
Econometrics2011
.pdf
CHAPTER 4. THE ALGEBRA OF LEAST SQUARES |
73 |
Alternatively, equation (4.4) writes the projection coe¢ cient as an explicit function of the population moments Qxy and Qxx: Their moment estimators are the sample moments
|
|
1 |
n |
|
|
b |
|
|
|
Xi |
|
|
1 |
|
|||
|
n |
|
|||
Qxy |
= |
|
xiyi |
|
|
b |
|
n |
=1 |
|
|
|
|
|
Xi |
|
|
Q |
xx |
= |
|
xix0 |
: |
|
|
n |
=1 |
|
The moment estimator of replaces the population moments in (4.4) with the sample moments:
= Q 1Q |
|
1 |
1 n |
|
|
||||||
b |
b |
1 |
|
bn |
|
|
|
||||
|
|
xx |
xy |
! |
|
|
X |
! |
|||
|
|
|
|
|
Xi |
|
|
||||
= |
|
|
n |
=1 xixi0 |
|
n |
i=1 xiyi |
||||
= |
|
|
n |
xixi0! 1 |
n |
xiyi |
! |
|
|||
|
|
Xi |
|
X |
|
|
|||||
|
|
=1 |
|
i=1 |
|
|
which is identical with (4.7).
Least Squares Estimation
b
De…nition 4.3.1 The least-squares estimator is
b
= argmin Sn( )
2Rk
where
Sn( ) = n1 Xn yi x0i 2 i=1
and has the solution
= |
n |
xixi0! 1 |
n |
xiyi!: |
b |
Xi |
|
X |
|
|
=1 |
|
i=1 |
|
Adrien-Marie Legendre
The method of least-squares was …rst published in 1805 by the French mathematician Adrien-Marie Legendre (1752-1833). Legendre proposed least-squares as a solution to the algebraic problem of solving a system of equations when the number of equations exceeded the number of unknowns. This was a vexing and common problem in astronomical measurement. As viewed by Legendre, (4.1) is a set of n equations with k unknowns. As the equations cannot be solved exactly, Legendre’s goal was to select to make the set of errors as small as possible. He proposed the sum of squared error criterion, and derived the algebraic solution presented above. As he noted, the …rst-order conditions (4.6) is a system of k equations with k unknowns, which can be solved by “ordinary”methods. Hence the method became known as Ordinary Least Squares and to this day we still use the abbreviation OLS to refer to Legendre’s estimation method.
CHAPTER 4. THE ALGEBRA OF LEAST SQUARES |
74 |
4.4Illustration
We illustrate the least-squares estimator in practice with the data set used to generate the estimates from Chapter 3. This is the March 2009 Current Population Survey, which has extensive information on the U.S. population. This data set is described in more detail in Section ? For this illustration, we use the sub-sample of non-white married non-military female wages earners with 12 years potential work experience. This sub-sample has 61 observations. Let yi be log wages and xi be an intercept and years of education. Then
|
|
|
|
1 n |
|
|
= |
3:025 |
|
|
|
|
|||
|
|
|
|
|
X |
|
|
|
|
|
|
|
|||
|
|
|
|
n |
i=1 xiyi |
47:447 |
|
|
|||||||
and |
1 |
n |
|
|
|
|
1 |
|
15:426 |
|
|||||
|
|
|
X |
|
|
|
|
|
|
|
|
: |
|
||
|
|
n |
i=1 xixi0 = |
15:426 |
243 |
|
|||||||||
Thus |
|
|
|
|
|
|
|
|
|
|
|
|
|
|
|
b |
|
|
|
1 |
|
15:426 |
1 |
|
|
|
3:025 |
|
|||
|
|
|
|
|
|
|
|
|
|
|
|
|
|
||
|
= |
15:426 |
|
243 |
|
47:447 |
|||||||||
|
= |
|
0:626 |
: |
|
|
|
|
|
|
|
(4.8) |
|||
|
0:156 |
|
|
|
|
|
|
|
We often write the estimated equation using the format
\ |
(4.9) |
log(W age) = 0:626 + 0:156 education: |
An interpretation of the estimated equation is that each year of education is associated with an 16% increase in mean wages.
