
Encyclopedia of Sociology Vol
.2.pdf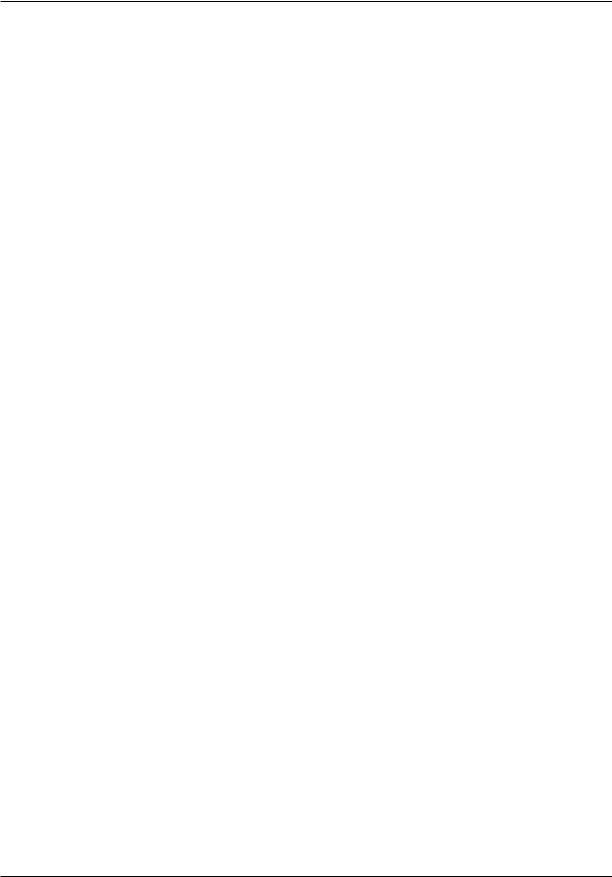
EVENT HISTORY ANALYSIS
Clemimsky and William R. Shadish, eds., Evaluation for the Twenty-first Century. Thousand Oaks, Calif.: Sage.
Figueredo, Aurelio 1993 ‘‘Critical Multiplism, MetaAnalysis, and Generalization: An Integrative Commentary. ‘‘In L. Sechrest, ed., Program Evaluation: A Pluralistic Enterprise (New Directions for Program Evaluation, No. 60). San Francisco: Jossey-Bass.
Freeman, Howard 1992 ‘‘Evaluation Research.’’ In E. Borgatta and M. Borgatta, eds., Encyclopedia of Sociology. New York: Macmillan.
Gore, Albert 1993 From Red Tape to Results: Creating a Government That Works Better and Costs Less. New York: Plume/Penguin.
Guba, Egon, and Yvonna Lincoln 1981 Effective Evaluation. San Francisco: Jossey-Bass.
Leviton, Laura, and Edward Hughes 1981 ‘‘Research on the Utilization of Evaluations: A Review and Synthesis.’’ Evaluation Review 5:525–548.
Lincoln, Yvonna 1991 ‘‘The Arts and Sciences of Program Evaluation.’’ Evaluation Practice 12:1–7.
Reichardt, Charles, and Sharon Rallis 1994 ‘‘The Relationship Between the Qualitative and Quantitative Research Traditions.’’ In Charles Reichardt and Sharon Rallis, eds., (New Directions for Program Evaluation, No. 61). San Francisco: Jossey-Bass.
Rossi, Peter 1994 The War Between the Quals and the Quants. Is a Lasting Peace Possible? In Charles Reichardt and Sharon Rallis, eds., The Qualitative– Quantitative Debate: New Perspectives (New Directions for Program Evaluation, No. 61). San Francisco: Jossey-Bass.
———, and Howard E. Freeman 1993 Evaluation, fifth ed. Newbury Park, Calif.: Sage.
Rutman, Leonard 1984 Evaluation Research Methods.
Newbury Park, Calif.: Sage.
Scriven, Michael 1991 Evaluation Thesaurus, fourth ed. Newbury Park, Calif.: Sage.
——— 1993 Hard-Won Lessons in Program Evaluation
(New Directions for Program Evaluation, No. 58). San Francisco: Jossey-Bass.
Sechrest, Lee 1992 ‘‘Roots: Back to Our First Generations.’’ Evaluation Practice 13:1–7.
Shadish, William 1993 ‘‘Critical Multiplism: A Research Strategy and Its Attendant Tactics.’’ In L. Sechrest, ed., Program Evaluation: A Pluralistic Enterprise (New Directions for Program Evaluation, No. 60). San Francisco: Jossey-Bass.
———, Thomas Cook, and Laura Leviton 1991 Foundations of Program Evaluation: Theories of Practice. Newbury Park, Calif.: Sage.
Sonnad, Subhash, and Edgar Borgatta 1992 ‘‘Evaluation Research and Social Gerontology.’’ Research on Aging 14:267–280.
