
Теория информации / Gray R.M. Entropy and information theory. 1990., 284p
.pdf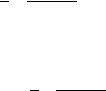
3.4. AMS SOURCES |
59 |
In order to evaluate ‡ we apply the ergodic decomposition of relative entropy rate (Corollary 2.4.2) and the ordinary ergodic decomposition to write
Z
Z
• inf
k
Z
„
dPˆ‡ = dPˆ inf Hmˆ jjm(k) (X)
k
„ |
|
„ |
|
(k) (X): |
dPˆH |
mˆ jjm |
(k) (X) = inf H |
mjjm |
|
|
k |
|
From Theorem 2.6.2, the right hand term is 0. If the integral of a nonnegative function is 0, the integrand must itself be 0 with probability one. Thus (3.25)
becomes
lim sup 1 ln mˆ(Xn) • 0; n!1 n m(Xn)
which with (3.23) completes the proof of the lemma. 2
We shall later see that the quantity
in(Xn; ˆ) = 1 ln mˆ(Xn) n m(Xn)
is the sample mutual information (in a generalized sense so that it applies to the usually non-discrete ˆ) and hence the lemma states that the normalized sample mutual information between the process outputs and the ergodic component function goes to 0 as the number of samples goes to inflnity.
The two previous lemmas immediately yield the following result.
Corollary 3.3.1: The conclusions of Theorem 3.1.1 hold for sources that are stationary.
3.4AMS Sources
The principal idea required to extend the entropy theorem from stationary sources to AMS sources is contained in Lemma 3.4.2. It shows that an AMS source inherits sample entropy properties from an asymptotically dominating stationary source (just as it inherits ordinary ergodic properties from such a source). The result is originally due to Gray and Kiefier [54], but the proof here is somewhat difierent. The tough part here is handling the fact that the sample average being considered depends on a speciflc measure. From Theorem 1.7.1, the stationary mean of an AMS source dominates the original source on tail events, that is, events in F1. We begin by showing that certain important events can be recast as tail events, that is, they can be determined by looking at only samples in the arbitrarily distant future. The following result is of this variety: It implies that sample entropy is unafiected by the starting time.
Lemma 3.4.1: Let fXng be a flnite alphabet source with distribution m. Recall that Xkn = (Xk; Xk+1; ¢ ¢ ¢ ; Xk+n¡1) and deflne the information density
i(Xk; Xkn¡k) = ln |
m(Xn) |
|
|
: |
|
|
||
|
m(Xk)m(Xkn¡k) |
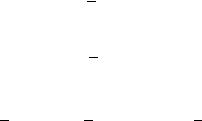
60 CHAPTER 3. THE ENTROPY ERGODIC THEOREM
Then
nlim |
1 |
i(Xk; Xkn¡k) = 0; m ¡ a:e:: |
n |
||
!1 |
|
|
Comment: The lemma states that with probability 1 the per-sample mutual information density between the flrst k samples and future samples goes to zero in the limit. Equivalently, limits of n¡1 ln m(Xn) will be the same as limits of n¡1 ln m(Xkn¡k) for any flnite k. Note that the result does not require even that the source be AMS. The lemma is a direct consequence of Lemma 2.7.1.
Proof: Deflne the distribution p = mXk £mXk ;Xk+1;¢¢¢, that is, a distribution for which all samples after the flrst k are independent of the flrst k samples. Thus, in particular, p(Xn) = m(Xk)m(Xkn). We will show that p >> m, in which case the lemma will follow from Lemma 2.7.1. Suppose that p(F ) = 0. If we denote Xk+ = Xk; Xk+1; ¢ ¢ ¢, then
X
0 = p(F ) = m(xk)mXk+ (Fxk );
xk
where Fxk is the section fx+k : (xk; x+k ) = x 2 F g. For the above relation to hold, we must have mXk+ (Fxk ) = 0 for all xk with m(xk) 6= 0. We also have, however, that
X
m(F ) = m(Xk = ak; Xk+ 2 Fak )
ak
X
=m(Xk = akjXk+ 2 Fak )m(Xk+ 2 Fak ):
ak
But this sum must be 0 since the rightmost terms are 0 for all ak for which
m(Xk = ak) is not 0. (Observe that we must have m(Xk = akjXk+ 2 Fak ) = 0 if m(Xk+ 2 Fak ) 6= 0 since otherwise m(Xk = ak) ‚ m(Xk = ak; Xk+ 2 Fak ) > 0, yielding a contradiction.) Thus p >> m and the lemma is proved. 2
For later use we note that we have shown that a joint distribution is dominated by a product of its marginals if one of the marginal distributions is discrete.