Equation (4.9) is called a bivariate regression as there are only two variables. A multivariate regression has two or more regressors, and allows a more detailed investigation. Let’s redo the example, but now including all levels of experience. This expanded sample includes 2454 observations. Including as regressors years of experience and its square (experience2=100) (we divide by 100 to simplify reporting), we obtain the estimates
\ |
2 |
(4.10) |
log(W age) = 1:06 + 0:116 education + 0:010 experience 0:014 experience =100: |
These estimates suggest a 12% increase in mean wages per year of education, holding experience constant.
4.5Least Squares Residuals
As a by-product of estimation, we de…ne the …tted or predicted value
0 b
y^i = xi
and the residual
0 b
e^i = yi y^i = yi xi :
Note that yi = y^i + e^i and
0 b
yi = xi + e^i:
(4.11)
(4.12)
We make a distinction between the error ei and the residual e^i: The error ei is unobservable while the residual e^i is a by-product of estimation. These two variables are frequently mislabeled, which can cause confusion.

CHAPTER 4. THE ALGEBRA OF LEAST SQUARES |
|
|
|
75 |
||||
Equation (4.6) implies that |
|
n |
|
|
|
|
|
|
|
|
|
|
|
|
|
|
|
|
|
Xi |
|
|
|
|
|
(4.13) |
|
|
xie^i = 0: |
|
|
|
|||
|
|
=1 |
|
|
|
|
|
|
To see this by a direct calculation, using (4.11) and (4.7), |
|
|
|
|
||||
n |
n |
|
|
|
|
|
|
|
X |
X |
n |
b |
|
|
|
|
|
|
n |
|
|
|
|
|
||
i=1 xie^i = |
i=1 xi yi xi0 |
|
|
|
|
|
|
|
|
Xi |
X |
|
|
|
|
|
|
= |
|
xiyi xixi0 |
|
|
|
|
|
|
|
=1 |
i=1 |
b |
|
xixi0! |
|
|
! |
|
n |
n |
n |
1 |
n |
|||
= |
Xi |
xiyi xixi0 |
X |
|
xiyi |
|||
|
X |
|
|
|
X |
|
||
|
=1 |
i=1 |
|
i=1 |
|
|
i=1 |
|
|
n |
n |
|
|
|
|
|
|
= |
Xxiyi Xxiyi |
|
|
|
|
|
||
|
i=1 |
i=1 |
|
|
|
|
|
|
= |
0: |
|
|
|
|
|
|
|
When xi contains a constant, an implication of (4.13) is
1 Xn
n i=1
e^i = 0:
Thus the residuals have a sample mean of zero and the sample correlation between the regressors and the residual is zero. These are algebraic results, and hold true for all linear regression estimates.
