
Словари и журналы / Психологические журналы / p63British Journal of Mathematical and Statistical Psycholog
.pdf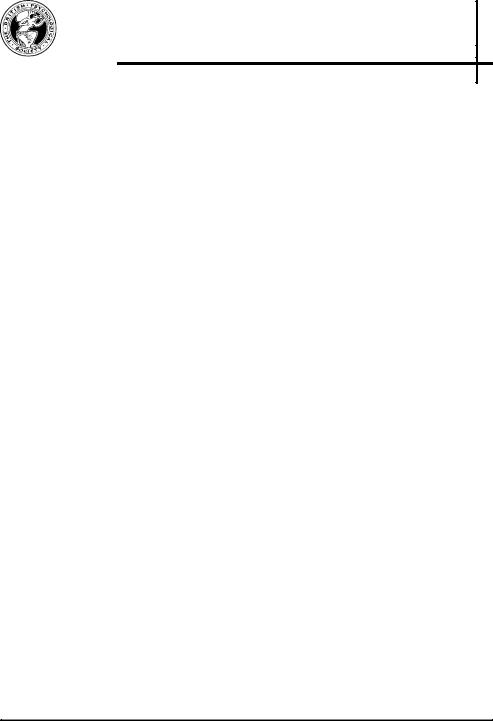
63
British Journal of Mathematical and Statistical Psychology (2002), 55, 63–91
© 2002 The British Psychological Society
www.bps.org.uk
Outcomes and sample selection: The case of a homelessness and substance abuse intervention
Michael R. Sosin*
School of Social Service Administration, University of Chicago, USA
In the likely event that some clients refuse to participate in a psychosocial Ž eld experiment, the estimates of the effects of the experimental treatment on client outcomes may suffer from sample selection bias, regardless of whether the statistical analyses include control variables. This paper explores ways of correcting for this bias with advanced correction strategies, focusing on experiments in which clients refuse assignment into treatment conditions. The sample selection modelling strategy, which is highly recommended but seldom applied to random sample psychosocial experiments, and some alternatives are discussed. Data from an experiment on homelessness and substance abuse are used to compare sample selection, conventional control variable, instrumental variable, and propensity score matching correction strategies. The empirical Ž ndings suggest that the sample selection modelling strategy provides reliable estimates of the effects of treatment, that it and some other correction strategies are awkward to apply when there is post-assignment rejection, and that the varying correction strategies provide widely divergent estimates. In light of these Ž ndings, researchers might wish regularly to compare estimates across multiple correction strategies.
1. Introduction
Psychologists often rely on random sample Želd experiments to verify theoretical propositions about the beneŽts of psychosocial treatments. Nevertheless, these experiments can be fraught with technical difŽculties. Perhaps one of the most signiŽcant is that the experiments can suffer from a type of sample selectivity that biases, or distorts, the statistical estimates of the effects of the experimental treatments on key client outcomes (behaviours). This is the case even when randomization is attempted.
*Requests for reprints should be addressed to Michael R. Sosin, School of Social Service Administration, University of Chicago, 969 E. 60th Street, Chicago, IL 60637, USA.
64 Michael R. Sosin
This potential for sample selection bias occurs under verycommon conditions, such as when some clients fail to participate in the Želd experiment and the ‘refusers’ have different characteristics from the acceptors (Baekeland & Lundwall, 1975; Becker & Maiman, 1975; Silberfeld & Glaser, 1978; Hickson, Mangione, Myers, & Scotch, 1982; Olkin & Lemle, 1984; Rosenstock, 1985; Noel, McCrady, Stout, & Fisher-Nelson, 1987; Powell et al., 1987; Mohl, Martinex, Ticknor, & Appleby, 1989; Copeland & Hall, 1992; Stark, 1992; Moak et al., 1993; Roffman et al., 1993; Simpson & Joe, 1993; Blood & Cornwall, 1994). Indeed, this is particularly clear (and common) in the experiments of interest here, in which there is ‘post-assignment’ refusal, whereby some clients fail to enrol in the treatment conditions to which they are assigned. Bias is clearly a risk in these experiments if the clients who actually participate in the control and the experimental conditions differ in traits or, as is explained below, if the acceptors and refusers differ in traits as a whole.