Lemma 3.4.2: Suppose that fXng is an AMS source with distribution m and suppose that m„ is a stationary source that asymptotically dominates m (e.g., m„ is the stationary mean). If there is an invariant function h such that
lim ¡ 1 ln m„ (Xn) = h; m„ ¡ a:e:;
n!1 n
then also,
lim ¡ 1 ln m(Xn) = h; m ¡ a:e:
n!1 n
Proof: For any k we can write using the chain rule for densities
¡n1 ln m(Xn) + n1 ln m(Xkn¡k) = ¡n1 ln m(XkjXkn¡k)

3.4. AMS SOURCES |
61 |
1 |
1 |
= ¡n i(Xk; Xkn¡k) ¡ n ln m(Xk):
From the previous lemma and from the fact that Hm(Xk) = ¡Em ln m(Xk) is flnite, the right hand terms converge to 0 as n ! 1 and hence for any k
|
|
|
|
nlim |
1 |
ln m(XkjXkn¡k) |
|
|||||||||
|
|
|
|
¡ |
|
|
|
|||||||||
n |
|
|||||||||||||||
!1 |
|
|
|
|
|
|
|
|
|
|
||||||
1 |
|
|
|
1 |
ln m(Xkn¡k)) = 0; m ¡ a:e:: |
|
||||||||||
= nlim (¡ |
|
ln m(Xn) + |
|
(3.26) |
||||||||||||
n |
n |
|||||||||||||||
!1 |
|
|
|
|
|
|
|
|
|
|
|
|
|
|
|
|
This implies that there is a subsequence k(n) ! 1 such that |
|
|||||||||||||||
1 |
ln m(X |
k(n) |
n¡k(n) |
) |
|
|||||||||||
|
|
|
|
¡ |
|
|
||||||||||
|
|
|
|
|
|
jXk(n) |
|
|||||||||
|
|
|
|
n |
|
|
||||||||||
1 |
|
|
|
|
|
1 |
ln m(Xkn(¡nk) (n)) ! 0; m ¡ a:e:: |
|
||||||||
= ¡ |
|
ln m(Xn) ¡ |
|
(3.27) |
||||||||||||
n |
n |
To see this, observe that (3.26) ensures that for each k there is an N (k) large
enough so that N (k) > N (k ¡ 1) and |
|
|
|
|
|
||
1 |
ln m(Xk |
XN(k)¡k) > 2¡k) |
|
2¡k: |
(3.28) |
||
m( |
|
• |
|||||
|
|||||||
j ¡ N (k) |
j |
k |
j |
|
|
Applying the Borel-Cantelli lemma implies that for any †,
m(j ¡ 1=N (k) ln m(XkjXkN(k)¡k)j > † i.o.) = 0:
Now let k(n) = k for N (k) • n < N (k + 1). Then
|
m(j ¡ 1=n ln m(X |
k(n) |
n¡k(n) |
)j > † i.o.) = 0 |
|
||||||
|
|
|
|
jXk(n) |
|
||||||
and therefore |
µ¡n |
|
|
|
|
|
¶ |
¡ |
|
||
n!1 |
n |
k(n) |
|
||||||||
lim |
|
1 |
ln m(Xn) + |
|
1 |
ln m(Xn¡k(n)) |
= 0; m |
|
a:e: |
||
|
|
|
|
as claimed in (3.27).