Given the residuals, we can construct an estimator for 2 = Ee2i :
|
1 |
Xi |
|
|
|
^2 = |
|
|
e^2 |
: |
(4.14) |
|
n |
|
i |
|
|
|
=1 |
|
|
|
4.6Model in Matrix Notation
For many purposes, including computation, it is convenient to write the model and statistics in matrix notation. The linear equation (3.24) is a system of n equations, one for each observation. We can stack these n equations together as
|
|
|
|
y1 |
= |
x10 + e1 |
|
|
|
|
||
|
|
|
|
y2 |
= |
x20 + e2 |
|
|
|
|
||
|
|
|
|
|
|
. |
|
|
|
|
|
|
|
|
|
|
|
|
. |
|
|
|
|
|
|
|
|
|
|
|
|
. |
|
|
|
|
|
|
|
|
|
|
yn = xn0 + en: |
|
|
|
|
||||
Now de…ne |
0 y2 |
1 |
|
|
0 x20 |
1 |
0 e2 |
1 |
|
|||
|
y1 |
|
|
|
x10 |
|
e1 |
|
|
|||
|
B y |
n |
C |
|
|
B x |
n0 |
C |
B e |
n |
C |
: |
|
B |
C |
|
|
B |
C |
B |
C |
||||
|
y = B ... |
C |
; X = B ... |
C; |
e = B ... |
C |
||||||
|
@ |
|
A |
|
|
@ |
|
A |
@ |
|
A |
|
Observe that y and e are n 1 vectors, and X is an n k matrix. Then the system of n equations can be compactly written in the single equation
y = X + e: |
(4.15) |

CHAPTER 4. THE ALGEBRA OF LEAST SQUARES |
76 |
Sample sums can also be written in matrix notation. For example
Xn
xix0i = X0X
i=1
Xn
xiyi = X0y:
i=1
Therefore |
X0X 1 X0y : |
= |
|
b |
|
The matrix version of (4.12) and estimated version of (4.15) is
b
y = X + e^;
or equivalently the residual vector is
b e^ = y X :
Using the residual vector, we can write (4.13) as
X0e^ = 0
and the error variance estimator (4.14) as
^2 = n 1e^0e^
(4.16)
(4.18)
(4.19)
Using matrix notation we have simple expressions for most estimators. This is particularly convenient for computer programming, as most languages allow matrix notation and manipulation.
Important Matrix Expressions
y |
= |
X + e |
|
||
b |
|
|
|
1 |
|
|
= |
X0X |
X0y |
||
e^ |
= |
y |
X |
|
|
X0e^ |
= |
0 |
b |
|
|
^2 = |
n 1e^0e^: |
|
Early Use of Matrices
The earliest known treatment of the use of matrix methods to solve simultaneous systems is found in Chapter 8 of the Chinese text The Nine Chapters on the Mathematical Art, written by several generations of scholars from the 10th to 2nd century BCE.

CHAPTER 4. THE ALGEBRA OF LEAST SQUARES |
77 |
4.7Projection Matrix
De…ne the matrix |
P = X X0X 1 X0: |
Observe that |
|
|
P X = X X0X 1 X0X = X: |
This is a property of a projection matrix. More generally, for any matrix Z which can be written as Z = X for some matrix (we say that Z lies in the range space of X); then
P Z = P X = X X0X 1 X0X = X = Z:
As an important example, if we partition the matrix X into two matrices X1 and X2 so that
X = [X1 X2] ;
then P X1 = X1.
The matrix P is symmetric and idempotent1. To see that it is symmetric,
P 0 = X X0X 1 X0 0
=X0 0 X0X 1 0 (X)0
=X X0X 0 1 X0
=X (X)0 X0 0 1 X0
=P :
To establish that it is idempotent, the fact that P X = X implies that
P P = P X X0X 1 X0
=X X0X 1 X0
=P :
The matrix P has the property that it creates the …tted values in a least-squares regression:
P y = X X0X 1 X0y = X = y^:
Because of this property, P is also known as the “hat |
matrix”. |
b |
|
Another useful property is that the trace of P equals the number of columns of X |
|
tr P = k: |
(4.20) |
Indeed, |
|
tr P = tr X X0X 1 X0
=tr X0X 1 X0X
=tr (Ik)
=k:
1 A matrix P is symmetric if P 0 = P : A matrix P is idempotent if P P = P : See Appendix A.8.
CHAPTER 4. THE ALGEBRA OF LEAST SQUARES |
|
78 |
|
(See Appendix A.4 for de…nition and properties of the trace operator.) |
|
||
The i’th diagonal element of P = X (X0X) 1 X0 |
is |
|
|
hii = x0 X0X 1 xi |
(4.21) |
||
which is called the leverage of the i’th observation.i |
The |
hii take values in [0; 1] and sum to k |
|
n |
|
|
|
Xi |
|
|
(4.22) |
hii = k |
|
||
=1 |
|
|
|
(See Exercise 4.8). |
|
|
|
4.8 Orthogonal Projection
De…ne
M= In P
= In X X0X 1 X0
where In is the n n identity matrix. Note that
MX = (In P ) X = X P X = X X = 0:
Thus M and X are orthogonal. We call M an orthogonal projection matrix or an annihilator matrix due to the property that for any matrix Z in the range space of X then
MZ = Z P Z = 0:
For example, MX1 = 0 for any subcomponent X1 of X, and MP = 0:
The orthogonal projection matrix M has many similar properties with P , including that M is
symmetric (M0 = M) and idempotent (MM = M). Similarly to (4.20) we can calculate |
|
tr M = n k: |
(4.23) |
While P creates …tted values, M creates least-squares residuals:
My = |
y P y |
= y |
= e^: |
(4.24) |
|
|
Xb |
|
Another way of writing (4.24) is
y = P y + My = y^ + e^:
This decomposition is orthogonal, that is
y^0e^ = (P y)0 (My) = y0P My = 0:
We can also use (4.24) to write an alternative expression for the residual vector. Substituting
y = X + e into e^ = My and using MX = 0 we …nd |
|
e^ = M (X + e) = Me: |
(4.25) |
which is free of dependence on the regression coe¢ cient . |
|
Another useful application of (4.24) is to the error variance estimator (4.19) |
|
^2 = n 1e^0e^
=n 1y0MMy
=n 1y0My;
the …nal equality since MM = M. Similarly using (4.25) we …nd
^2 = n 1e0Me:
CHAPTER 4. THE ALGEBRA OF LEAST SQUARES |
79 |
4.9 Regression Components
Partition |
|
|
|
|
X = [X1 |
X2 |
] |
|
|
and |
|
|
|
|
= |
1 |
: |
|
|
2 |
|
|
||
Then the regression model can be rewritten as |
|
|
|
|
y = X1 1 + X2 2 |
+ e: |
(4.26) |
The OLS estimator of = ( 01; 02)0 is obtained by regression of y on X = [X1 X2] and can be written as
|
y = X + e^ = X1 1 + X2 2 + e^: |
(4.27) |
||||
|
expressions for |
|
and |
: |
|
|
We are interested in algebraic |
b |
1 |
b |
2 |
b |
|
The algebra for the estimator is identical as that for the population coe¢ cients as presented in |
||||||||||||||||||||
Section 3.19. |
|
|
|
|
|
|
|
b |
|
|
|
|
b |
|
|
|
|
|||
Q |
and Q |
|
as |
|
|
|
|
|
|
|
|
|
|
|
|
|
|
|
|
|
Partition bxx |
bxy |
|
|
2 b |
|
b |
3 |
|
2 |
1 |
X10 X1 |
1 |
X10 X2 |
3 |
||||||
|
|
xx |
|
|
|
1 |
1 |
|||||||||||||
|
Q |
|
|
= |
Q11 |
Q12 |
= |
|
n |
|
n |
|||||||||
|
b |
|
|
|
4 |
|
|
5 |
|
6 |
n |
2 |
n |
2 |
7 |
|||||
|
|