Alarmingly, and perhaps surprisingly, statisticians suggest that this and other types of sample selection bias are likely to endure when popular theoretical and analysis strategies are employed—for instance, when no attempt is made to generalize the Žndings beyond the sample of participating clients, when control variables are used to compensate for the differences between the clients who accept and refuse services, and when researchers use ‘intent-to-treat’ analyses in which the refusers are incorporated as if they participated (Breen, 1996; Berk, 1983; Maddala, 1983). In essence, statisticians imply that caution must be exercised in interpreting conventionally calculated estimates of the effects of treatment in the broad range of experiments whose estimating samples do not perfectly represent their populations (Berk, 1983).
Of course, various advanced analyses strategies may be used to correct for sample selection bias. Perhaps one of the most heavily recommended is the sample selection modelling strategy (Heckman, 1976, 1979; Hausman & Wise, 1977; Willis & Rosen, 1979; Maddala, 1983; Tienda, Smith, & Ortiz, 1987; Breen, 1996). This strategy predicts outcomes when holding constant a special, particularly appropriate term or set of terms. It provides estimates that can be used to increase or reduce the researcher’s conŽdence in the conventionally calculated results. However, the modelling strategy is rarely applied to psychosocial Želd experiments, particularly when there is postassignment selectivity. This might re•ect the difŽculty of application, but is still regrettable since it leaves extra doubt about the possible implications of bias.
Furthermore, there is little existing work on comparing estimates under sample selection and other correction strategies. This too is regrettable because it leaves doubt over the consequences of choosing a particular approach to bias correction. The matter is important because it may be that the sample selection strategy, which relies on severe assumptions about the limits of conventional analyses, comes to nearly the same empirical conclusions as strategies that are more forgiving.
This paper further explores the application of the sample selection modelling strategy and its alternatives for random experiments that have post-assignment selectivity. It explains why there can be bias in estimates of the effects of treatment, how sample selection models attempt to correct for that bias, the difŽculties that arise in using the sample selection modelling strategy in this manner, and possible ways of overcoming the difŽculties. It then analyses data from one experiment to assess whether the sample selection modelling strategy can be applied and whether it locates sufŽcient biases to warrant application. Finally, it considers how estimates vary across correction strategies.

Outcomes and sample selection |
65 |
2. Background
Conventional ‘control variable’ models predict the outcomes of interest with a variable (or variables) that represents the treatment condition, and with control variables that ostensibly hold constant the factors on which the treatment and control groups are likely to differ (analyses of variance are statistically similar). However, proponents of sample selection corrections suggest that bias problems may remain in these models when experiments have post-assignment selection and refusal is systematic. This is most clearly explained with respect to an experiment that only has information on the individuals who accept assignment into the treatment conditions.
Statisticians argue that the control variables in such an experiment are likely to undercorrect for the true differences between the acceptors and refusers. This re•ects the fact that the control variables rarely fully account for all of the factors that distinguish the acceptors and refusers; some of these factors will be unknown or perhaps even virtually unknowable, while others will not be perfectly represented in any realworld data set. The use of control variables thus can lead to an unknown degree of underor overestimation of the effects of the experimental treatment on outcomes.
The statisticians also suggest that estimates of the effects of treatment are likelyto be biased if the relations between the measured independent (control or experimental) and outcome variables are different among the acceptors and the refusers (when known factors are held constant). Here, the estimated relations will not precisely represent the population’s relations. Moreover, there may be powerful reasons to suspect that acceptors and refusers routinely react differently to, and thus evince different relations among, the same variables; after all, the two groups even have different reactions to the offer of services (and this is not fully accounted for by known variables).1
Statistically speaking, the selection problem means that the regression that predicts the effects of a treatment on an outcome is plagued by a correlation between the residuals and dependent variable; the regression line is biased compared to the line that would emerge if all members of the sample participated.2 This is a major concern, because regressions are accurate only when there is no correlation. Further, the circumstances that were mentioned in the Introduction may similarly spur violations of the assumptions that allow for accurate regressions. In particular, there is a risk of bias when the outcome’s mean score or even variance differs for acceptors and refusers (regardless of whether the acceptors of each intervention condition differ in traits), in that the computed means and variances in the regression estimation formula will be distorted when only analysing the acceptors (Heckman, 1976; Berk, 1983; Maddala, 1983; Breen, 1996). Bias may exist when the researcher does not generalize beyond the sample of acceptors, in that there will be a correlated error term within that sample. For example, the distribution of the dependent variable is likely to be truncated, and this distorts the variance, at least (Berk, 1983).