In a similar manner we can also choose the sequence so that
n!1 |
µ¡n ln m„ (X |
|
) + n ln m„ (Xk(n) )¶ |
= 0; m„ ¡ a:e:; |
||
lim |
1 |
|
n |
1 |
n¡k(n) |
|
|
|
|
|
|
that is, we can choose N (k) so that (3.4.3) simultaneously holds for both m and m„ . Invoking the entropy ergodic theorem for the stationary m„ (Corollary 3.3.1) we have therefore that
1 |
n¡k(n) |
„ |
|
|
|
nlim ¡ |
|
ln m„ (Xk(n) |
) = h; m„ |
¡ a:e:: |
(3.29) |
n |
|||||
!1 |
|
|
|
|
|
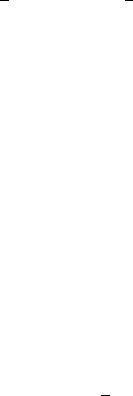
62 |
CHAPTER 3. THE ENTROPY ERGODIC THEOREM |
From Markov’s inequality (Lemma 4.4.3 of [50])
1 |
1 |
|
m(Xn) |
|
||
m„ (¡ |
|
ln m(Xkn) • ¡ |
|
ln m„ (Xkn) ¡ †) = m„ ( |
k |
‚ en†) |
n |
n |
m„ (Xn) |
||||
|
|
|
|
|
k |
|
|
e¡n†E |
|
m(Xn¡k) |
k |
X |
6 |
|
|
k |
||||
• |
m„ |
= e¡n† |
|
|
||
|
m„ (Xn¡k) |
xn¡k :m„ (xkn¡k )=0 |
||||
|
|
|
k |
m(xn¡k) |
m„ (xn¡k) |
|
e¡n†: |
|
k |
• |
|||
m„ (xkn¡k) |
||||
k |
|
Hence taking k = k(n) and again invoking the Borel-Cantelli lemma we have that
m„ (¡ 1 ln m(Xn¡k(n)) • ¡ 1 ln m„ (Xn¡k(n)) ¡ † i.o.) = 0
n k(n) n k(n)
or, equivalently, that
|
|
|
1 |
m(Xn¡k(n)) |
|
|
|
|
||
lim inf |
ln |
k(n) |
|
0; m„ |
|
a:e:: |
||||
¡n |
|
‚ |
¡ |
|||||||
n!1 |
m„ (Xn¡k(n)) |
|
|
|||||||
|
|
|
|
|
k(n) |
|
|
|
|
|
Therefore from (3.29) |
|
|
|
|
|
|
|
|
||
lim inf |
|
1 |
ln m(Xn¡k(n)) |
|
h; m„ |
|
a:e:: |
|||
¡n |
‚ |
¡ |
||||||||
n |
!1 |
|
k(n) |
|
|
|||||
|
|
|
|
|
|
|
|
|
T
(3.30)
(3.31)
The above event is in the tail ¾-fleld F1 = n ¾(Xn; Xn+1; ¢ ¢ ¢) since it can be determined from Xk(n); ¢ ¢ ¢ for arbitrarily large n and since h is invariant. Since m„ dominates m on the tail ¾-fleld (Theorem 1.7.2), we have also
lim inf |
|
1 |
ln m(Xn¡k(n)) |
|
h; m |
|
a:e: |
||||||
¡n |
‚ |
¡ |
|||||||||||
n |
!1 |
|
|
k(n) |
|
|
|
||||||
|
|
|
|
|
|
|
|
|
|
|
|||
and hence by (3.4.2) |
|
|
|
|
|
|
|
|
|
|
|
|
|
|
lim inf |
|
|
1 |
ln m(Xn |
) ‚ h; m ¡ a:e: |
|||||||
|
¡n |
||||||||||||
|
n |
!1 |
|
||||||||||
|
|
|
|
|
|
|
|
|
|
|
which proves half of the lemma.
Since
m„ ( lim ¡ 1 ln m„ (Xn) 6= h) = 0
n!1 n
and since m„ asymptotically dominates m (Theorem 1.7.1), given † > 0 there is
a k such that |
|
||
1 |
ln m„ (Xkn) = h) ‚ 1 |
|
|
m(nlim ¡ |
|
¡ †: |
|
n |
|||
!1 |
|
|
|
Again applying Markov’s inequality and the Borel-Cantelli lemma as in the development of (3.29) we have that
lim inf |
1 |
ln |
m„ (Xn) |
0; m |
|
a:e; |
|||
|
|
k |
|
|
|||||
|
|
m(Xn) ‚ |
¡ |
||||||
n |
!1 |
¡n |
|
|
|||||
|
|
|
|
k |
|
|
|

3.5. THE ASYMPTOTIC EQUIPARTITION PROPERTY |
63 |
|||
which implies that |
|
|
||
1 |
ln m(Xkn) • h) ‚ 1 |
|
|
|
m(lim sup ¡ |
|
¡ † |
|
|
n |
|
|||
n!1 |
|
|
and hence also that
m(lim sup ¡ 1 ln m(Xn) • h) ‚ 1 ¡ †:
n!1 n
Since † can be made arbitrarily small, this proves that m-a.e.
