
- •Stellingen
- •Propositions
- •List of Figures
- •List of Tables
- •1 Introduction
- •Introduction
- •Affect, emotion, and related constructs
- •Affective Computing: A concise overview
- •The closed loop model
- •Three disciplines
- •Human-Computer Interaction (HCI)
- •Health Informatics
- •Three disciplines, one family
- •Outline
- •2 A review of Affective Computing
- •Introduction
- •Vision
- •Speech
- •Biosignals
- •A review
- •Time for a change
- •3 Statistical moments as signal features
- •Introduction
- •Emotion
- •Measures of affect
- •Affective wearables
- •Experiment
- •Participants
- •Equipment and materials
- •Procedure
- •Data reduction
- •Results
- •Discussion
- •Comparison with the literature
- •Use in products
- •4 Time windows and event-related responses
- •Introduction
- •Data reduction
- •Results
- •Mapping events on signals
- •Discussion and conclusion
- •Interpreting the signals measured
- •Looking back and forth
- •5 Emotion models, environment, personality, and demographics
- •Introduction
- •Emotions
- •Modeling emotion
- •Ubiquitous signals of emotion
- •Method
- •Participants
- •International Affective Picture System (IAPS)
- •Digital Rating System (DRS)
- •Signal processing
- •Signal selection
- •Speech signal
- •Heart rate variability (HRV) extraction
- •Normalization
- •Results
- •Considerations with the analysis
- •The (dimensional) valence-arousal (VA) model
- •The six basic emotions
- •The valence-arousal (VA) model versus basic emotions
- •Discussion
- •Conclusion
- •6 Static versus dynamic stimuli
- •Introduction
- •Emotion
- •Method
- •Preparation for analysis
- •Results
- •Considerations with the analysis
- •The (dimensional) valence-arousal (VA) model
- •The six basic emotions
- •The valence-arousal (VA) model versus basic emotions
- •Static versus dynamic stimuli
- •Conclusion
- •IV. Towards affective computing
- •Introduction
- •Data set
- •Procedure
- •Preprocessing
- •Normalization
- •Baseline matrix
- •Feature selection
- •k-Nearest Neighbors (k-NN)
- •Support vector machines (SVM)
- •Multi-Layer Perceptron (MLP) neural network
- •Discussion
- •Conclusions
- •8 Two clinical case studies on bimodal health-related stress assessment
- •Introduction
- •Post-Traumatic Stress Disorder (PTSD)
- •Storytelling and reliving the past
- •Emotion detection by means of speech signal analysis
- •The Subjective Unit of Distress (SUD)
- •Design and procedure
- •Features extracted from the speech signal
- •Results
- •Results of the Stress-Provoking Story (SPS) sessions
- •Results of the Re-Living (RL) sessions
- •Overview of the features
- •Discussion
- •Stress-Provoking Stories (SPS) study
- •Re-Living (RL) study
- •Stress-Provoking Stories (SPS) versus Re-Living (RL)
- •Conclusions
- •9 Cross-validation of bimodal health-related stress assessment
- •Introduction
- •Speech signal processing
- •Outlier removal
- •Parameter selection
- •Dimensionality Reduction
- •k-Nearest Neighbors (k-NN)
- •Support vector machines (SVM)
- •Multi-Layer Perceptron (MLP) neural network
- •Results
- •Cross-validation
- •Assessment of the experimental design
- •Discussion
- •Conclusion
- •10 Guidelines for ASP
- •Introduction
- •Signal processing guidelines
- •Physical sensing characteristics
- •Temporal construction
- •Normalization
- •Context
- •Pattern recognition guidelines
- •Validation
- •Triangulation
- •Conclusion
- •11 Discussion
- •Introduction
- •Hot topics: On the value of this monograph
- •Applications: Here and now!
