
- •Matrix computations on systems equipped with GPUs
- •Introduction
- •The evolution of hardware for High Performance Computing
- •The programmability issue on novel graphics architectures
- •About this document. Motivation and structure
- •Motivation and goals
- •Structure of the document
- •Description of the systems used in the experimental study
- •Performance metrics
- •Hardware description
- •Software description
- •The FLAME algorithmic notation
- •The architecture of modern graphics processors
- •The graphics pipeline
- •Programmable pipeline stages
- •The Nvidia G80 as an example of the CUDA architecture
- •The architecture of modern graphics processors
- •General architecture overview. Nvidia Tesla
- •Memory subsystem
- •The GPU as a part of a hybrid system
- •Arithmetic precision. Accuracy and performance
- •Present and future of GPU architectures
- •Conclusions and implications on GPU computing
- •BLAS on single-GPU architectures
- •BLAS: Basic Linear Algebra Subprograms
- •BLAS levels
- •Naming conventions
- •Storage schemes
- •BLAS on Graphics Processors: NVIDIA CUBLAS
- •Evaluation of the performance of NVIDIA CUBLAS
- •Improvements in the performance of Level-3 NVIDIA CUBLAS
- •gemm-based programming for the Level-3 BLAS
- •Systematic development and evaluation of algorithmic variants
- •Experimental results
- •Impact of the block size
- •Performance results for rectangular matrices
- •Performance results for double precision data
- •Padding
- •Conclusions
- •LAPACK-level routines on single-GPU architectures
- •LAPACK: Linear Algebra PACKage
- •LAPACK and BLAS
- •Naming conventions
- •Storage schemes and arguments
- •LAPACK routines and organization
- •Cholesky factorization
- •Scalar algorithm for the Cholesky factorization
- •Blocked algorithm for the Cholesky factorization
- •Computing the Cholesky factorization on the GPU
- •Basic implementations. Unblocked and blocked versions
- •Padding
- •Hybrid implementation
- •LU factorization
- •Scalar algorithm for the LU factorization
- •Blocked algorithm for the LU factorization
- •LU factorization with partial pivoting
- •Computing the LU factorization with partial pivoting on the GPU
- •Basic implementations. Unblocked and blocked versions
- •Padding and hybrid algorithm
- •Reduction to tridiagonal form on the graphics processor
- •The symmetric eigenvalue problem
- •Reduction to tridiagonal form. The LAPACK approach
- •Reduction to tridiagonal form. The SBR approach
- •Experimental Results
- •Conclusions
- •Matrix computations on multi-GPU systems
- •Linear algebra computation on multi-GPU systems
- •Programming model and runtime. Performance considerations
- •Programming model
- •Transfer management and spatial assignation
- •Experimental results
- •Impact of the block size
- •Number of data transfers
- •Performance and scalability
- •Impact of data distribution
- •Conclusions
- •Matrix computations on clusters of GPUs
- •Parallel computing memory architectures
- •Shared memory architectures
- •Distributed memory and hybrid architectures
- •Accelerated hybrid architectures
- •Parallel programming models. Message-passing and MPI
- •ScaLAPACK
- •PLAPACK
- •Elemental
- •Description of the PLAPACK infrastructure
- •Layered approach of PLAPACK
- •Usage of the PLAPACK infrastructure. Practical cases
- •Porting PLAPACK to clusters of GPUs
- •Experimental results
- •Conclusions
- •Conclusions
- •Conclusions and main contributions
- •Contributions for systems with one GPU
- •Contributions for clusters of GPUs
- •Related publications
- •Publications directly related with the thesis topics
- •Publications indirectly related with the thesis topics
- •Other publications
- •Open research lines
- •FLAME algorithms for the BLAS-3 routines

4.7. REDUCTION TO TRIDIAGONAL FORM ON THE GRAPHICS PROCESSOR
The construction of QB that produces the reduction to band form can also be easily done in the GPU, as this computation is basically composed of calls to BLAS-3 kernels.
Reduction to tridiagonal form
Routine SBRDT in the SBR toolbox is responsible for reducing the banded matrix B to tridiagonal form by means of Householder reflectors. Let QT denote the orthogonal transforms which yield this reduction, that is QTT BQT = T . On exit, the routine returns the tridiagonal matrix T and, upon request, accumulates these transforms, forming the matrix Q = QBQT Rn×n so that
QT AQ = QTT (QTB AQB )QT = QTT BQT = T .