Stephan, A. Stephen 1935 ‘‘Prospects and Possibilities: The New Deal and the New Social Research.’’ Social Forces 13:515–521.
Suchman, Edward 1967 Evaluative Research: Principles and Practice in Public Service and Social Action Programs. New York: Russell Sage Foundation.
Weiss, Carol 1975 ‘‘Evaluation Research in the Political Context.’’ In Marcia Guttentag and Elmer Struenning, eds., Handbook of Evaluation Research, vol. 1. Beverly Hills, Calif.: Sage.
——— 1987 ‘‘Evaluating Social Programs: What Have We Learned?’’ Society 25:40–45.
KARL KOSLOSKI
EVENT HISTORY ANALYSIS
Event history analysis is a collection of statistical methods for the analysis of longitudinal data on the occurrence and timing of events. As used in sociology, event history analysis is very similar to linear or logistic regression analysis, except that the dependent variable is a measure of the likelihood or speed of event occurrence. As with other regression methods, event history analysis is often used to develop causal or predictive models for the occurrence of events. Event history analysis has become quite popular in sociology since the mid 1980s, with applications to such diverse events as divorces (Bennett et al. 1988), births (Kallan and Udry 1986), deaths (Moore and Hayward 1990), job changes (Carroll and Mayer 1986), organizational foundings (Halliday et al. 1987), migrations (Baydar et al. 1990), and friendship choices (Hallinan and Williams 1987).
Although event history methods have been developed and utilized by statistical practitioners in a variety of disciplines, the term event history analysis is primarily used in sociology and closely allied disciplines. Elsewhere the methodology is known as survival analysis (biology and medicine), failure-time analysis (engineering), or duration analysis (economics). Introductory treatments for social scientists can be found in Teachman (1983), Allison (1984, 1995), Tuma and Hannan (1984), Kiefer (1988), and Blossfeld and Rohwer (1995).
869

EVENT HISTORY ANALYSIS
For a biostatistical point of view, see Collett (1994), Kleinbaum (1996), or Klein and Moeschberger (1997).
EVENT HISTORY DATA
The first requirement for an event history analysis is event history data. An event history is simply a longitudinal record of when events occurred for an individual or a sample of individuals. For example, an event history might be constructed by asking a sample of people to report the dates of any past changes in marital status. If the goal is a causal analysis, the event history should also include information on explanatory variables. Some of these, such as race and gender, will be constant over time while others, such as income, will vary. If the timing of each event is known with considerable precision (as with exact dates of marriages), the data are called continuous-time data. Frequently, however, events are only known to have occurred within some relatively large interval of time, for example, the year of a marriage. Such data are referred to as discrete-time data or grouped data.
Event history data are often contrasted with panel data, in which the individual’s status is known only at a set of regular, fixed points in time. For example, employment status and other variables may be measured in annual interviews. Panel data collected at frequent intervals can often be treated as discrete-time event history data. But if the intervals between data collections are long, one of the major attractions of event history analysis can be lost—the ability to disentangle causal ordering. While this capability is by no means unequivocal, the combination of event history data and event history analysis is perhaps the best available nonexperimental methodology for studying causal relationships.
PROBLEMS WITH CONVENTIONAL
METHODS
Despite the attractiveness of event history data, they typically possess two characteristics that make conventional statistical methods highly unsuitable. Censoring is the most common problem. Suppose, for example, that the aim is to study the causes of divorce. The sample might consist of a
number of couples who married in 1990 and who are followed for the next five years. For the couples who get divorced, the length of the marriage is the principal variable of interest. But a large fraction of the couples will not divorce during the fiveyear interval. Marriages that are still in progress when the study ends are said to be censored. The problem is to combine the data on timing with the data on occurrence in a statistically consistent fashion. Ad hoc methods, such as excluding the censored cases or assigning the maximum length of time observed, can lead to substantial biases or loss of precision.
The second problem is time-varying explanatory variables (also known as time-dependent covariates). Suppose, in our divorce example, that the researcher wants to include number of children as a predictor of divorce. But number of children may change over the marriage, and it is not obvious how such a variable should be included in a regression model. If there were no censored cases, one might be tempted to regress the length of the marriage on the number of children at the end of the marriage. But longer marriages are likely to have produced more children simply because more time is available to have them. This would produce a spurious positive relationship between number of children and the length of the marriage.
One method for dealing with the censoring problem has been around since the seventeenth century and is still widely used—the life table. The life table is one example of a variety of methods that are primarily concerned with estimating the distribution of event times without regard for the effects of explanatory variables. For a comprehensive survey of such methods, see Elandt-Johnson and Johnson (1980). The remainder of this article focuses on regression methods that estimate the effects of explanatory variables on the occurrence and timing of events.
ACCELERATED FAILURE-TIME MODELS
Suppose the goal is to estimate a model predicting the timing of first marriages, and the sample consists of women who are interviewed at age twentyfive. For each woman (i=1, . . . ,n), we learn her age in days at the time of the marriage, denoted by Ti.
