
- •Stellingen
- •Propositions
- •List of Figures
- •List of Tables
- •1 Introduction
- •Introduction
- •Affect, emotion, and related constructs
- •Affective Computing: A concise overview
- •The closed loop model
- •Three disciplines
- •Human-Computer Interaction (HCI)
- •Health Informatics
- •Three disciplines, one family
- •Outline
- •2 A review of Affective Computing
- •Introduction
- •Vision
- •Speech
- •Biosignals
- •A review
- •Time for a change
- •3 Statistical moments as signal features
- •Introduction
- •Emotion
- •Measures of affect
- •Affective wearables
- •Experiment
- •Participants
- •Equipment and materials
- •Procedure
- •Data reduction
- •Results
- •Discussion
- •Comparison with the literature
- •Use in products
- •4 Time windows and event-related responses
- •Introduction
- •Data reduction
- •Results
- •Mapping events on signals
- •Discussion and conclusion
- •Interpreting the signals measured
- •Looking back and forth
- •5 Emotion models, environment, personality, and demographics
- •Introduction
- •Emotions
- •Modeling emotion
- •Ubiquitous signals of emotion
- •Method
- •Participants
- •International Affective Picture System (IAPS)
- •Digital Rating System (DRS)
- •Signal processing
- •Signal selection
- •Speech signal
- •Heart rate variability (HRV) extraction
- •Normalization
- •Results
- •Considerations with the analysis
- •The (dimensional) valence-arousal (VA) model
- •The six basic emotions
- •The valence-arousal (VA) model versus basic emotions
- •Discussion
- •Conclusion
- •6 Static versus dynamic stimuli
- •Introduction
- •Emotion
- •Method
- •Preparation for analysis
- •Results
- •Considerations with the analysis
- •The (dimensional) valence-arousal (VA) model
- •The six basic emotions
- •The valence-arousal (VA) model versus basic emotions
- •Static versus dynamic stimuli
- •Conclusion
- •IV. Towards affective computing
- •Introduction
- •Data set
- •Procedure
- •Preprocessing
- •Normalization
- •Baseline matrix
- •Feature selection
- •k-Nearest Neighbors (k-NN)
- •Support vector machines (SVM)
- •Multi-Layer Perceptron (MLP) neural network
- •Discussion
- •Conclusions
- •8 Two clinical case studies on bimodal health-related stress assessment
- •Introduction
- •Post-Traumatic Stress Disorder (PTSD)
- •Storytelling and reliving the past
- •Emotion detection by means of speech signal analysis
- •The Subjective Unit of Distress (SUD)
- •Design and procedure
- •Features extracted from the speech signal
- •Results
- •Results of the Stress-Provoking Story (SPS) sessions
- •Results of the Re-Living (RL) sessions
- •Overview of the features
- •Discussion
- •Stress-Provoking Stories (SPS) study
- •Re-Living (RL) study
- •Stress-Provoking Stories (SPS) versus Re-Living (RL)
- •Conclusions
- •9 Cross-validation of bimodal health-related stress assessment
- •Introduction
- •Speech signal processing
- •Outlier removal
- •Parameter selection
- •Dimensionality Reduction
- •k-Nearest Neighbors (k-NN)
- •Support vector machines (SVM)
- •Multi-Layer Perceptron (MLP) neural network
- •Results
- •Cross-validation
- •Assessment of the experimental design
- •Discussion
- •Conclusion
- •10 Guidelines for ASP
- •Introduction
- •Signal processing guidelines
- •Physical sensing characteristics
- •Temporal construction
- •Normalization
- •Context
- •Pattern recognition guidelines
- •Validation
- •Triangulation
- •Conclusion
- •11 Discussion
- •Introduction
- •Hot topics: On the value of this monograph
- •Applications: Here and now!
- •TV experience
- •Knowledge representations
- •Computer-Aided Diagnosis (CAD)
- •Visions of the future
- •Robot nannies
- •Digital Human Model
- •Conclusion
- •Bibliography
- •Summary
- •Samenvatting
- •Dankwoord
- •Curriculum Vitae
- •Publications and Patents: A selection
- •Publications
- •Patents
- •SIKS Dissertation Series

6.3 Method
an extended VA model incorporates, instead of one bipolar valence dimension, two unipolar valence dimensions: one for positive and one for negative valence. Hence, the extended valence-arousal model incorporates three dimensions, instead of two. This approach was also adopted for the current study.
6.3 Method
This section is compressed similarly to the Method section in Chapter 5. This will facilitate the comparison between both studies, which will have the focus of this chapter. Only those elements that deviate from the work presented in Chapter 5 will be reported here.