The matrix T is constructed in routine SBRDT one column at the time: at each iteration, the elements below the first sub-diagonal of the current column are annihilated using a Householder reflector; the reflector is then applied to both sides of the matrix, resulting in a bulge which has to be chased down along the band. The computation is cast in terms of BLAS-2 operations at best (SYMV and SYR2 for two-sided updates, and GEMV and GER for one-sided updates) and the total cost is 6n2w + 8nw2 flops.
Let Q denote the product of all the Householder reflectors needed to obtain T from B. These transforms are usually accumulated by applying the corresponding sequence of reflectors to the orthogonal matrix that results from the reduction of A to the band matrix B. These transformations can be applied in a blocked fashion by exploiting the WY representation [70]. The accumulation of Q is therefore entirely performed by the fast BLAS-3 GEMM kernel.
If the eigenvectors are desired, then the orthogonal matrix QB produced in the first step (reduction from full to banded form) needs to be updated with the orthogonal transforms computed during the reduction from banded to tridiagonal form building QBQT . This accumulation requires O(n3) flops and can be reformulated almost entirely in terms of calls to level-3 BLAS kernels, even though this reformulation is less trivial than for the first step [32]. However, the matrix Q = QBQT still needs to be applied as part of the back-transform stage, to obtain X := QXT , adding 2n3 flops to the cost of building the matrix containing the eigenvectors of A.
We do not propose to o -load the reduction of the banded matrix to tridiagonal form on the GPU as this is a fine-grained computation which does not lend itself to an easy implementation on this architecture. However, the accumulation of the orthogonal factor produced by this step and the back-transform merely require operations alike the matrix-matrix product, which can be e ciently computed on the GPU.
4.7.4.Experimental Results
Evaluating the performance of the routines for the reduction to tridiagonal form is an elaborate task, due to the large number of factors that have an influence on it. Among them, we will focus on block size, bandwidth for the SBR toolbox, and number of cores. In the following experiments we aim at determining the optimal configuration of these parameters, before proceeding to show a full comparison of the two approaches. For simplicity, we only report results for four problem sizes: 2,048, 6,144, 10,240 and 24,576, and the best bandwidths (w) and block sizes (b) detected in each case, though a complete experiment was carried out for many other values.
Building BLAS-2 and BLAS-3 kernels
We start by analyzing the performance of the low-level kernels involved in the reduction to condensed forms (tridiagonal and banded for the LAPACK and SBR approaches, respectively). For the LAPACK routine SYTRD, these are the symmetric matrix-vector product (SYMV) and the
109
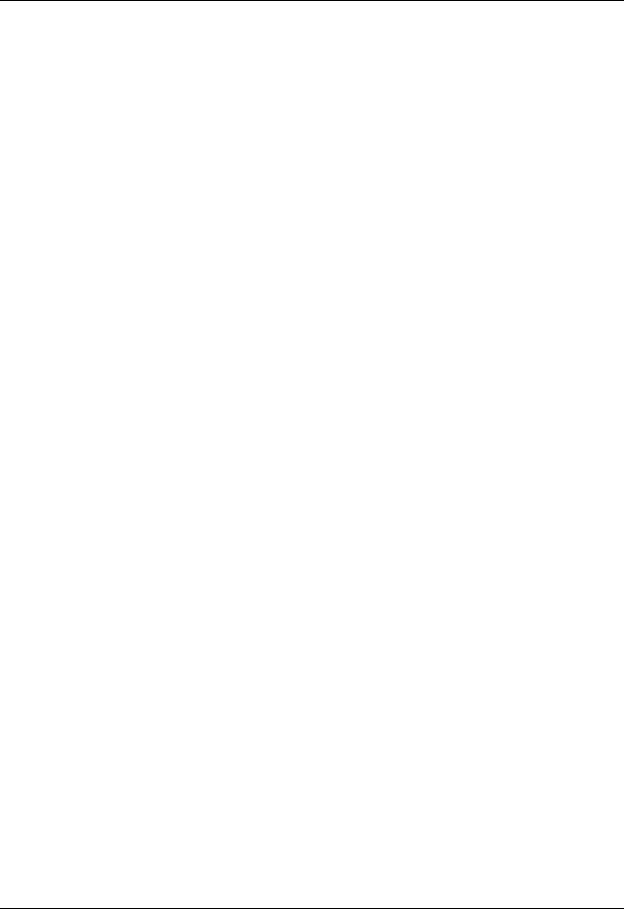
CHAPTER 4. LAPACK-LEVEL ROUTINES ON SINGLE-GPU ARCHITECTURES
symmetric rank-2k update (SYR2K). For the SBR routine SYRDB, the kernels are the symmetric matrix-matrix product (SYMM) and SYR2K. Table 4.3 reports results on 1, 4 and 8 cores of the Intel Xeon Nehalem processors in PECO. For the BLAS-3 kernels (SYMM and SYR2K), we also report the performance on the single GPU in this platform using the implementation in CUBLAS as well as our own implementations, which cast most computations in terms of the general matrix-matrix product [83] (column labeled as “Our BLAS”). The matrix dimensions of SYR2K and SYMM are chosen so that they match the shape of the blocks encountered during the reduction to condensed forms (n is the problem size, while k plays the role of the block size b for SYTRD and that of the bandwidth w for SYRDB). For reference, we also include the performance of the general matrixvector product (GEMV) and matrix-matrix product (GEMM) kernels.