870

EVENT HISTORY ANALYSIS
For women who still were not married at age twenty-five (the censored cases), Ti* is their age in days at the time of the interview. We also have data on a set of explanatory variables xi1, . . . , x11. For the moment, let us suppose that these are variables that do not change over time, such as race, parents’ education, and eighth-grade test scores.
One class of models that is appropriate for data such as these is the accelerated failure-time (AFT) model. The general formulation is
log T i = β0 + β1xi1 +...+ β2xi2 + εi |
(1) |
By taking the logarithm on the left-hand side, we ensure that Ti is always greater than 0, regardless of the values of the x variables. Specific cases of this general model are obtained by choosing particular distributions for the random disturbance εi. The most common distributions are normal, extremevalue, logistic, and log-gamma. These imply that Ti has distributions that are, respectively, lognormal, Weibull, log-logistic, and gamma, which are the names usually given to these models. The disturbance term εi is assumed to be independent of the x’s and to have constant variance.
If there are no censored data, these models can be easily estimated by ordinary least-squares regression of log T on the x’s. The resulting coefficients are best linear unbiased estimators. But the presence of censored data requires a different method. The standard approach is maximum likelihood, which combines the censored and uncensored data in an optimal fashion. Maximum likelihood estimation for these models is now widely available in several statistical packages (e.g., BMDP, LIMDEP, SAS, SYSTAT, Stata).
Here’s an example from criminology. In the early 1970s, 432 inmates from Maryland state prisons were followed for one year after their release (Rossi et al. 1980). The event of interest is the first arrest that occurred to each person during the one-year observation period. Only 26 percent of the released inmates were arrested. We’ll use the following variables:
ARREST |
1 if arrested, otherwise 0 |
WEEK |
Week of first arrest for those who |
were arrested (ranges 1 to 52); for those not arrested, week 52
FIN 1 if they received financial aid after release, otherwise 0
AGE |
Age in years at the time of release |
RACE |
1 if black, otherwise 0 |
MAR |
1 if married at the time of release, |
otherwise 0 |
|
PRIO |
Number of prior convictions |
Using these data, I estimated a Weibull version of the accelerated failure time model by maximum likelihood with the SAS® statistical package. Results are shown in Table 1. Looking first at the p- values, we see that race and marital status do not have a significant impact on the timing of arrests. On the other hand, we see highly significant effects of age and number of prior convictions, and a just barely significant effect of financial aid.
The negative coefficient for PRIO tells us that having more prior convictions is associated with shorter times to arrest. The positive coefficient for AGE tells us that inmates who were older when they released have longer times to arrest. Similarly, those who got financial aid have longer times to arrest. We can interpret the magnitudes of the coefficients by applying the transformation 100[exp(ß)−1], which gives the percentage change in time to event for a 1-unit increase in a particular independent variable. For PRIO we get 100[exp(−.071)−1]=−6.8, which tells us that each additional conviction lowers the time to arrest by 6.8 percent, controlling for other variables in the model. For FIN we get 100[exp(.268)−1]=31. Those who got financial aid have times to arrest that are 31 percent longer than those who did not get financial aid.
In addition to the Weibull model, I also estimated gamma, lognormal, and log-logistic models. Results were very similar across the different models.
PROPORTIONAL HAZARDS MODELS
A second class of regression models for continu- ous-time data is the proportional hazards model. To explain this model, it is first necessary to define the hazard function, denoted by h(t), which is the fundamental dependent variable. Let P(t+∆t) be the conditional probability that an event occurs in the time interval (t+∆t), given that it has not already
871

EVENT HISTORY ANALYSIS
Results from Weibull Regression Model Predicting the Time of First Arrest
Variable |
Coefficient |
Standard Error |
Chi-Square |
p-value |
FIN |
.268 |
.137 |
3.79 |
.05 |
AGE |
.043 |
.015 |
8.00 |
.004 |
RACE |
-.226 |
.220 |
1.06 |
.30 |
MAR |
.353 |
.269 |
1.73 |
.19 |
PRIO |
-.071 |
.020 |
12.85 |
.0003 |
Intercept |
4.037 |
.402 |
|
|
Table 1
occurred prior to t. To get the hazard function, we divide this probability by the length of the interval ∆t, and take the limit as ∆t goes to 0:
h(t) = lim |
P (t,t + ∆t) |
(2) |
|
∆t |
|||
∆t→0 |
|
Other common symbols for the hazard function are r(t) and λ(t). The hazard may be regarded as the instantaneous likelihood that an event will occur at exactly time t. It is not a probability, however, since it may be greater than 1.0 (although never less than 0).
If h(t) has a constant value c, it can be interpreted as the expected number of events in a 1- unit interval of time. Alternatively, 1/c is the expected length of time until the next event. Suppose, for example, that the events are residence changes, time is measured in years, and the estimated hazard of a residence change is .20. That would imply that, for a given individual, the expected number of changes in a year is .20 and the expected length of time between changes is 1/.20 = 5 years.