- •TV experience
- •Knowledge representations
- •Computer-Aided Diagnosis (CAD)
- •Visions of the future
- •Robot nannies
- •Digital Human Model
- •Conclusion
- •Bibliography
- •Summary
- •Samenvatting
- •Dankwoord
- •Curriculum Vitae
- •Publications and Patents: A selection
- •Publications
- •Patents
- •SIKS Dissertation Series

3
Statistical moments as signal features
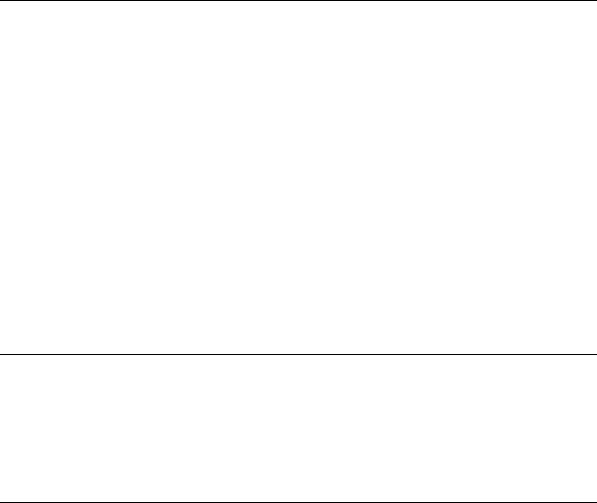
Abstract
To improve Human-Computer Interaction (HCI), computers need to be able to recognize and respond properly to their user’s emotional state. This is a fundamental application of affective computing, which relates to, arises from, or deliberately influences emotion. As a first step to a system that recognize emotions of individual users, this research focused on how emotional experiences are expressed in six parameters (i.e., mean, absolute deviation, standard deviation, variance, skewness, and kurtosis) of not baseline-corrected physiological measurements of the ElectroDermal Activity (EDA) and of three ElectroMyoGraphy (EMG) signals: frontalis (EMG1), corrugator supercilii (EMG2), and zygomaticus major (EMG3). Twenty-four participants were asked to watch film scenes of 120 seconds, which they then rated. These ratings enabled us to distinguish four classes of emotions: negative, positive, mixed, and neutral. The skewness and kurtosis of the EDA, the skewness of the EMG2, and four parameters of EMG3, discriminate between the four emotion classes and explained 36.8% − 61.8% of the variance between the emotion four classes. This, despite the coarse time windows that were used. Moreover, rapid processing of the signals proved to be possible. This enables tailored HCI facilitated by an emotional awareness of systems.
This chapter is an adapted and extended version of:
Broek, E.L. van den, Schut, M.H., Westerink, J.H.D.M., Herk, J. van, and Tuinenbreijer, K. (2006). Computing emotion awareness through facial electromyography. Lecture Notes in Computer Science (Human-Computer Interaction), 3979, 51–62.
which is also published as:
Westerink, J.H.D.M., Broek, E.L. van den, Schut, M.H., Herk, J. van, and Tuinenbreijer, K. (2008). Computing emotion awareness through galvanic skin response and facial electromyography. In J.H.D.M. Westerink, M. Ouwerkerk, T. Overbeek, W.F. Pasveer, and B. de Ruyter (Eds.), Probing Experience: From Academic Research to Commercial Propositions (Part II: Probing in order to feed back), Chapter 14, p. 137–150. Series: Philips Research Book Series , Vol. 8. Dordrecht, The Netherlands: Springer Science + Business Media B.V.
and is filed as:
Westerink, J.H.D.M., Broek, E.L. van den, Schut, M.H., Tuinenbreijer, K. (2007). Higher order GSR-measurement interpretation indicating emotions. International Patent Application No. PCT/IB2008/050477 (PH007322), filed on February 11.

3.1 Introduction
3.1 Introduction
Computers are experienced by their users as cold hearted (i.e., “marked by lack of sympathy, interest, or sensitivity” [448]). However, ’during the past decade rapid advances in spoken language technology, natural language processing, dialog modeling, multi-modal interfaces, animated character design, and mobile applications all have stimulated interest in a new class of conversational interfaces’ [504]. The progress made in this broad range of research and technology enables the rapid computation and modeling of empathy for humancomputer interaction (HCI) purposes. The latter is of importance since conversation is, apart from being an information exchange, a social activity, which is inherently enforcing [504]. Futurists envision embodied, social artificial systems that interact in a natural manner with us. Such systems need to sense its user’s emotional state.