The performance of SYMV increases with the number of cores and is significantly higher than that of GEMV. When the problem size is n = 2,048, the matrix fits into the L3 caches of PECO (8 MB) which explains the much higher GFLOPS rate of SYMV. The same does not hold for GEMV as all the matrix needs to be stored and not just half of it (lower or upper triangle). We will consider these numbers as the basis for our investigation; in other words, justifying the figures yielded by the particular implementation of these kernels in the multi-threaded BLAS implementation is not the purpose of this dissertation.
The two BLAS-3 kernels involved in the computation increase their GFLOPS rate with the number of cores on PECO. This behavior plays a key role in the global performance of the whole reduction process on this architecture.
The results also illustrate the higher performance delivered by the GPU for most BLAS-3 kernels. Although our own implementations of the symmetric BLAS-3 kernels for the GPU deliver higher GFLOPS rates than those from CUBLAS, they are still quite below the performance of the matrix-matrix product kernel in CUBLAS. However, in all cases the performance attained by the GPU is higher than that delivered by the multi-core CPU. The improvement in the performance of the SYMM kernel is of special relevance for the reduction to tridiagonal form using the SBR routines.
The LAPACK approach
We next analyze the gains of a multi-core execution of the LAPACK routines SYTRD and, in case QXT is required, ORMTR. Table 4.4 reports the execution time (in seconds) for di erent values of problem size, block size (b) and number of cores.
Let us comment first on the routine that performs the reduction from full to tridiagonal form, SYTRD. The block size does not play a role in the performance of it. Increasing the number of cores yields a reduction in the execution time on PECO but with moderate speed-up; for example, 3× and 4.2× speed-ups are attained for the largest problem sizes using, respectively, 4 and 8 cores.
The accumulation of the orthogonal transformations via routine ORMTR requires less time and is, in general, more e cient than the reduction stage. This is to be expected, as most of the computations in the accumulation are cast in terms of BLAS-3 kernels (GEMM), while only half of those in SYTRD are BLAS-3. Representative speed-ups of routine ORMTR in PECO are 3.7× and 6.6×, attained respectively using 4 and 8 cores for the largest problem size. Note also that this routine benefits from using block sizes (e.g., 192 and 256) much larger than those that resulted optimal for SYTRD.