The same 40 volunteers participated in this study as did in the study presented in Chapter 5 and they were treated identically. The speech and ECG signals were recorded with the same devices and processed with the same software as has been reported in Chapter 5. The results of the revised, short scale of the Eysenck Personality Questionnaire (EPQRSS) [187] completed by the participants in Chapter 5 were also included in the analyses of the current study. For details, see the Methods section of Chapter 5
Instead of looking at IAPS pictures [374, 452], as was the case in the study presented in Chapter 5, dynamic stimuli were chosen [235, 237, 570, 700, 701]. To elicit an emotional response, the participants watched a selection of the six movie scenes that were introduced in Chapters 3 and 4. The movie scenes were presented on the same screen as the IAPS images of Chapter 5 were. Each of the six movie scenes that were shown had a duration of 3 minutes and 18 seconds. After each scene, the participants had 30 seconds to describe the most emotional part of the scene, followed by a rest period of 60 seconds. During these 90 seconds (speaking and resting), a gray screen was shown. The experiment started and finished, displaying a gray screen during 90 seconds.
6.4 Preparation for analysis
The speech signal of two participants was not recorded due to technical problems. The speech signals of two other participants were too noisy. The speech signals of these four participants were excluded from further analyses. With nine participants, either a significant amount of noise was present in their ECG or the signal was completely absent. The ECG signals of these participants were omitted from further processing. In total, 13 corruptions of signals were detected in the signals of 11 participants. So, the recordings of 2 participants suffered from 2 types of noise. Through interpolation, corrections could have been made for the absence of this data. However, this would have decreased the reliability of the analyses done. Therefore, we chose to omit all data for participants for whom prob-
103
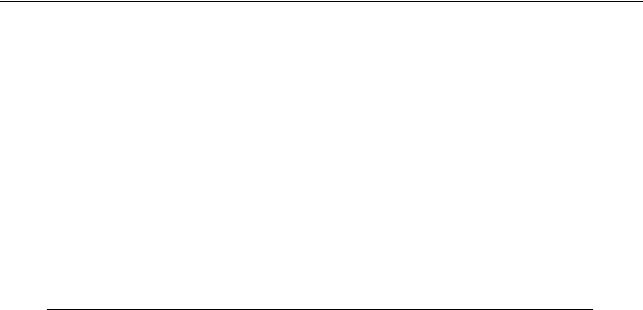
6 Static versus dynamic stimuli
Table 6.1: The six film scenes with the average ratings given by the participants on the positive valence, negative valence, and arousal Likert scales. From the positive and negative valence ratings, three valence categories can be derived: neutral, positive, and negative. Using the scores on arousal, two arousal categories can be determined: low and high
film scene |
|
valence |
|
|
arousal |
|
positive |
negative |
category |
score |
category |
Color bars |
0.13 |
2.51 |
neutral |
0.49 |
low |
Final Destina- |
2.59 |
4.38 |
neutral |
6.54 |
high |
tion |
|
|
|
|
|
The bear |
5.79 |
0.74 |
positive |
3.49 |
low |
Tarzan |
7.31 |
0.26 |
positive |
4.77 |
high |
Pink flamingos |
0.49 |
7.18 |
negative |
6.00 |
low‡ |
Cry freedom |
0.56 |
7.90 |
negative |
7.69 |
high |
Average |
2.81 |
3.83 |
|
4.83 |
|
‡ This score is higher than average. Nevertheless, it has been categorized as low. This was done for two reasons: 1) The experienced arousal is low relative to the other film scene with which a negative valence was experienced and 2) This categorization facilitated a balanced design, which enabled the preferred statistical analyses.
lems were encountered with the recordings. This resulted in data from 29 participants that could be analyzed.
Of each of the 29 participants, the sound recorded during the study lasted approximately 25 minutes; however, only the parts in which the participants spoke were of interest. Those parts in which the participants did not speak were automatically omitted from the speech signal processing pipeline.
To assess the emotions experienced by the participants, again the Digital Rating System (DRS) was used, as was introduced in Chapter 5. As in the study presented in Chapter 5, the DRS included three scales: positive valence, negative valence, and arousal, see also Table 6.1. For each film scene, the average ratings were calculated on each of the three scales over all participants. This resulted in a classification of the film scenes into two categories (i.e., high and low) for each of the three scales: positive, negative, and arousal. From these classifications, we derived three categories for valence: positive, negative and neutral. The category neutral denotes neither a positive valence nor a negative valence. In addition, two categories for arousal were derived: high arousal and low arousal. Together, these two categorized dimensions of the VA model depicted six emotion classes. Each of the 6 basic emotions was represented in this research by one film fragment.
104