
- •Table of Contents
- •Foreword
- •Chapter 1. A Quick Walk Through
- •Workfile: The Basic EViews Document
- •Viewing an individual series
- •Looking at different samples
- •Generating a new series
- •Looking at a pair of series together
- •Estimating your first regression in EViews
- •Saving your work
- •Forecasting
- •What’s Ahead
- •Chapter 2. EViews—Meet Data
- •The Structure of Data and the Structure of a Workfile
- •Creating a New Workfile
- •Deconstructing the Workfile
- •Time to Type
- •Identity Noncrisis
- •Dated Series
- •The Import Business
- •Adding Data To An Existing Workfile—Or, Being Rectangular Doesn’t Mean Being Inflexible
- •Among the Missing
- •Quick Review
- •Appendix: Having A Good Time With Your Date
- •Chapter 3. Getting the Most from Least Squares
- •A First Regression
- •The Really Important Regression Results
- •The Pretty Important (But Not So Important As the Last Section’s) Regression Results
- •A Multiple Regression Is Simple Too
- •Hypothesis Testing
- •Representing
- •What’s Left After You’ve Gotten the Most Out of Least Squares
- •Quick Review
- •Chapter 4. Data—The Transformational Experience
- •Your Basic Elementary Algebra
- •Simple Sample Says
- •Data Types Plain and Fancy
- •Numbers and Letters
- •Can We Have A Date?
- •What Are Your Values?
- •Relative Exotica
- •Quick Review
- •Chapter 5. Picture This!
- •A Simple Soup-To-Nuts Graphing Example
- •A Graphic Description of the Creative Process
- •Picture One Series
- •Group Graphics
- •Let’s Look At This From Another Angle
- •To Summarize
- •Categorical Graphs
- •Togetherness of the Second Sort
- •Quick Review and Look Ahead
- •Chapter 6. Intimacy With Graphic Objects
- •To Freeze Or Not To Freeze Redux
- •A Touch of Text
- •Shady Areas and No-Worry Lines
- •Templates for Success
- •Point Me The Way
- •Your Data Another Sorta Way
- •Give A Graph A Fair Break
- •Options, Options, Options
- •Quick Review?
- •Chapter 7. Look At Your Data
- •Sorting Things Out
- •Describing Series—Just The Facts Please
- •Describing Series—Picturing the Distribution
- •Tests On Series
- •Describing Groups—Just the Facts—Putting It Together
- •Chapter 8. Forecasting
- •Just Push the Forecast Button
- •Theory of Forecasting
- •Dynamic Versus Static Forecasting
- •Sample Forecast Samples
- •Facing the Unknown
- •Forecast Evaluation
- •Forecasting Beneath the Surface
- •Quick Review—Forecasting
- •Chapter 9. Page After Page After Page
- •Pages Are Easy To Reach
- •Creating New Pages
- •Renaming, Deleting, and Saving Pages
- •Multi-Page Workfiles—The Most Basic Motivation
- •Multiple Frequencies—Multiple Pages
- •Links—The Live Connection
- •Unlinking
- •Have A Match?
- •Matching When The Identifiers Are Really Different
- •Contracted Data
- •Expanded Data
- •Having Contractions
- •Two Hints and A GotchYa
- •Quick Review
- •Chapter 10. Prelude to Panel and Pool
- •Pooled or Paneled Population
- •Nuances
- •So What Are the Benefits of Using Pools and Panels?
- •Quick (P)review
- •Chapter 11. Panel—What’s My Line?
- •What’s So Nifty About Panel Data?
- •Setting Up Panel Data
- •Panel Estimation
- •Pretty Panel Pictures
- •More Panel Estimation Techniques
- •One Dimensional Two-Dimensional Panels
- •Fixed Effects With and Without the Social Contrivance of Panel Structure
- •Quick Review—Panel
- •Chapter 12. Everyone Into the Pool
- •Getting Your Feet Wet
- •Playing in the Pool—Data
- •Getting Out of the Pool
- •More Pool Estimation
- •Getting Data In and Out of the Pool
- •Quick Review—Pools
- •Chapter 13. Serial Correlation—Friend or Foe?
- •Visual Checks
- •Testing for Serial Correlation
- •More General Patterns of Serial Correlation
- •Correcting for Serial Correlation
- •Forecasting
- •ARMA and ARIMA Models
- •Quick Review
- •Chapter 14. A Taste of Advanced Estimation
- •Weighted Least Squares
- •Heteroskedasticity
- •Nonlinear Least Squares
- •Generalized Method of Moments
- •Limited Dependent Variables
- •ARCH, etc.