The SBR approach
We next study the parallelism of the two-step SBR approach: reduction of a general matrix to band form (SYRDB) and subsequent reduction to tridiagonal form (SBRDT). Also, we include in the
110
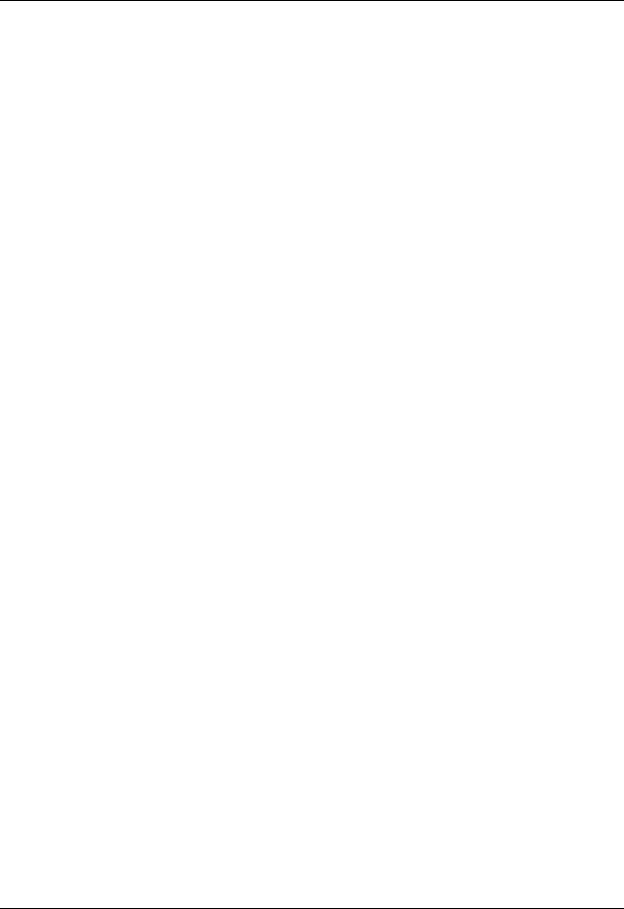
4.7. REDUCTION TO TRIDIAGONAL FORM ON THE GRAPHICS PROCESSOR
|
|
|
|
|
|
|
SYMV. y := Ax + y |
|
|
|
|
|
GEMV. y := Ax + y |
|
|
|||||||||
|
|
|
|
|
|
|
A Rn×n symmetric, x,y Rn |
|
|
|
|
A Rn×n, x,y Rn |
|
|
||||||||||
|
|
|
n |
|
|
1 core |
|
4 cores |
|
8 cores |
|
1 core |
|
4 cores |
|
8 cores |
|
|
||||||
|
|
|
|
|
|
|
|
|
|
|
|
|
|
|
|
|
|
|
|
|
|
|
|
|
|
|
2048 |
|
|
8.26 |
34.5 |
|
60.0 |
|
|
|
5.15 |
|
8.47 |
8.31 |
|
|
|||||||
|
|
6144 |
|
|
7.80 |
17.7 |
|
21.6 |
|
|
|
5.07 |
|
9.13 |
10.7 |
|
|
|||||||
|
10240 |
|
|
6.69 |
18.3 |
|
22.1 |
|
|
|
4.70 |
|
9.26 |
11.2 |
|
|
||||||||
|
24576 |
|
|
5.75 |
16.0 |
|
21.0 |
|
|
|
3.16 |
|
8.45 |
10.8 |
|
|
||||||||
|
|
|
|
|
|
|
|
|
|
|
|
|
|
|
|
|
|
|
|
|
|
|
|
|
|
|
|
|
|
|
|
|
|
|
|
|
|
|
|
|
|
|
|
|
|||||
|
|
|
|
|
|
|
SYR2K. C:= ABT + BAT + C |
|
|
|
|
|
GEMM. C := ABT + C |
|||||||||||
|
|
|
|
|
|
A,B Rn×k, C Rn×n symmetric |
|
|
|
|
A,B Rn×k, C Rn×n |
|||||||||||||
n |
k |
|
|
1 core |
4 cores |
|
8 cores |
CUBLAS |
Our BLAS |
|
|
1 core |
|
4 cores |
|
8 cores |
CUBLAS |
|||||||
|
|
|
|
|
|
|
|
|
|
|
|
|
|
|
|
|
|
|
|
|||||
|
|
|
|
|
|
|
|
|
|
|
|
|
|
|
||||||||||
|
32 |
|
|
14.0 |
|
55.4 |
|
91.0 |
53.2 |
53.2 |
|
|
|
15.0 |
|
58.6 |
|
116.4 |
157.2 |
|||||
2048 |
64 |
|
|
15.5 |
|
62.5 |
|
116.3 |
74.4 |
159.2 |
|
|
|
16.7 |
|
65.9 |
|
129.3 |
185.5 |
|||||
|
96 |
|
|
16.5 |
|
65.3 |
|
122.2 |
78.0 |
162.9 |
|
|
|
17.3 |
|
68.4 |
|
135.9 |
192.2 |
|||||
|
32 |
|
|
13.6 |
|
50.3 |
|
89.6 |
55.9 |
56.0 |
|
|
|
15.0 |
|
59.6 |
|
106.9 |
161.0 |
|||||
6144 |
64 |
|
|
15.7 |
|
59.8 |
|
112.3 |
78.4 |
124.2 |
|
|
|
16.7 |
|
66.6 |
|
123.1 |
185.0 |
|||||
|
96 |
|
|
16.8 |
|
64.4 |
|
122.6 |
81.8 |
126.3 |
|
|
|
17.4 |
|
69.2 |
|
137.8 |
195.1 |
|||||
|
32 |
|
|
13.8 |
|
51.2 |
|
83.9 |
56.4 |
56.4 |
|
|
|
15.0 |
|
59.6 |
|
116.5 |
159.3 |
|||||
10240 |
64 |
|
|
15.8 |
|
60.9 |
|
113.8 |
79.2 |
114.2 |
|
|
|
16.7 |
|
66.7 |
|
130.6 |
182.2 |
|||||
|
96 |
|
|
16.9 |
|
65.3 |
|
123.7 |
78.1 |
116.7 |
|
|
|
17.5 |
|