Like a probability (from which it is derived), the hazard is never directly observed. Nevertheless, it governs both the occurrence and timing of events, and models formulated in terms of the hazard may be estimated from observed data.
The general proportional hazards (PH) model is given by
log h i (t) = α(t) + β1xi1 +...+ βkxik |
(3) |
where α(t) may be any function of time. It is called the proportional hazards model because the ratio of the hazards for any two individuals is a constant over time. Notice that, unlike the AFT model,
there is no disturbance term in this equation. That does not mean that the model is deterministic, however, because there is random variation in the relationship between h(t) and the observed occurrence and timing of events.
Different versions of the PH model are obtained by choosing specific forms for α(t). For example, the Gompertz model sets α(t=αi+α2t, which says that the hazard is an increasing (or decreasing) function of time. Similarly, the Weibull model has α(t)=αi+α2 log t. (The Weibull model is the only model that is a member of both the AFT class and the PH class.) The exponential model—a special case of both the Weibull and the Gompertz mod- els—sets α(t) = α, a constant over time. For any specific member of the PH class, maximum likelihood is the standard approach to estimation.
In a path-breaking paper, the British statistician David Cox (1972) showed how the PH model could be estimated without choosing a specific functional form for α(t), using a method called partial likelihood. This method is very much like maximum likelihood, except that only a part of the likelihood function is maximized. Specifically, partial likelihood takes account only of the ordering of events, not their exact timing. The combination of partial likelihood and the proportional hazards model has come to be known as Cox regression. The method has become extremely popular because, although some precision is sacrificed, the resulting estimates are much more robust. Computer programs that implement this method are now available in most full-featured statistical packages (SPSS, SAS, LIMDEP, BMDP, S-Plus, Stata, SYSTAT).
As an example, we’ll estimate a proportional hazards model for the recidivism data discussed
872

EVENT HISTORY ANALYSIS
Results from Cox Regression Model Predicting the Hazard of First Arrest
Variable |
Coefficient |
Standard Error |
Chi-Square |
p-value |
FIN |
-.373 |
.191 |
3.82 |
.05 |
AGE |
-.061 |
.021 |
8.47 |
.004 |
RACE |
.317 |
.308 |
1.06 |
.30 |
MAR |
-.493 |
.375 |
1.73 |
.19 |
PRIO |
.099 |
.027 |
13.39 |
.0003 |
Intercept |
4.037 |
.402 |
|
|
Table 2
earlier. The chi-squares and p-values shown in Table 2 for the five variables are remarkably similar to those in Table 1 that were obtained with maximum likelihood estimation of a Weibull model. On the other hand, the coefficients are noticeably different in magnitude and even have signs that are reversed from those in Table 1. The sign reversal is a quite general phenomenon that stems from the fact that the dependent variable is the time of the event in the AFT model and the hazard of the event in the PH model. People with high hazards are very likely to have events at any point in time, so their times to events tend to be short. By contrast, people with low hazards tend to have long times until event occurrence.
To interpret the magnitudes of the coefficients, we can use the same transformation used for the AFT models. Specifically, 100[exp(ß)−1] gives the percentage change in the hazard of an event for a 1-unit increase in a particular independent variable. Thus, for FIN we have 100[exp(−.373)−1] = −31, which says that those who got financial aid have hazards of arrest that are 31 percent lower than those who did not get aid. For AGE we have 100[exp(−.061)−1] = −6. Each additional year of age at release yields a 6 percent reduction in the hazard of arrest. Finally, each additional conviction is associated with 100[exp(.099)−1] = 10.4 percent increase in the hazard of an arrest.
The partial likelihood method also allows one to easily introduce time-varying explanatory variables. For example, suppose that the hazard for arrest is thought to depend on both financial aid (x1) and employment status (x2, coded 1 for employed, 0 for unemployed). A suitable PH model might be
log h(t) = α(t) + β1x1 + β2x2(t) |
(4) |
which says that the hazard at time t depends on financial aid, on employment status at time t, and on time itself. If longitudinal data on income are available, models such as this can be estimated in a straightforward fashion with the partial likelihood method. How to do this is shown in Chapter 5 of Allison (1995).
MULTIPLE KINDS OF EVENTS
To this point, it has been assumed that all events can be treated alike. In many applications, however, there is a compelling need to distinguish among two or more types of events. For example, if the events of interest are job terminations, one might expect that explanatory variables would have vastly different effects on voluntary and involuntary terminations. For recidivism studies, it might be desirable to distinguish arrests for crimes against persons and crimes against property. The statistical analysis should take these distinctions into account.
All of the methods already discussed can be easily applied to multiple kinds of events. In essence, a separate model is estimated for each kind of event. In doing an analysis for one kind of event, one simply treats other kinds of events as though the individual were censored at the time when the event occurred, a method known as ‘‘competing risks.’’ Thus, no new methodology is required to handle this situation.