|
|
|
Q21 |
Q22 |
|
6 |
|
|
|
X0 X1 |
|
|
X0 X2 |
7 |
||||
|
|
|
|
|
|
|
|
|
|
|
||||||||||
and similarly Qxy |
|
|
|
|
b |
|
b |
|
|
4 |
2 |
1 |
|
3 |
|
5 |
||||
|
|
|
|
|
|
|
2 |
b |
3 |
|
|
|
|
|||||||
|
|
|
|
|
xy |
|
|
|
|
|
|
|
||||||||
|
|
|
|
|
|
|
1 |
|
|
|
||||||||||
|
|
|
|
|
Q |
= |
4 |
Q1y |
5 |
= |
|
nX10 y |
: |
|
||||||
|
|
|
|
|
b |
|
b |
|
6 |
|
n 2 |
7 |
|
|
||||||
|
|
|
|
|
|
|
|
|
|
4 |
|
|
|
5 |
|
|
||||
|
|
|
|
|
|
|
|
Q2y |
|
|
6 |
|
|
X0 y |
7 |
|
|
|||
|
|
|
|
|
|
|
|
|
|
|
|
|
|
By the partitioned
Qxx1 |
|
2 |
Q11 |
= |
b |
||
b |
|
4 |
Q21 |
where Q11 2 =bQ11 |
|||
Thus |
|
|
b |
b |
|
|
matrix inversion formula (A.4) |
|
|
1b |
|
|
|
|||||||||||
b |
|
3 |
|
= 2 |
b21 |
b22 |
3 = 2 |
|
|
|
1 |
||||||
Q |
12 |
|
1 |
|
Q11 |
Q12 |
|
|
|
Q 1 |
|
|
|||||
|
|
5 |
|
def |
6 |
|
|
|
7 6 |
|
|
|
|
|
|
|
|
Q22 |
|
|
Q Q |
|
|
Q22 |
|
1Q21Q11 |
|||||||||
b |
|
|
|
4 |
|
|
|
5 4 |
|
|
|
|
|
|
|
||
|
|
|
1 |
|
and Q |
b |
= Q |
Qb |
|
|
1 |
Q |
|
: |
|||
Q Q |
Q |
|
b |
|
|
Q |
12 |
||||||||||
b |
12 b |
22 b21 |
b22 1 |
|
b22 b21 b11 |
b |
|
|
|
b |
|
|
|
|
|
|
|
|
|
1 |
|
|
|
|
|
||
= |
|
2 ! |
|
|
|
|
|||
b |
" |
b |
|
Q1112 |
Q 1 |
||||
= |
|
Q 1 Q |
|
||||||
|
|
|
|
b |
|
b |
|
b |
|
|
|
|
22b1 |
|
21 |
11 |
|||
|
|
Q 1 |
Q |
1y |
2 |
||||
= |
|
|
|
11 |
2 |
|
|||
|
Q 1 |
Q |
|
|
! |
||||
|
|
b |
|
b |
2y 1 |
||||
|
|
b |
22 1 b |
Q2211 |
|
#" |
Q1112Q12Q221 |
|
|
b b |
b |
|
b |
|
|
b |
|
b1 |
b |
3 |
|
Q1112Q12Q221 |
(4.28) |
||||
|
b |
|
|
5 |
|
|
|
|
7 |
|
|
|
Q22 1 |
|
|
#
b
Q1y
b
Q2y
Now
Q11 2 = Q11 Q12Q221Q21 |
|
|
|
|
|
1 |
|
|
|
|||||||
|
|
1 |
|
|
1 |
|
1 |
|
|
|
1 |
|
|
|||
b |
= |
b |
X10 |
Xb1 |
b |
Xb10 X2 |
|
|
X20 |
X2 |
|
|
X20 |
X1 |
||
|
|
|
|
|
|
|||||||||||
|
n |
|
|
|
||||||||||||
|
|
n |
|
|
n |
|
|
n |
|
|||||||
|
= |
1 |
X0 |
M2X1 |
|
|
|
|
|
|
|
|
|
|
||
|
|
|
|
|
|
|
|
|
|
|
|
|||||
|
|
n 1 |
|
|
|
|
|
|
|
|
|
|
|
|
|

CHAPTER 4. THE ALGEBRA OF LEAST SQUARES |
|
|
|
|
|
|
|
|
where |
|
|
|
|
|
|
|
|
M2 = In X2 X20 X2 1 X120 |
|
|
|
|
||||
is the orthogonal projection matrix for X |
: Similarly Q |
= |
|
X0 |
M |
X |
|
where |
|
|
|||||||
2 |
b22 1 |
1 n |
2 |
1 |
|
2 |
|
|
M1 = In X1 X10 X1 |
X10 |
|
|
|
|
|||
is the orthogonal projection matrix for X1 |
: Also |
|
|
|
|
|
|
|
b
Q1y 2
b |
|
|
|
|
|
and Q |
= |
|
1 |
X0 |
M1y: |
|
|
||||
2y 1 |
|
n 2 |
|
Therefore
and
= |
Q1y Q12Q221Q2y |
|
|
1 1 |
|
|||||||||||
|
1 |
|
1 |
|
X |
|
1 X |
X |
|
|
||||||
= |
|
b |
X0 |
y b |
|
bX0 |
b |
|
|
0 |
|
|
|
|
X0 |
y |
|
|
|
|
|
|
|||||||||||
|
|
n 1 |
n 1 |
|
2 n 2 2 |
|
n 2 |
|
=n1 X01M2y
1 = X10 |
M2X1 1 X10 M2y |
b |
|
2 = X20 |
M1X2 1 X20 M1y |
: |
b |
|
|
These are algebraic expressions for the sub-coe¢ cient estimates from (4.27).