69.4 |
|
137.7 |
187.3 |
|||||
|
32 |
|
|
13.9 |
|
51.0 |
|
89.9 |
57.4 |
53.4 |
|
|
|
14.7 |
|
58.3 |
|
108.9 |
156.0 |
|||||
24576 |
64 |
|
|
16.0 |
|
60.9 |
|
113.6 |
79.1 |
116.9 |
|
|
|
16.6 |
|
64.6 |
|
129.9 |
186.2 |
|||||
|
96 |
|
|
16.5 |
|
65.1 |
|
123.9 |
83.4 |
112.2 |
|
|
|
17.3 |
|
68.8 |
|
137.5 |
189.9 |
|||||
|
|
|
|
|
|
|
|
|
|
|
|
|
|
|
|
|||||||||
|
|
|
|
|
|
|
SYMM. C := AB + C |
|
|
|
|
|
|
|
GEMM. C := AB + C |
|||||||||
|
|
|
|
|
|
A Rn×n symmetric, B,C Rn×k |
|
|
|
|
A Rn×n, B,C Rn×k |
|||||||||||||
n |
k |
|
|
1 core |
4 cores |
|
8 cores |
CUBLAS |
Our BLAS |
|
|
1 core |
|
4 cores |
|
8 cores |
CUBLAS |
|||||||
|
|
|
|
|
|
|
|
|
|
|
|
|
||||||||||||
|
32 |
|
|
12.2 |
|
29.7 |
|
46.9 |
89.7 |
106.5 |
|
|
|
15.0 |
|
59.9 |
|
99.2 |
177.5 |
|||||
2048 |
64 |
|
|
14.7 |
|
45.2 |
|
75.4 |
97.1 |
183.4 |
|
|
|
16.7 |
|
66.2 |
|
105.3 |
279.0 |
|||||
|
96 |
|
|
15.7 |
|
49.3 |
|
80.4 |
97.9 |
189.4 |
|
|
|
17.4 |
|
68.7 |
|
133.5 |
290.1 |
|||||
|
32 |
|
|
11.9 |
|
28.5 |
|
42.9 |
94.1 |
129.6 |
|
|
|
15.1 |
|
59.5 |
|
116.7 |
327.5 |
|||||
6144 |
64 |
|
|
14.5 |
|
43.9 |
|
71.8 |
99.1 |
188.4 |
|
|
|
16.8 |
|
66.5 |
|
132.2 |
339.3 |
|||||
|
96 |
|
|
15.6 |
|
48.5 |
|
77.5 |
100.4 |
198.1 |
|
|
|
17.5 |
|
69.3 |
|
132.2 |
338.2 |
|||||
|
32 |
|
|
11.1 |
|
25.3 |
|
39.4 |
76.0 |
113.5 |
|
|
|
15.0 |
|
59.5 |
|
116.7 |
321.9 |
|||||
10240 |
64 |
|
|
13.9 |
|
40.5 |
|
66.6 |
76.5 |
175.8 |
|
|
|
16.8 |
|
66.6 |
|
132.4 |
346.9 |
|||||
|
96 |
|
|
15.2 |
|
45.3 |
|
73.4 |
77.5 |
180.0 |
|
|
|
17.4 |
|
69.4 |
|
135.8 |
348.0 |
|||||
|
|
|
|
|
|
|
|
|
|
|
|
|
|
|
|
|
|
|
|
|||||
|
32 |
|
|
10.8 |
|
24.8 |
|
37.9 |
77.8 |
110.0 |
|
|
|
14.7 |
|
58.3 |
|
108.4 |
328.0 |
|||||
20480 |
64 |
|
|
13.5 |
|
39.3 |
|
63.3 |
66.8 |
176.7 |
|
|
|
16.6 |
|
66.0 |
|
131.3 |
344.9 |
|||||
|
96 |
|
|
15.0 |
|
44.6 |
|
65.7 |
65.9 |
179.0 |
|
|
|
17.3 |
|
68.9 |
|
135.3 |
346.0 |
|||||
|
|
|
|
|
|
|
|
|
|
|
|
|
|
|
|
|
|
|
|
|
|
|
|
|
Table 4.3: Performance (in GFLOPS) of the BLAS kernels SYMV (top), SYR2K (middle) and SYMM (bottom) and the corresponding matrix-vector and matrix-matrix products (for reference) on PECO. Peak performance for 1, 4 and 8 cores of this platform are 18.2, 72.6 and 145.3 GFLOPS, respectively.