An alternative approach is to estimate a single event history model for the timing of events, without distinguishing different event types. Then,
873

EVENT HISTORY ANALYSIS
after eliminating all the individuals who did not have events (the censored cases), one estimates a logistic regression model for the determinants of the type of event. This method of analysis is most appropriate when the different kinds of events are functionally alternative ways of achieving a single objective. For example, the event might be purchase of a computer and the two different types might be a Windows-based computer versus a Macintosh computer.
REPEATED EVENTS
The discussion so far has presumed that each individual experiences no more than one event. Obviously, however, such events as childbirths, job changes, arrests, and car purchases can occur many times over the life of an individual. The methods already described have been routinely applied to cases of repeated events, taking one of two alternative approaches. One approach is to do a separate analysis for each successive event. For example, one event history model is estimated for the birth of the first child, a second model is estimated for the birth of the second child, and so on. The alternative approach is to break each individual’s event history into a set of intervals between events, treat each of these intervals as a distinct observation, and then pool all the intervals into a single analysis.
Neither of these alternatives is entirely satisfactory. The sequential analysis is rather tedious, wastes information if the process is invariant across the sequence, and is prone to selection biases for later events in the sequence. The pooled analysis, on the other hand, makes the rather questionable assumption that the multiple intervals for a single individual are independent. This can lead to standard errors that are biased downward and test statistics that are biased upward.
Several methods are available for dealing with the lack of independence. One is to estimate standard errors and test statistics using the robust method developed by White (1982). These robust standard errors have been incorporated into some Cox regression programs (e.g., Stata, S-Plus). Another is to do a ‘‘fixed-effects’’ Cox regression that stratifies on the individual (Allison 1996; Yamaguchi 1986). These models can be estimated with most Cox regression software, and they have the advantage of automatically controlling for all stable
characteristics of the individual. On the other hand, fixed-effects models cannot produce coefficient estimates for stable characteristics such as sex or race. Finally, there are ‘‘random-effects’’ or ‘‘frailty’’ models that explicitly build the dependence into the model as an additional random disturbance. Unfortunately, there is little commercial software available to estimate random-effects event history models.
DISCRETE-TIME METHODS
When event times are measured coarsely, the con- tinuous-time methods already discussed may yield somewhat biased estimates. In such cases, methods specifically designed for discrete-time data are more appropriate (Allison 1982). Moreover, such methods are easily employed and are particularly attractive for handling large numbers of timevarying explanatory variables.
Suppose that the time scale is divided into a set of equal intervals, indexed by t = 1, 2, 3, . . . The discrete-time analog of the hazard function, denoted by Pt, is the conditional probability that an event occurs in interval t, given that it has not occurred prior to t. A popular model for expressing the dependence of Pt on explanatory variables is the logit model
log(1–PPit it )= αt + β1xi1 +...+ β2xi2 (5)
where the subscript on αt indicates that the intercept may differ for each interval of time. Similarly the explanatory variables may take on different values at each interval of time. This model can be estimated by the method of maximum likelihood, using the following computational strategy:
1.Break each individual’s event history into a set of discrete time units, for example, person-years.
2.Create a dependent variable that has a value of 1 for time units in which events occurred; otherwise use 0. Explanatory variables are assigned whatever values they had at the beginning of the time unit.
3.Pool all these time units, and estimate a logistic regression model using a standard maximum likelihood logit program.
874

EVOLUTION: BIOLOGICAL, SOCIAL, CULTURAL
Other models and computational methods are also available for the discrete-time case. These methods can be easily extended to allow for multiple kinds of events and repeated events.
REFERENCES
Kiefer, Nicholas M. 1988 ‘‘Economic Duration Data and Hazard Functions.’’ Journal of Economic Literature
26:646–679.
Klein, John P., and Melvin Moeschberger 1997 Survival Analysis: Techniques for Censored and Truncated Data. New York: Springer-Verlag.
Allison, Paul D. 1982 ‘‘Discrete Time Methods for the Analysis of Event Histories.’’ In Samuel Leinhardt, ed., Sociological Methodology 1982. San Francisco: Jossey-Bass.
———1984 Event History Analysis. Beverly Hills, Calif.: Sage.
———1995 Survival Analysis Using the SAS® System: A Practical Guide. Cary, N.C.: SAS Institute.
———1996 ‘‘Fixed Effects Partial Likelihood for Repeated Events.’’ Sociological Methods and Research
25:207–222.
Baydar, Nazli, Michael J. White, Charles Simkins, and Ozer Babakol 1990 ‘‘Effects of Agricultural Development Policies on Migration in Peninsular Malaysia.’’
Demography 27:97–110.