- •Maximum Likelihood—Rolling Your Own
- •System Estimation
- •Vector Autoregressions—VAR
- •Quick Review?
- •Chapter 15. Super Models
- •Your First Homework—Bam, Taken Up A Notch!
- •Looking At Model Solutions
- •More Model Information
- •Your Second Homework
- •Simulating VARs
- •Rich Super Models
- •Quick Review
- •Chapter 16. Get With the Program
- •I Want To Do It Over and Over Again
- •You Want To Have An Argument
- •Program Variables
- •Loopy
- •Other Program Controls
- •A Rolling Example
- •Quick Review
- •Appendix: Sample Programs
- •Chapter 17. Odds and Ends
- •How Much Data Can EViews Handle?
- •How Long Does It Take To Compute An Estimate?
- •Freeze!
- •A Comment On Tables
- •Saving Tables and Almost Tables
- •Saving Graphs and Almost Graphs
- •Unsubtle Redirection
- •Objects and Commands
- •Workfile Backups
- •Updates—A Small Thing
- •Updates—A Big Thing
- •Ready To Take A Break?
- •Help!
- •Odd Ending
- •Chapter 18. Optional Ending
- •Required Options
- •Option-al Recommendations
- •More Detailed Options
- •Window Behavior
- •Font Options
- •Frequency Conversion
- •Alpha Truncation
- •Spreadsheet Defaults
- •Workfile Storage Defaults
- •Estimation Defaults
- •File Locations
- •Graphics Defaults
- •Quick Review
- •Index
- •Symbols
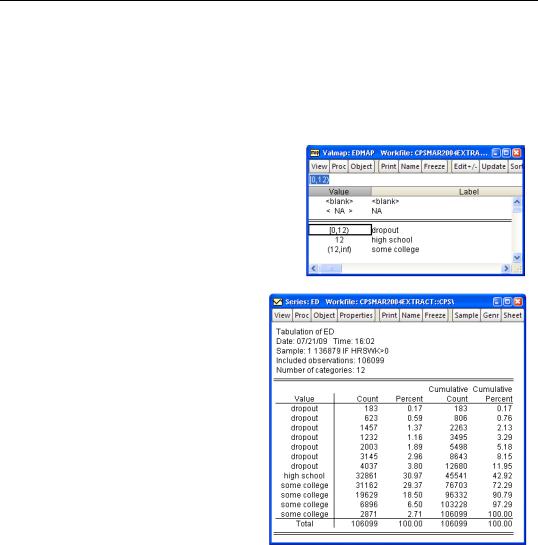
112—Chapter 4. Data—The Transformational Experience
Many-To-One Mappings
Value maps can be used to group a range of codes for the purpose of display. Instead of a single value in the value map, enter a range in parentheses. For example “(-inf, 12)” specifies all values less than 12. Parentheses are used to specify open intervals, square brackets are used for closed intervals. So “(-inf, 12]” is all values less than or equal to 12.
The series ED in “CPSMAR2004Extract.wf1” measures education in years. We could use the value map shown to the right to group education into three displayed values.
The many-to-one mapping is only for display purposes. If we analyze the data, all the underlying categories are still there. For example, here’s a tabulation of ED. Everyone with less than a high school education is labeled “dropout,” but they’re still tabulated in separate categories according to the years of education they’ve had. That’s why we see seven “dropout” rows on the right.
Relative Exotica
EViews has lots of functions for transforming data. You’ll never need most of these functions, but the one you do need you’ll need bad.
Stats-By
We met several data summary functions such as @mean above in the section Functions Are Where It’s @. Sometimes one wants a summary statistic computed by group. We might want a series that assigned the mean education for women to women and the mean education for men to men. This is accomplished with the Stats-By family of functions: @meansby(x,y),
@mediansby(x,y), etc. (See the Command and Programming Reference for more func-
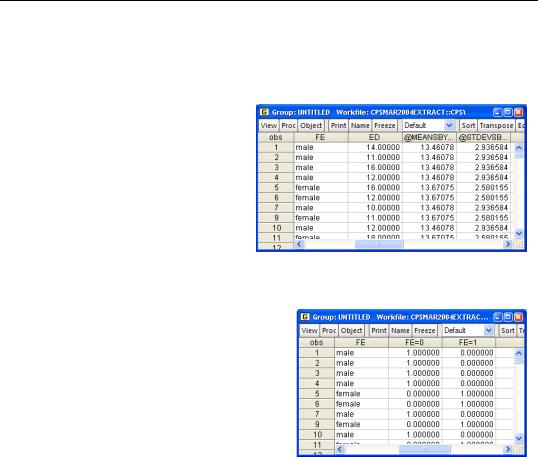
Relative Exotica—113
tions.) These functions summarize the data in X according to the groups in Y. (Optionally, a sample can be used as a third argument.) Thus the command:
show fe ed @meansby(ed,fe) @stdevsby(ed,fe)
shows gender and years of education followed by the mean and standard deviation of education for women if the individual is female, and the mean and standard deviation of education for men if the individual is male.