111

CHAPTER 4. LAPACK-LEVEL ROUTINES ON SINGLE-GPU ARCHITECTURES
|
|
|
|
SYTRD: |
|
|
|
ORMTR: |
|
||
|
|
|
Full→Tridiagonal |
|
|
|
Accum. QXT |
|
|||
n |
|
b |
1 core |
|
4 cores |
8 cores |
|
b |
1 core |
4 cores |
8 cores |
|
|
|
|
|
|
|
|
|
|
|
|
|
|
32 |
1.11 |
|
0.34 |
0.23 |
|
128 |
1.22 |
0.41 |
0.27 |
2048 |
|
64 |
1.11 |
|
0.35 |
0.23 |
|
192 |
1.23 |
0.41 |
0.28 |
|
|
96 |
1.11 |
|
0.36 |
0.25 |
|
256 |
1.27 |
0.41 |
0.27 |
|
|
|
|
|
|
|
|
|
|
|
|
|
|
32 |
40.4 |
|
11.4 |
9.2 |
|
128 |
28.8 |
8.60 |
5.20 |
6144 |
|
64 |
39.9 |
|
11.3 |
8.4 |
|
192 |
28.2 |
8.32 |
5.09 |
|
|
96 |
40.1 |
|
11.3 |
10.4 |
|
256 |
29.0 |
8.46 |
5.08 |
|
|
32 |
156.3 |
|
52.4 |
40.6 |
|
128 |
128.5 |
36.6 |
21.1 |
10240 |
|
64 |
152.5 |
|
51.9 |
40.5 |
|
192 |
127.4 |
35.9 |
21.1 |
|
|
96 |
152.6 |
|
52.1 |
40.9 |
|
256 |
126.4 |
35.3 |
21.9 |
|
|
32 |
2522.1 |
|
812.5 |
590.3 |
|
128 |
1767.1 |
488.1 |
275.2 |
24576 |
|
64 |
2453.6 |
|
796.8 |
600.9 |
|
192 |
1732.0 |
471.5 |
272.0 |
|
|
96 |
2444.4 |
|
795.0 |
582.4 |
|
256 |
1720.0 |
466.0 |
262.7 |
Table 4.4: Execution time (in seconds) for the LAPACK routine(s) on PECO.
analysis the routines that construct the orthogonal factor Q (SYGTR to build QB and SBRDT to accumulate Q := QB QT ) and compute QXT (GEMM) in the back-transform. Remember that while the computational cost of the first step is inversely proportional to the bandwidth, w, the cost of the second step is directly proportional to it. In other words, a larger bandwidth requires a smaller number of computations for the first step, transferring a larger part of the flops to the second step.
Table 4.5 displays results for these experiments. For the discussion, consider the following five cases:
1.Reduction of a dense matrix to banded form (Step 1). On the multi-core, the usage of a larger number of cores or the increase of the bandwidth results in a smaller execution time. The execution time on the GPU, on the other hand, is quite independent of w and outperforms the Intel-based architectures for all problem sizes except n = 2,048.
2.Reduction of banded matrix to tridiagonal form (Step 2). Using more than a single core yields no gain. As expected, a larger value of w translates into a longer execution time of this step.
3.Building the orthogonal factor resulting from Case 1 (Step 1). On the Intel cores, the execution time and parallelism of routine SYGTR is quite similar to those of SYRDB discussed in Case 1. Compared with the results obtained on 8 cores of PECO, the GPU in this platform accelerates the execution by a considerable factor, between 2.5×–3×.