Bennett, Neil G., Ann Klimas Blanc, and David E. Bloom 1988 ‘‘Commitment and the Modern Union: Assessing the Link Between Premarital Cohabitation and Subsequent Marital Stability.’’ American Sociological Review 53:127–138.
Blossfeld, Hans-Peter, and Götz Rohwer 1995 Techniques of Event History Modeling: New Approaches to Causal Modeling. Mahwah, N.J.: Lawrence Earlbaum.
Carroll, Glenn R., and Karl Ulrich Mayer 1986 ‘‘JobShift Patterns in the Federal Republic of Germany: The Effects of Social Class, Industrial Sector, and Organizational Size.’’ American Sociological Review
51:323–341.
Collett, D. 1994 Modelling Survival Data in Medical Research. London: Chapman and Hill.
Cox, David R. 1972 ‘‘Regression Models and Life Tables.’’ Journal of the Royal Statistical Society, Series B, 34:187–202.
Elandt-Johnson, R. C., and N. L. Johnson 1980 Survival Models and Data Analysis. New York: Wiley.
Halliday, Terence C., Michael J. Powell, and Mark W. Granfors 1987 ‘‘Minimalist Organizations: Vital Events in State Bar Associations, 1870–1930.’’ American Sociological Review 52:456–471.
Hallinan, Maureen, and Richard A. Williams 1987 ‘‘The Stability of Students’ Interracial Friendships.’’ American Sociological Review 52:653–664.
Kallan, Jeffrey, and J. R. Udry 1986 ‘‘The Determinants of Effective Fecundability Based on the First Birth Interval.’’ Demography 23:53–66.
Kleinbaum, David G. 1996 Survival Analysis: A SelfLearning Text. New York: Springer-Verlag.
Moore, David E., and Mark D. Hayward 1990 ‘‘Occupational Careers and Mortality of Elderly Men.’’ Demography 27:31–53.
Rossi, P. H., R. A. Berk, and K. J. Lenihan 1980 Money, Work and Crime. New York: Academic Press.
Teachman, Jay D. 1983 ‘‘Analyzing Social Processes: Life Tables and Proportional Hazards Models.’’ Social Science Research 12:263–301.
Tuma, Nancy Brandon, and Michael T. Hannan 1984
Social Dynamics: Models and Methods. Orlando, Fla.: Academic Press.
White, Halbert 1982 ‘‘Maximum Likelihood Estimation of Misspecified Models.’’ Econometrica 50:1–25.
Yamaguchi, Kazuo 1986 ‘‘Alternative Approaches to Unobserved Heterogeneity in the Analysis of Repeated Events.’’ In Nancy Brandon Tuma, ed., Sociological Methodology 1986. Washington, D.C.: American Sociological Association.
PAUL D. ALLISON
EVOLUTION: BIOLOGICAL, SOCIAL, CULTURAL
The diverse forms of life on earth have emerged probably from a common source, through a process of evolution that has the following characteristics:
1.The course of evolution does not always proceed along a straight path (for example, from simple to complex forms).
Instead, it can meander like a stream, directed largely by environmental circumstances, although also affected by limitations on the capacities of organisms to respond to environmental challenges, and by unpredictable ‘‘chance’’ factors. Occasionally it reverses direction in certain respects, as when our own distant monkeylike ancestors became adapted to life in
875

EVOLUTION: BIOLOGICAL, SOCIAL, CULTURAL
the trees, and our more recent ancestors readapted to living on the ground. When the course of evolution does reverse direction, it generally does so with respect to comparatively few features only, not all features. Thus, we humans retain various characteristics evolved earlier in connection with life in the trees: stereoscopic vision, visual acuity, reduced sense of smell, and hands adapted for grasping.
2.Different groups of organisms sometimes evolve in similar directions in certain respects when exposed to similar environmental conditions. Thus whales—descen- dants of mammals that lived on land— acquired fishlike shapes when they adapted to life in the water. However, parallelism in evolutionary development generally remains limited: different evolutionary lines do not come close to ‘‘merging.’’ Whales, even though living in the
ocean and acquiring fishlike shapes, retain basic anatomical and physiological features of mammals, quite different from those of fish.
The life cycle of a human being involves fixed stages (infancy, childhood, adolescence, adulthood, old age) that represent an unfolding of innate potentialities and that culminate in inevitable death. The evolution of a new biological species, by contrast, is a unique historical development involving movement in directions shaped primarily by environmental pressures, without any inevitable ‘‘death’’ or other pre-determined end state.
A ‘‘Lamarckian’’ evolutionary process (named after Jean Baptiste Lamarck, 1744–1829), would involve inheritance of acquired characteristics. Thus, if evolution followed the Lamarckian pattern, giraffes might have acquired their long necks because in each generation necks were stretched (perhaps to obtain food high up in trees, or to detect approaching enemies), and because the effect of each generation’s stretching tended to be inherited by the generation that followed. However, Lamarckian ideas were found to be invalid long ago.