Researchers mayattempt to deal with a bias problem byemploying the intent-to-treat strategy (Gueron & Pauly, 1991), whereby the refusers are followed up, and their scores
1For example, the true results might be that mental health problems increase substance abuse for acceptors, but that they do not have this effect for refusers (when they enter an experiment). This implies that inaccuracies may occur when controlling for mental health problems and predicting the level of substance abuse within the sample of acceptors.
2That is, if the true equation (the one that includes both acceptor and refusers) has a well-behavederror term with zero mean and no correlation to the dependent variable, the selected sample may not. For example, if the correlation between mental health symptoms and substance abuse is positive only in the sample of acceptors, then the regression line estimated from the acceptors will have too many negative residuals at high levels of substance abuse symptoms, and too many positive residuals at low levels of symptoms, compared to what would exist in the complete sample.
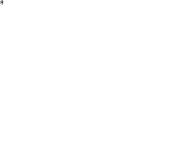
66 Michael R. Sosin
on outcome variables are combined with the scores of the members of the treatment groups to which they originally were assigned. But that does not necessarily offer a full solution, not least because the inclusion of the refusers may be unfair, in that it incorporates the outcomes of individuals who lack treatment. Further, while certain researchers argue that the intent-to-treat strategy still estimates how the entire population fares, some statisticians worry that it can instead incorrectly calculate relations (thus distorting the estimates). For instance, in the example application considered later in this paper, if an experiment teaches clients new ways of coping with personal problems, that knowledge should affect the wayin which their mental health symptoms relate to their substance abuse outcomes. But because the refusers lack that training, they may evince different relations between mental health symptoms and substance abuse. In general, it can be argued that analyses that include data from the refusers still might provide inaccurate estimates of the relations among control variables, and of the coefŽcients attached to variables that correlate with the control variables— including the variable measuring the treatment effect. The example reported below also questions the common claims that intent-to-treat approaches only have a downward estimation bias, and do not unduly suppress estimates because post-assignment refusers would not beneŽt from treatment if they participated.
2.1. Sample selection models
The apparent advantages of correcting bias with the sample selection modelling strategy can be illustrated by considering the most straightforward model. This has two equations. The Žrst, the selection equation, uses a probit model to predict acceptance against refusal. The second, the outcome equation, uses regression among the acceptors to predict the impact of the experimental variables on the outcome. The outcome equation also may contain other predictors.
In the straightforward sample selection model, the outcome equation corrects for selection by controlling for lambda (l). This statistic is the inverse Mills ratio: the density of the predicted probability of refusal as estimated from the selection equation, divided by one minus the distribution of this probability. Conceptually speaking, controlling for lambda compensates for the characteristics of the refusers because it provides higher scores to individuals who are most like them (Berk, 1983).
The sample selection strategy can be more formally explained following Maddala (1983), Greene (1991) and Breen (1996). Assume there is an underlying continuous selection process that results in a theoretical propensity to refuse as measured by a
variable called z . This propensity is predicted by a matrix of variables, w i |
(for each |
subject i; the subscript is assumed for z ), a matrix of coefŽcients, a¢, and an error term: |
|
z = a¢w i + ui . |
(1) |
Because there is selection, the underlying equation is only observable as a probit equation. This can be viewed as predicting an observed z, where a score of zero represents refusal and a score of one represents acceptance:
z = |
» |
0 |
z |
<0. |
|
|
1 |
z |
> 0, |
Note that, to calculate lambda in the correct way, the above equation is set up to predict acceptance. The remainder of this paper thus presents issues in such terms.
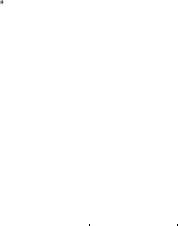
Outcomes and sample selection |
67 |
Assume also that the underlying outcome equation predicting the outcome y is represented as
y = b¢x i + ei , |
(2) |
where b¢ is a matrix of coefŽcients and x i is a matrix of variables (including the dummy variable indicating whether an individual is enrolled in an experimental or control condition). The equation only is observable as y when z = 1.