4.Accumulating the orthogonal transforms corresponding to Case 2 (Step 2). By far, this is the most expensive operation of the five cases in the Intel cores, though it exhibits a certain degree of parallelism, which helps in reducing its weight on the overall process. The speed-up attained by the GPU for the larger problem dimensions is impressive.
5.Back-transform. The cost of this operation is comparable to that in Case 3. The best results is always attained with 8 cores and the GPU yields a notable acceleration.
Note that a study needs to take into account that the choice of bandwidth cannot be done independently from other factors. Therefore, we delay further comments on the data in the previous tables to the next subsection. There, we elaborate on the optimal combination of the factors that determine the overall performance of this approach.
112

4.7. REDUCTION TO TRIDIAGONAL FORM ON THE GRAPHICS PROCESSOR
|
|
|
|
|
|
|
|
|
|
1st step (SYRDB): |
|
|
|
|
|
2nd step (SBRDT): |
|
|
||||||||||||||||
|
|
|
|
|
|
|
|
|
|
|
|
Full→Band |
|
|
|
|
|
Band→Tridiagonal |
|
|
||||||||||||||
|
|
|
n |
|
w |
|
1 core |
|
4 cores |
|
|
8 cores |
|
GPU |
|
|
1 core |
|
4 cores |
|
8 cores |
|
|
|||||||||||
|
|
|
|
|
|
|
|
|
|
|
|
|
|
|
|
|
|
|
|
|
|
|
|
|
|
|
|
|
|
|
|
|
|
|
|
|
|
|
|
32 |
|
0.89 |
|
0.34 |
0.23 |
|
0.21 |
|
|
0.37 |
|
|
|
1.64 |
|
|
1.72 |
|
|
||||||||||
|
|
2048 |
|
64 |
|
0.81 |
|
0.28 |
0.19 |
|
0.20 |
|
|
0.45 |
|
|
|
1.08 |
|
|
1.03 |
|
|
|||||||||||
|
|
|
|
|
96 |
|
0.80 |
|
0.27 |
0.19 |
|
0.22 |
|
|
0.57 |
|
|
|
0.90 |
|
|
0.91 |
|
|
||||||||||
|
|
|
|
|
|
|
|
|
|
|
|
|
|
|
|
|
|
|
|
|
|
|
|
|
|
|
|
|
|
|
|
|
||
|
|
|
|
|
32 |
|
23.6 |
|
8.3 |
5.2 |
|
2.78 |
|
|
3.48 |
|
|
14.93 |
|
17.1 |
|
|
||||||||||||
|
|
6144 |
|
64 |
|
20.8 |
|
6.2 |
3.7 |
|
2.27 |
|
|
4.88 |
|
|
|
9.92 |
|
10.1 |
|
|
||||||||||||
|
|
|
|
|
96 |
|
19.9 |
|
5.9 |
3.6 |
|
2.29 |
|
|
5.42 |
|
|
|
8.23 |
|
|
8.91 |
|
|
||||||||||
|
|
|
|
|
32 |
|
112.6 |
|
41.1 |
26.7 |
|
10.81 |
|
|
9.51 |
|
|
41.1 |
|
43.3 |
|
|
||||||||||||
|
|
10240 |
|
64 |
|
95.9 |
|
29.6 |
18.7 |
|
9.72 |
|
|
11.7 |
|
|
27.5 |
|
26.3 |
|
|
|||||||||||||
|
|
|
|
|
96 |
|
90.9 |
|
27.3 |
16.1 |
|
10.39 |
|
|
15.1 |
|
|
23.1 |
|
25.3 |
|
|
||||||||||||
|
|
|
|
|
32 |
|
1589.9 |
|
569.0 |
354.3 |
|
112.6 |
|
|
54.2 |
|
237.3 |
|
258.0 |
|
|
|||||||||||||
|
|
24576 |
|
64 |
|