80
(4.29)
(4.30)
4.10Residual Regression
As …rst recognized by Ragnar Frisch, expressions (4.29) and (4.30) can be used to show that the least-squares estimators 1 and 2 can be found by a two-step regression procedure.
|
idempotent, M |
|
|
= M |
|
M |
|
|
and thus |
|
||||||||
Take (4.30). Since M1 isb |
|
b |
|
1 |
|
1 |
|
|
|
1 |
|
|
|
|
||||
= X0 M1X2 |
1 X0 M1y |
|
|
|||||||||||||||
b |
2 |
|
2 |
|
|
|
|
|
|
|
|
2 |
|
2 |
1 |
|
|
|
|
|
2 |
|
|
1 |
0 |
|
|
|
|
|
|
||||||
|
|
|
0 |
|
|
|
|
|
|
|
|
|||||||
|
|
|
|
|
|
|
|
|
1 |
|
|
X0 |
1 |
|
||||
|
|
= |
X0 M1M1X2 |
|
|
|
|
y |
||||||||||
|
|
f f |
|
|
|
f |
|
|
|
|
|
|
|
M |
M |
|||
|
|
= |
|
|
|
|
|
|
|
|
|
|
|
|
|
|||
where |
|
X2X2 X2e~1 |
|
|
|
|
||||||||||||
|
|
|
|
|
|
|
|
|
|
|
|
|
|
|
|
|
|
|
|
|
|
|
X |
|
= M |
X |
2 |
|
|
|
|
|
|
||||
|
|
|
|
f2 |
|
|
1 |
|
|
|
|
|
|
|
|
and
e~1 = M1y:
b
Thus the coe¢ cient estimate 2 is algebraically equal to the least-squares regression of e~1 on
f
X2: Notice that these two are y and X2, respectively, premultiplied by M1. But we know that multiplication by M1 is equivalent to creating least-squares residuals. Therefore e~1 is simply the
f
least-squares residual from a regression of y on X1; and the columns of X2 are the least-squares residuals from the regressions of the columns of X2 on X1:
We have proven the following theorem.