A different approach developed by Charles Darwin (1859) and others does not assume that
acquired characteristics (such as effects of neckstretching) are inherited. Rather, it assumes (1) random or randomlike variation among the offspring in each generation (with some giraffes happening by chance to have longer necks than others); (2) natural selection, involving tendencies for certain variants (longer-necked giraffes) to survive and reproduce more than others; and (3) preservation through inheritance of the effects of natural selection (longer-necked giraffes tending to have similarly long-necked offspring, although—as suggested above—there would still be some random variation among these offspring with respect to neck length).
Darwinian ideas challenged traditional Christian religious beliefs by suggesting (1) that we are descended from ape-like creatures and, ultimately, from elementary life forms; (2) that our evolution was basically an unplanned outcome of diverse environmental pressures rather than something planned in advance; and (3) that the earth is old enough for evolutionary processes to have had time to produce the variety and complexity of life forms that we actually find. Although today still resisted by many on religious grounds, Darwinian theory ultimately came to be generally accepted by biologists, with important modifications and certain disagreements about details, and in combination with new knowledge in other areas of biology and other scientific disciplines that was not available to Darwin (see Gould 1982; Stebbins and Ayala 1985; Mayr and Provine 1998).
An inherited trait that has evolved over a long period of time (like the giraffe’s long neck) has very likely evolved because it makes some contribution to the survival and reproduction of the organisms possessing it, and because it has consequently emerged and persisted through the Darwinian mechanisms outlined above. However, some traits may appear and persist that are neutral in their implications for survival and reproduction, and even traits that have negative implications may appear and persist if they happen to be linked with traits whose implications are positive.
Bioevolutionary explanations of human social phenomena, sometimes under the label of ‘‘sociobiology’’ (see Wilson 1975), pose a challenge to sociology, as the following example suggests. Stepparents tend to abuse their stepchildren
876

EVOLUTION: BIOLOGICAL, SOCIAL, CULTURAL
more than biological parents tend to abuse their own children. Sociologists would ordinarily seek to explain this in a way that utilizes sociological and social-psychological concepts only, avoiding assumptions about inherited and biologically evolved traits. A sociobiologist, by contrast, would be more likely to view it as a human manifestation or extension of a biologically evolved tendency prevailing widely among nonhuman mammals: a tendency for individual mammals in stepparent-like positions to kill their ‘‘stepchildren,’’ thus increasing their own prospects for producing survivable offspring and perpetuating their own genes (see Beckstrom 1993, pp. 23–29).
An evolutionary explanation of an aspect of human life or society may present that aspect as having emerged (1) as a part of, or a continuation of, biological evolution (as illustrated in the discussion of stepparental child abuse above), or (2) through a process analogous to biological evolution but nevertheless distinct from it, that is, a process of social or cultural evolution. Concepts analogous to those of biological evolution are especially applicable to aspects of culture such as science (Hull 1988) and technology (Basalla 1988) in which the cumulative character of culture is most strongly manifested. Competing scientific hypotheses are the ‘‘randomlike variations’’ in science as an evolutionary process. Research results that evaluate such hypotheses select some of them for survival and others for extinction, and the knowledge that constitutes the outcome of this process in any given generation of scientists is ‘‘inherited’’ by subsequent generations through textbooks, teaching, and research publications. In technological evolution, positive selection (i.e., acceptance) of variations (innovations) depends not only on research results (i.e., on how well they ‘‘work’’) but also on costs, competitive pressures, compatibility with prevailing culture, and other factors.
The evolutionary model is not appropriately applied to social or cultural changes that are cyclical, easily reversed, primarily planned, or repetitive. It may be usefully applied when a complex social or cultural transformation that would be hard to repeat or to reverse occurs gradually without being planned, through environmentally determined selections from among divergent alternative directions of change, and with enough
stability to preserve the results of past environmental influence (see Campbell 1965; Richter 1982, pp. 19-34).
Not every process that is called ‘‘evolutionary’’ seems to deserve that label. Some theories pertaining to major transformations of society illustrate this point.
The idea that human society evolves from simple beginnings through comparatively fixed stages came to be commonly accepted in the nineteenth century, although different theorists had different conceptions of what these stages were. Thus we find analyses of transitions from theological to metaphysical to ‘‘positivistic’’ thought-styles (Comte 1875); from savagery to barbarism to civilization (Morgan 1877); from tribalism to slavery, feudalism, capitalism, and then communism (Marx and Engels [1846] 1947); and from simple to compounded, doubly compounded and then triply compounded societies (Spencer [1885–1886] 1967).
