = 1 – F(–s)
F(s)= es ⁄ (1 + es)
Textbook hint: Textbooks usually describe the relation between probability and index in a logit with prob(union= 1)= F(s) rather than
prob(union= 1)= 1 – F(–s) . The two are equivalent for a logit (or a probit), but differ for some other models.