lim sup ¡n¡1 ln m(Xn) • h;
which completes the proof of the lemma. 2
The lemma combined with Corollary 3.3.1 completes the proof of Theorem 3.1.1. 2
3.5The Asymptotic Equipartition Property
Since convergence almost everywhere implies convergence in probability, Theorem 3.1.2 has the following implication: Suppose that fXng is an AMS ergodic
„
source with entropy rate H. Given † > 0 there is an N such that for all n > N the set
f n j ¡1 ¡ „ j ‚ g
Gn = x : n hn(x) H †
¡ ( „ + ) ¡ ( „ ¡ )
= fxn : e n H † • m(xn) • e n H † g
has probability greater then 1 ¡ †. Furthermore, as in the proof of the theorem,
there can be no more than e ( „ + ) n-tuples in G . Thus there are two sets of n-
n H †
n
„
tuples: a \good" set of approximately enH n-tuples having approximately equal
probability of e¡ „ and the complement of this set which has small total prob-
nH
ability. The set of good sequences are often referred to as \typical sequences" in the information theory literature and in this form the theorem is called the asymptotic equipartition property or the AEP.
As a flrst information theoretic application of an ergodic theorem, we consider a simple coding scheme called an \almost noiseless source code." As we often do, we consider logarithms to the base 2 when considering speciflc coding applications. Suppose that a random process fXng has a flnite alphabet A with
jj jj „ „ jj jj
cardinality A and entropy rate H. Suppose that H < log A , e.g., A might have 16 symbols, but the entropy rate is slightly less than 2 bits per symbol rather than log 16 = 4. Larger alphabets cost money in either storage or communication applications. For example, to communicate a source with a 16 letter alphabet sending one letter per second without using any coding and using a binary communication system we would need to send 4 binary symbols (or four bits) for each source letter and hence 4 bits per second would be required. If the alphabet only had 4 letters, we would need to send only 2 bits per second. The question is the following: Since our source has an alphabet of size 16 but
64 CHAPTER 3. THE ENTROPY ERGODIC THEOREM
an entropy rate of less than 2, can we code the original source into a new source with an alphabet of only 4 letters so as to communicate the source at the smaller rate and yet have the receiver be able to recover the original source? The AEP suggests a technique for accomplishing this provided we are willing to tolerate occasional errors.
We construct a code of the original source by flrst picking a small † and
„
a – small enough so that H + – < 2. Choose a large enough n so that the AEP holds giving a set Gn of good sequences as above with probability greater
|
„ |
2n |
using |
|
|
n(H+–) |
|||
than 1 ¡ †. Index this collection of fewer than 2 |
|
< 2 sequences |
||
|
n |
|
||
binary 2n-tuples. The source Xk is parsed into blocks of length n as Xkn |
= |
(Xkn; Xkn+1; ¢ ¢ ¢ ; X(k+1)n) and each block is encoded into a binary 2n-tuple as follows: If the source n-tuple is in Gn, the codeword is its binary 2n-tuple index.
Select one of the unused binary 2n-tuples as the error index and whenever an n-tuple is not in Gn, the error index is the codeword. The receiver or decoder than uses the received index and decodes it as the appropriate n-tuple in Gn. If the error index is received, the decoder can declare an arbitrary source sequence or just declare an error. With probability at least 1 ¡ † a source n-tuple at a particular time will be in Gn and hence it will be correctly decoded. We can make this probability as small as desired by taking n large enough, but we cannot in general make it 0.
The above simple scheme is an example of a block coding scheme. If considered as a mapping from sequences into sequences, the map is not stationary, but it is block stationary in the sense that shifting an input block by n results in a corresponding block shift of the encoded sequence by 2n binary symbols.
Chapter 4
Information Rates I
4.1Introduction
Before proceeding to generalizations of the various measures of information, entropy, and divergence to nondiscrete alphabets, we consider several properties of information and entropy rates of flnite alphabet processes. We show that codes that produce similar outputs with high probability yield similar rates and that entropy and information rate, like ordinary entropy and information, are reduced by coding. The discussion introduces a basic tool of ergodic theory{ the partition distance{and develops several versions of an early and fundamental result from information theory{Fano’s inequality. We obtain an ergodic theorem for information densities of flnite alphabet processes as a simple application of the general Shannon-McMillan-Breiman theorem coupled with some deflnitions. In Chapter 6 these results easily provide L1 ergodic theorems for information densities for more general processes.
4.2Stationary Codes and Approximation
We consider the behavior of entropy when codes or measurements are taken on the underlying random variables. We have seen that entropy is a continuous function with respect to the underlying measure. We now wish to flx the measure and show that entropy is a continuous function with respect to the underlying measurement.