When societies, cultures, or civilizations are said to pass from childhood to adulthood, and then to old age, or are said to grow and then decline, we have an analogy not with the evolution of a species but rather with the human life cycle, as illustrated in the works of Oswald Spengler and Arnold J. Toynbee. Nineteenth century ‘‘socialevolutionary’’ theories departed from the life-cy- cle model in that they did not involve the idea of decline or old age followed by death as the end point of a cycle. Quite the opposite: the trends they described tended to culminate in triumphant achievements—’’positivism’’ (Comte), ‘‘civilization’’ (Morgan), ‘‘communism’’ (Marx and Engels), and societies ‘‘compounded’’ many times (Spencer). In this one respect, but only in this respect, these theories resembled the bioevolutionary model: biological species do not have to become extinct— even though many of them actually do—in the same sense that individuals have to die. However, these theories nevertheless reflect a life-cycle model, insofar as they involve one society after another following essentially the same pattern of development, just as one infant after another follows the same general route to adulthood. And they do not appear to involve, in any major way, the evolutionary mechanisms of random variation and selection. In fact, they are primarily theories of progress, not of evolution in any sense that biologists
877

EVOLUTION: BIOLOGICAL, SOCIAL, CULTURAL
would recognize, regardless of the ‘‘evolutionary’’ label commonly attached to them (Nisbet 1969).
The social-evolutionary idea fell into disfavor among sociologists around the turn of the twentieth century, but was revived several decades later with, for example, Talcott Parsons’ analysis of primitive, intermediate, and modern societies (1977), and Lenski and Lenski’s (1982) analysis of transitions from hunting-and-gathering to horticultural, agrarian, and industrial societies. Although based on more accurate and more extensive facts than were available in the nineteenth century, these newer schemes pertaining to the evolution of whole societies are nevertheless basically similar to their nineteenth century predecessors in that they present various societies as passing through specific stages in a predominantly unidirectional pattern, which, as noted above, represents a divergence from the bioevolutionary model. This is not necessarily a defect: the development of societies of new types may simply not be a process to which concepts of a bioevolutionary sort are fully applicable.
Evolutionary concepts have been used not only in attempts to understand some social and cultural phenomena, as discussed above, but also in attempts to formulate and justify certain social policies. In the late nineteenth and early twentieth centuries, evolutionary concepts and associated slogans such as ‘‘survival of the fittest’’ appeared to provide rationales for what have been labelled ‘‘Social Darwinist’’ policies. Focusing on the selective (rather than on the random-variation) phase of the evolutionary process, various business, political, ideological, and military leaders in several countries, along with some scholars in several disciplines (including the sociologists Herbert Spencer and William Graham Sumner), emphasized the importance of the struggle for survival in maintaining a hardy population and a vigorous society, and opposed social welfare measures that they thought would mitigate that struggle. Supporters of this approach differed in several ways: some emphasized individual struggle, others, group (e.g., racial or national) struggle; some emphasized nonviolent means (e.g., economic competition), and others, armed conflict (see Hofstadter 1955).
A statement by Darwin himself illustrates the basic idea underlying ‘‘Social Darwinism.’’ Although Darwin did not oppose smallpox vaccination, he
worried about its effects: ‘‘There is reason to believe that vaccination has preserved thousands, who from a weak constitution would formerly have succumbed to smallpox. Thus the weak members of civilized societies propagate their kind. No one who has attended to the breeding of domestic animals will doubt that this must be highly injurious to the race of man’’ (Darwin [1871] 1897, p. 134).
Darwin did not believe that war led to the selective survival of the fittest people, as he thought smallpox tended to do. Quite the opposite: he saw war as destroying ‘‘the finest’’ young men while those with ‘‘poor constitutions’’ would avoid fighting and thus survive and reproduce.
But evolutionary concepts can be applied at diverse levels. Nineteenth-century war might have led to the selective survival of societies or governments that were ‘‘fittest’’ in some sense, even if not to the survival of the ‘‘fittest’’ young men within particular societies. Some Social Darwinists adopted that perspective. Thus: ‘‘Storm purifies the air and destroys the frail trees, leaving the sturdy oaks standing. War is the test of a nation’s political, physical and intellectual worth. The State in which there is much that is rotten may vegetate for a while in peace, but in war its weakness is revealed. . . . It is better to spend money on armaments and battleships than luxury, motormania and other sensual living’’ (Baron Karl von Stengel, cited by Angell 1911, p. 168).
There is a problem here: what an exceedingly powerful storm is most likely to leave standing are not sturdy oaks but blades of grass. And this leads to a question: what does ‘‘survival of the fittest’’ actually mean? Defining the fittest as ‘‘those who survive’’ would make the phrase tautological, but any other definition would very likely make it untrue except under a limited range of conditions. In any case, ‘‘fitness’’ is not necessarily equivalent to ‘‘desirability’’ as we might define that, or to what the people of a society actually desire.
The problem of defining ‘‘fitness’’ may be illustrated by examining what Darwin said about smallpox. Suppose people who would have succumbed to smallpox if not vaccinated, but who were actually saved by vaccination, have descendants who inherit their potential vulnerability to that disease. In this respect, such descendants might be considered relatively ‘‘unfit.’’ But if these descendants are all protected from smallpox by
878