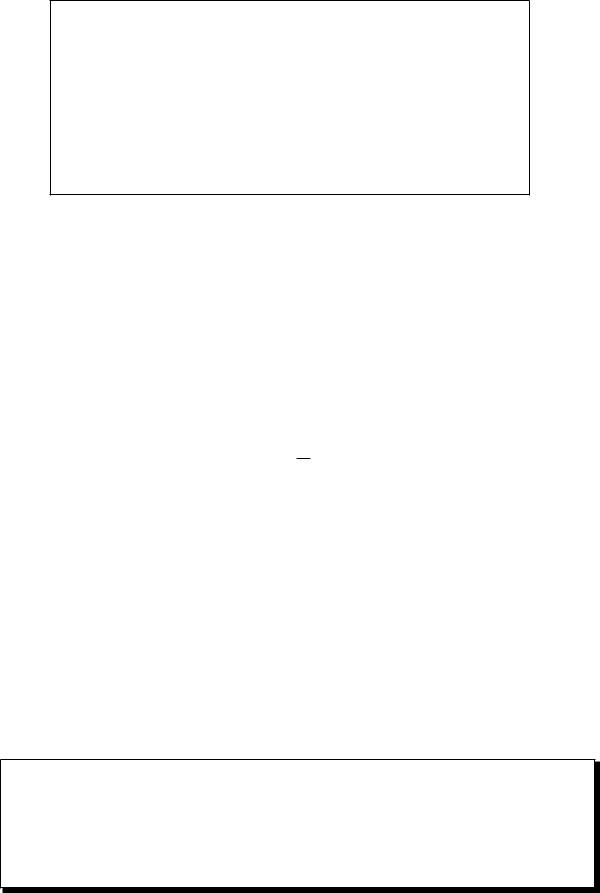
CHAPTER 4. THE ALGEBRA OF LEAST SQUARES |
81 |
Theorem 4.10.1 Frisch-Waugh-Lovell
In the model (4.26), the OLS estimator of 2 and the OLS residuals e^ may be equivalently computed by either the OLS regression (4.27) or via the following algorithm:
1. |
Regress y on X1; obtain residuals e~1; |
|
|
|
2. |
Regress X2 on X1; obtain residuals X2; |
|
|
|
|
f |
b |
|
and residuals e^: |
3. |
Regress e~1 on X2; obtain OLS |
estimates |
2 |
|
f |
|
In some contexts, the FWL theorem can be used to speed computation, but in most cases there is little computational advantage to using the two-step algorithm. Rather, the primary use is theoretical.
A common application of the FWL theorem, which you may have seen in an introductory econometrics course, is the demeaning formula for regression. Partition X = [X1 X2] where X1 is the vector of observed regressors and X2 = is a vector of ones . In this case,
Observe that |
|
|
|
|
M2 = I 0 1 0: |
|
||||||||||||||||||||||||
|
|
|
|
|
|
|
|
|
|
|
|
|
|
|
|
|
|
|
|
|
|
|
|
|
|
|||||
|
|
|
|
|
|
X |
= |
M X1 |
|
|
|
|
|
|
|
|
|
|
|
|
|
|
||||||||
|
|
|
|
|
|
f1 |
= X12 0 |
|
|
1 0X1 |
|
|||||||||||||||||||
|
|
|
|
|
|
|
|
|
= |
X1 |
X |
1 |
|
|
|
|
|
|
|
|
|
|||||||||
and |
|
|
|
|
|
|
|
|
|
|
|
|
|
|
|
|
|
|
|
|
|
|
|
|
|
|
||||
|
|
|
|
|
|
|
|
y~ = M2y |
|
|
|
|
|
|
|
|
|
|
|
|
|
|
||||||||
|
|
|
|
|
|
|
|
|
= |
y |
|
|
|
|
1 |
y |
|
|||||||||||||
|
|
|
|
|
|
|
|
|
= |
y |
y; |
0 |
|
|
|
|
0 |
|
|
|
|
|
|
|||||||
|
|
|
|
|
|
|
|
|
|
|
|
|
|
|
|
|
|
|
|
|
|
|
|
|
|
|
|
|
||
which are “demeaned”. The FWL theorem says that |
|
|
is the OLS estimate from a regression of |
|||||||||||||||||||||||||||
yi |
|
on x1i |
|
1 : |
|
|
|
|
|
|
|
|
|
|
|
|
|
|
|
b |
1 |
|
|
|
|
|
|
|
||
y |
x |
|
|
|
|
|
|
|
|
|
|
|
|
|
|
|
|
|
|
|
|
|
|
|
|
|||||
|
|
|
1 = |
n |
(x1i |
|
1) (x1i |
|
|
1)0! 1 |
|
|
|
|
n |
(x1i |
|
1) (yi |
|
)! |
: |
|||||||||
|
|
|
Xi |
x |
x |
|
|
|
|
|
x |
y |
||||||||||||||||||
|
|
|
b |
|
|
|
|
|
|
|
|
|
|
|
|
|
|
|
|
|
X |
|
||||||||
|
|
|
|
|
=1 |
|
|
|
|
|
|
|
|
|
|
|
|
|
|
|
|
|
i=1 |
|
|
|
|
|
|
Thus the OLS estimator for the slope coe¢ cients is a regression with demeaned data.