1330.2 |
|
404.3 |
235.5 |
|
99.3 |
|
|
72.9 |
|
159.2 |
|
157.7 |
|
|
||||||||||||||
|
|
|
|
|
96 |
|
1251.2 |
|
370.7 |
220.5 |
|
105.3 |
|
|
96.8 |
|
133.3 |
|
140.3 |
|
|
|||||||||||||
|
|
|
|
|
|
|
|
|
|
|
|
|
|
|
|
|
|
|
|
|
|
|
|
|
|
|
|
|
|
|
|
|||
|
|
|
|
|
|
|
|
|
|
|
|
|
|
|
|
|
|
|
|
|
|
|
|
|
|
|||||||||
|
|
|
|
|
1st step (SYGTR): |
|
|
|
|
|
|
|
2nd step (SBRDT): |
|
|
|
|
|
|
|
Back-transform |
|||||||||||||
|
|
|
|
|
|
|
|
|
|
|
|
|
|
|
|
|
|
|
|
|
|
|
|
|
|
|
|
|
|
|
|
(GEMM): |
||
|
|
|
|
|
|
|
|
Build QB |
|
|
|
|
|
|
Accum. Q := QB QT |
|
|
|
|
|
|
Comp. QXT |
||||||||||||
n |
w |
|
1 core |
|
|
4 cores |
8 cores |
GPU |
|
|
1 core |
|
4 cores |
|
8 cores |
|
GPU |
|
|
8 cores |
GPU |
|||||||||||||
|
|
|
|
|
|
|
|
|
|
|
|
|
|
|
|
|
|
|
|
|
|
|
|
|||||||||||
|
32 |
|
0.81 |
|
|
0.33 |
0.25 |
|
0.07 |
|
|
2.31 |
|
|
1.28 |
|
|
1.38 |
|
|
0.76 |
|
|
|
|
|
||||||||
2048 |
64 |
|
0.73 |
|
|
0.26 |
0.20 |
|
0.04 |
|
|
1.86 |
|
|
0.83 |
|
|
0.55 |
|
|
0.42 |
|
|
0.12 |
0.07 |
|||||||||
|
96 |
|
0.70 |
|
|
0.25 |
0.19 |
|
0.03 |
|
|
1.61 |
|
|
0.54 |
|
|
0.36 |
|
|
0.26 |
|
|
|
|
|
||||||||
|
32 |
|
21.2 |
|
|
|
7.35 |
7.04 |
|
1.68 |
|
|
65.4 |
|
|
33.0 |
|
|
35.7 |
|
|
|
6.24 |
|
|
|
|
|
||||||
6144 |
64 |
|
19.0 |
|
|
|
5.83 |
3.86 |
|
1.77 |
|
|
51.8 |
|
|
22.1 |
|
|
14.5 |
|
|
|
3.09 |
|
|
3.36 |
1.50 |
|||||||
|
96 |
|
18.3 |
|
|
|
5.62 |
3.67 |
|
1.75 |
|
|
44.3 |
|
|
14.5 |
|
|
|
9.5 |
|
|
|
1.74 |
|
|
|
|
|
|||||
|
|
|
|
|
|
|
|
|
|
|
|
|
|
|
|
|
|
|
|
|
|
|
|
|
|
|
|
|||||||
|
32 |
|
97.5 |
|
|
|
32.5 |
22.7 |
|
6.81 |
|
|
291.0 |
|
|
150.8 |
|
|
163.4 |
|
|
32.4 |
|
|
|
|
|
|||||||
10240 |
64 |
|
87.5 |
|
|
|
25.6 |
17.2 |
|
6.44 |
|
|
235.1 |
|
|
102.3 |
|
|
66.6 |
|
|
12.6 |
|
|
15.0 |
6.46 |
||||||||
|
96 |
|
84.1 |
|
|
|
24.7 |
16.5 |
|
5.61 |
|
|
203.1 |
|
|
67.2 |
|
|
43.9 |
|
|
|
6.27 |
|
|
|
|
|
||||||
|
32 |
|
1399.0 |
|
|
|
456.8 |
310.7 |
|
94.6 |
|
|
4149.5 |
|
|
2166.7 |
|
|
2403.4 |
|
|
101.8 |
|
|
|
|
|
|||||||
24576 |
64 |
|
1232.0 |
|
|
|
353.0 |
217.3 |
|
88.0 |
|
|
3390.2 |
|
|
1465.0 |
|
|
956.6 |
|
|
55.3 |
|
|
209.5 |
89.3 |
||||||||
|
96 |
|
1177.0 |
|
|
|
377.7 |
207.6 |
|
81.0 |
|
|
2898.4 |
|
|
969.9 |
|
|
638.8 |
|
|
30.9 |
|
|
|
|
|
|||||||
|
|
|
|
|
|
|
|
|
|
|
|
|
|
|
|
|
|
|
|
|
|
|
|
|
|
|
|
|
|
|
|
|
|
|
Table 4.5: Execution time (in seconds) for the SBR routines on PECO.
113