Say we have two flnite alphabet measurements f and g on a common probability space having a common alphabet A. Suppose that Q and R are the corresponding partitions. A common metric or distance measure on partitions in ergodic theory is
1 |
X |
|
|
jQ ¡ Rj = |
|
P (Qi¢Ri); |
(4.1) |
2 |
|||
|
|
i |
|
which in terms of the measurements (assuming they have distinct values on distinct atoms) is just Pr(f 6= g). If we consider f and g as two codes on a common
65
66 |
CHAPTER 4. INFORMATION RATES I |
space, random variable, or random process (that is, flnite alphabet mappings), then the partition distance can also be considered as a form of distance between the codes. The following lemma shows that entropy of partitions or measurements is continuous with respect to this distance. The result is originally due to Fano and is called Fano’s inequality [37].
Lemma 4.2.1: Given two flnite alphabet measurements f and g on a common probability space (›; B; P ) having a common alphabet A or, equivalently, the given corresponding partitions Q = ff ¡1(a); a 2 Ag and R = fg¡1(a); a 2 Ag, deflne the error probability Pe = jQ ¡ Rj = Pr(f 6= g). Then
H(f jg) • h2(Pe) + Pe ln(jjAjj ¡ 1)
and
jH(f ) ¡ H(g)j • h2(Pe) + Pe ln(M ¡ 1)
and hence entropy is continuous with respect to partition distance for a flxed measure.
Proof: Let M = jjAjj and deflne a measurement
r : A £ A ! f0; 1; ¢ ¢ ¢ ; M ¡ 1g
by r(a; b) = 0 if a = b and r(a; b) = i if a 6= b and a is the ith letter in the alphabet Ab = A ¡ b. If we know g and we know r(f; g), then clearly we know f since either f = g (if r(f; g) is 0) or, if not, it is equal to the r(f; g)th letter in the alphabet A with g removed. Since f can be considered a function of g and r(f; g),
H(f jg; r(f; g)) = 0
and hence
H(f; g; r(f; g)) = H(f jg; r(f; g)) + H(g; r(f; g)) = H(g; r(f; g)):
Similarly
H(f; g; r(f; g)) = H(f; g):
From Lemma 2.3.2
H(f; g) = H(g; r(f; g)) • H(g) + H(r(f; g))
or
H(f; g) ¡ H(g) = H(f jg) • H(r(f; g))
M¡1
X
= ¡P (r = 0) ln P (r = 0) ¡ P (r = i) ln P (r = i):
i=1
P
Since P (r = 0) = 1 ¡ Pe and since i6=0 P (r = i) = Pe, this becomes
M¡1 |
P (r = i) P (r = i) |
|
||
X |
|
|
|
|
H(f jg) • ¡(1 ¡ Pe) ln(1 ¡ Pe) ¡ Pe |
Pe |
ln |
Pe |
¡ Pe ln Pe |
i=1 |
|
|
|
|
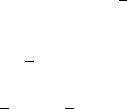
4.2. STATIONARY CODES AND APPROXIMATION |
67 |
• h2(Pe) + Pe ln(M ¡ 1)
since the entropy of a random variable with an alphabet of size M ¡ 1 is no greater than ln(M ¡1). This proves the flrst inequality. Since H(f ) • H(f; g) = H(f jg) + H(g), this implies
H(f ) ¡ H(g) • h2(Pe) + Pe ln(M ¡ 1):
Interchanging the roles of f and g completes the proof. 2
The lemma can be used to show that related information measures such as mutual information and conditional mutual information are also continuous with respect to the partition metric. The following corollary provides useful extensions. Similar extensions may be found in Csisz¶ar and K˜orner [26].