Ragnar Frisch
Ragnar Frisch (1895-1973) was co-winner with Jan Tinbergen of the …rst Nobel Memorial Prize in Economic Sciences in 1969 for their work in developing and applying dynamic models for the analysis of economic problems. Frisch made a number of foundational contributions to modern economics beyond the Frisch-Waugh-Lovell Theorem, including formalizing consumer theory, production theory, and business cycle theory.

CHAPTER 4. THE ALGEBRA OF LEAST SQUARES |
82 |
4.11Prediction Errors
The least-squares residual e^i are not true prediction errors, as they are constructed based on the full sample including yi. A proper prediction for yi should be based on estimates constructed only using the other observations. We can do this by de…ning the leave-one-out OLS estimator of as that obtained from the sample of n 1 observations excluding the i’th observation:
Here, X( i) and y( i) value for yi is
( i) = |
0 |
1 |
|
X |
xjxj0 |
1 1 0 |
1 |
|
xjyj |
1 |
||
n |
1 |
n |
1 |
|||||||||
b |
@ |
|
|
|
1A @ |
|
X |
A |
||||
|
|
|
j=i |
|
|
|
|
|
j=i |
|
||
|
X(0 |
|
|
6 |
|
|
|
|
|
6 |
|
|
= |
i)X( i) |
|
X( i)y( i): |
|
(4.31) |
are the data matrices omitting the i’th row. The leave-one-out predicted
0 b
y~i = xi ( i);
and the leave-one-out residual or prediction error is
e~i = yi y~i:
b
A convenient alternative expression for ( i) (derived below) is
|
|
( i) = (1 hii) 1 X0X 1 xie^i |
|
||||||
where hii are the leverage |
values as de…ned in (4.21). |
|
|
|
|
|
|||
|
b |
b |
|
|
|
|
|
|
|
Using (4.32) we can simplify the expression for the prediction error: |
|||||||||
e~i |
= yi xi0 ( i) |
|
1 |
|
X0X |
1 |
|
||
|
|
|
^ |
xi0 |
|
||||
|
|
|
|
|
|
xie^i |
|||
|
|
= yi xi0 b + (1 hii) |
|
|
|
= e^i + (1 hii) 1 hiie^i = (1 hii) 1 e^i:
(4.32)
(4.33)
A convenient feature of this expression is that it shows that computation of e~i is based on a simple linear operation, and does not really require n separate estimations.
One use of the prediction errors is to estimate the out-of-sample mean squared error
~2 = |
1 |
n |
e~i2 |
|
|
Xi |
|||
|
|
|
||
n =1 |
|
|||
1 |
n |
|
||
|
|
Xi |
(1 hii) 2 e^i2: |
|
= n |
||||
=1 |
||||
|
|
|
p
This is also known as the mean squared prediction error. Its square root ~ = ~2 is the prediction standard error.
Proof of Equation (4.32). The Sherman–Morrison formula (A.3) from Appendix A.5 states that for nonsingular A and vector b
A bb0 1 = A 1 + 1 b0A 1b 1 A 1bb0A 1:
This implies
X0X xix0i 1 = X0X 1 + (1 hii) 1 X0X 1 xix0i X0X 1