Given these equations, the desired result is the following expected value of y:
E[ y| x i in sample] = E[ y| x i , z = 1]
=E[ y| x i , a¢w i + ui > 0]
=b¢x i + E[ei | ui > ± a¢w i ].
Statistical theory then suggests the expected value of a variable in a bivariate distribution (e) conditional on another (u):
E[ y| x i in sample] = b¢x i + rjeju |
»f³[1 ± F(± ai¢w i )]´¼. |
(3) |
|
|
|
± a¢w |
|
In (3), r is the correlation between the error terms of the selection and outcome equations, f is the density function of the selection equation, F is the distribution function of the selection equation, je is the standard deviation of the outcome variable in equation (3), and ju is the standard deviation of the selection equation. The second standard deviation is conventionally set at one; its value, which cannot be determined, does not affect the other parameters. Thus rjeju reduces to rje, otherwise abbreviated here as v. Given the deŽnition of the inverse Mills ratio, (3) may thus be written in its common form as
E[ y| x i in sample] = b¢x i + vl. |
(4) |
When applied to a sample, a regression is used to estimate b¢, v and a random error term (Heckman, 1976, 1979; Berk, 1983; Maddala, 1983; Breen, 1996).
The entire outcome equation (4) estimates coefŽcients with selection controlled. It also provides rho, which in the example at hand is the estimated correlation between outcomes and the propensity to refuse. Rho can be used to help determine how refusers fare if they accept the intervention. The selection and outcome equations also can be used together to estimate sample members’ predicted scores on the outcome.
Sample selection models can be unbiased when the selection equation fails to include every predictor of acceptance or refusal. However, their accuracy depends on other assumptions. One is that there is ‘self-selection’, by which individuals varyin their propensity to refuse along a continuous scale. Asecond is that the selection equation is unbiased. Athird is that the rejecters and acceptors come from a single distribution. But even if these assumptions are accepted, three statistical problems can arise in the experiments of interest. The problems and their possible solutions are reviewed below.
2.2. Sample selection identiŽ cation problems
The Žrst issue is that the selection equation generallymust include at least one predictor that is not used in the outcome equation. This is necessary because sample selection models cannot be estimated when the selection and outcome equations are linear forms of each other, and other possible ways of adjusting for this tend to fail empirically

68 Michael R. Sosin
(Stolzenberg & Relles, 1997). The requirement is more than a technical matter; the included variable or variables must truly predict the decision to accept or reject services and must truly fail to predict the outcome. Thus, the Žrst problem is that, for obvious reasons,3 it is not possible to choose such variables empirically.
The solution to this problem must be to develop a convincing theoretical justiŽcation for the choice of variables. Fortunately, this can be reasonably straightforward for many experiments. For example, a strong theory may suggest that clients accept treatment more readily when they perceive that their beneŽts are greater and their costs are lower. This gives rise to three sets of hypotheses: clients accept the intervention’s treatment more readily when they are exposed to recruitment techniques that portray the beneŽts of treatment in a convincing way (the techniques may vary over time in experiments); clients accept less readily when they know about alternate sources of help and thus have less reason to believe they will gain a unique beneŽt from the intervention; and clients accept the treatment more readily when they receive supportive ‘cues’ from the environment, such as the referral of an expert. It also is possible to posit that acceptance is affected by the motivation to participate in treatment.
As is required, the theory may suggest that these perceived costs and beneŽts, as well as certain types of motivation, do not affect treatment outcomes (and thus can be kept out of the outcome equation). For example, it can be posited that alcohol use outcomes are not affected by the techniques that are used to recruit individuals into treatment or by the availability of outside sources of help; such matters are irrelevant once clients receive services. Of course, as is generally the case for statistical strategies, results are only as convincing as the theory and measures employed.
2.3. Tobit analysis problems and sample selection
The second problem arises because scores on the outcome variable may tend to bunch at zero. This occurs commonly because a successful intervention can completelyreduce many clients’ symptoms, but it means that the distribution of the dependent variable is not normal. Accordingly, the straightforward sample selection model can be inaccurate because it relies on a regression, even though the distribution of the outcome variable can violate an assumption that justiŽes this.