Empathic artificial systems can, for example, prevent user frustration in HCI. Users frequently feel frustrated by various causes; for example, error messages, timed out/dropped/ refused connections, freezes, long download time, and missing/ hard-to-find features [94]. Picard [518] posed the prevention of user frustration as one of the main goals in HCI. When prevention is not sufficient, online detection and reduction of frustration is needed. Biosignals are useful in detecting frustration [521]. According to Hone [286], an (embodied) affective agent, using techniques of active listening and emotion-awareness could reduce user frustration.
The current chapter discusses the emotions people can experience and their expression in and detection through ASP, in Section 3.2 and Section 3.3. Next, in Section 3.4, affective wearables are introduced in which the proposed apparatus for the measurement of the biosignals can be embedded. In Section 3.5, we present an experiment into the appropriateness of various statistical measures derived from biosignals, followed by a reduction of the data in Section 3.6. The experimental results are described in Section 3.7. The chapter ends with Section 3.8 in which the results are discussed, limitations are denoted, and future research is described.
3.2 Emotion
Despite the complexity of the concept of emotion, most researchers agree that emotions are acute affective states that exist for a relatively short period of time and are related to a particular event, object, or action [502, 521]. In relation with physiology, emotions are predominantly described as points in a two-dimensional space of affective valence and arousal, in which valence represents overall pleasantness of emotional experiences ranging from negative to positive, while arousal represents the intensity level of emotion, ranging from calm to excited [372, 647]. This allows us to tell the difference between 4 rough classes of emotions,
43

3 Statistical moments as signal features
when differentiated between both high and low valence and high and low arousal. Some researchers even differentiate between nine classes by including a neutral section on both the valence and arousal axes. However, in principle, any number of classes can be defined, where the valence and arousal axes are not necessarily divided with the same precision [61].
The valence-arousal model, however, does not account for mixed emotions: positive and negative at the same moment. In order to be able to cope with mixed emotions, Larsen et al. [380] and Konijn and Hoorn [357] suggest that valence should be unipolar instead of bipolar. When valence is rated on two scales, one for the intensity of positive affect and one for the intensity of negative affect, mixed emotions, in the sense of both positive and negative emotions, will show. As an extension to the valence-arousal model, a unipolar valence axis, with separated positive and negative axes, might allow for a better discrimination between different emotions.
In the current research, we only explored the valence axis. The reason is that the simplest differentiation of emotions is a differentiation between positive and negative emotions. In most cases of HCI, this is sufficient to improve the dialog between user and computer; for example, when a user has a negative emotion, the computer can adapt its dialog to that, depending on the context.
3.3 Measures of affect
The roots in research toward psychophysiological aspects of emotions lay in Darwin’s book ‘The expression of emotions in man and animals’, which he wrote in 1872. The overall assumption is that emotion arouses the autonomic nervous system (ANS), which alters the physiological state. This is expressed in various physiological measures, often stimulated through the ANS; for example, heart rate, blood pressure, respiration rate, ElectroDermal Activity (EDA), and muscle activity (see Table 1.1). The main advantage of using autonomic physiological measures is that autonomic variables are regulated by the ANS, which controls functions outside the individual’s conscious control [85]. In this research, we focused on how emotional experiences, rated to their positive and negative affect, are expressed in four biosignals:
•EDA (also termed GSR) [62], which is a measure of the conductivity of the skin: arousal of the ANS influences sweat glands to produce more sweat; consequently, skin conductivity increases. EDA was chosen because it is an autonomic variable; hence, it cannot be controlled by the user [136].
•Three EMG signals: frontalis, corrugator supercilii, and zygomaticus major [664]. EMG measures muscle activity of a certain muscle. These measures were chosen because a great deal of emotional expression is located in the face [380, 592, 664]. Facial EMG
44