Expand the Dummies
The @expand function isn’t really a data transformation function at all. Instead, @expand(x) creates a set of temporary series. One series is created for each unique value of X and the value of a given series is 1 for observations where X equals the corresponding value. For example, @expand(fe) creates two series in the command:
show fe @expand(fe)
If you give @expand more than one series as
an argument, as in @expand(x,y,z), series are created for all possible combinations of the values of the series.
The primary use of @expand is as part of a regression specification, where it generates a complete set of dummy variables. Because it’s often desirable to omit one series from the complete set, @expand can take an optional last argument, @dropfirst or @droplast. The former omits the first category from the set of series generated and the latter omits the last category. (For more detail, see the Command and Programming Reference).
@expand can also be used in algebraic expressions, with each resulting temporary series being inserted in the expression in turn. The command,
ls lnwage c ed*@expand(fe)
is equivalent to:
ls lnwage c ed*(fe=0) ed*(fe=1)
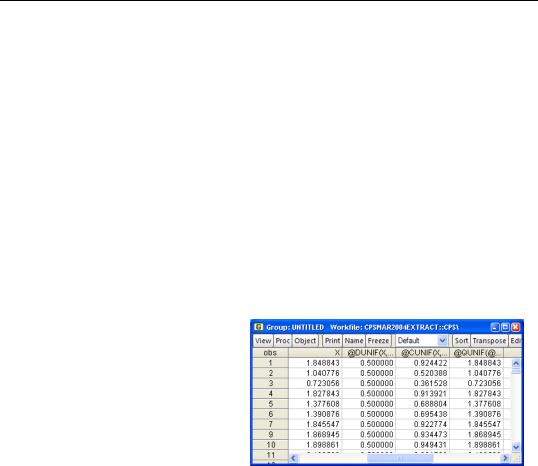
114—Chapter 4. Data—The Transformational Experience
and estimates separate returns to education for men and women.
Statistical Functions
Uniform and standard normal random number generators were described earlier in the chapter. EViews supplies families of statistical functions organized according to specific probability distributions. A function name beginning with “@r” is a random number generator, a name beginning with “@d” evaluates the probability density function (also called the “pdf”), a name beginning with “@c” evaluates the cumulative distribution function (or “cdf”), and a name beginning with “@q” gives the quantile, or inverse cdf. In each case, the @-sign and initial letter are followed by the name of the distribution.
As an example, the name used for the uniform distribution is “unif.” So @runif(a,b) generates random numbers distributed uniformly between A and B. (This means that @rnd is a synonym for @runif(0,1).) We can make up an example with:
series x = @runif(0,2)
show x @dunif(x,0,2) @cunif(x,0,2) @qunif(@cunif(x,0,2),0,2)
which randomly resulted in the data shown to the right. The first column, X, is a random number randomly distributed between 0 and 2. The second column gives the pdf for X, which for this distribution always equals 0.5. The third column gives the cdf. Just for fun, the last column reports the inverse cdf of the cdf, which is the original X, just as it should be.
A variety of probability distributions are discussed in the Command and Programming Reference. Probably the most commonly used are “norm,” for standard normal, and “tdist,” for Student’s t. Here are a few examples:
=@qtdist(.95, 30) = 1.69726
=@qtdist(0.05/2, 30) = -2.04227
=@qnorm(.025) = -1.95996
Quick Review
Data in EViews can be either numbers or text. A wide set of data manipulation functions are available. In particular, the “expected” set of algebraic manipulations all work as expected. You can use numbers to conveniently represent dates. EViews also provides control over the

Quick Review—115
visual display of data. Value maps and display formats play a big role in data display. In particular, value maps let you see meaningful labels in place of arbitrary numerical codes.

116—Chapter 4. Data—The Transformational Experience