Fortunately, this problem can be handled by altering the form of the model since one of the many available options (Winship & Mare, 1992) is to use a tobit regression outcome equation (Tobin, 1958; Breen, 1996). Under the tobit, the bunched scores at a lower (or upper) ‘bound’ are allowed to vary further. This variation is calculated with the aid of information on the general relations between the outcome variable and the independent variables.
As noted in the Appendix, where the estimation method for a tobit sample selection model is outlined, the method continues to estimate the outcome coefŽcients, rho and sigma. Correction is accomplished with the aid of a series of terms that make use of the Mills ratio in a complex way. Nevertheless, the equation corrects in the general manner that was explained above, essentially by controlling for the propensity to reject the intervention.
3These assumptions cannot be veriŽed by estimating a sample selection model when including the same variables in the selection and outcome equation.

Outcomes and sample selection |
69 |
2.4. Sample selection model estimation
The third problem is most relevant to experiments that have post-assignment selectivity. It can be explained in three steps. First, recall that any experiment has at least two conditions, an experimental and control condition. Each condition may require a separate correction term; if refusal occurs after randomization, then selectivity may be of a different kind in each condition.
Second, consider that there are two widely used estimation techniques for sample selection models in general. One uses a maximum likelihood approach to estimate the selection and outcome equations simultaneously. An alternative, computationally less expensive, two-stage technique (Heckman, 1979) is sequential: it estimates the selection equation, calculates the inverse Mills ratio on its basis, estimates the outcome equation using this ratio, and corrects the error terms in the outcome equation. But the only known model for dealing with differential sample selection among multiple treatment conditions relies on a two-stage technique, by which different selection equations are estimated for each condition.
Finally, consider that this two-stage technique cannot be applied to tobit analyses, which always require maximum likelihood estimation. Indeed, it may be worth noting that maximum likelihood estimation might be statistically preferable in general since simulations raise doubt over the accuracy of two-stage corrections, while so far providing consistent support for maximum likelihood corrections.4 The trend among experts is to avoid two-stage approaches. In any case, the third problem is that it is difŽcult to estimate an accurate, maximum likelihood model for the experiments of interest.
The solution may be to use a maximum likelihood sample selection model for experimental situations in which there are special ways of applying it. For example, that model may be used when individuals refuse the experimental condition but do not refuse assignment into a control condition (in which no treatment is required). In this case, a single selection equation can be estimated in a maximum likelihood framework. The selection equation will include a binary variable (zero for ‘no’, one for ‘yes’) indicating whether each individual is in a given experimental condition, along with interaction terms that multiply this variable by each of the variables that predicts acceptance. The selection equation then estimates the effect of each variable on the probability of acceptance, but onlyfor the individuals who are in the given experimental
4Monte Carlo simulations provide ambiguous evidence about the accuracy of the two-stage technique for regressions. The simulation by Stolzenberg and Relles (1990), which was particularly instrumental in raising the controversy, found that twostage corrections are generally no more accurate than control variable estimators, and that they can be less accurate under certain circumstances. As Breen (1996) insightfully suggests, earlier Monte Carlo results by Nelson (1984) anticipated the Ž nding, but contained sufŽ cient simulations to bound some of the conditions under which it might occur. Essentially, these results suggest that the two-stage model may be more accurate (less biased) than control function estimators when rho is high, and when the independentvariablesin the selection equation explain little of the variance in the independentvariablesthat are included in the outcome equation. The two-stage method also tends to be more accurate when there are fewer refusers (Breen, 1996). Indeed, many of these are also conditions where there is more bias in the control function estimators. However, these are also the conditions under which the maximum likelihood estimator clearly is more efŽ cient (it Ž nds lower variances) than the two-stage estimator. Moreover, Nelson’s (1984) simulations suggest that the maximum likelihood estimator, but not necessarily the two-stage estimator, reduces bias if there is some selectivity, and if the explained variance in the outcome equation is less than 0.9. To be sure, there are an insufŽcient number of simulations to provide exact guidance on the relative attributes of estimators. However, the above and other work (Hartman, 1991) suggests that the two-stage approach generally is less efŽ cient than the maximum likelihood approach and may at times be less accurate than a control variable estimator, and that the maximum likelihood approach tends to be more accurate than control function estimators when there is some bias (Stolzenberg and Relles, 1990; Hartman, 1991; Stolzenberg and Relles, 1997; Nelson, 1984). This may imply that it is prudent to use the maximum likelihood approach.