Corollary 4.2.1: Given two sequences of measurements ffng and fgng with flnite alphabet A on a common probability space, deflne
n¡1
Pe(n) = n1 X Pr(fi 6= gi):
i=0
Then
n1 H(f njgn) • Pe(n) ln(jjAjj ¡ 1) + h2(Pe(n))
and
jn1 H(f n) ¡ n1 H(gn)j • Pe(n) ln(jjAjj ¡ 1) + h2(Pe(n)):
If ffn; gng are also AMS and hence the limit
|
|
|
|
„ |
(n) |
|
|
|
|
|
|
|||
|
|
|
|
Pe = lim Pe |
|
|
|
|
|
|
||||
|
|
|
|
|
|
n!1 |
|
|
|
|
|
|
||
exists, then if we deflne |
|
|
|
|
|
|
|
|
|
|
|
|
|
|
„ |
|
1 |
H(f |
n |
n |
1 |
|
(H(f |
n |
n |
|
n |
|
|
H(f jg) = nlim |
|
|
|
jg |
) = nlim |
|
|
|
; g |
) ¡ |
H(g |
)); |
||
|
n |
|
n |
|
||||||||||
!1 |
|
|
|
|
!1 |
|
|
|
|
|
|
|
|
|
where the limits exist since the processes are AMS, then |
|
|
|
|||||||||||
„ |
|
|
|
|
„ |
|
|
|
|
|
„ |
|
|
|
H(f jg) • Pe ln(jjAjj ¡ 1) + h2(Pe) |
|
|
|
|||||||||||
„ |
|
|
„ |
|
|
„ |
|
|
|
„ |
|
|
||
jH(f ) |
¡ H(g)j • Pe ln(jjAjj ¡ 1) + h2(Pe): |
|
|
Proof: From the chain rule for entropy (Corollary 2.5.1), Lemma 2.5.2, and Lemma 4.2.1
|
n¡1 |
n¡1 |
|
H(f njgn) = H(fijf i; gn) • |
H(fijgi) |
|
i=0 |
i=0 |
|
X |
X |
n¡1 |
n¡1 |
|
XX
•H(fijgi) • (Pr(fi 6= gi) ln(jjAjj ¡ 1) + h2(Pr(fi 6= gi)))
i=0 |
i=0 |
68 CHAPTER 4. INFORMATION RATES I
from the previous lemma. Dividing by n yields the flrst inequality which implies the second as in the proof of the previous lemma. If the processes are jointly AMS, then the limits exist and the entropy rate results follows from the continuity of h2 by taking the limit. 2
The per-symbol probability of error Pe(n) has an alternative form. Recall that the (average) Hamming distance between two vectors is the number of positions in which they difier, i.e.,
d(1)H (x0; y0) = 1 ¡ –x0;y0 ;
where –a;b is the Kronecker delta function (0 if a = b and 1 otherwise), and
|
|
|
n¡1 |
|
dH(n)(xn; yn) = |
i=0 |
dH(1)(xi; yi): |
||
|
|
|
X |
|
We have then that |
|
|
||
1 |
|
(n) |
|
|
Pe(n) = E µ |
|
dH |
(f n; gn)¶ ; |
|
n |
the normalized average Hamming distance.
The next lemma and corollary provide a useful tool for approximating complicated codes by simpler ones.
Lemma 4.2.2: Given a probability space (›; B; P ) suppose that F is a generating fleld: B = ¾(F). Suppose that B-measurable Q is a partition of › and † > 0. Then there is a partition Q0 with atoms in F such that jQ¡Q0j • †.
Proof: Let jjAjj = K. From Theorem 1.2.1 given ° > 0 we can flnd sets Ri 2 F such that P (Qi¢Ri) • ° for i = 1; 2; ¢ ¢ ¢ ; K ¡ 1. The remainder of the proof consists of set theoretic manipulations showing that we can construct the desired partition from the Ri by removing overlapping pieces. The algebra is given for completeness, but it can be skipped. Form a partition from the sets as
|
|
|
i¡1 |
|
|
|
|
|
Q0i = Ri ¡ |
j[ |
|
|
|
|
|
|
Rj ; i = 1; 2; ¢ ¢ ¢ ; K ¡ 1 |
|
|||||
|
|
|
=1 |
|
|
|
|
|
|
|
K¡1 |
|
|
|
|
|
|
|
i[ |
|
|
|
|
|
|
Q0K = ( |
Q0i)c: |
|
|
|
|
|
|
|
=1 |
|
|
|
|
For i < K |
P (Qi¢Q0i) = P (Qi [ Q0i) ¡ P (Qi \ Q0i) |
|
|||||
|
|
||||||
|
• P (Qi |
[ |
|
\ |
[ |
|
|
|
Ri) ¡ P (Qi |
(Ri ¡ |
Rj )): |
(4.2) |
|||
|
|
|
|
|
j<i |
|
|
The rightmost term can be written as |
|
[ |
\ |
\ |
|||
\ |
[ |
|
\ |
||||
P (Qi |
(Ri ¡ Rj )) = P ((Qi |
Ri) ¡ ( |
Qi |
Ri |
Rj )) |
||
|
j<i |
|
|
|
j<i |
|
|