70 Michael R. Sosin
condition. Further, this equation provides scores of zero on all predictors for the individuals who are in the control condition. This means that its intercept represents the predicted probability of acceptance for such clients (when transformed by manipulation of probit coefŽcients). If the estimation process works, this should be virtually 1.
However, when this procedure is implemented, clients who are not in the treatment condition never have a ‘no’ score on acceptance, and a probit-style selection equation is problematic whenever there is such an ‘empty cell’. Good programs can estimate the results, but there will be huge standard error for the independent variable that has an empty cell (that is, for the variable representing whether a client is in an experimental treatment).
The estimates of the effects of treatment that are derived from the selection equation would be corroborated, and thus would inspire more conŽdence, if at least two additional Žndings emerge. First, results would be partly supported if the intercept in the selection equation indeed translates into a predicted probability of acceptance of almost 1 for control clients. Second, results would be corroborated if the expected values from the outcome analysis are replicated (for a given intervention condition) by a sample selection model that predicts the outcome for a single intervention condition. This replicated model would leave out the variable that represents the intervention condition, while it would not require interaction terms (it only includes one experimental condition). The calculation problems thereby would be avoided. Corroborative analyses thus seem to be worth pursuing.
2.5. Other correction strategies
The majority of other popular correction strategies estimate the outcomes of the refusers as if they were ‘missing at random’ data. This means the strategies estimate the outcome scores of refusers, which essentially are missing data (the true scores are missing), under the assumption that acceptors and refusers react similarly to the variables that are found in the data set. The strategies therefore use the scores of acceptors (respondents) to estimate the scores of refusers (non-respondents) by such techniques as mean score substitution, ‘hot deck’ substitution, a weighting of responses to re-establish the theoretically or methodologically determined proportions of various groupings of respondents, estimation of missing scores (outcomes) from regressions that predict the outcomes among the acceptors, propensity score matching, or maximum likelihood techniques that replace the missing values with estimates that are based on observed relationships (Little & Rubin, 1987). Other strategies provide conŽdence intervals around estimates. The most common of these is Rubin’s mixture model—see Rubin (1977) and Land and McCall (1993); see also Manski’s (1989, 1990) nonparametric technique.
These strategies may be useful in different ways. For example, when searching for a point estimate of the effect of treatment, the regression technique may distort the variance, because it provides the same score on the outcome to individuals who have the same set of traits. The maximum likelihood or so-called EM technique should not have this problem. But for now, one key issue is that all of the strategies that treat the outcome scores as if they are missing at random tend to be inaccurate if the acceptors and refusers truly do not react similarly to the measured variables. A second key issue is that strategies that calculate conŽdence intervals, while informative, can provide wide intervals when many individuals refuse treatment.5 In sum, the sample selection model belongs to a small class of strategies (another of which will be discussed below) that deal

Outcomes and sample selection |
71 |
with situations where there is concern over whether acceptors and refusers react differently to measured variables, and where there is a desire for a point estimate of the effects of the experiment.
2.6. Analysis plan
There may be doubt over whether the sample selection strategy can affect results sufŽciently to be worth the considerable effort. Further, the statistical problems may give rise to some uncertainty over whether the sample selection modelling strategy can be employed in experiments that have post-assignment selectivity. It also is not clear whether the somewhat computationally simpler strategies that assume that the outcome data are missing at random provide similar or different Žndings, and thus whether the choice of a strategy is of particular consequence.
In light of these questions, this paper examines the sample selection model in a complex way. First, the sample selection modelling strategyis applied to an experiment to determine whether its use appreciably alters estimates of the effects of treatment. Second, the two types of corroborative analysis are undertaken to determine if the results may be reliable. Finally, the estimates of the effects of experiment treatment that arise when using the sample selection and various other point-estimate correction strategies are compared.
3. Example application
3.1. Experimental design
The Želd experiment of interest was designed to consider whether homeless clients with substance abuse problems reduce drinking, drug use and homelessness when they are provided ‘progressive independence’ case management and housing services (Sosin, Schwingen, & Yamaguchi, 1993; Sosin et al., 1994; Marlatt & Gordon, 1985). Its sample was gathered between March 1991 and October 1992, when all clients from three key Chicago inner-city short-term (21to 28-day) alcohol and drug treatment programmes were screened for homelessness. Those clients who met speciŽed criteria6 were apportioned into a housing condition, a case-management condition and a control condition. The Žrst condition provided case-management services and access to a set of apartments; the second combined the case-management package with aid in locating community housing. Clients in the control (comparison) condition were told about community resources (Moos & Finney, 1983; Moos, 1984; Hopper & Baumohl, 1994).
While arguably a side-issue here, it may be worth noting that randomization was handled in a complex way in this experiment. First, during each recruitment visit, clients who were in one treatment site were screened for a single experimental condition. Second, across recruitment visits to a site, the clients were screened for varying conditions. These procedures were recommended byrecruitment site directors, who did not want to stir up controversies by allowing clients in a single cohort to be
5Despite some debate about this (Wainer, 2000), it also is not feasible to apply the one mixture model that calculates the responses for all refusers based on the pattern of responses that is found in a subset whose outcome scores can be determined; it is rarely possible to directly measure how a subset of refusers reacts to an experiment.
6These included a rarely used criterion for imminent homelessness, as well as criteria for current or previous homelessness (three months in the last two years).

72 Michael R. Sosin
offered different treatment options. According to multiple tests by researchers from the project and the funding agency, these procedures did not create a measurable bias.
Sites were more frequentlysampled for the case-management intervention, and were least frequently sampled for the housing intervention. This was accomplished to offset anticipated differences in rates of post-assignment refusal. Sample weights are used here to compensate for the fact that different numbers of recruitment visits were made to each site.
Selectivity
Only six individuals who were recruited to this experiment rejected randomization, and none of the 187 assigned clients refused the control condition by speciŽcally declining future contact for the follow-up research interviews. However, 14 out of 150 assigned clients refused the supported housing condition. An understandably much greater 189 of 285 refused case-management services (generallyby neglecting to make the required Žrst telephone call). These rates of refusal are at worst typical (Blumberg, Shipley, & Shandler, 1973; Bahr & Caplow, 1974; Colson, 1990; Grigsby, Baumann, Gregorich, & Roberts-Gray, 1990; Stark, 1992; Abel & Cummings, 1993; Stahler et al., 1993), but they can still spur estimation bias.
The investigators decided against attempting to limit refusal by convincing clients to reject the experiment before assignment, that is, by asking them to withdraw if they might not be willing to accept an unfavourable treatment condition. It was felt that it might not be possible to convince the clients to withdraw, in that homeless individuals have strong incentives to remain in the experiment long enough to determine if they will obtain housing. There was also concern that a restrictive recruitment policy might give rise to a biased sample that did not represent the original population.7 Moreover, the policy defeats the purpose of aiming an intervention at the broad group of underserved homeless adults. It was decided instead to use sample selection equations to correct for selective refusal. It was also decided to collect follow-up data on clients who refused assignment. These data may be used to employ the intent-to-treat analysis strategy.
3.2. Statistical methods: First comparisons
The Žrst comparisons are of the estimates that arise under sample selection corrections and two conventional analysis strategies when similar equations are used.
Regression type and dependent variables
All of the outcome equations assess the impacts of the experimental treatments on drinking, drug use and homelessness ‘outcomes’, using as the measures of outcomes
7While it is possible to argue that selection issues also arise from the failure of some clients to complete a follow-up interview, we focus on selective entrance into the sample because it is generally a central concern. Further, it was a crucial problem in this experiment, while there is evidence that selective follow-up did not give rise to appreciable bias. Because many locating techniques were used, 70% of all sample members were located for the second follow-up interview. Locating techniques included searches of voting and other records, jail searches, trips to shelters, offers of Ž nancial incentivesfor calling in, follow-up calls to contacts provided by clients, and client payments for each interview. A bivariate probit analysis completed for this paper Ž nds little relation between selectivity out of the interventionsand the failure to be located for the relevant follow-up interview, suggesting that this selection problem can be treated as random. As one statistician suggests, estimation is only possible for virtually any data set (and analysis strategy) if some phenomena are considered random or of little consequence (Maddala, 1983).